⚠️ 以下所有内容总结都来自于 大语言模型的能力,如有错误,仅供参考,谨慎使用
🔴 请注意:千万不要用于严肃的学术场景,只能用于论文阅读前的初筛!
💗 如果您觉得我们的项目对您有帮助 ChatPaperFree ,还请您给我们一些鼓励!⭐️ HuggingFace免费体验
2025-02-20 更新
AV-Flow: Transforming Text to Audio-Visual Human-like Interactions
Authors:Aggelina Chatziagapi, Louis-Philippe Morency, Hongyu Gong, Michael Zollhoefer, Dimitris Samaras, Alexander Richard
We introduce AV-Flow, an audio-visual generative model that animates photo-realistic 4D talking avatars given only text input. In contrast to prior work that assumes an existing speech signal, we synthesize speech and vision jointly. We demonstrate human-like speech synthesis, synchronized lip motion, lively facial expressions and head pose; all generated from just text characters. The core premise of our approach lies in the architecture of our two parallel diffusion transformers. Intermediate highway connections ensure communication between the audio and visual modalities, and thus, synchronized speech intonation and facial dynamics (e.g., eyebrow motion). Our model is trained with flow matching, leading to expressive results and fast inference. In case of dyadic conversations, AV-Flow produces an always-on avatar, that