⚠️ 以下所有内容总结都来自于 大语言模型的能力,如有错误,仅供参考,谨慎使用
🔴 请注意:千万不要用于严肃的学术场景,只能用于论文阅读前的初筛!
💗 如果您觉得我们的项目对您有帮助 ChatPaperFree ,还请您给我们一些鼓励!⭐️ HuggingFace免费体验
2025-02-21 更新
Qwen2.5-VL Technical Report
Authors:Shuai Bai, Keqin Chen, Xuejing Liu, Jialin Wang, Wenbin Ge, Sibo Song, Kai Dang, Peng Wang, Shijie Wang, Jun Tang, Humen Zhong, Yuanzhi Zhu, Mingkun Yang, Zhaohai Li, Jianqiang Wan, Pengfei Wang, Wei Ding, Zheren Fu, Yiheng Xu, Jiabo Ye, Xi Zhang, Tianbao Xie, Zesen Cheng, Hang Zhang, Zhibo Yang, Haiyang Xu, Junyang Lin
We introduce Qwen2.5-VL, the latest flagship model of Qwen vision-language series, which demonstrates significant advancements in both foundational capabilities and innovative functionalities. Qwen2.5-VL achieves a major leap forward in understanding and interacting with the world through enhanced visual recognition, precise object localization, robust document parsing, and long-video comprehension. A standout feature of Qwen2.5-VL is its ability to localize objects using bounding boxes or points accurately. It provides robust structured data extraction from invoices, forms, and tables, as well as detailed analysis of charts, diagrams, and layouts. To handle complex inputs, Qwen2.5-VL introduces dynamic resolution processing and absolute time encoding, enabling it to process images of varying sizes and videos of extended