⚠️ 以下所有内容总结都来自于 大语言模型的能力,如有错误,仅供参考,谨慎使用
🔴 请注意:千万不要用于严肃的学术场景,只能用于论文阅读前的初筛!
💗 如果您觉得我们的项目对您有帮助 ChatPaperFree ,还请您给我们一些鼓励!⭐️ HuggingFace免费体验
2025-02-21 更新
MagicGeo: Training-Free Text-Guided Geometric Diagram Generation
Authors:Junxiao Wang, Ting Zhang, Heng Yu, Jingdong Wang, Hua Huang
Geometric diagrams are critical in conveying mathematical and scientific concepts, yet traditional diagram generation methods are often manual and resource-intensive. While text-to-image generation has made strides in photorealistic imagery, creating accurate geometric diagrams remains a challenge due to the need for precise spatial relationships and the scarcity of geometry-specific datasets. This paper presents MagicGeo, a training-free framework for generating geometric diagrams from textual descriptions. MagicGeo formulates the diagram generation process as a coordinate optimization problem, ensuring geometric correctness through a formal language solver, and then employs coordinate-aware generation. The framework leverages the strong language translation capability of large language models, while formal mathematical solving ensures geometric correctness. We further introduce MagicGeoBench, a benchmark dataset of 220 geometric diagram descriptions, and demonstrate that MagicGeo outperforms current methods in both qualitative and quantitative evaluations. This work provides