actively listens and reacts to the audio-visual input of a user. Through extensive experiments, we show that our method outperforms prior work, synthesizing natural-looking 4D talking avatars. Project page: https://aggelinacha.github.io/AV-Flow/
我们介绍了AV-Flow,这是一种视听生成模型,它只需要文本输入就能生成逼真的4D对话虚拟形象。与之前假设存在语音信号的先期工作不同,我们是联合合成语音和视觉。我们展示了类似人类的语音合成、同步的嘴唇动作、生动的面部表情和头部姿势;所有这些都仅由文本字符生成。我们的方法的核心在于两个并行扩散变压器的架构。中间的高速公路连接确保了音频和视觉模式之间的通信,因此,语音语调与面部动态(如眉毛动作)实现了同步。我们的模型采用流程匹配进行训练,从而实现了生动的表现和快速的推理。在双人对话的情况下,AV-Flow生成一个始终开启的虚拟形象,它积极聆听并反应用户的视听输入。通过大量实验,我们证明我们的方法优于以前的工作,可以生成逼真的4D对话虚拟形象。项目页面:https://aggelinacha.github.io/AV-Flow/
论文及项目相关链接
Summary
我们推出了AV-Flow,一种仅通过文本输入即可动画化生成写实4D对话角色的音频-视觉生成模型。与之前假设存在语音信号的早期工作不同,我们联合合成语音和视觉。我们实现了逼真的人声合成、同步的唇部动作、生动的面部表情和头部姿态;所有这些都仅通过文本字符生成。该方法的核心在于两个并行扩散变压器的架构,中间的高速通道连接确保了音频和视觉模式之间的通信,从而实现同步的语音语调以及面部动态(如眉毛动作)。我们的模型采用流程匹配进行训练,可实现生动的成果和快速推理。在对话场景中,AV-Flow可生成始终开启的化身,积极聆听并响应用户的视听输入。经过广泛实验验证,我们的方法优于早期工作,能够合成逼真的4D对话角色。
Key Takeaways
- AV-Flow是一个音频-视觉生成模型,可通过文本输入生成写实的4D对话角色。
- 该模型联合合成语音和视觉,无需预先存在的语音信号。
- AV-Flow可以同步实现人声合成、唇部动作、面部表情和头部姿态。
- 模型的核心在于其两个并行扩散变压器的架构,通过中间的高速通道连接实现音频和视觉模式的通信。
- AV-Flow模型采用流程匹配进行训练,以产生生动的结果和快速推理。
- 在对话场景中,AV-Flow生成的化身能够积极响应用户的视听输入。
