⚠️ 以下所有内容总结都来自于 大语言模型的能力,如有错误,仅供参考,谨慎使用
🔴 请注意:千万不要用于严肃的学术场景,只能用于论文阅读前的初筛!
💗 如果您觉得我们的项目对您有帮助 ChatPaperFree ,还请您给我们一些鼓励!⭐️ HuggingFace免费体验
2025-02-21 更新
Symmetrical Visual Contrastive Optimization: Aligning Vision-Language Models with Minimal Contrastive Images
Authors:Shengguang Wu, Fan-Yun Sun, Kaiyue Wen, Nick Haber
Recent studies have shown that Large Vision-Language Models (VLMs) tend to neglect image content and over-rely on language-model priors, resulting in errors in visually grounded tasks and hallucinations. We hypothesize that this issue arises because existing VLMs are not explicitly trained to generate texts that are accurately grounded in fine-grained image details. To enhance visual feedback during VLM training, we propose S-VCO (Symmetrical Visual Contrastive Optimization), a novel finetuning objective that steers the model toward capturing important visual details and aligning them with corresponding text tokens. To further facilitate this detailed alignment, we introduce MVC, a paired image-text dataset built by automatically filtering and augmenting visual counterfactual data to challenge the model with hard contrastive cases involving Minimal Visual Contrasts. Experiments show that our method consistently improves VLM performance across diverse benchmarks covering various abilities and domains, achieving up to a 22% reduction in hallucinations, and significant gains in vision-centric and general