a scalable, accurate solution for automated diagram generation, with significant implications for educational and academic applications.
几何图表在传达数学和科学概念方面起着关键作用,但传统的图表生成方法通常是手动进行的,且资源消耗大。虽然文本到图像生成技术在照片级真实图像方面取得了进展,但由于需要精确的空间关系和几何特定数据集的稀缺,创建准确的几何图表仍然是一个挑战。本文针对这一问题,提出了一种无需训练的几何图表生成框架MagicGeo,该框架可根据文本描述生成几何图表。MagicGeo将图表生成过程表述为坐标优化问题,通过形式化语言求解器确保几何正确性,然后采用坐标感知生成。该框架利用大型语言模型的强大语言翻译能力,同时形式化的数学求解保证了几何正确性。此外,我们还介绍了MagicGeoBench数据集,包含了220个几何图表描述,并证明MagicGeo在定性和定量评估方面均优于当前方法。这项工作为自动图表生成提供了可扩展、准确的解决方案,对教育和学术应用具有重要意义。
论文及项目相关链接
Summary
几何图表在表达数学和科学概念时至关重要,但传统的图表生成方法往往手动且资源消耗大。文本转图像生成在逼真的图像方面取得了进展,但由于需要精确的空间关系和几何特定数据集的稀缺性,创建准确的几何图表仍然是一个挑战。本文提出了MagicGeo,这是一个无需训练即可从文本描述生成几何图表的框架。