- 经过实验验证,AV-Flow在合成逼真的4D对话角色方面优于早期的工作。
点此查看论文截图
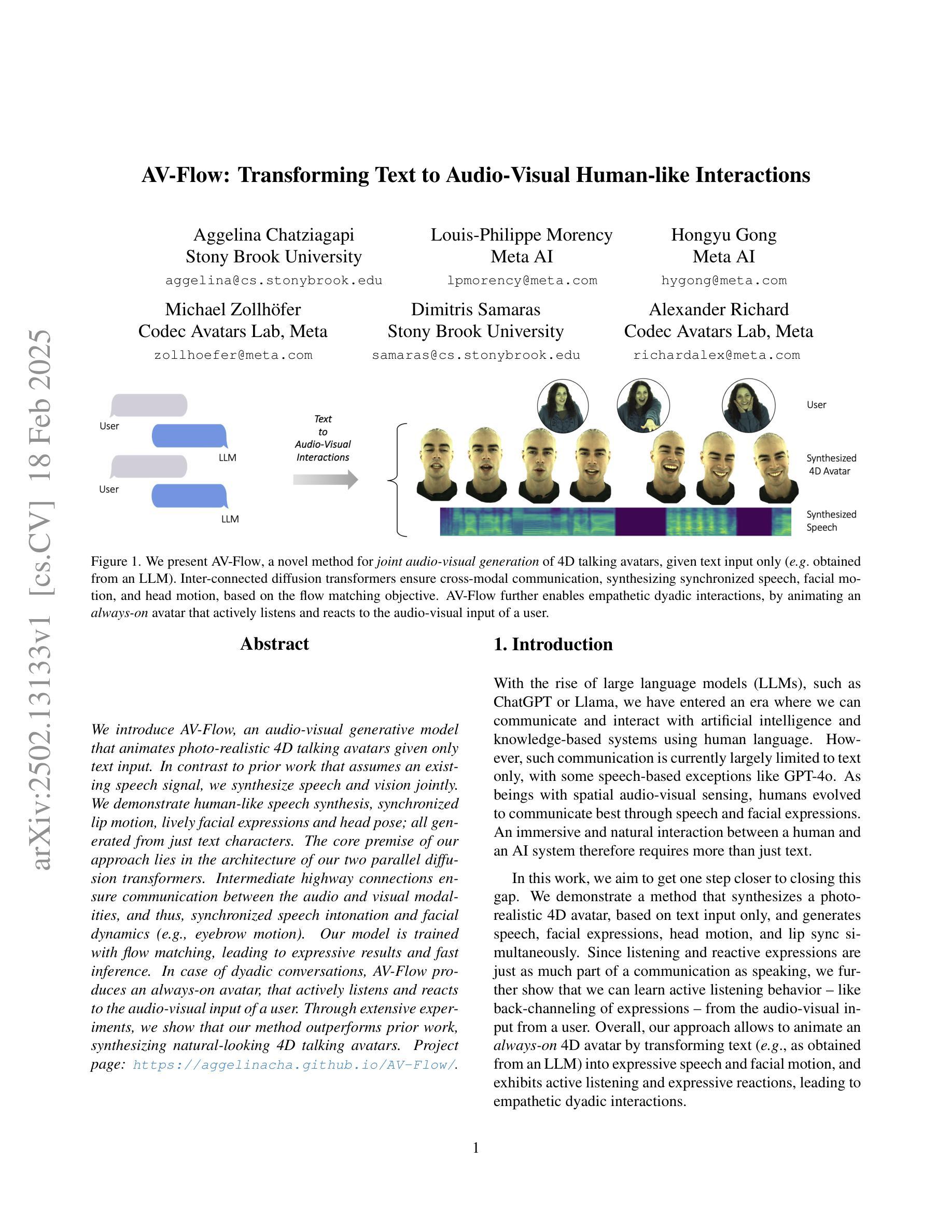
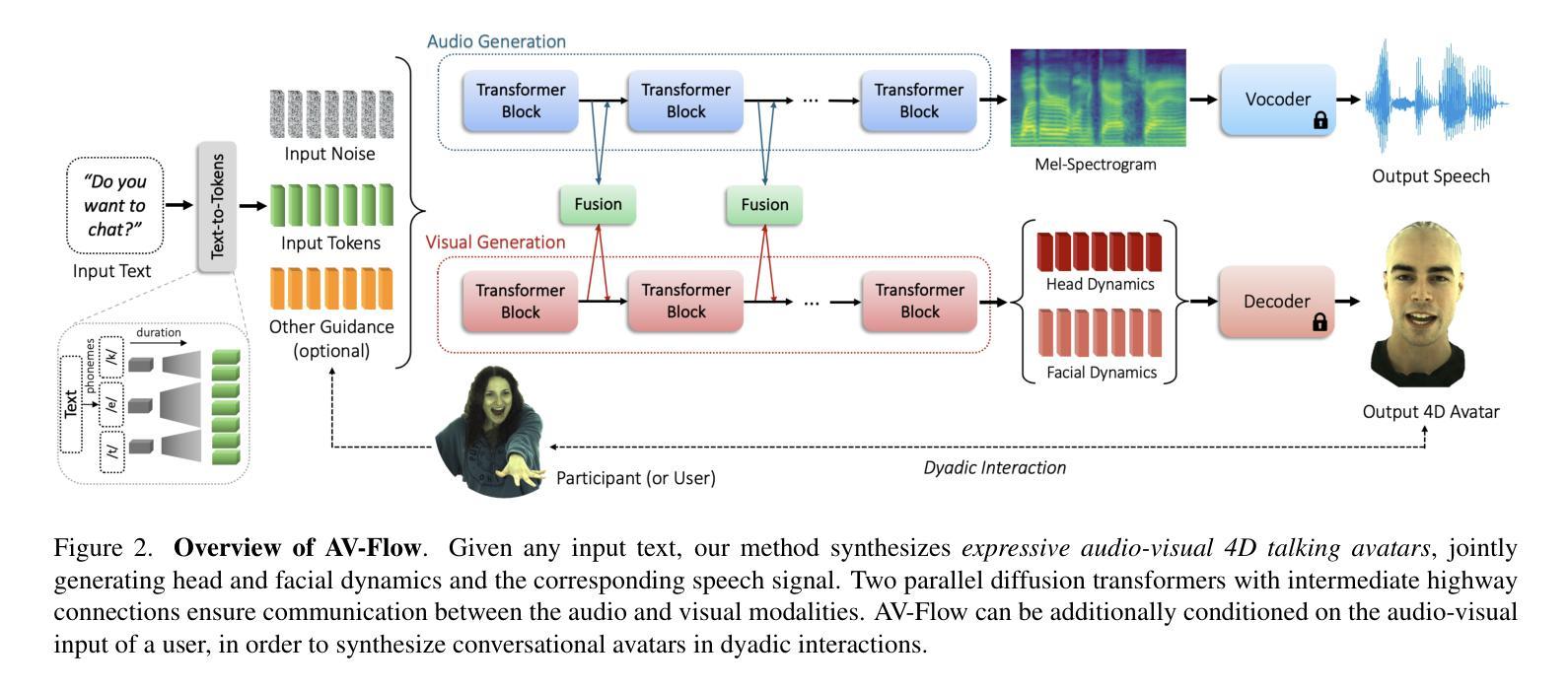
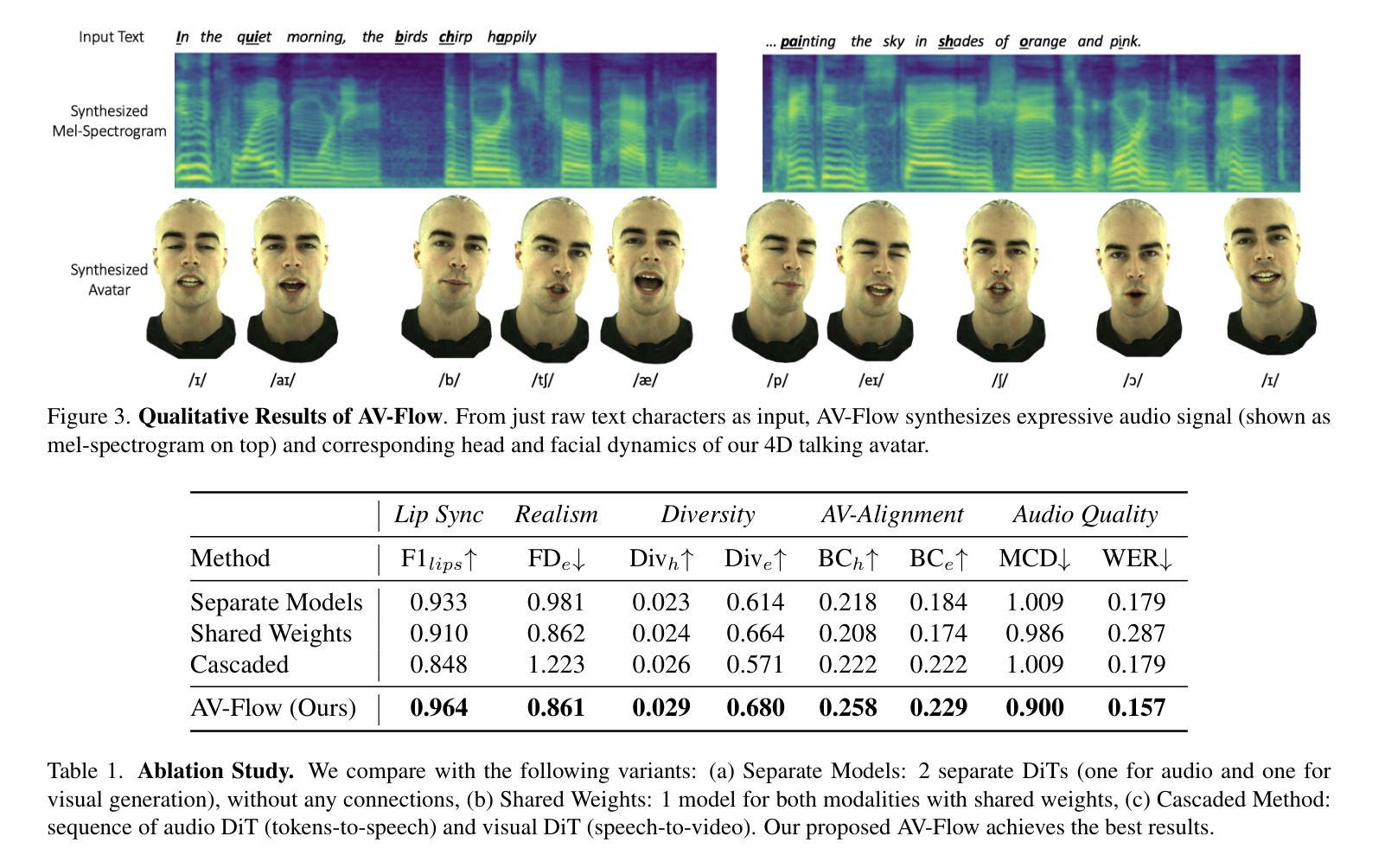
High-Fidelity Music Vocoder using Neural Audio Codecs
Authors:Luca A. Lanzendörfer, Florian Grötschla, Michael Ungersböck, Roger Wattenhofer
While neural vocoders have made significant progress in high-fidelity speech synthesis, their application on polyphonic music has remained underexplored. In this work, we propose DisCoder, a neural vocoder that leverages a generative adversarial encoder-decoder architecture informed by a neural audio codec to reconstruct high-fidelity 44.1 kHz audio from mel spectrograms. Our approach first transforms the mel spectrogram into a lower-dimensional representation aligned with the Descript Audio Codec (DAC) latent space before reconstructing it to an audio signal using a fine-tuned DAC decoder. DisCoder achieves state-of-the-art performance in music synthesis on several objective metrics and in a MUSHRA listening study. Our approach also shows competitive performance in speech synthesis, highlighting its potential as a universal vocoder.