durations (up to hours) with second-level event localization. This allows the model to natively perceive spatial scales and temporal dynamics without relying on traditional normalization techniques. By training a native dynamic-resolution Vision Transformer (ViT) from scratch and incorporating Window Attention, we reduce computational overhead while maintaining native resolution. As a result, Qwen2.5-VL excels not only in static image and document understanding but also as an interactive visual agent capable of reasoning, tool usage, and task execution in real-world scenarios such as operating computers and mobile devices. Qwen2.5-VL is available in three sizes, addressing diverse use cases from edge AI to high-performance computing. The flagship Qwen2.5-VL-72B model matches state-of-the-art models like GPT-4o and Claude 3.5 Sonnet, particularly excelling in document and diagram understanding. Additionally, Qwen2.5-VL maintains robust linguistic performance, preserving the core language competencies of the Qwen2.5 LLM.
我们推出了Qwen2.5-VL,作为Qwen视觉语言系列的最新旗舰模型,它在基础能力和创新功能方面都取得了显著进展。Qwen2.5-VL在理解和与世界互动方面取得了重大突破,通过增强视觉识别、精确的对象定位、稳健的文档解析和长视频理解来实现。Qwen2.5-VL的一个突出特点是它使用边界框或点准确定位对象的能力。它提供了从发票、表单和表格中稳健的结构数据提取,以及图表、图表和布局的详细分析。为了处理复杂的输入,Qwen2.5-VL引入了动态分辨率处理和绝对时间编码,使其能够处理各种尺寸的图像和延长时长的视频(长达数小时)以及二级事件定位。这允许模型在无需依赖传统归一化技术的情况下,原生地感知空间尺度和时间动态。