MagicGeo将图表生成过程表述为坐标优化问题,通过形式语言求解器确保几何正确性,然后采用坐标感知生成。该框架利用大型语言模型的强大语言翻译能力,同时形式数学求解确保几何正确性。此外,还介绍了MagicGeoBench,包含220个几何图表描述的基准数据集,并证明MagicGeo在定性和定量评估中都优于当前方法。这项研究为自动化图表生成提供了可扩展且准确的解决方案,对教育学术应用具有重大影响。
Key Takeaways
- 几何图表在表达数学和科学概念时具有关键作用,但传统生成方法存在手动且资源密集的问题。
- 文本转图像生成在创建准确的几何图表方面存在挑战,主要由于需要精确的空间关系和几何特定数据集的稀缺性。
- MagicGeo框架实现了从文本描述生成几何图表而无需训练,通过坐标优化问题表述图表生成过程。
- MagicGeo利用形式语言求解器确保几何正确性,并采用坐标感知生成方法。
- 大型语言模型的强大翻译能力与形式数学求解相结合,提高了MagicGeo的性能。
- 引入了MagicGeoBench基准数据集,包含220个几何图表描述,用于评估图表生成方法。
点此查看论文截图
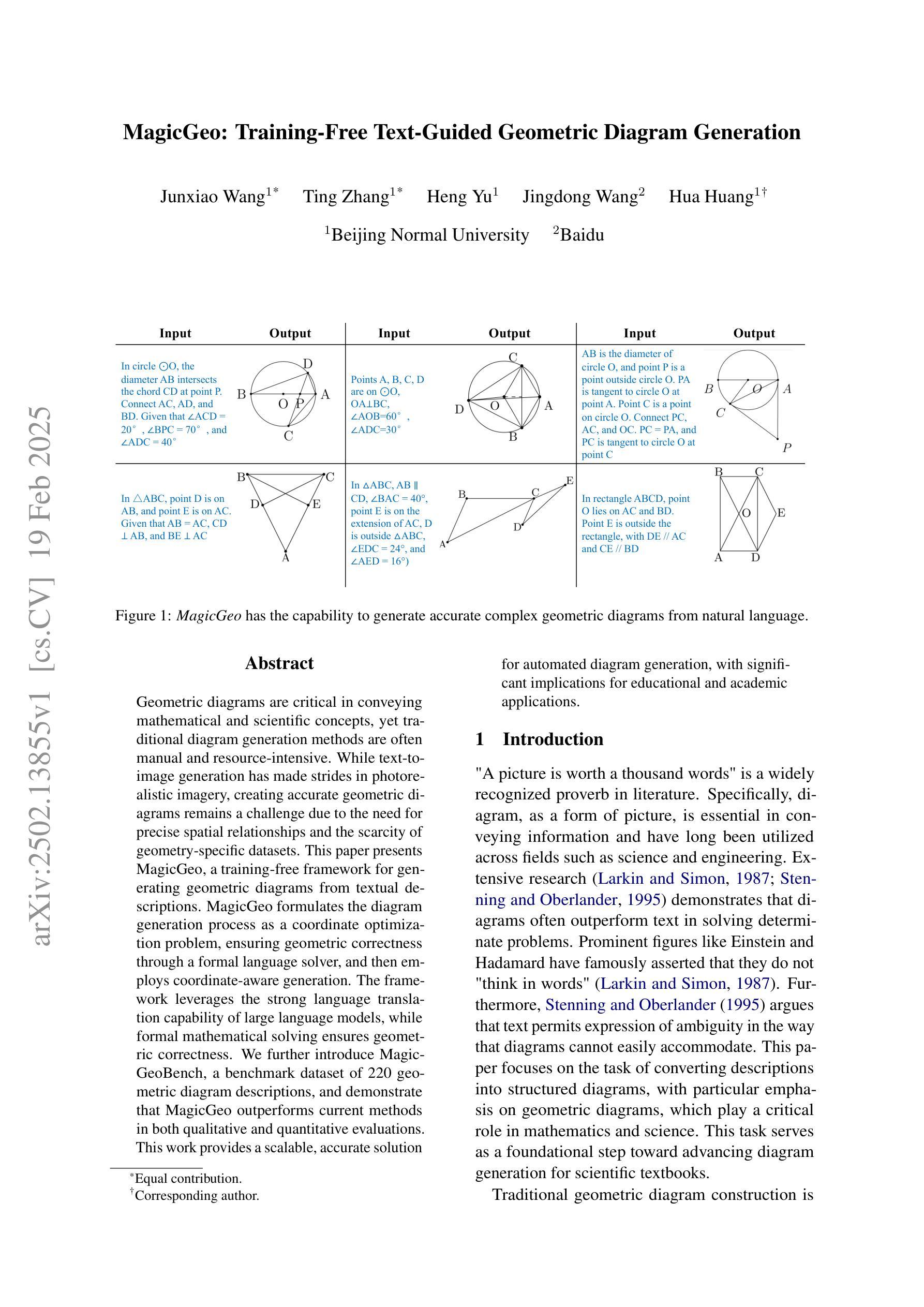
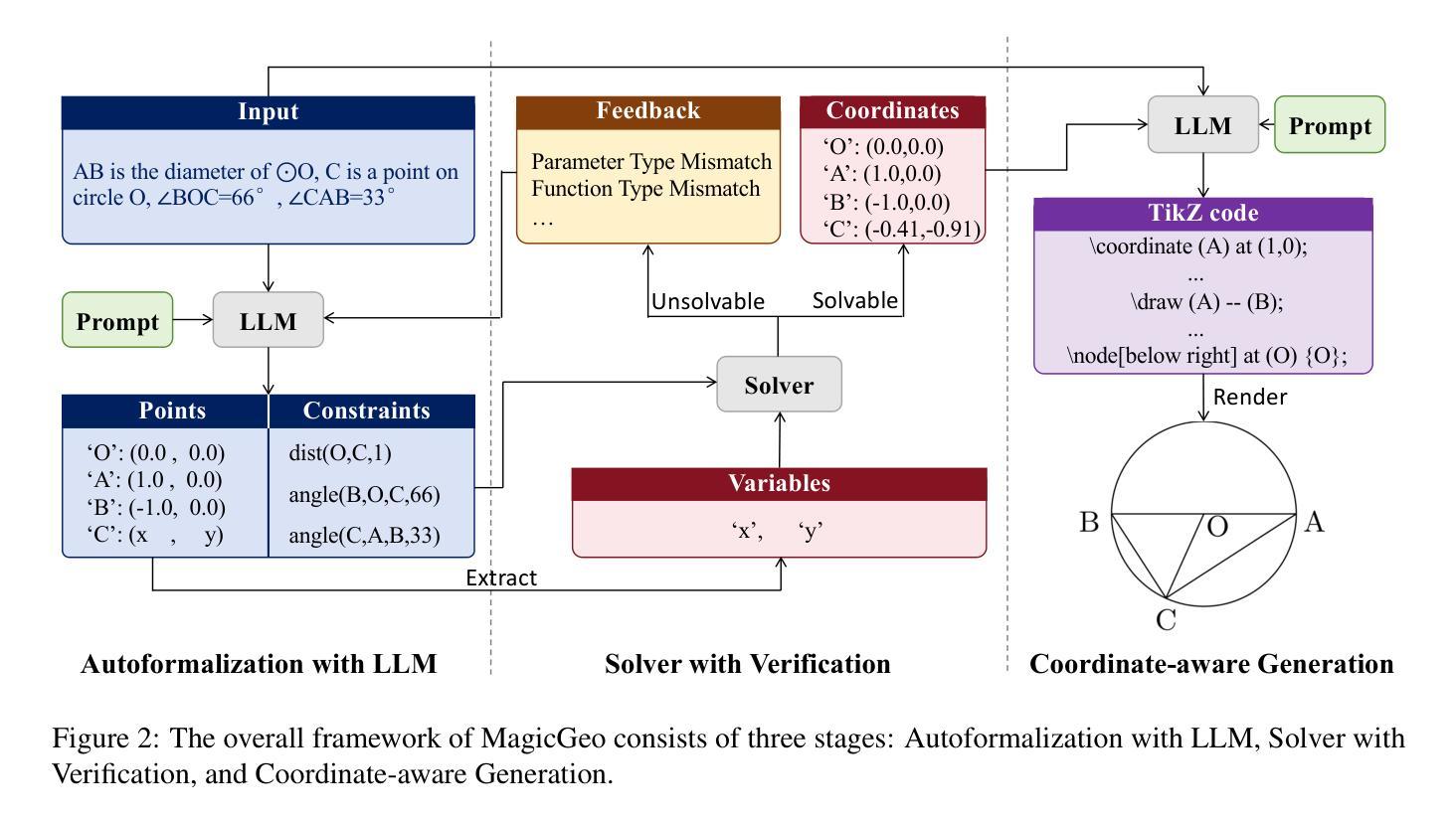
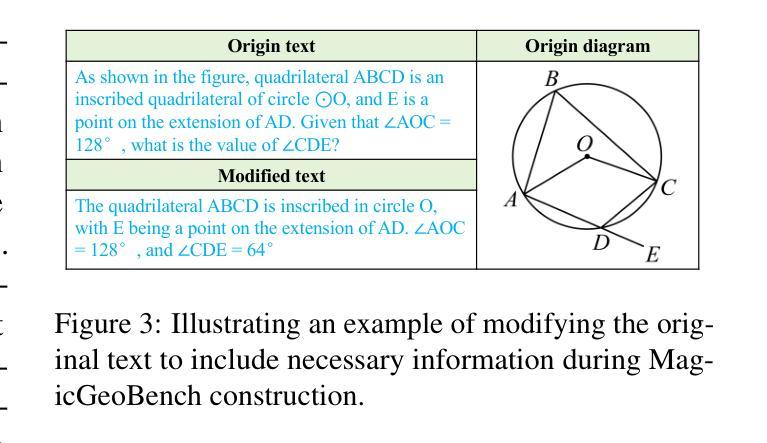
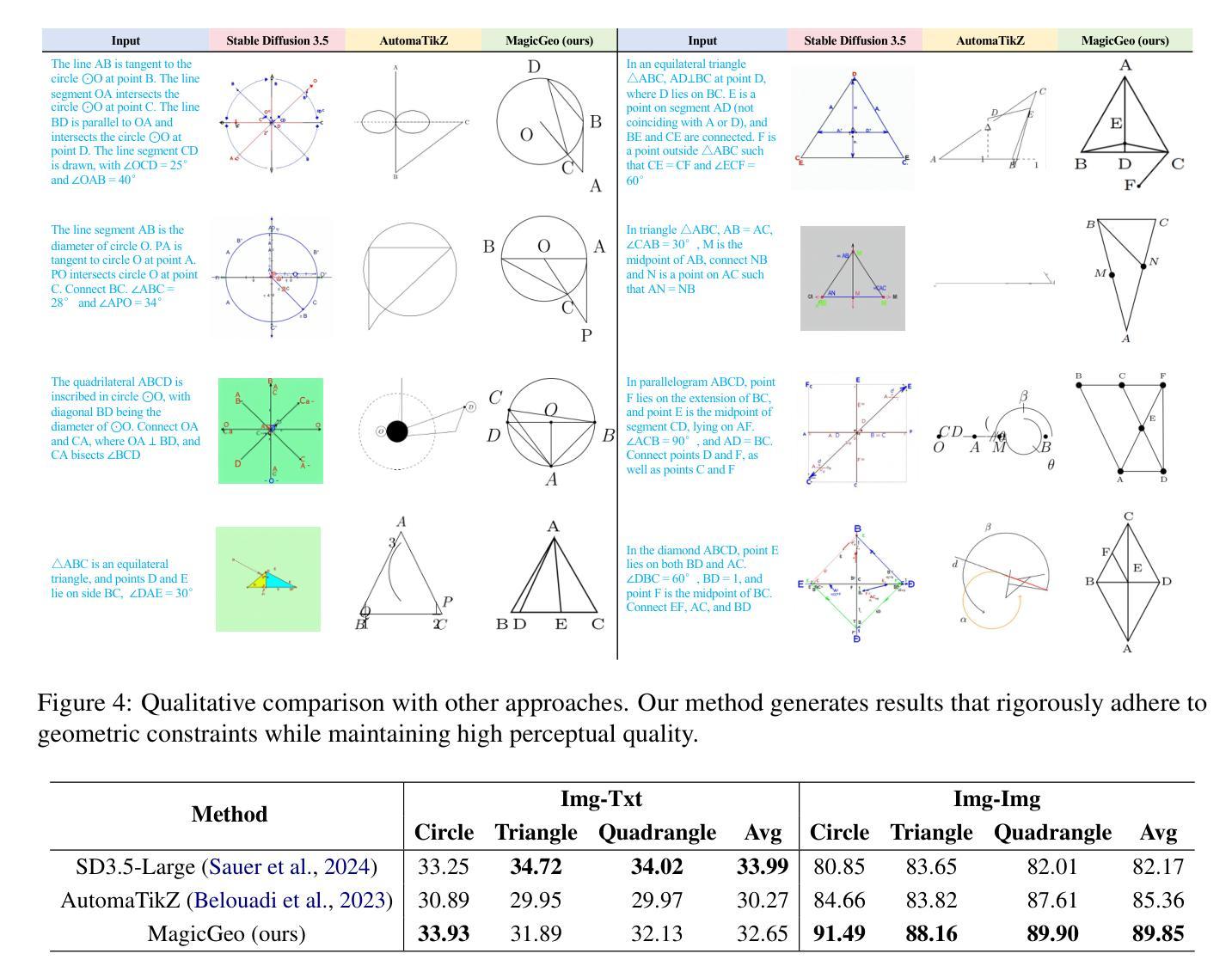
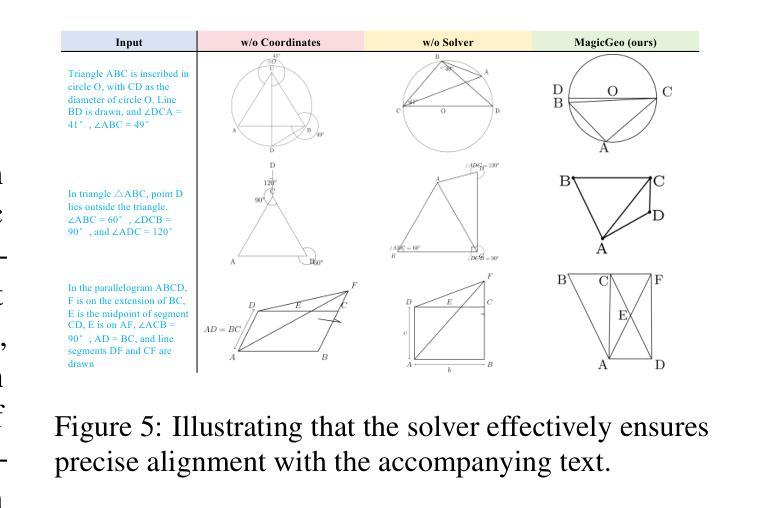