尽管神经声码器在高保真语音合成方面取得了显著进展,但在复音音乐方面的应用仍被探索得很少。在这项工作中,我们提出了DisCoder,这是一种利用生成对抗编码器-解码器架构的神经网络声码器,该架构结合了神经网络音频编解码器,可以从梅尔频谱重建高保真44.1kHz音频。我们的方法首先将梅尔频谱转换为与描述音频编解码器(DAC)潜在空间对齐的低维表示,然后使用经过精细调整的DAC解码器将其重建为音频信号。DisCoder在多个客观指标和MUSHRA听力研究中的音乐合成方面达到了最新技术水平。我们的方法还在语音合成中表现出有竞争力的性能,突显了其作为通用声码器的潜力。
论文及项目相关链接
PDF Accepted at ICASSP 2025
Summary
神经网络vocoder在高保真语音合成方面取得了显著进展,但在多音音乐方面的应用仍然被忽视。本研究提出了DisCoder,这是一种利用生成对抗编码器-解码器架构的神经网络vocoder,借鉴神经音频编码器的信息,从梅尔频谱重建高保真44.1kHz音频。DisCoder在音乐合成方面达到了多项客观指标和MUSHRA听力测试的最先进水平,同时在语音合成方面表现也极具竞争力,凸显了其作为通用vocoder的潜力。
Key Takeaways
- 神经网络vocoder在高保真语音合成方面取得显著进展,但在多音音乐方面的应用仍待探索。
- DisCoder是一种新型的神经网络vocoder,采用生成对抗编码器-解码器架构。
- DisCoder借鉴神经音频编码器的信息,能够从梅尔频谱重建高保真音频。
- DisCoder在音乐合成方面达到了多项客观指标的最先进水平。
- DisCoder在MUSHRA听力测试中表现出卓越性能。
- DisCoder在语音合成方面也具有竞争力。
点此查看论文截图
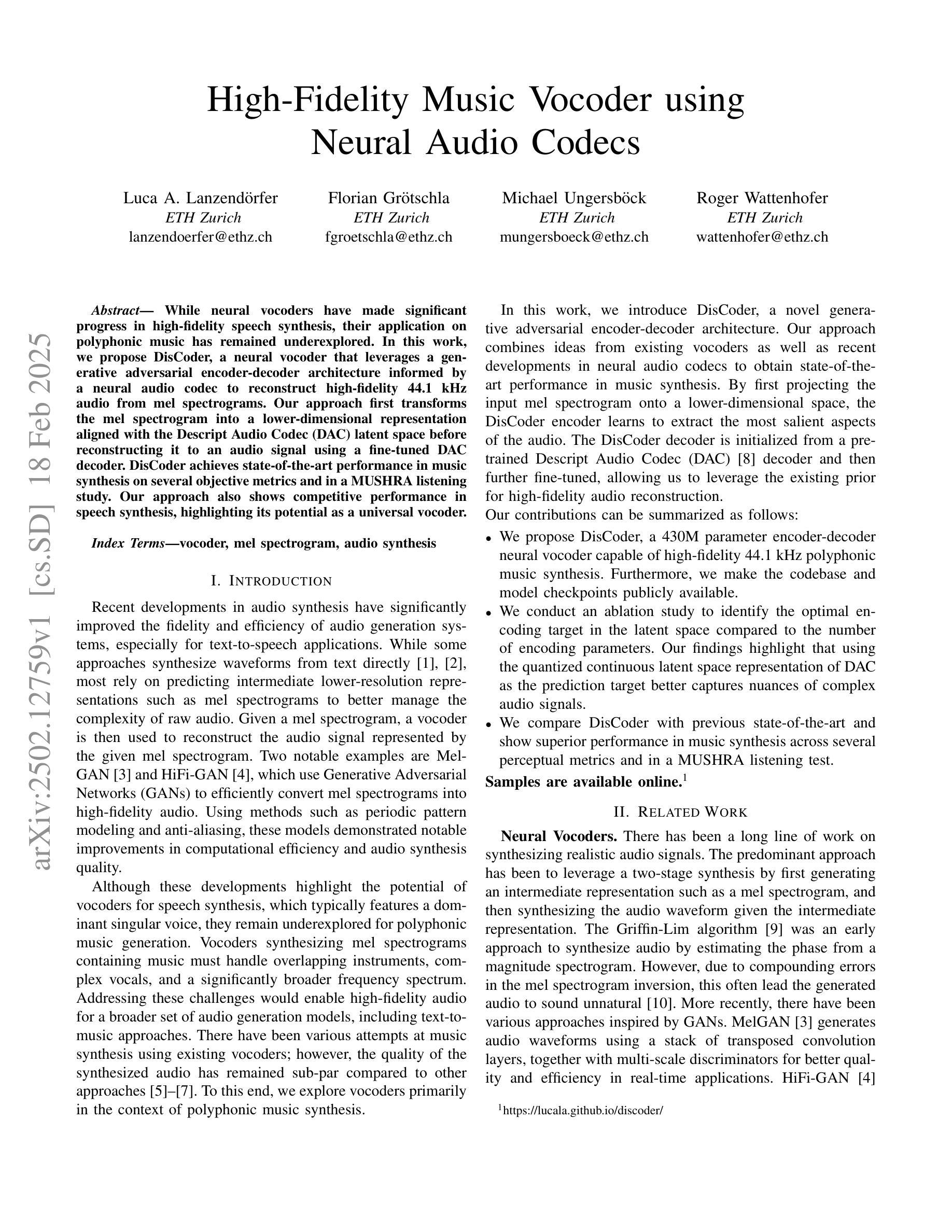
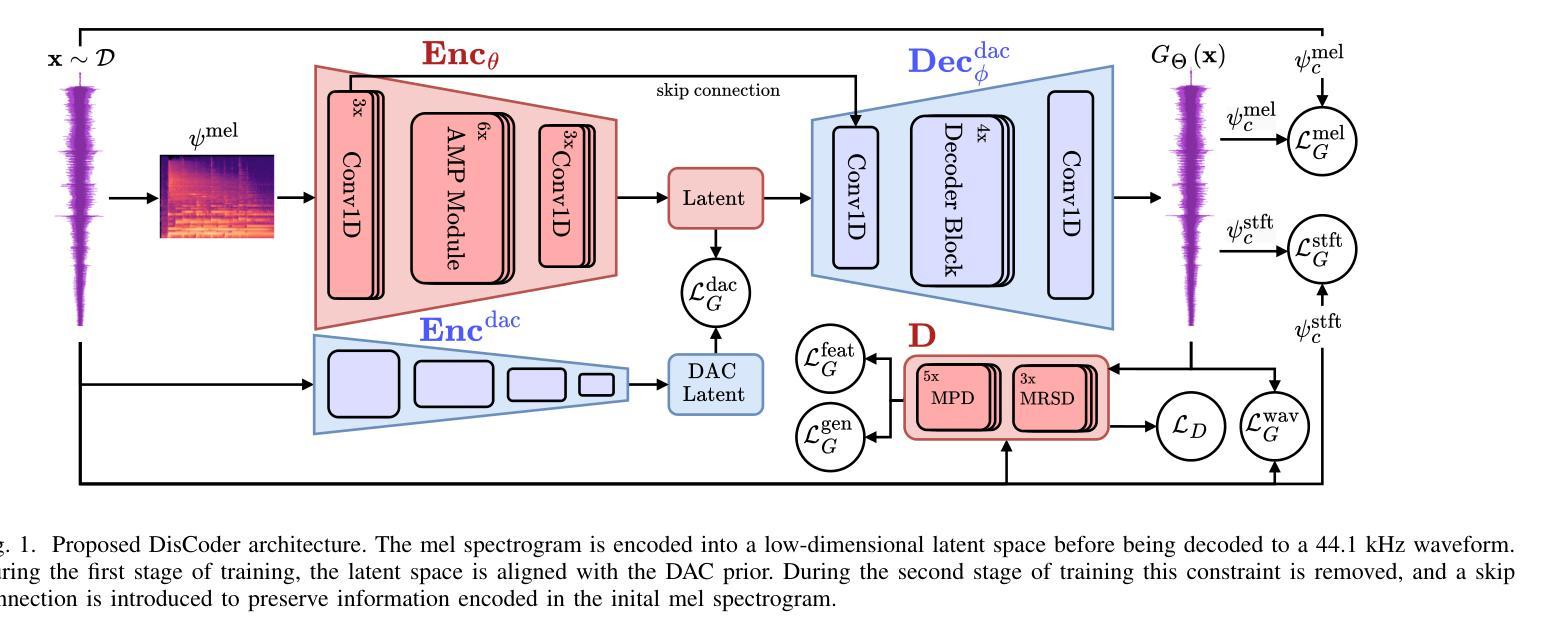
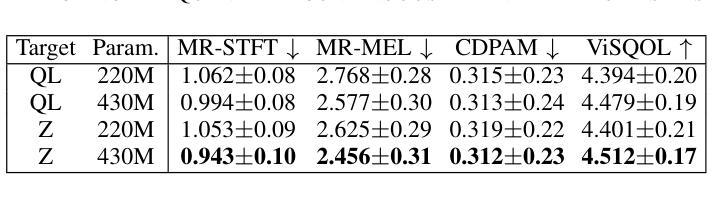
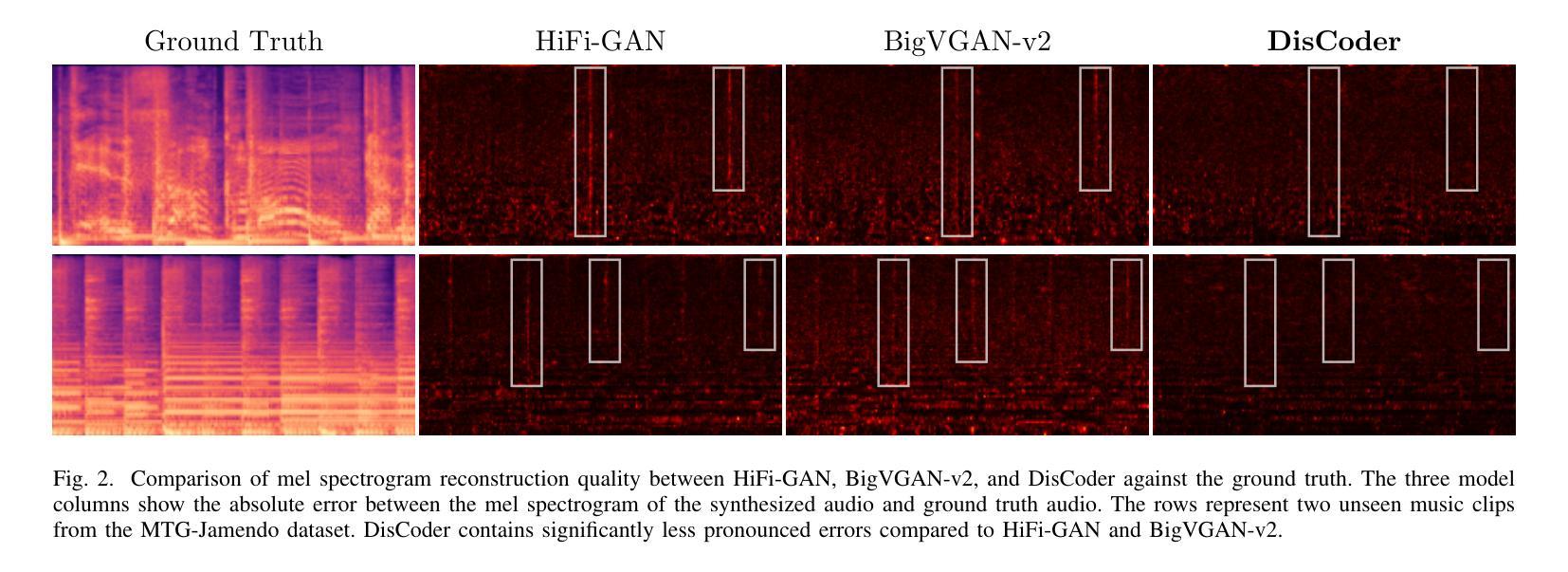

Step-Audio: Unified Understanding and Generation in Intelligent Speech Interaction
Authors:Ailin Huang, Boyong Wu, Bruce Wang, Chao Yan, Chen Hu, Chengli Feng, Fei Tian, Feiyu Shen, Jingbei Li, Mingrui Chen, Peng Liu, Ruihang Miao, Wang You, Xi Chen, Xuerui Yang, Yechang Huang, Yuxiang Zhang, Zheng Gong, Zixin Zhang, Hongyu Zhou, Jianjian Sun, Brian Li, Chengting Feng, Changyi Wan, Hanpeng Hu, Jianchang Wu, Jiangjie Zhen, Ranchen Ming, Song Yuan, Xuelin Zhang, Yu