⚠️ 以下所有内容总结都来自于 大语言模型的能力,如有错误,仅供参考,谨慎使用
🔴 请注意:千万不要用于严肃的学术场景,只能用于论文阅读前的初筛!
💗 如果您觉得我们的项目对您有帮助 ChatPaperFree ,还请您给我们一些鼓励!⭐️ HuggingFace免费体验
2025-02-21 更新
GS-QA: Comprehensive Quality Assessment Benchmark for Gaussian Splatting View Synthesis
Authors:Pedro Martin, António Rodrigues, João Ascenso, Maria Paula Queluz
Gaussian Splatting (GS) offers a promising alternative to Neural Radiance Fields (NeRF) for real-time 3D scene rendering. Using a set of 3D Gaussians to represent complex geometry and appearance, GS achieves faster rendering times and reduced memory consumption compared to the neural network approach used in NeRF. However, quality assessment of GS-generated static content is not yet explored in-depth. This paper describes a subjective quality assessment study that aims to evaluate synthesized videos obtained with several static GS state-of-the-art methods. The methods were applied to diverse visual scenes, covering both 360-degree and forward-facing (FF) camera trajectories. Moreover, the performance of 18 objective quality metrics was analyzed using the scores resulting from the subjective study, providing insights into their strengths, limitations, and alignment with human perception. All videos and scores are made available providing a comprehensive database that can be used as benchmark on GS view synthesis and objective quality metrics.
高斯平铺(GS)为实时三维场景渲染提供了有前景的替代选择,即对神经网络辐射场(NeRF)的替代方案。使用一组三维高斯来代表复杂的几何形状和外观,GS实现了比神经网络方法更快的渲染速度和更低的内存消耗。然而,关于GS生成的静态内容的质量评估尚未进行深入研究。本文描述了一项主观质量评估研究,旨在评估通过几种最新静态GS技术获得合成视频的质量。这些方法应用于多种视觉场景,涵盖全景和正向(FF)相机轨迹。此外,使用主观研究的结果分析了客观质量指标的性能表现,并对其优势、局限性和与人类感知的一致性进行了洞察。所有视频和分数都可用,可为GS视图合成和客观质量指标提供一个基准数据库。
论文及项目相关链接
Summary
本文介绍了高斯Splatting(GS)作为一种实时3D场景渲染的神经网络辐射场(NeRF)替代方案。GS使用一组3D高斯分布来表示复杂的几何形状和外观,与NeRF中的神经网络方法相比,具有更快的渲染速度和更低的内存消耗。然而,对于GS生成的静态内容的质量评估尚未深入研究。本文描述了一项主观质量评估研究,旨在评估使用几种先进的静态GS方法获得的合成视频的质量。这些方法应用于各种视觉场景,包括360度和面向前的相机轨迹。此外,通过对主观研究结果的得分分析,对18项客观质量指标的性能进行了评估,揭示了它们的优点、局限性以及与人类感知的契合度。所有视频和分数均提供,为GS视图合成和客观质量指标提供了一个综合数据库,可供基准测试使用。
Key Takeaways
- 高斯Splatting(GS)提供了一种实时3D场景渲染的替代方案,使用3D高斯分布表示复杂几何和外观。
- GS相比神经网络方法NeRF,具有更快的渲染速度和更低的内存消耗。
- 目前对于GS生成的静态内容的质量评估尚未得到深入研究。
- 论文进行了一项主观质量评估研究,评估了使用多种先进的静态GS方法合成的视频质量。