A Survey on Bridging EEG Signals and Generative AI: From Image and Text to Beyond
Authors:Shreya Shukla, Jose Torres, Abhijit Mishra, Jacek Gwizdka, Shounak Roychowdhury
Integration of Brain-Computer Interfaces (BCIs) and Generative Artificial Intelligence (GenAI) has opened new frontiers in brain signal decoding, enabling assistive communication, neural representation learning, and multimodal integration. BCIs, particularly those leveraging Electroencephalography (EEG), provide a non-invasive means of translating neural activity into meaningful outputs. Recent advances in deep learning, including Generative Adversarial Networks (GANs) and Transformer-based Large Language Models (LLMs), have significantly improved EEG-based generation of images, text, and speech. This paper provides a literature review of the state-of-the-art in EEG-based multimodal generation, focusing on (i) EEG-to-image generation through GANs, Variational Autoencoders (VAEs), and Diffusion Models, and (ii) EEG-to-text generation leveraging Transformer based language models and contrastive learning methods. Additionally, we discuss the emerging domain of EEG-to-speech synthesis, an evolving multimodal frontier. We highlight key datasets, use cases, challenges, and EEG feature encoding methods that underpin generative approaches. By providing a structured overview of EEG-based generative AI, this survey aims to equip researchers and practitioners with insights to advance neural decoding, enhance assistive technologies, and expand the frontiers of brain-computer interaction.
脑机接口(BCIs)与生成式人工智能(GenAI)的融合为脑信号解码开辟了新的前沿领域,为实现辅助通信、神经表征学习和多模式融合提供了可能。特别是利用脑电图(EEG)的脑机接口,提供了一种非侵入性的方式,将神经活动转化为有意义的输出。深度学习领域的最新进展,包括生成对抗网络(GANs)和基于变压器的自然语言大模型(LLMs),极大地提高了基于EEG的图像、文本和语音生成能力。本文对基于EEG的多模式生成领域的最新研究进行了文献综述,重点关注了(i)通过GANs、变分自动编码器(VAEs)和扩散模型实现的EEG到图像生成;(ii)利用基于Transformer的自然语言模型和对比学习方法实现的EEG到文本生成。此外,我们还讨论了新兴的EEG到语音合成领域,这是一个不断发展的多模式前沿领域。本文重点介绍了关键数据集、应用场景、挑战以及支撑生成方法的EEG特征编码方法。通过对基于EEG的生成式人工智能的结构性概述,本综述旨在为研究人员和实践者提供洞察力,以推动神经解码的发展,提高辅助技术的效能,并拓展脑机交互的边界。
论文及项目相关链接
Summary