我们通过从头开始训练本地动态分辨率视觉转换器(ViT)并融入窗口注意力机制,在减少计算开销的同时保持了原始分辨率。因此,Qwen2.5-VL不仅在静态图像和文档理解方面表现出色,还作为能够在现实世界的场景中执行推理、工具使用和任务执行的交互式视觉代理,如操作计算机和移动设备。Qwen2.5-VL有三种尺寸可供选择,适用于从边缘AI到高性能计算的多种用例。旗舰版Qwen2.5-VL-72B模型与GPT-4o和Claude 3.5 Sonnet等最先进的模型相匹配,特别是在文档和图表理解方面表现尤为出色。此外,Qwen2.5-VL保持了稳健的语言性能,保留了Qwen2.5 LLM的核心语言能力。
论文及项目相关链接
Summary
Qwen2.5-VL模型是Qwen视觉语言系列的最新旗舰产品,具有显著的能力和创新的特性。它实现了对世界的理解和交互的重大突破,通过增强视觉识别、精确对象定位、稳健的文档解析和长视频理解等功能实现。模型具备准确的对象定位能力,能够从发票、表单和表格中提取结构化数据,以及详细分析图表、图表和布局。通过引入动态分辨率处理和绝对时间编码,该模型能够处理各种尺寸的图像和长达数小时的视频,并实现二级事件定位。它采用从头开始训练的动态分辨率视觉变压器(ViT)并融入窗口注意力机制,降低了计算成本同时保持原生分辨率。因此,Qwen2.5-VL不仅在静态图像和文档理解方面表现出色,还具备作为智能视觉代理的能力,可在实际场景中执行推理、工具使用和任务操作,如操作计算机和移动设备。该模型有三种尺寸,适用于边缘AI到高性能计算的多种用例。旗舰Qwen2.5-VL-72B模型与GPT-4o和Claude 3.5 Sonnet等先进模型相媲美,尤其在文档和图表理解方面表现出众。同时保持稳健的语言性能,继承了Qwen2.5 LLM的核心语言技能。
Key Takeaways
- Qwen2.5-VL是Qwen视觉语言系列的最新旗舰模型,具有显著的能力和创新的特性。
- 它实现了增强视觉识别、精确对象定位、文档解析和长视频理解等功能。
- 模型具备准确的对象定位能力,包括从各种文档中准确提取结构化数据。
- 通过动态分辨率处理和绝对时间编码,模型能够处理复杂的输入,包括不同尺寸的图像和长时间视频。
- Qwen2.5-VL采用先进的Vision Transformer技术,通过窗口注意力机制降低计算成本。
- 该模型不仅在静态图像和文档理解方面表现出色,还具备智能视觉代理的能力,适用于实际场景中的推理、工具使用和任务操作。
点此查看论文截图
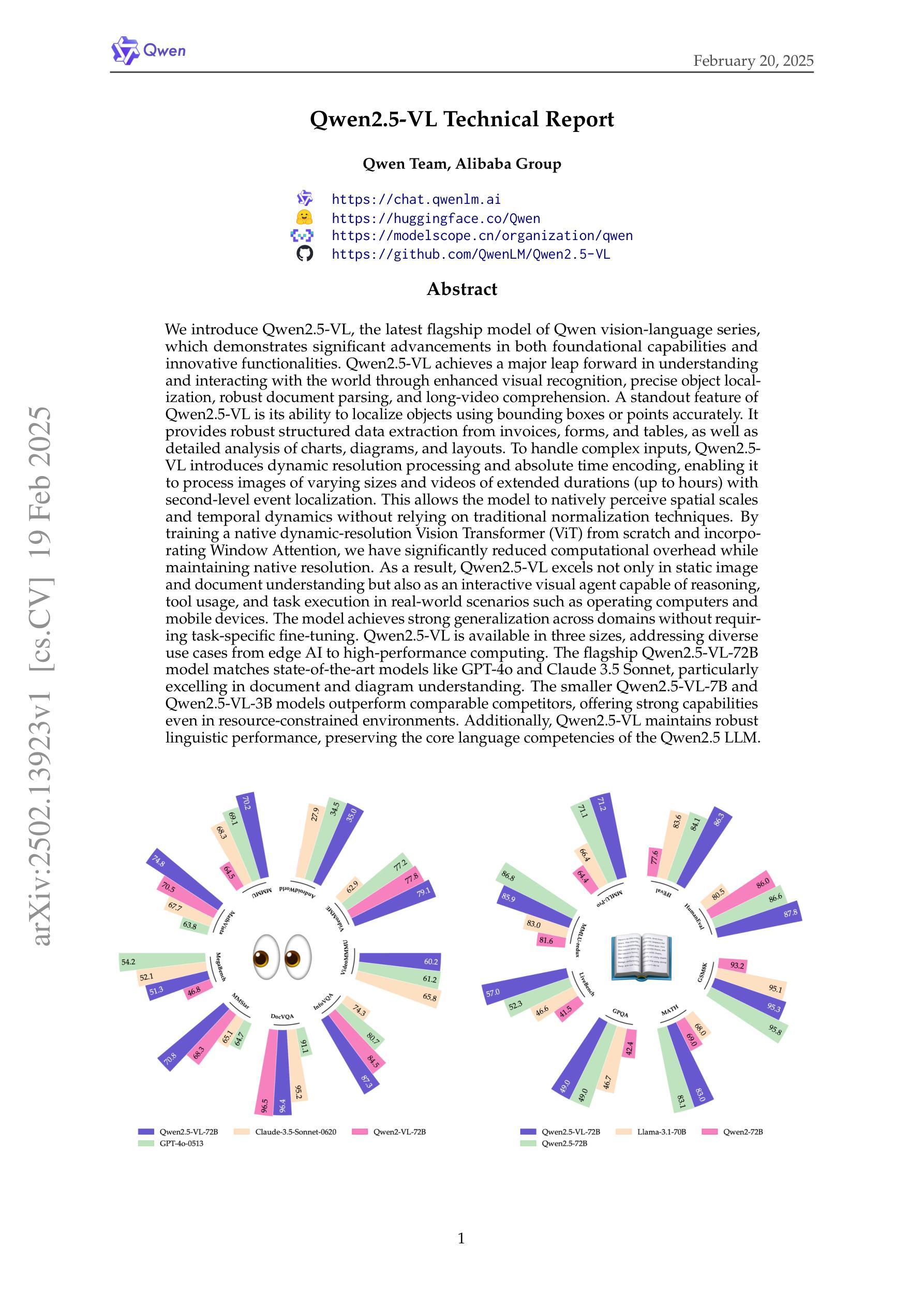
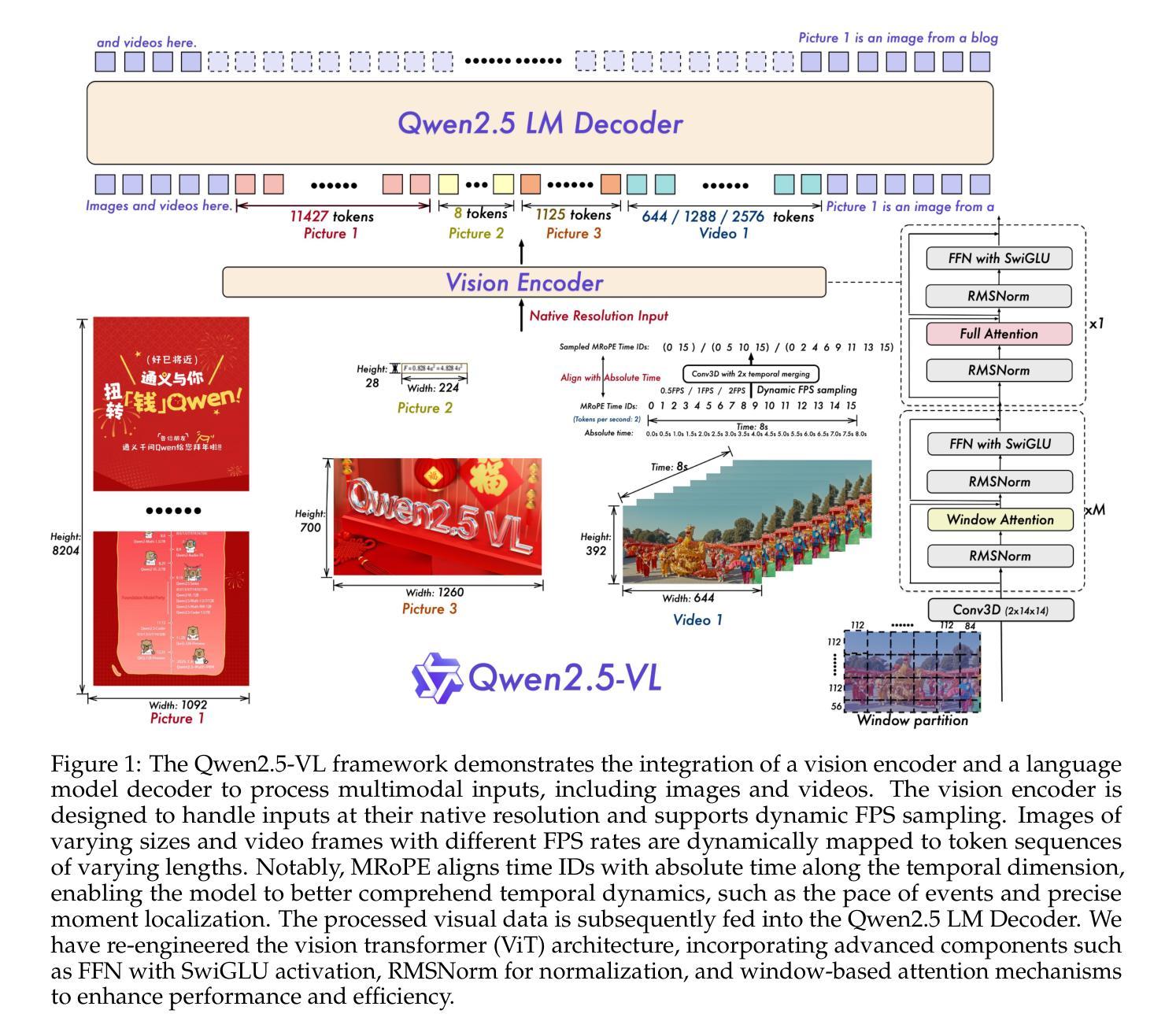
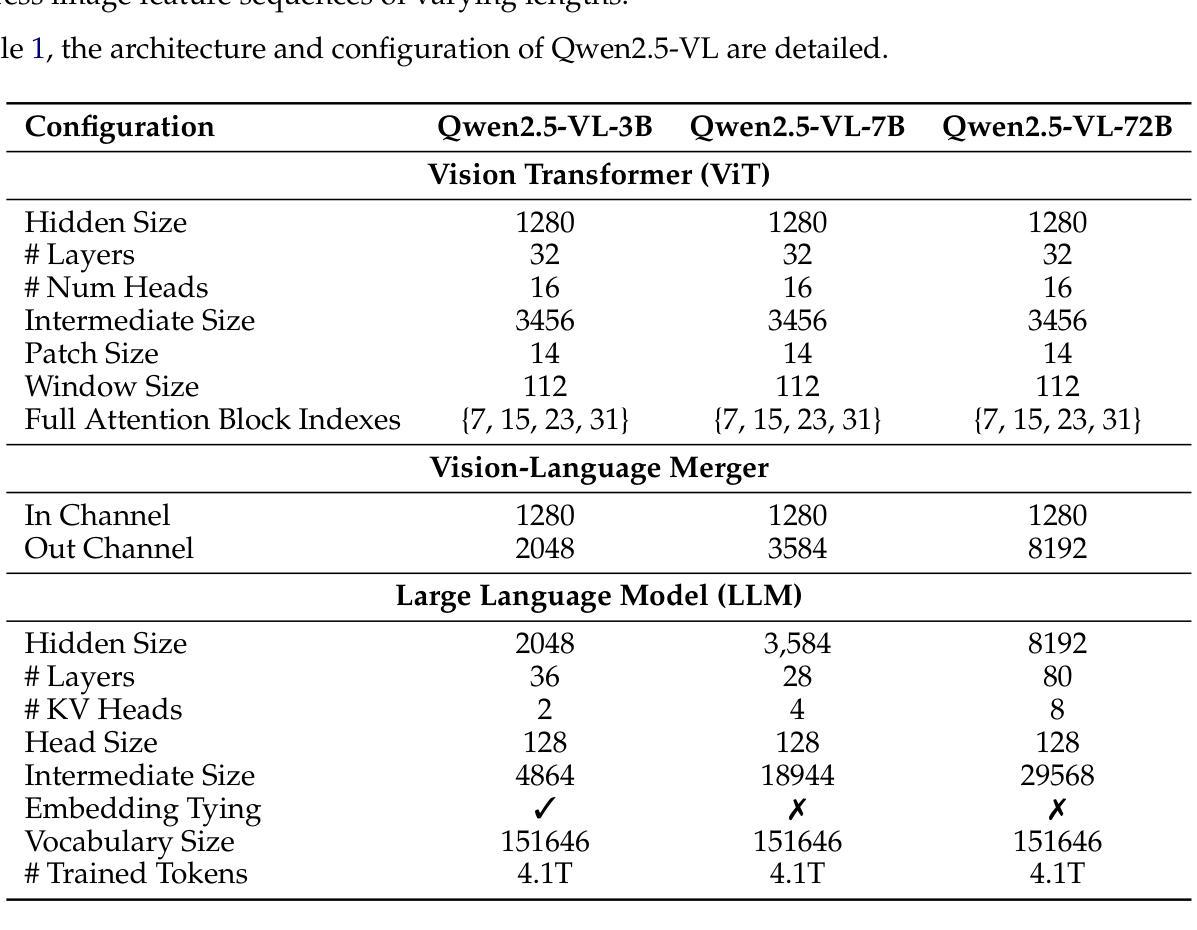
MobileViM: A Light-weight and Dimension-independent Vision Mamba for 3D Medical Image Analysis
Authors:Wei Dai, Steven Wang, Jun Liu