Zhou, Bingxin Li, Buyun Ma, Hongyuan Wang, Kang An, Wei Ji, Wen Li, Xuan Wen, Xiangwen Kong, Yuankai Ma, Yuanwei Liang, Yun Mou, Bahtiyar Ahmidi, Bin Wang, Bo Li, Changxin Miao, Chen Xu, Chenrun Wang, Dapeng Shi, Deshan Sun, Dingyuan Hu, Dula Sai, Enle Liu, Guanzhe Huang, Gulin Yan, Heng Wang, Haonan Jia, Haoyang Zhang, Jiahao Gong, Junjing Guo, Jiashuai Liu, Jiahong Liu, Jie Feng, Jie Wu, Jiaoren Wu, Jie Yang, Jinguo Wang, Jingyang Zhang, Junzhe Lin, Kaixiang Li, Lei Xia, Li Zhou, Liang Zhao, Longlong Gu, Mei Chen, Menglin Wu, Ming Li, Mingxiao Li, Mingliang Li, Mingyao Liang, Na Wang, Nie Hao, Qiling Wu, Qinyuan Tan, Ran Sun, Shuai Shuai, Shaoliang Pang, Shiliang Yang, Shuli Gao, Shanshan Yuan, Siqi Liu, Shihong Deng, Shilei Jiang, Sitong Liu, Tiancheng Cao, Tianyu Wang, Wenjin Deng, Wuxun Xie, Weipeng Ming, Wenqing He, Wen Sun, Xin Han, Xin Huang, Xiaomin Deng, Xiaojia Liu, Xin Wu, Xu Zhao, Yanan Wei, Yanbo Yu, Yang Cao, Yangguang Li, Yangzhen Ma, Yanming Xu, Yaoyu Wang, Yaqiang Shi, Yilei Wang, Yizhuang Zhou, Yinmin Zhong, Yang Zhang, Yaoben Wei, Yu Luo, Yuanwei Lu, Yuhe Yin, Yuchu Luo, Yuanhao Ding, Yuting Yan, Yaqi Dai, Yuxiang Yang, Zhe Xie, Zheng Ge, Zheng Sun, Zhewei Huang, Zhichao Chang, Zhisheng Guan, Zidong Yang, Zili Zhang, Binxing Jiao, Daxin Jiang, Heung-Yeung Shum, Jiansheng Chen, Jing Li, Shuchang Zhou, Xiangyu Zhang, Xinhao Zhang, Yibo Zhu
Real-time speech interaction, serving as a fundamental interface for human-machine collaboration, holds immense potential. However, current open-source models face limitations such as high costs in voice data collection, weakness in dynamic control, and limited intelligence. To address these challenges, this paper introduces Step-Audio, the first production-ready open-source solution. Key contributions include: 1) a 130B-parameter unified speech-text multi-modal model that achieves unified understanding and generation, with the Step-Audio-Chat version open-sourced; 2) a generative speech data engine that establishes an affordable voice cloning framework and produces the open-sourced lightweight Step-Audio-TTS-3B model through distillation; 3) an instruction-driven fine control system enabling dynamic adjustments across dialects, emotions, singing, and RAP; 4) an enhanced cognitive architecture augmented with tool calling and role-playing abilities to manage complex tasks effectively. Based on our new StepEval-Audio-360 evaluation benchmark, Step-Audio achieves state-of-the-art performance in human evaluations, especially in terms of instruction following. On open-source benchmarks like LLaMA Question, shows 9.3% average performance improvement, demonstrating our commitment to advancing the development of open-source multi-modal language technologies. Our code and models are available at https://github.com/stepfun-ai/Step-Audio.