脑机接口(BCI)与生成式人工智能(GenAI)的融合为脑信号解码开拓了新领域,推动了辅助通信、神经表征学习和多模式融合。利用脑电图(EEG)的BCI为非侵入性神经活动转化为有意义输出提供了手段。深度学习领域的最新进展,包括生成对抗网络(GANs)和基于Transformer的大型语言模型(LLMs),已显著改进基于EEG的图像、文本和语音生成。本文综述了EEG基多模式生成的最新进展,重点讨论EEG转图像生成的GANs、变分自动编码器(VAEs)和扩散模型以及EEG转文本生成的基于Transformer的语言模型和对比学习方法。同时,概述了新兴的EEG语音合成领域,这是一个不断发展的多模式前沿领域。
Key Takeaways
- BCI与GenAI融合为脑信号解码开拓新领域。
- EEG为非侵入性神经活动转化为有意义输出提供手段。
- 深度学习在EEG基图像、文本和语音生成中有显著改进。
- GANs、VAEs、扩散模型用于EEG转图像生成。
- 基于Transformer的语言模型和对比学习方法用于EEG转文本生成。
- EEG语音合成是新兴的多模式前沿领域。
点此查看论文截图
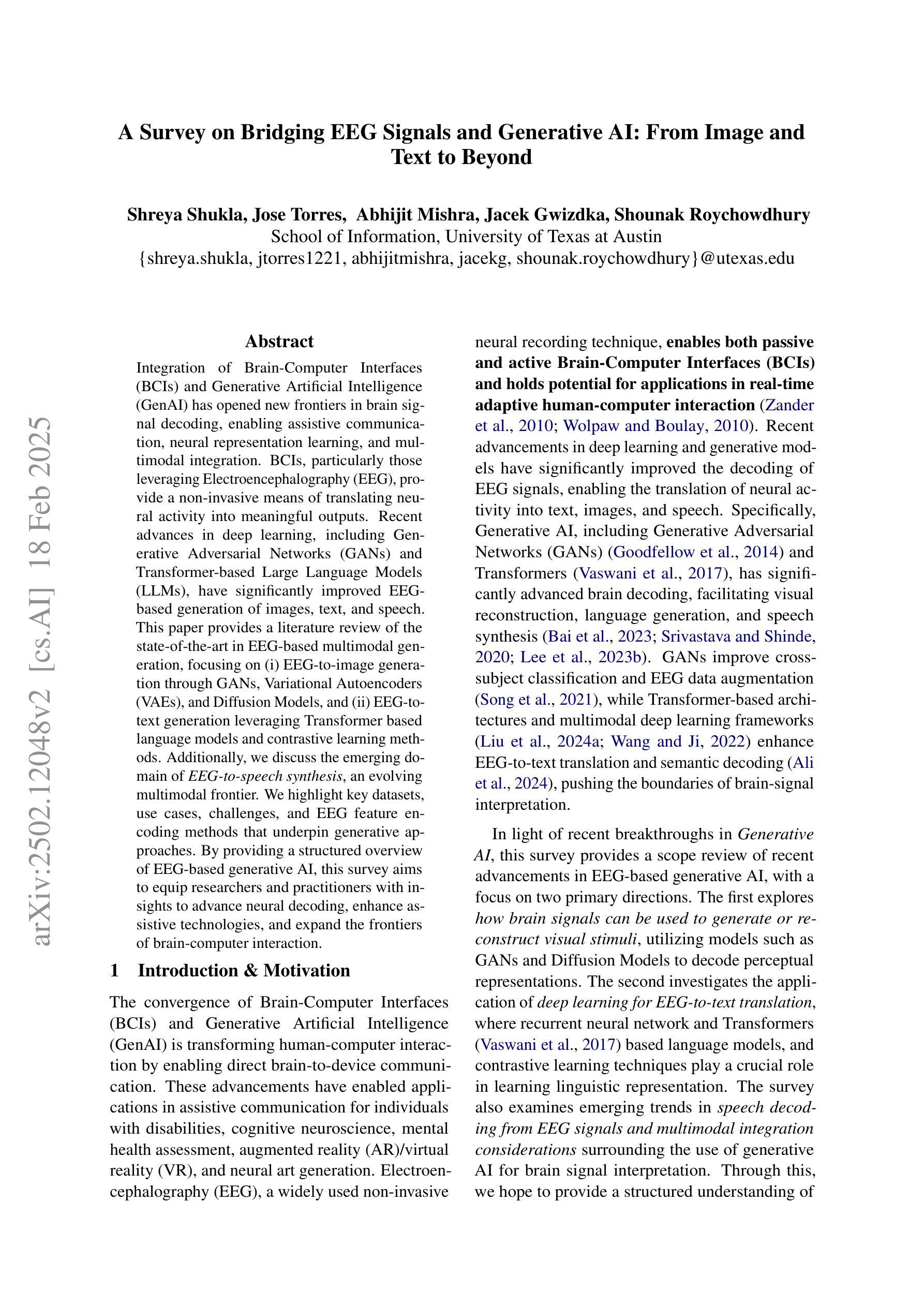
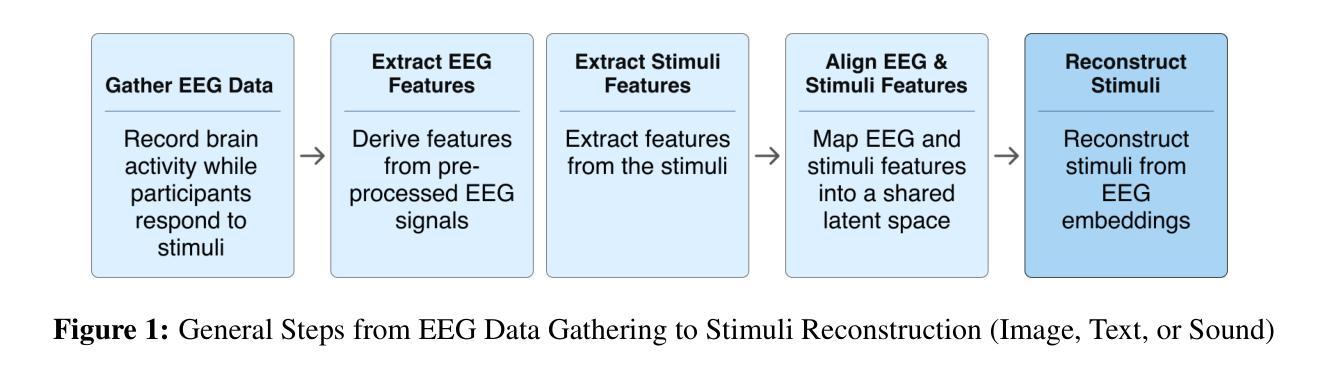
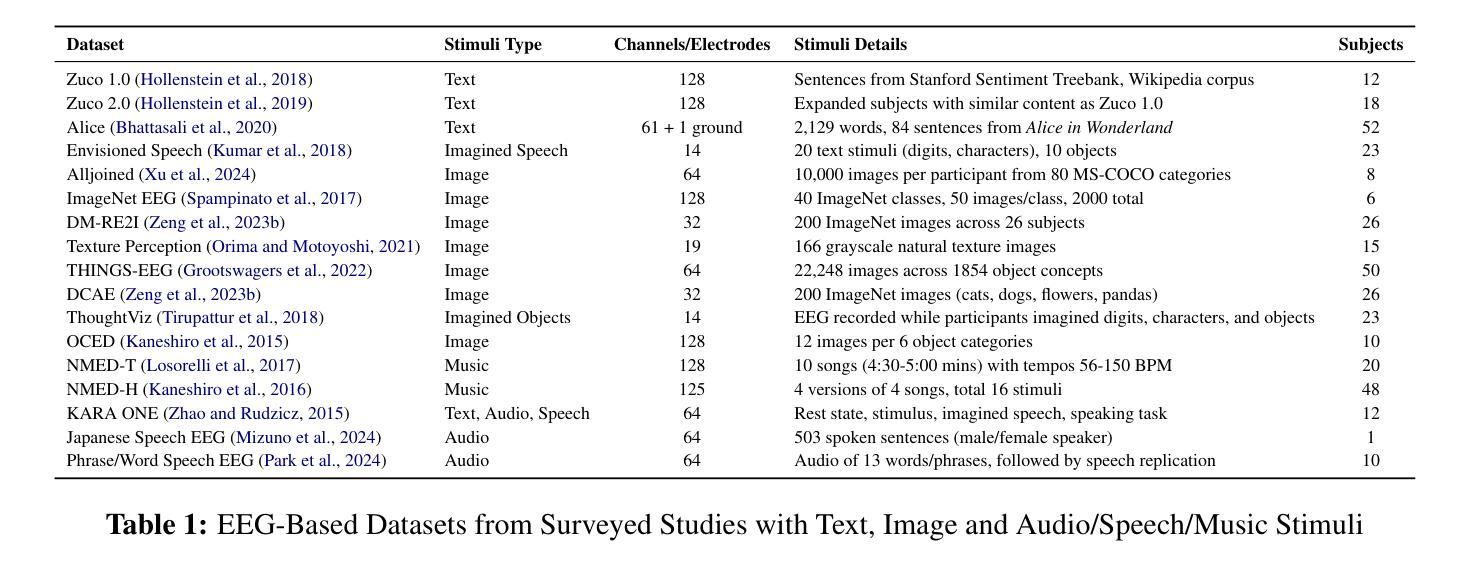
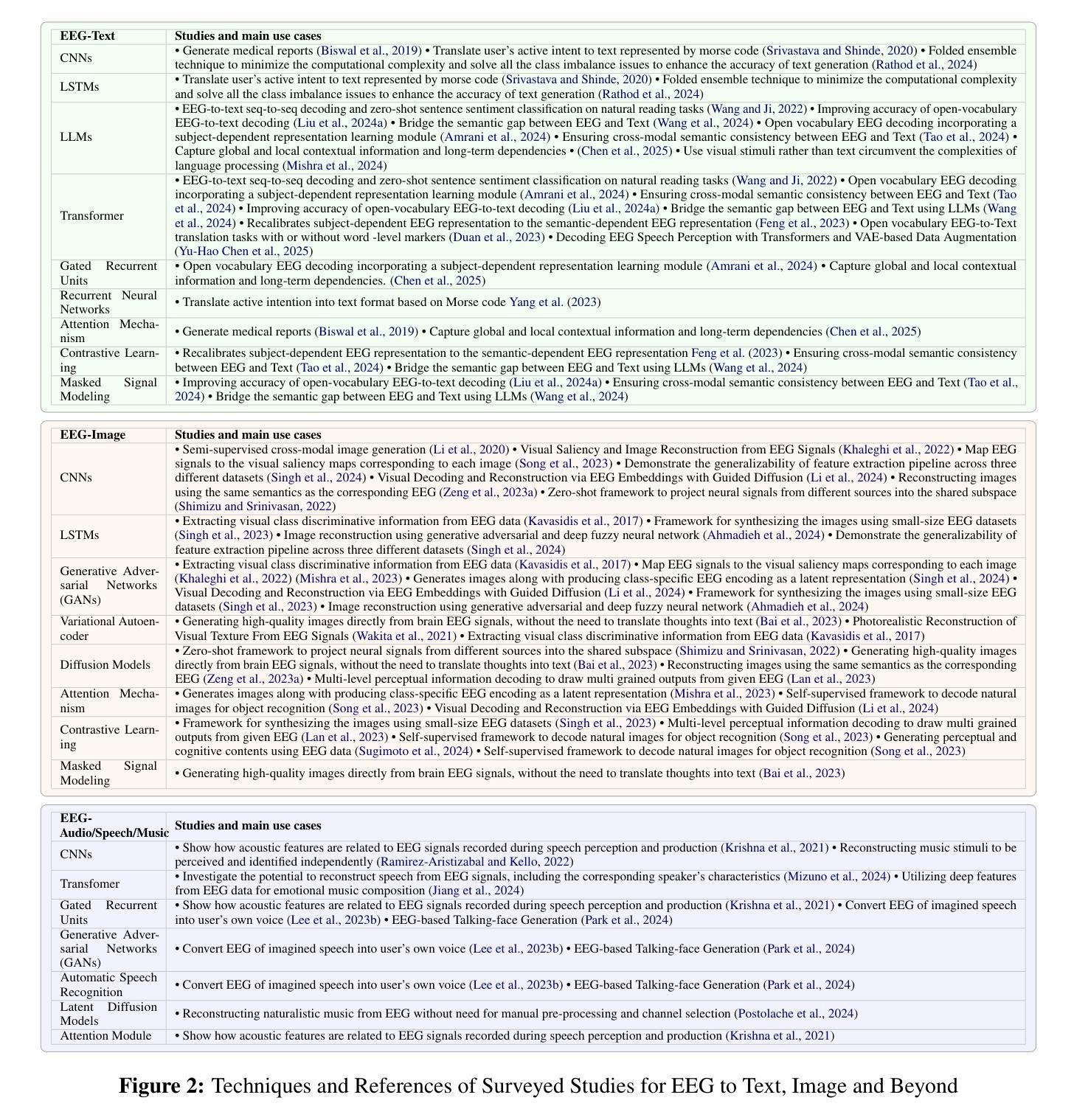
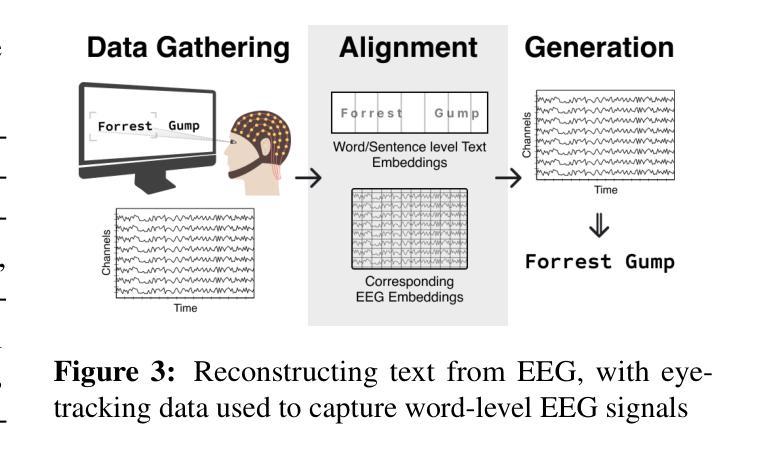