tasks. Notably, these improvements become increasingly pronounced in benchmarks with higher visual dependency. In short, S-VCO offers a significant enhancement of VLM’s visually-dependent task performance while retaining or even improving the model’s general abilities. We opensource our code at https://s-vco.github.io/
最近的研究表明,大型视觉语言模型(VLMs)往往忽略图像内容,过度依赖语言模型的先验知识,导致视觉定位任务出错和出现幻觉。我们假设这个问题是因为现有的VLMs并没有经过明确的训练来生成准确基于精细图像细节的文字。为了增强VLM训练过程中的视觉反馈,我们提出了S-VCO(对称视觉对比优化),这是一种新的微调目标,引导模型捕捉重要的视觉细节,并将它们与相应的文本标记对齐。为了进一步促进这种详细的对齐,我们引入了MVC,这是一个通过自动过滤和增强视觉反事实数据构建的配对图像文本数据集,以挑战涉及最小视觉对比的困难对比案例。实验表明,我们的方法在不同的基准测试上都能提高VLM的性能,涵盖各种能力和领域,幻觉减少了高达22%,在视觉为中心的任务和一般任务上都有显著的进步。值得注意的是,在视觉依赖性较高的基准测试中,这些改进尤为突出。简而言之,S-VCO在保留甚至提高模型一般能力的同时,显著提高VLM的视觉依赖任务性能。我们的代码已开源在https://s-vco.github.io/。