实时语音交互作为人机交互的基本接口,具有巨大的潜力。然而,当前的开源模型面临诸如语音数据采集成本高、动态控制不足和智能有限的局限性。为了应对这些挑战,本文介绍了Step-Audio,这是首个投入生产的开源解决方案。主要贡献包括:1)一个拥有130B参数的统一语音文本多模态模型,实现了统一的理解和生成能力,其中Step-Audio-Chat版本已开源;2)一个生成式语音数据引擎,建立了一个经济实惠的语音克隆框架,并通过蒸馏技术产生了开源的轻量级Step-Audio-TTS-3B模型;3)一个指令驱动的精细控制系统,能够实现方言、情感、歌唱和RAP等方面的动态调整;4)一个增强的认知架构,增加了工具调用和角色扮演能力,以有效管理复杂任务。基于我们新的StepEval-Audio-360评估基准,Step-Audio在人文评估中达到了最先进的性能,尤其在执行指令方面。在LLaMA Question等开源基准测试中,平均性能提高了9.3%,这证明了我们推动开源多模态语言技术发展的承诺。我们的代码和模型可在https://github.com/stepfun-ai/Step-Audio获取。
论文及项目相关链接
Summary
本文介绍了一项名为Step-Audio的开源多模态语音技术解决方案,该技术解决了当前开源模型在语音交互方面的挑战。主要贡献包括:一个统一的语音文本多模态模型,一个生成式语音数据引擎,一个指令驱动的精细控制系统,以及一个增强认知架构。基于新的评估基准StepEval-Audio-360,Step-Audio在语音交互方面达到了最佳性能,展示了其开源多模态语言技术的先进性和提高能力。其代码和模型在GitHub上开源共享。
Key Takeaways
以下是该文本的主要观点或发现:
- Step-Audio是一个开源的语音交互解决方案,解决了当前模型的挑战。
- Step-Audio提供了一个统一的语音文本多模态模型,实现了理解和生成的一体化。
- Step-Audio引入了生成式语音数据引擎,建立了可负担的语音克隆框架并通过蒸馏技术产生轻量级的模型。
- Step-Audio具有指令驱动的精细控制系统,能够动态调整方言、情感、歌唱和RAP等。
- Step-Audio增强了认知架构,增加了工具调用和角色扮演能力以有效管理复杂任务。
点此查看论文截图
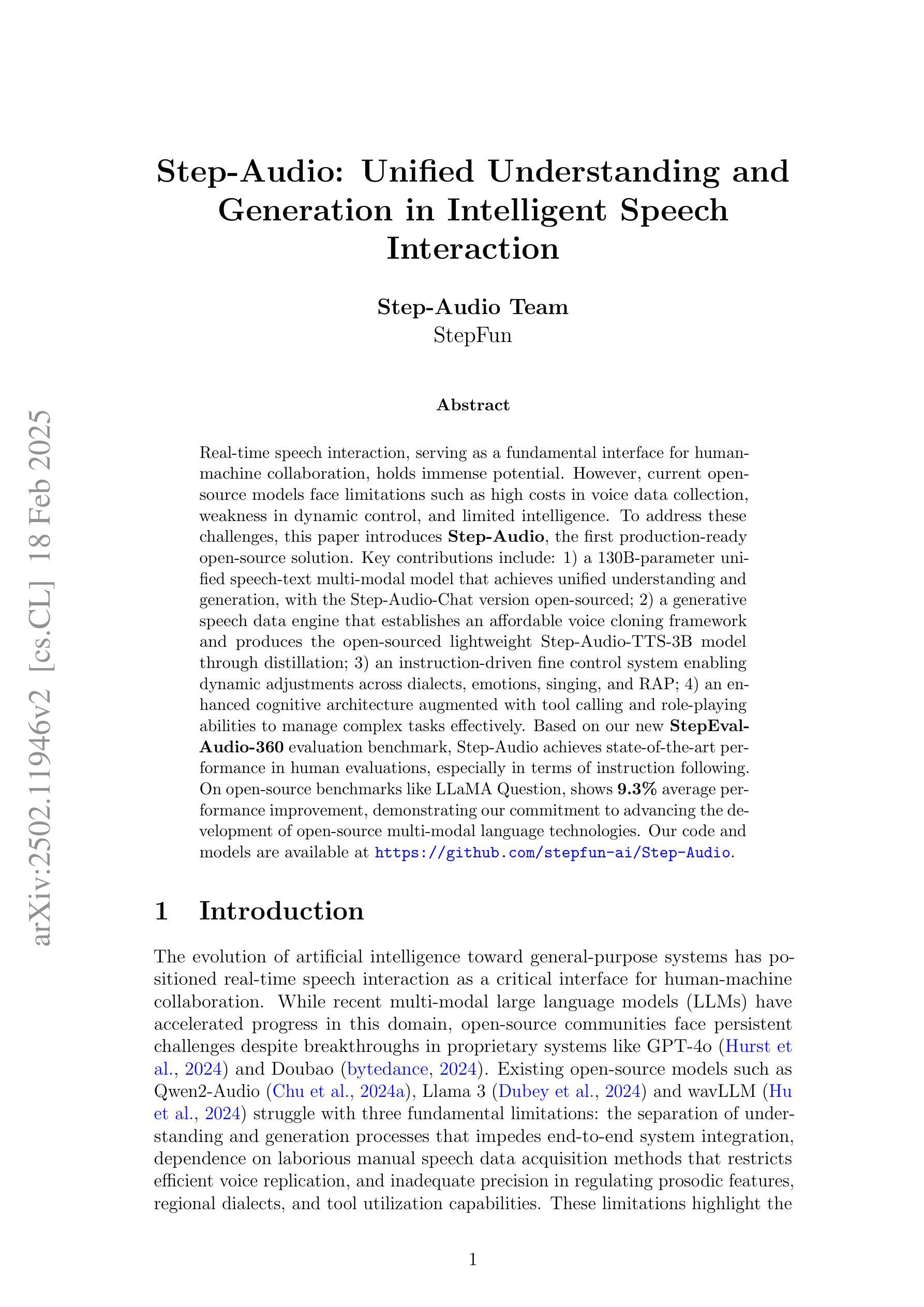
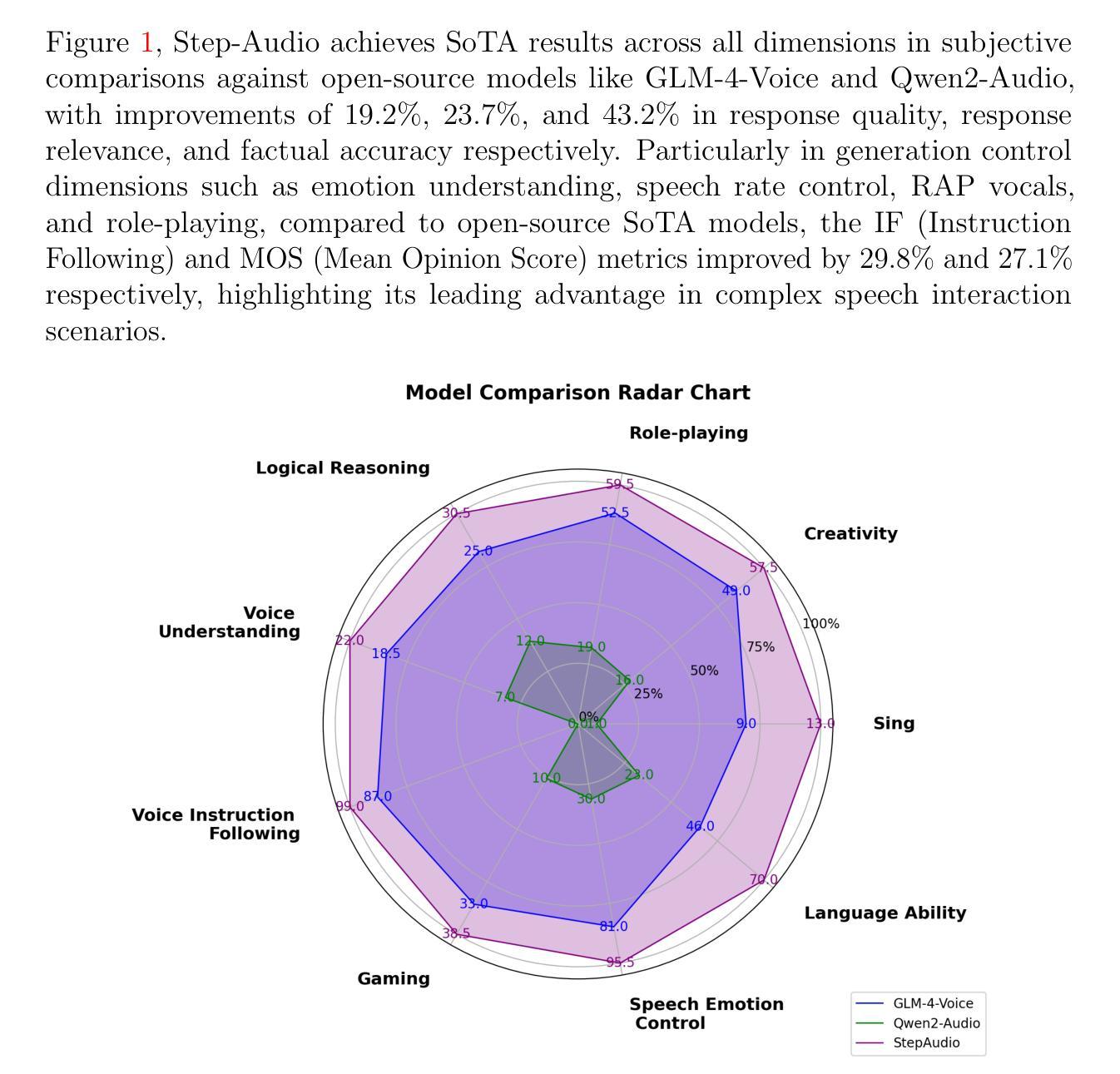
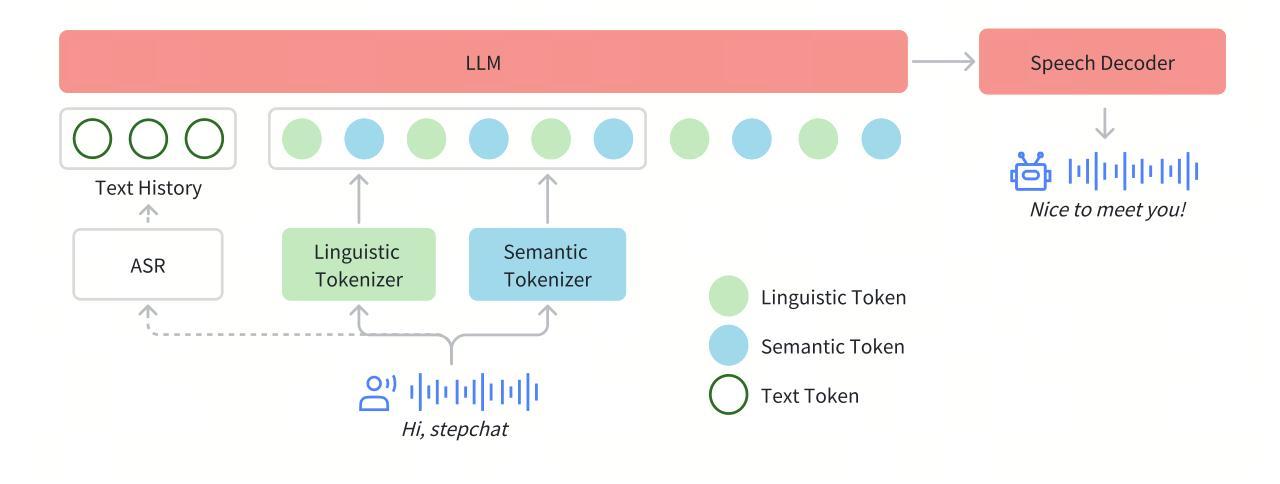