- 这些方法应用于不同的视觉场景,包括360度和面向前的相机轨迹。
- 论文分析了18项客观质量指标的性能,揭示了它们的优点、局限性以及与人类感知的契合度。
点此查看论文截图
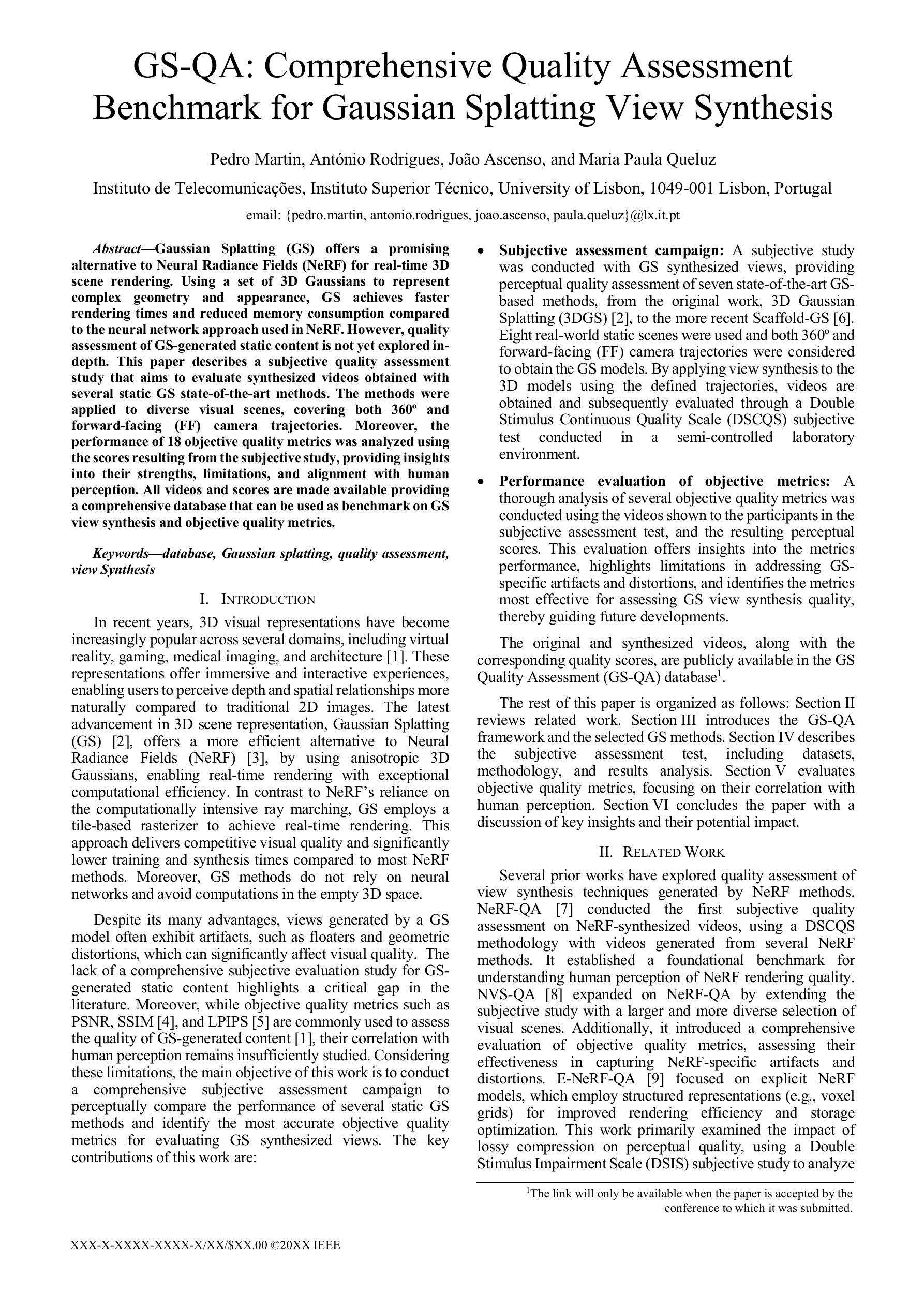
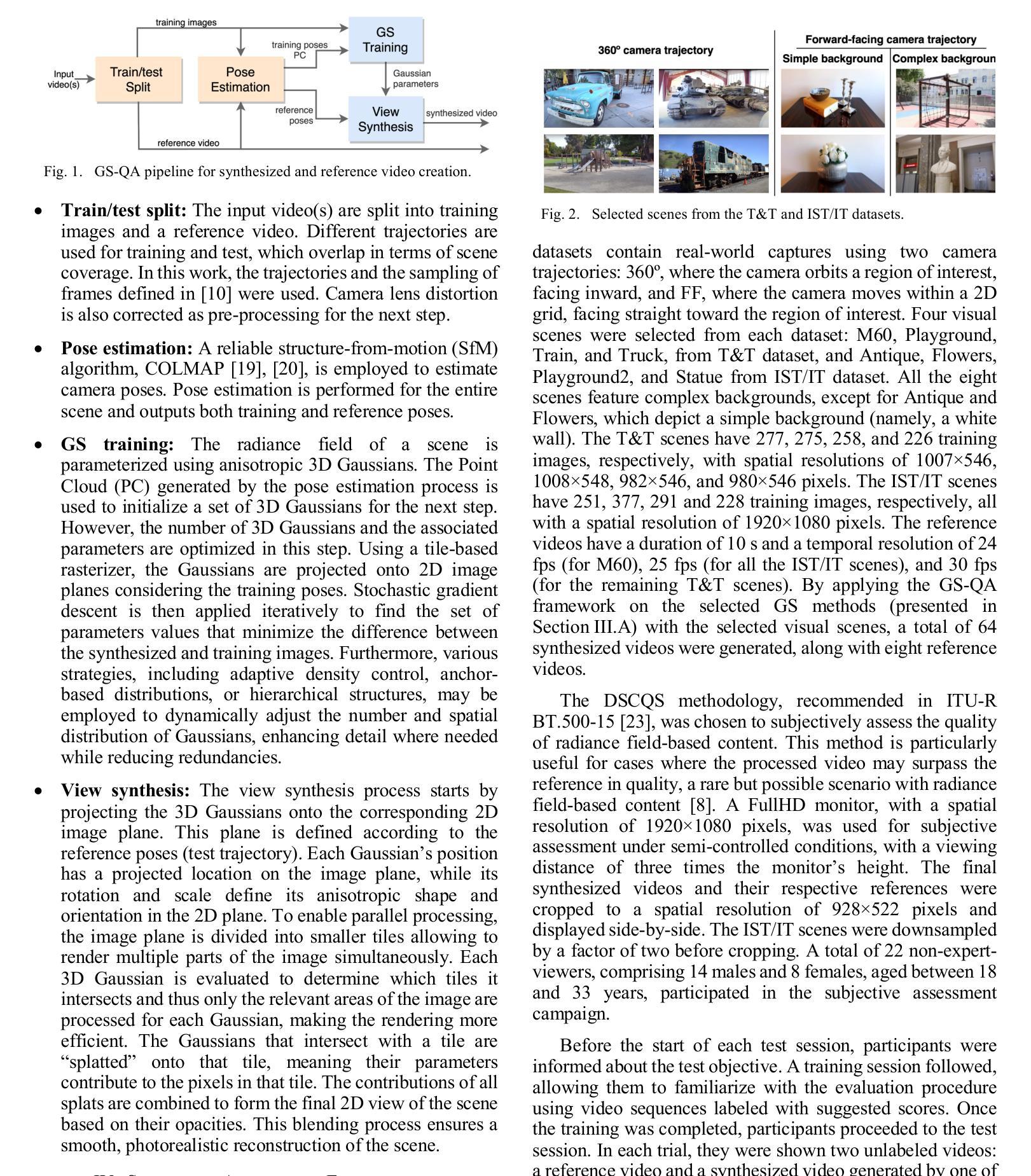
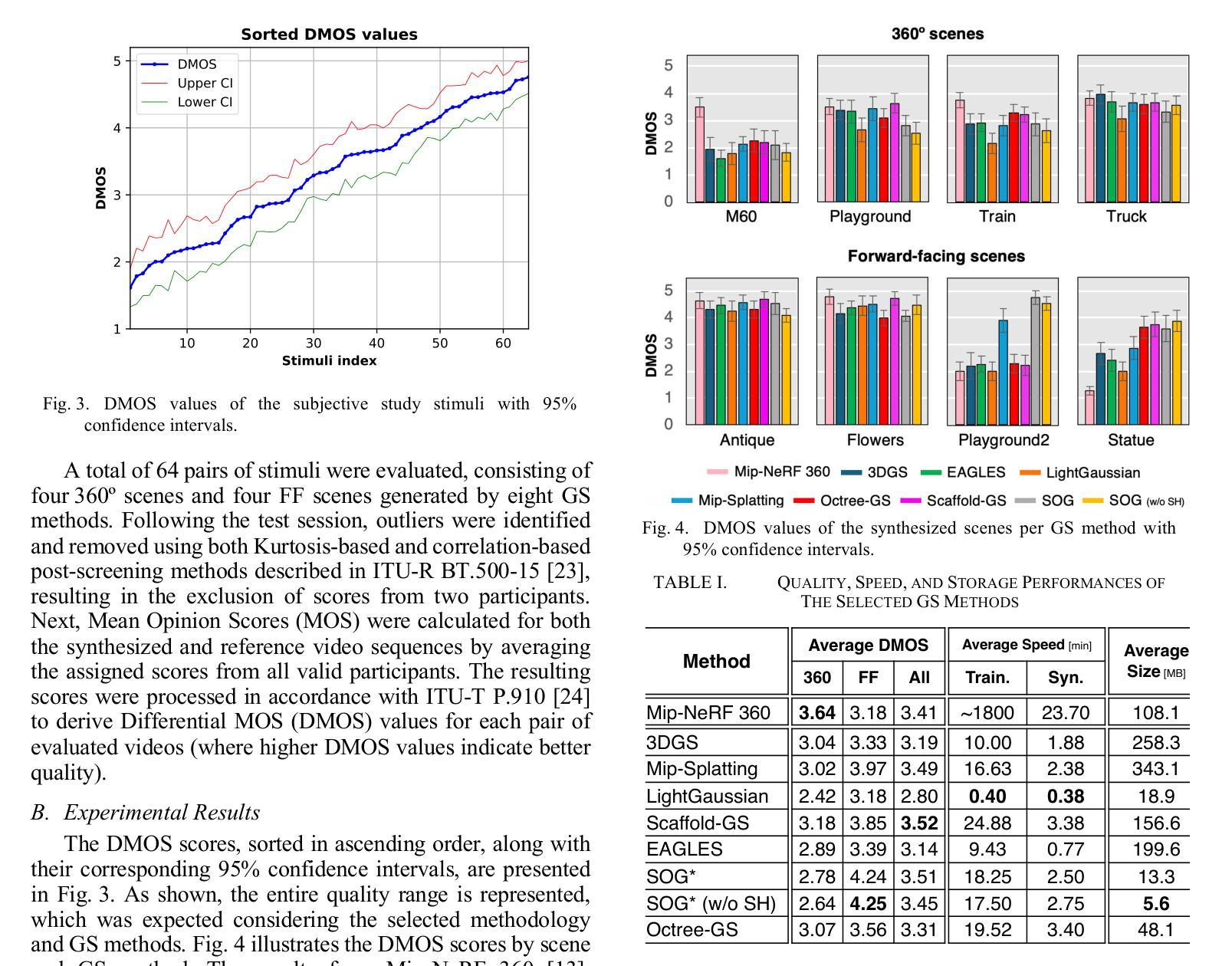
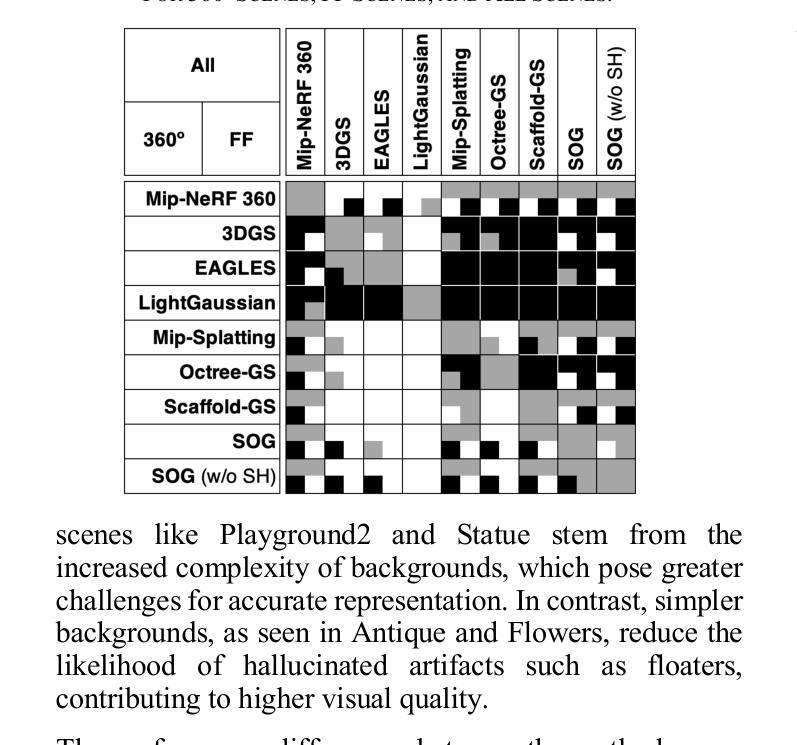
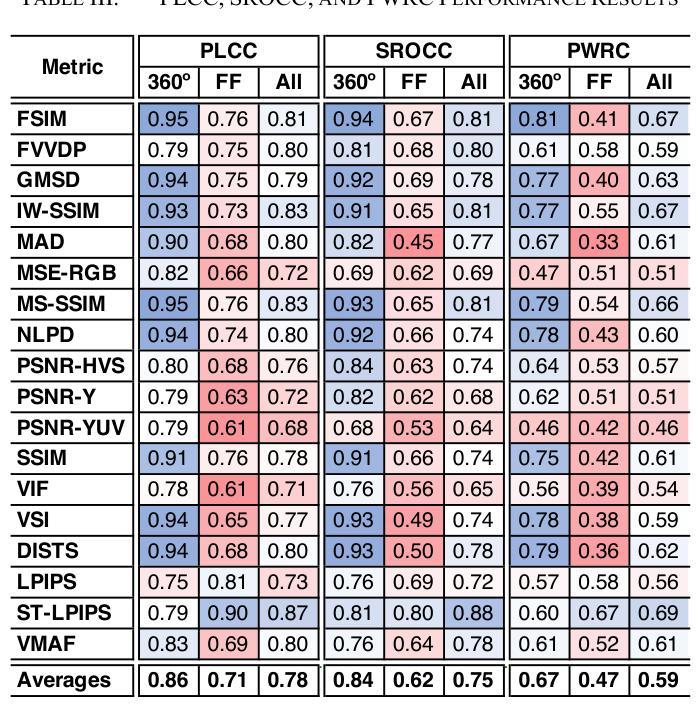
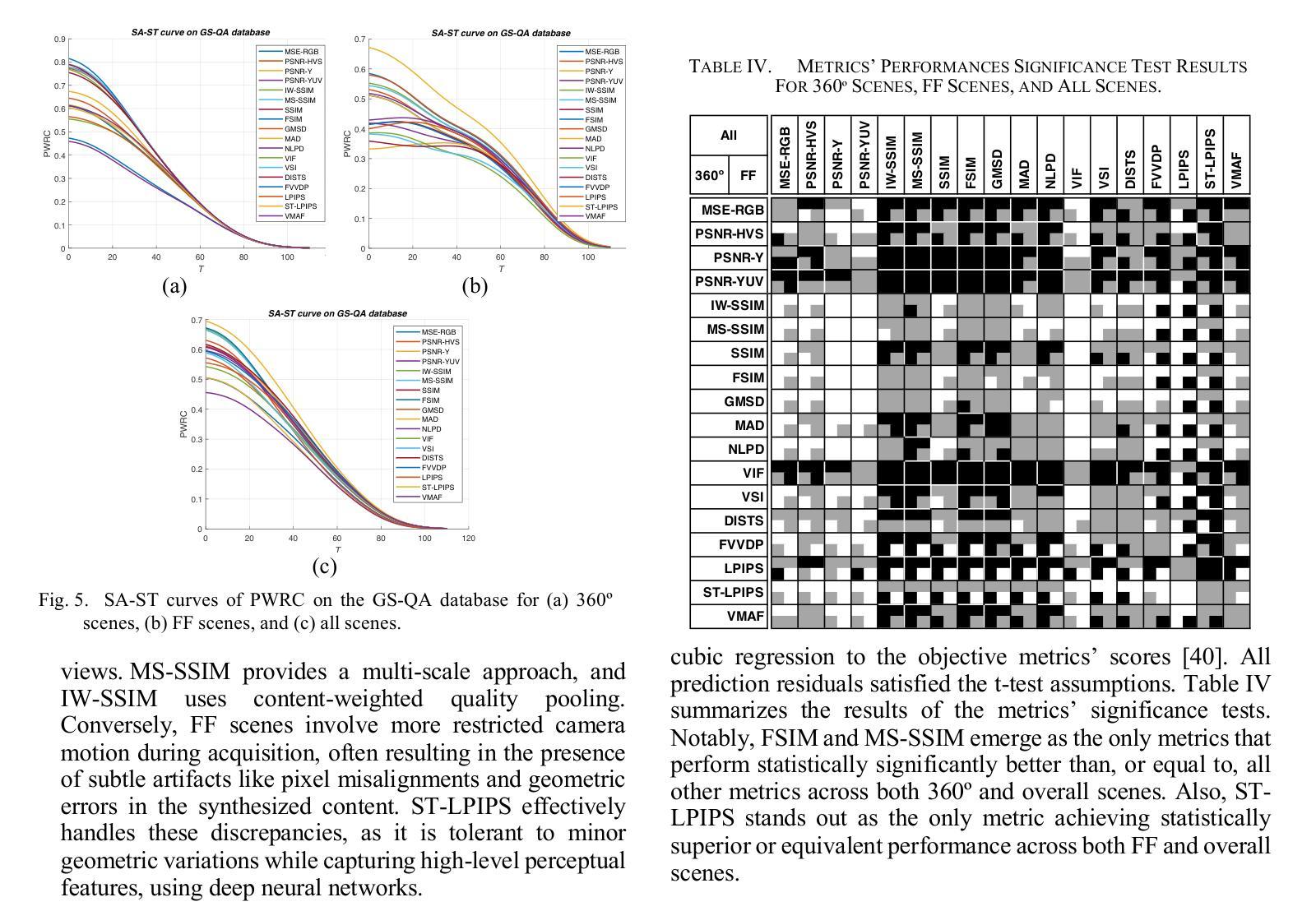
WRF-GS: Wireless Radiation Field Reconstruction with 3D Gaussian Splatting
Authors:Chaozheng Wen, Jingwen Tong, Yingdong Hu, Zehong Lin, Jun Zhang
Wireless channel modeling plays a pivotal role in designing, analyzing, and optimizing wireless communication systems. Nevertheless, developing an effective channel modeling approach has been a longstanding challenge. This issue has been escalated due to the denser network deployment, larger antenna arrays, and wider bandwidth in 5G and beyond networks. To address this challenge, we put forth WRF-GS, a novel framework for channel modeling based on wireless radiation field (WRF) reconstruction using 3D Gaussian splatting. WRF-GS employs 3D Gaussian primitives and neural networks to capture the interactions between the