论文及项目相关链接
PDF Project Website: https://s-vco.github.io/
Summary
本文指出大型视觉语言模型(VLMs)在处理视觉任务时存在忽视图像内容、过度依赖语言模型先验的问题,导致错误和幻觉。为解决这一问题,提出了S-VCO(对称视觉对比优化)方法,通过新增训练目标来强化视觉反馈,使模型更关注重要视觉细节,并与文本标记对齐。同时,引入MVC数据集,通过自动筛选和增强视觉反事实数据,挑战涉及最小视觉对比的对比性案例。实验表明,该方法在不同领域和能力的基准测试中均提高了VLM性能,减少了幻觉,并在视觉依赖度高的测试中表现尤为突出。
Key Takeaways
- 大型视觉语言模型(VLMs)在处理视觉任务时存在忽视图像内容的问题。
- VLMs过度依赖语言模型先验,导致错误和幻觉。
- S-VCO方法通过新增训练目标来强化视觉反馈,提高模型对重要视觉细节的捕捉能力。
- S-VCO方法与文本标记对齐,提高模型性能。
- 引入MVC数据集,通过自动筛选和增强视觉反事实数据,提高模型对最小视觉对比的敏感性。
- 实验表明,S-VCO方法在不同基准测试中均提高了VLM性能。
点此查看论文截图
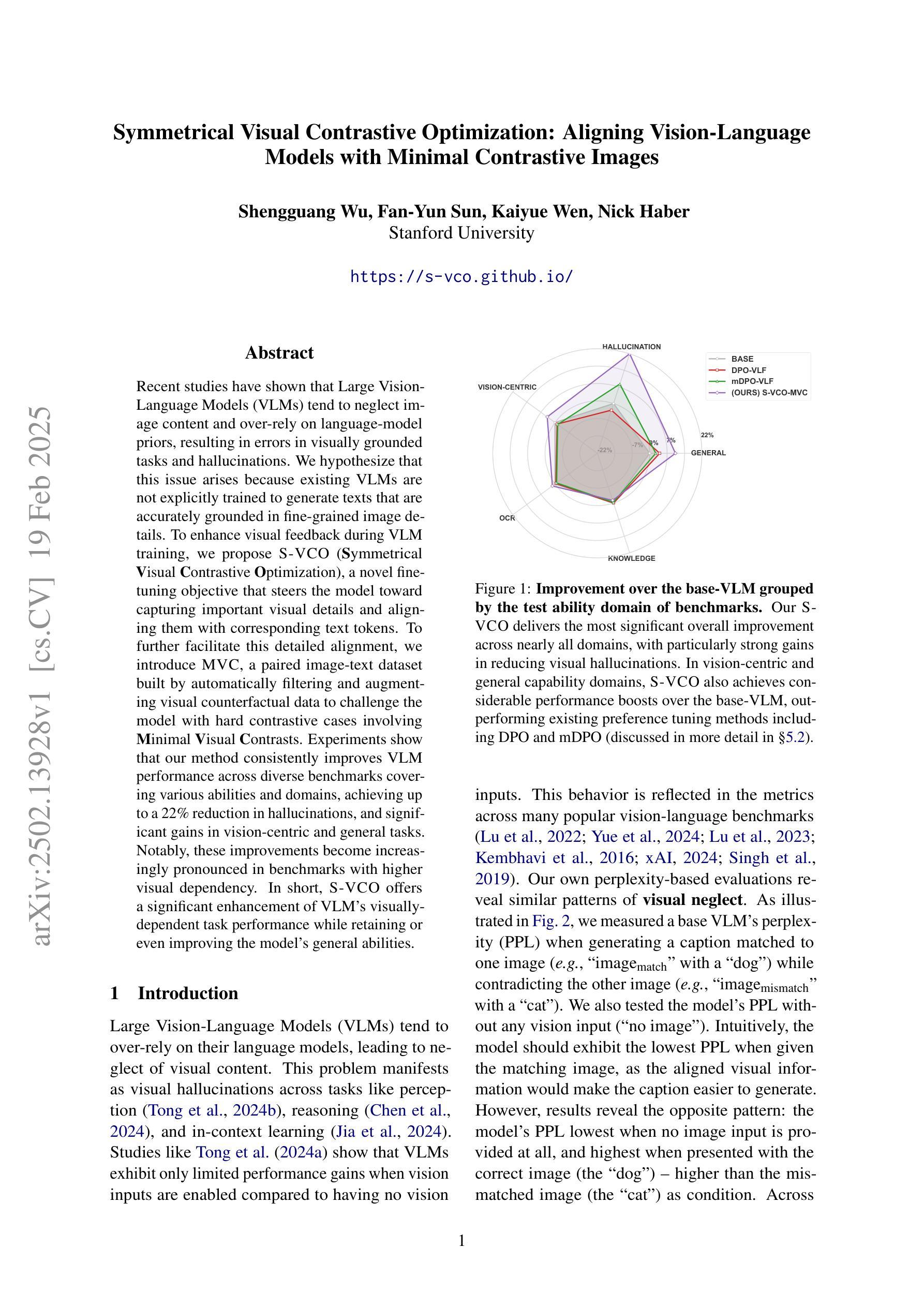
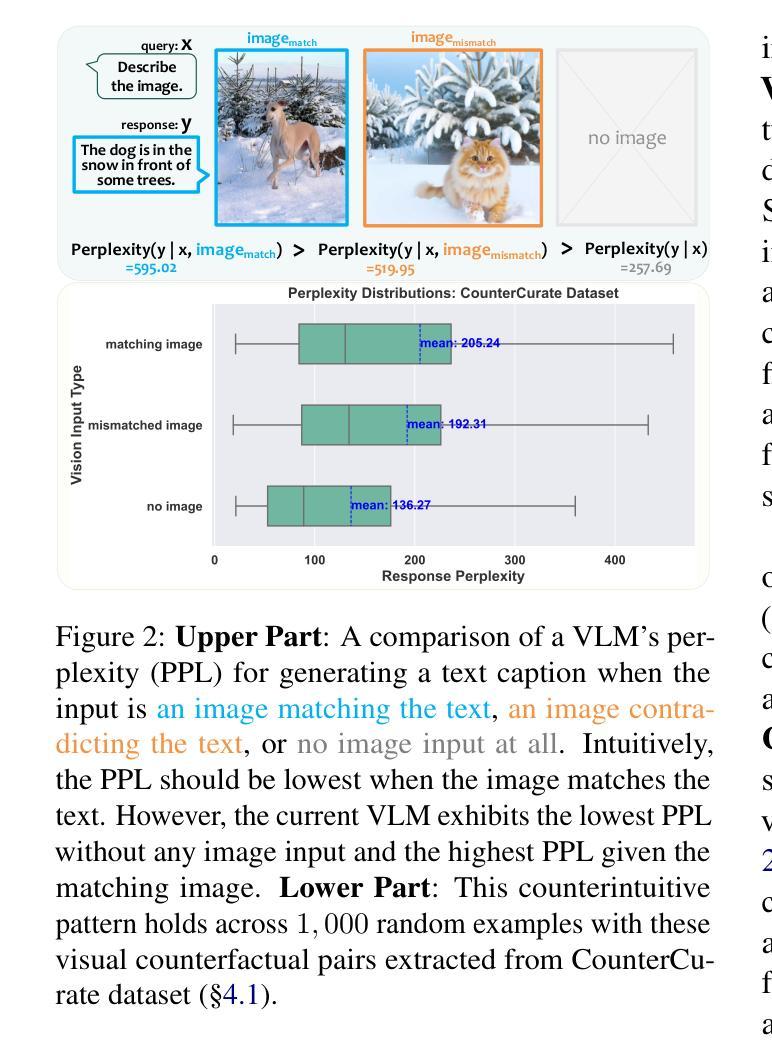
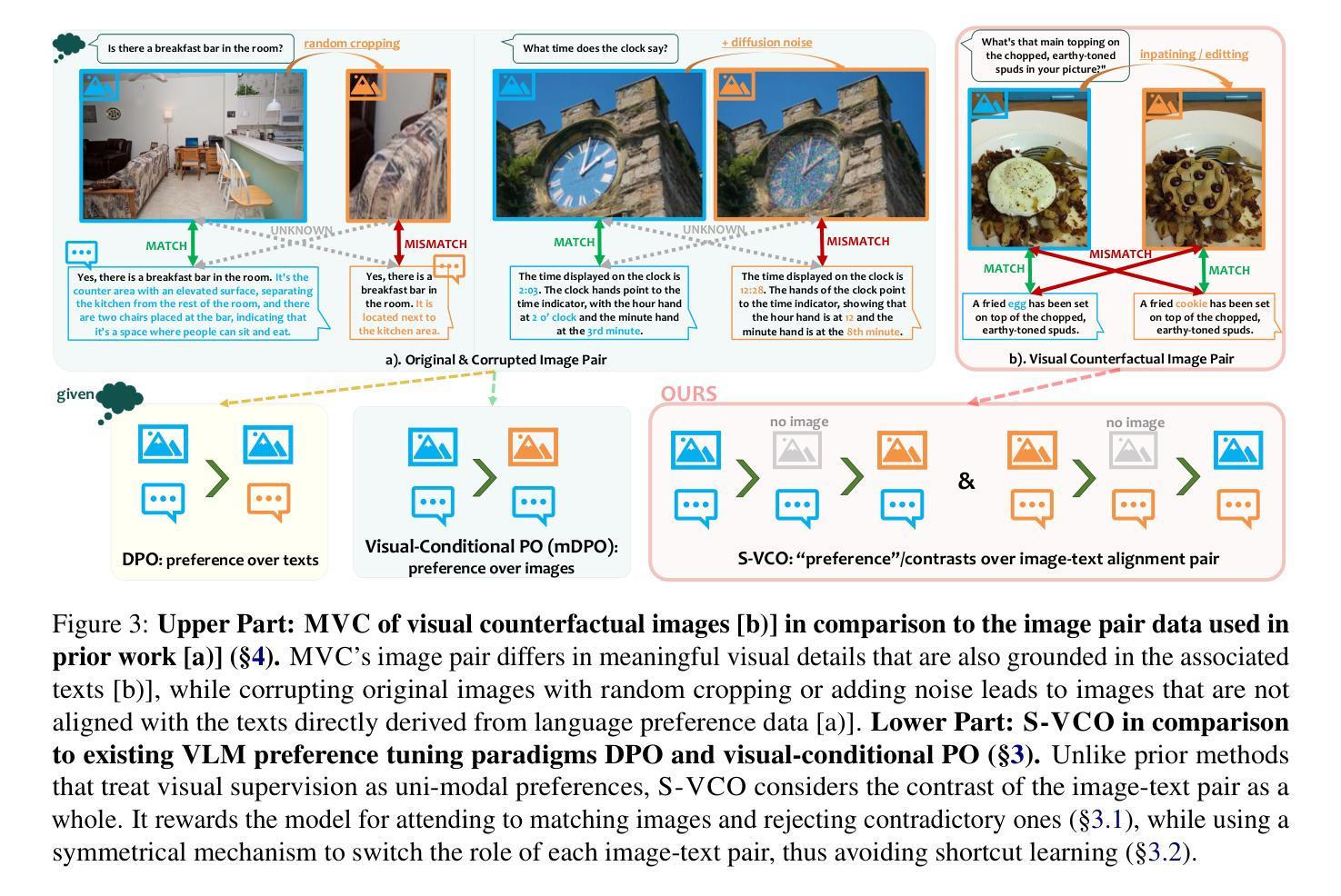
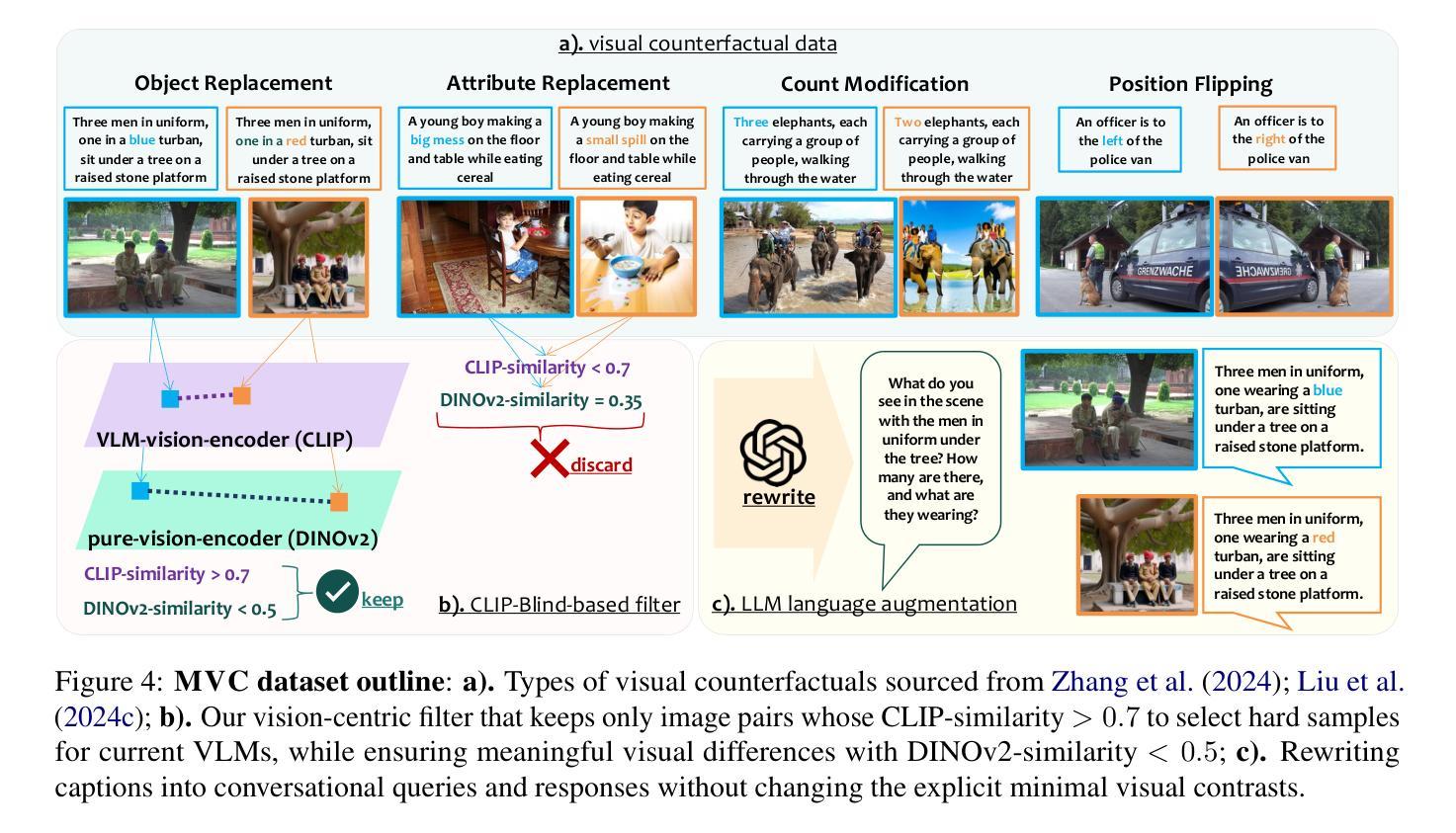