environment and radio signals, enabling efficient WRF reconstruction and visualization of the propagation characteristics. The reconstructed WRF can then be used to synthesize the spatial spectrum for comprehensive wireless channel characterization. Notably, with a small number of measurements, WRF-GS can synthesize new spatial spectra within milliseconds for a given scene, thereby enabling latency-sensitive applications. Experimental results demonstrate that WRF-GS outperforms existing methods for spatial spectrum synthesis, such as ray tracing and other deep-learning approaches. Moreover, WRF-GS achieves superior performance in the channel state information prediction task, surpassing existing methods by a significant margin of more than 2.43 dB.
无线信道建模在无线通讯系统的设计、分析和优化中扮演着核心角色。然而,开发有效的信道建模方法一直是一个长期存在的挑战。由于5G及以后网络的网络部署更密集、天线阵列更大、带宽更宽,这个问题进一步加剧。为了应对这一挑战,我们提出了WRF-GS,这是一种基于无线辐射场(WRF)重建的信道建模新型框架,采用三维高斯Splatting技术。WRF-GS利用三维高斯基元和神经网络来捕捉环境与无线电信号之间的相互作用,能够实现WRF的有效重建和传播特性的可视化。重建的WRF然后可用于合成空间频谱,以全面表征无线信道。值得注意的是,WRF-GS仅通过少量测量就能在给定场景毫秒内合成新的空间频谱,从而支持延迟敏感型应用。实验结果表明,WRF-GS在合成空间频谱方面的性能优于现有方法,如射线追踪和其他深度学习的方法。此外,WRF-GS在信道状态信息预测任务上也取得了卓越的性能,显著优于现有方法,提高了超过2.43分贝。
论文及项目相关链接
PDF accepted to the IEEE International Conference on Computer Communications (INFOCOM 2025)
Summary
WRF-GS是一个基于无线辐射场重建的无线信道建模新框架,利用三维高斯基元和神经网络捕获环境与无线电信号间的交互,实现高效WRF重建和传播特性可视化。WRF-GS能合成空间频谱,全面表征无线信道,且仅需少量测量数据,即可在毫秒内对给定场景合成新的空间频谱,适用于延迟敏感型应用。实验表明,WRF-GS在合成空间频谱和预测信道状态信息方面优于现有方法。
Key Takeaways
- WRF-GS是一个基于无线辐射场重建的无线信道建模框架。
- 该框架利用三维高斯基元和神经网络进行环境与无线电信号的交互捕获。
- WRF-GS能实现WRF的高效重建和传播特性可视化。
- WRF-GS能合成空间频谱,全面表征无线信道。
- 该框架对测量数据需求少,能快速合成新的空间频谱,适用于延迟敏感型应用。
- 实验显示WRF-GS在合成空间频谱方面优于现有方法,如射线追踪和其他深度学习方法。
点此查看论文截图
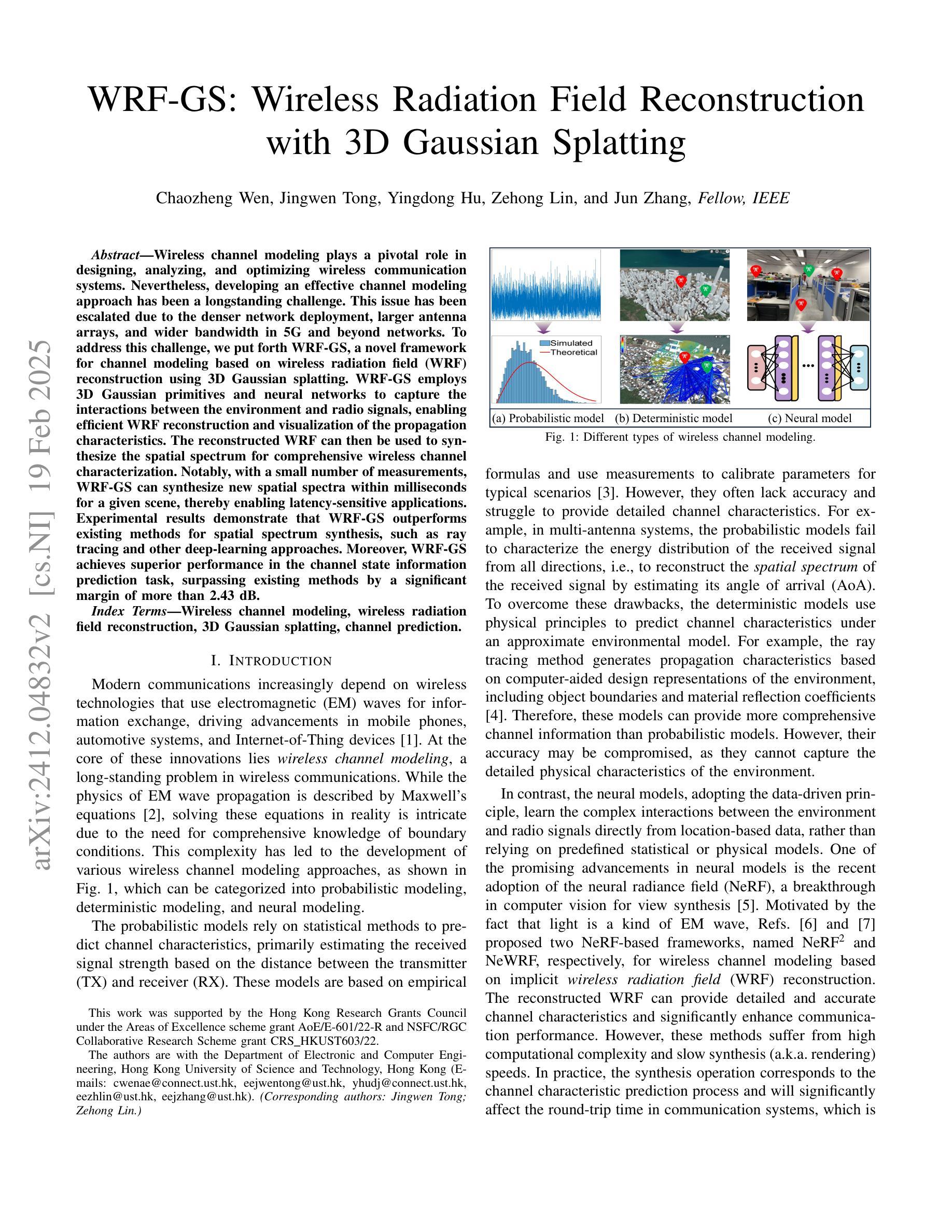
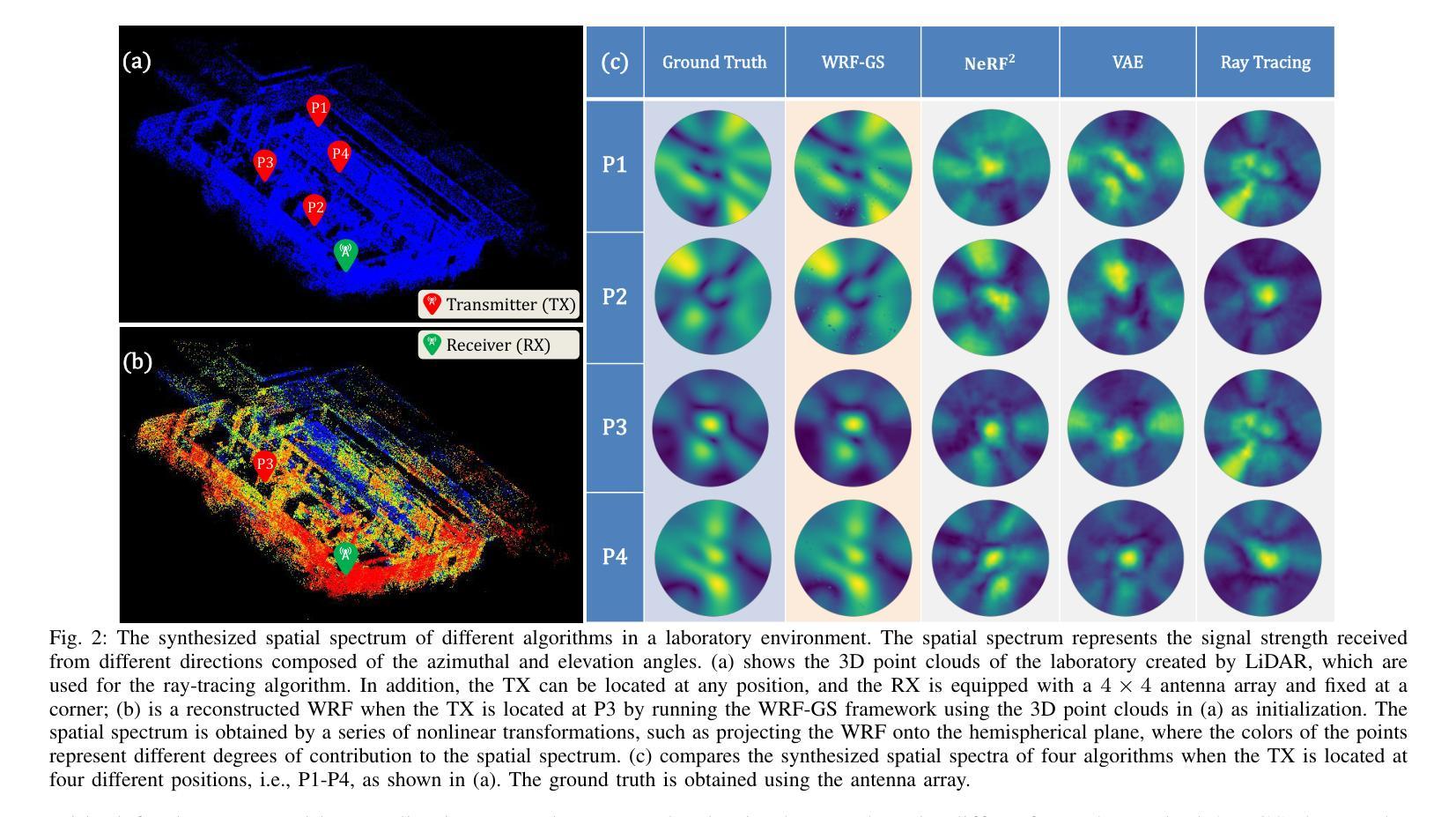
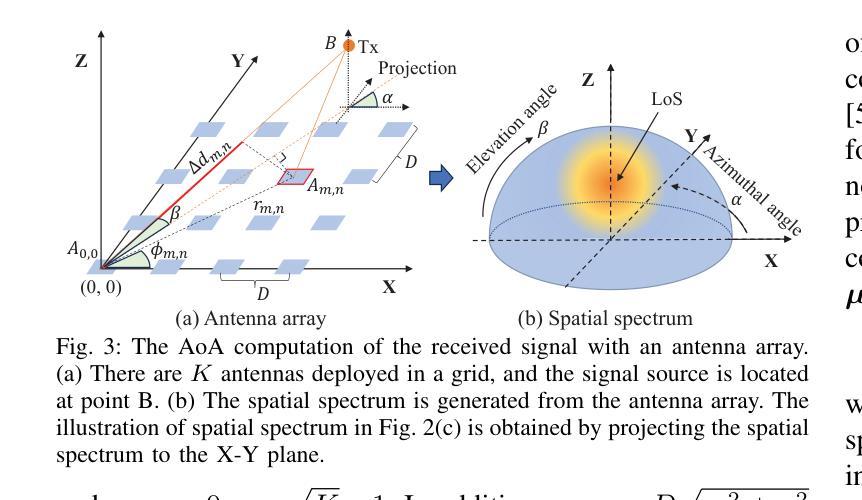
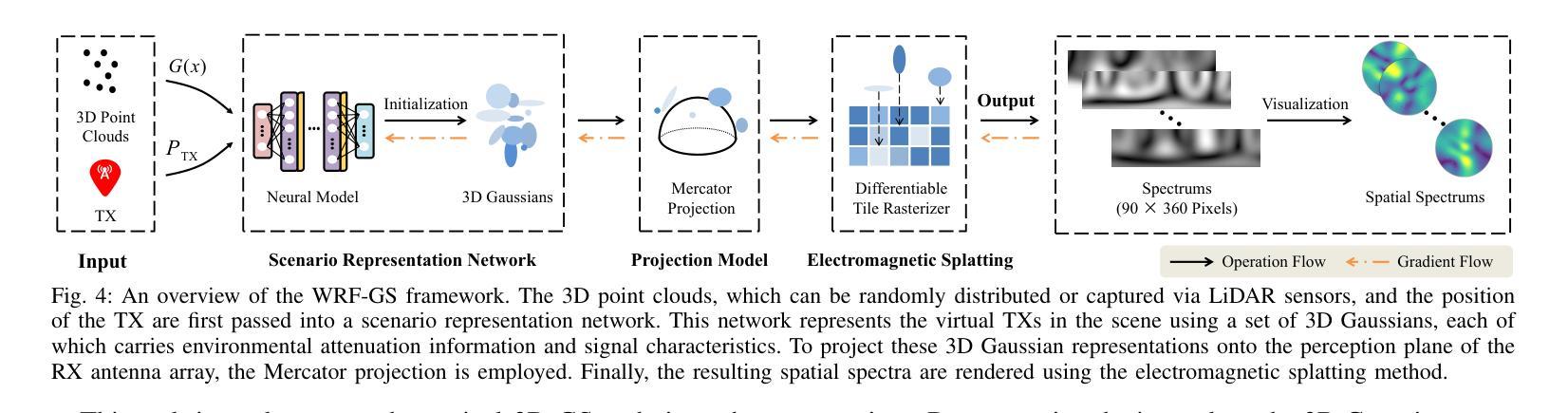
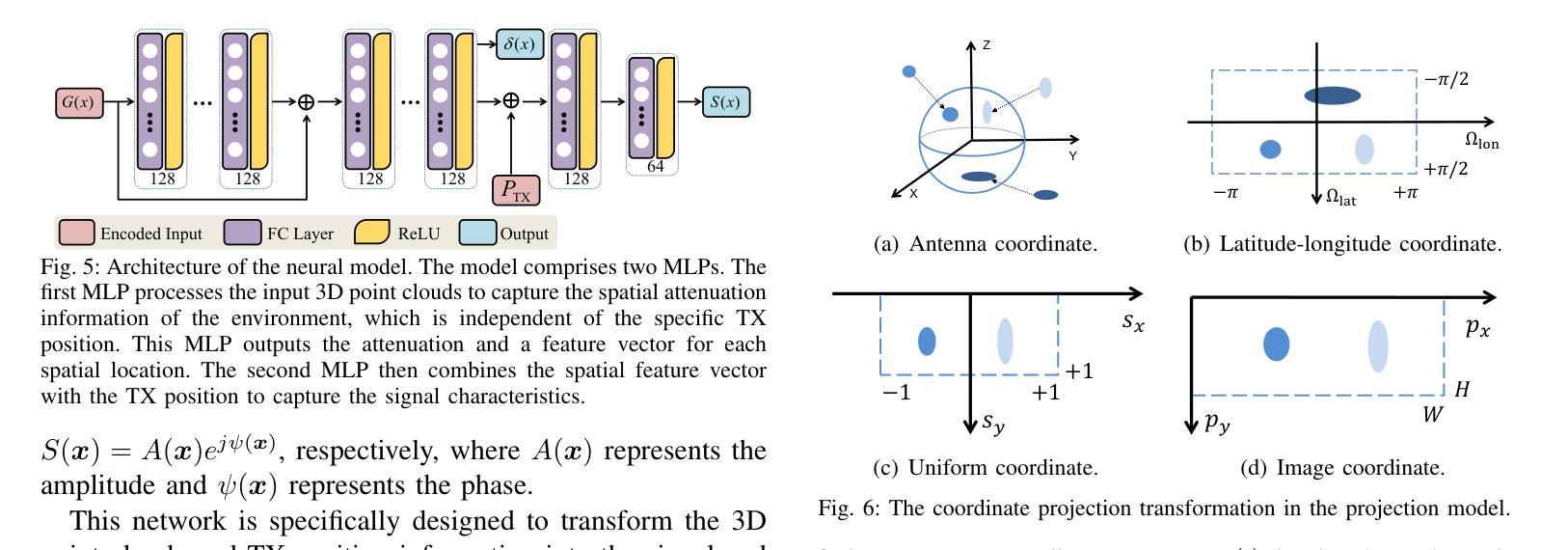
Hybrid Explicit Representation for Ultra-Realistic Head Avatars
Authors:Hongrui Cai, Yuting Xiao, Xuan Wang, Jiafei Li, Yudong Guo, Yanbo Fan, Shenghua Gao, Juyong Zhang
We introduce a novel approach to creating ultra-realistic head avatars and rendering them in real-time (>30fps at $2048 \times 1334$ resolution). First, we propose a hybrid explicit representation that combines the advantages of two primitive-based efficient rendering techniques. UV-mapped 3D mesh is utilized to capture sharp and rich textures on smooth surfaces, while 3D Gaussian Splatting is employed to represent complex geometric structures. In the pipeline of modeling an avatar, after tracking parametric models based on captured multi-view RGB videos, our goal is to simultaneously optimize the texture and opacity map of mesh, as well as a set of 3D Gaussian splats localized and rigged onto the mesh facets. Specifically, we perform $\alpha$-blending on the color and opacity values based on the merged and re-ordered z-buffer from the rasterization results of mesh and 3DGS. This process involves the mesh and 3DGS adaptively fitting the captured visual information to outline a high-fidelity digital avatar. To avoid artifacts caused by Gaussian splats crossing the mesh facets, we design a stable hybrid depth sorting strategy. Experiments illustrate that our modeled results exceed those of state-of-the-art approaches.
我们介绍了一种创建超逼真的头部化身并在实时环境中渲染它们的新方法(在$2048 \times 1334$分辨率下实现超过30帧的实时渲染)。首先,我们提出了一种混合显式表示方法,结合了两种基于原始的高效渲染技术的优点。UV映射的3D网格用于捕捉光滑表面上锐利且丰富的纹理,而3D高斯喷涂则用于表示复杂的几何结构。在化身建模过程中,我们在基于捕获的多视角RGB视频跟踪参数模型后,目标是同时优化网格的纹理和遮罩映射,以及定位在网格面控上的一组3D高斯喷涂。具体来说,我们对网格和3DGS的光栅化结果合并后的重排序的z缓冲器中的颜色和遮罩值执行alpha混合。这一过程使网格和3DGS自适应地适应捕获的视觉信息,以构建高保真数字化身。为了避免高斯喷涂穿过网格面而产生的伪影,我们设计了一种稳定的混合深度排序策略。实验表明,我们的建模结果超过了最先进的方法。
论文及项目相关链接
PDF 16 pages
Summary
该文本介绍了一种创建超逼真头像并将其实时渲染的新方法。该方法结合了两种基于原始的高效渲染技术的优点,使用UV映射的3D网格捕捉平滑表面的纹理,并采用三维高斯散斑技术呈现复杂几何结构。该模型在捕捉多视角RGB视频后,优化网格的纹理和不透明度图,以及定位并固定在网格面片上的三维高斯溅射集。实验表明,该方法的效果超过了当前先进技术。
Key Takeaways
- 介绍了一种结合两种原始渲染技术的新方法,用于创建超逼真头像并进行实时渲染。
- 利用UV映射的3D网格捕捉平滑表面的丰富纹理。
- 使用三维高斯溅射技术呈现复杂几何结构。
- 模型在追踪基于捕捉的多视角RGB视频参数模型后,同时优化网格的纹理和不透明度图以及三维高斯溅射集。
- 通过合并和重新排序的z缓冲器进行α混合,实现网格和三维高斯溅射的融合。
- 设计了一种稳定的混合深度排序策略,以避免因高斯溅射跨越网格面片而产生伪影。
点此查看论文截图
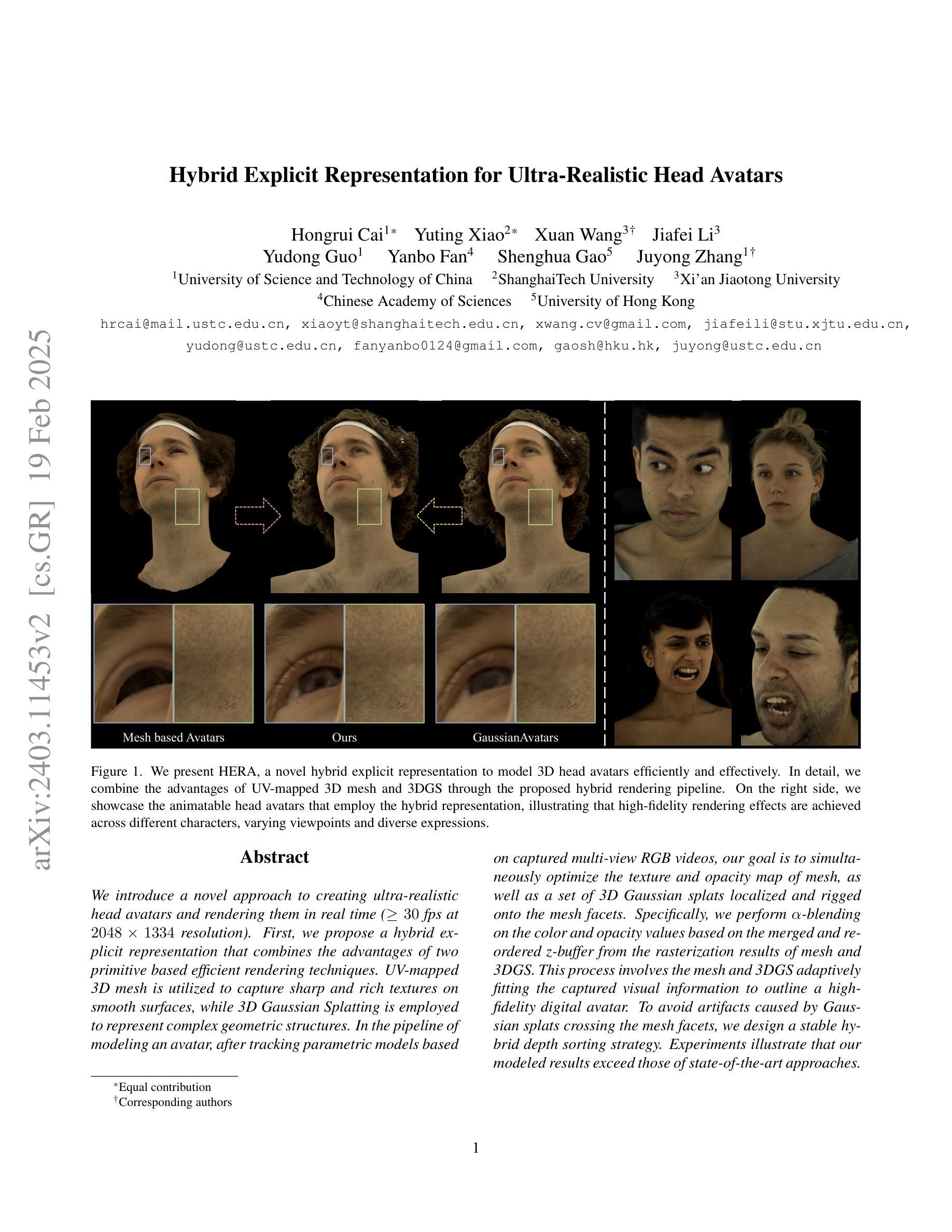
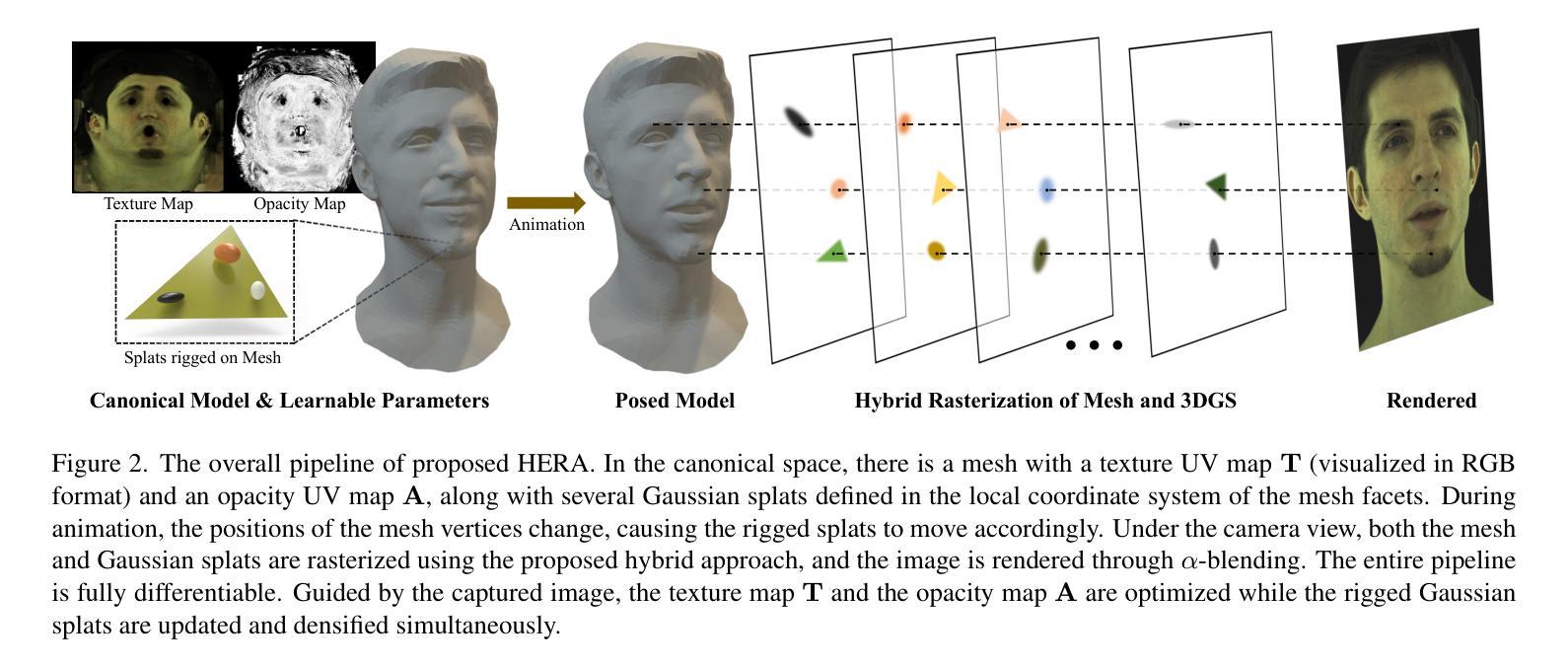
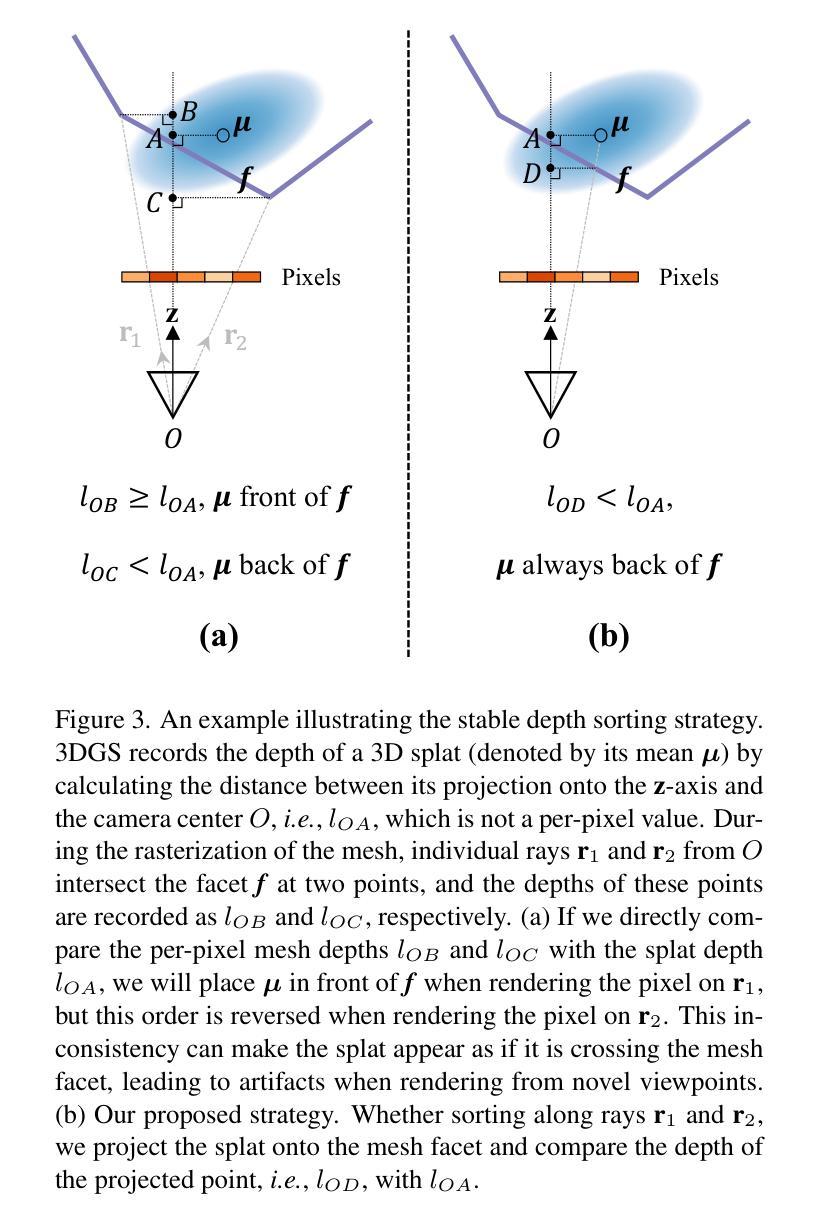
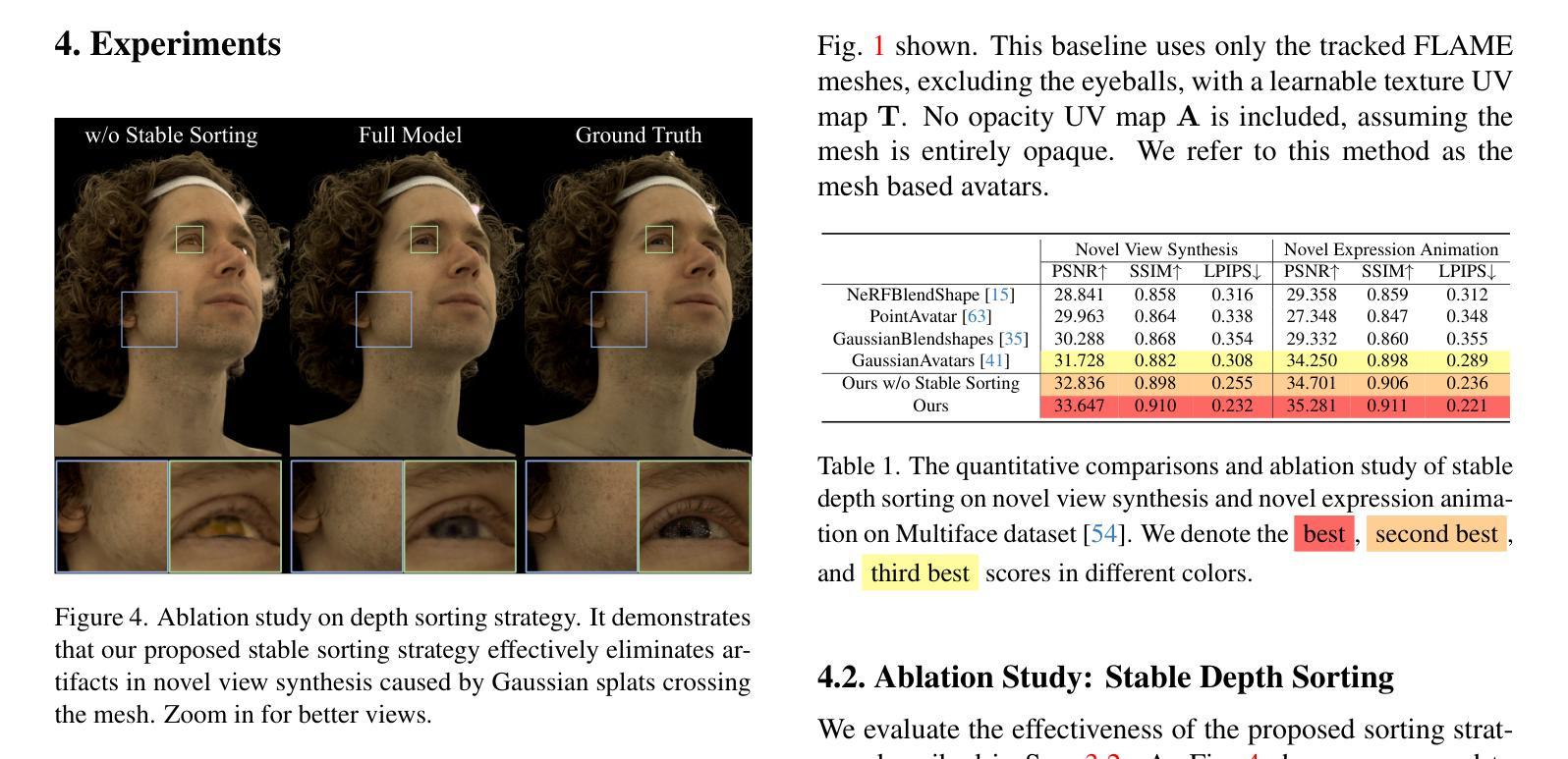