Efficient evaluation of three-dimensional (3D) medical images is crucial for diagnostic and therapeutic practices in healthcare. Recent years have seen a substantial uptake in applying deep learning and computer vision to analyse and interpret medical images. Traditional approaches, such as convolutional neural networks (CNNs) and vision transformers (ViTs), face significant computational challenges, prompting the need for architectural advancements. Recent efforts have led to the introduction of novel architectures like the ``Mamba’’ model as alternative solutions to traditional CNNs or ViTs. The Mamba model excels in the linear processing of one-dimensional data with low computational demands. However, Mamba’s potential for 3D medical image analysis remains underexplored and could face significant computational challenges as the dimension increases. This manuscript presents MobileViM, a streamlined architecture for efficient segmentation of 3D medical images. In the MobileViM network, we invent a new dimension-independent mechanism and a dual-direction traversing approach to incorporate with a vision-Mamba-based framework. MobileViM also features a cross-scale bridging technique to improve efficiency and accuracy across various medical imaging modalities. With these enhancements, MobileViM achieves segmentation speeds exceeding 90 frames per second (FPS) on a single graphics processing unit (i.e., NVIDIA RTX 4090). This performance is over 24 FPS faster than the state-of-the-art deep learning models for processing 3D images with the same computational resources. In addition, experimental evaluations demonstrate that MobileViM delivers superior performance, with Dice similarity scores reaching 92.72%, 86.69%, 80.46%, and 77.43% for PENGWIN, BraTS2024, ATLAS, and Toothfairy2 datasets, respectively, which significantly surpasses existing models.
高效评估三维(3D)医学影像对于医疗诊断和治疗实践至关重要。近年来,深度学习和计算机视觉在医学图像分析和解读方面的应用受到了广泛关注。传统的卷积神经网络(CNNs)和视觉转换器(ViTs)面临着巨大的计算挑战,促使对架构进步的迫切需求。近期的研究工作引入了诸如“Mamba”模型等新型架构,作为对传统CNNs或ViTs的替代解决方案。Mamba模型在处理一维数据的线性方面表现出卓越的性能,并且计算需求较低。然而,Mamba在3D医学图像分析方面的潜力尚未得到充分探索,随着维度的增加,可能会面临重大的计算挑战。
论文及项目相关链接
PDF The code is accessible through: https://github.com/anthonyweidai/MobileViM_3D/
Summary
本文提出了MobileViM架构,这是一种用于高效处理三维医学图像分割的模型。该模型引入了新的维度独立机制和双向遍历方法,结合基于视觉Mamba的框架。MobileViM还采用跨尺度桥接技术,提高了不同医学影像模态的效率和准确性。其性能超过现有深度学习模型,可在单个图形处理单元上实现超过每秒90帧的分割速度,同时实现较高的Dice相似性得分。
Key Takeaways
- MobileViM是一个针对三维医学图像分割的模型架构,旨在高效处理医学图像。
- MobileViM引入了新的维度独立机制和双向遍历方法,以提高处理效率。
- 该模型结合了视觉Mamba的框架,并采用跨尺度桥接技术来提高准确性和效率。
- MobileViM实现了每秒超过90帧的分割速度,超过了现有深度学习模型的性能。
- MobileViM在多个数据集上的Dice相似性得分较高,显示出其优越性。
- 该模型在处理三维医学图像方面具有潜力,但需要更多的研究来探索其在其他医学影像模态中的应用。
点此查看论文截图
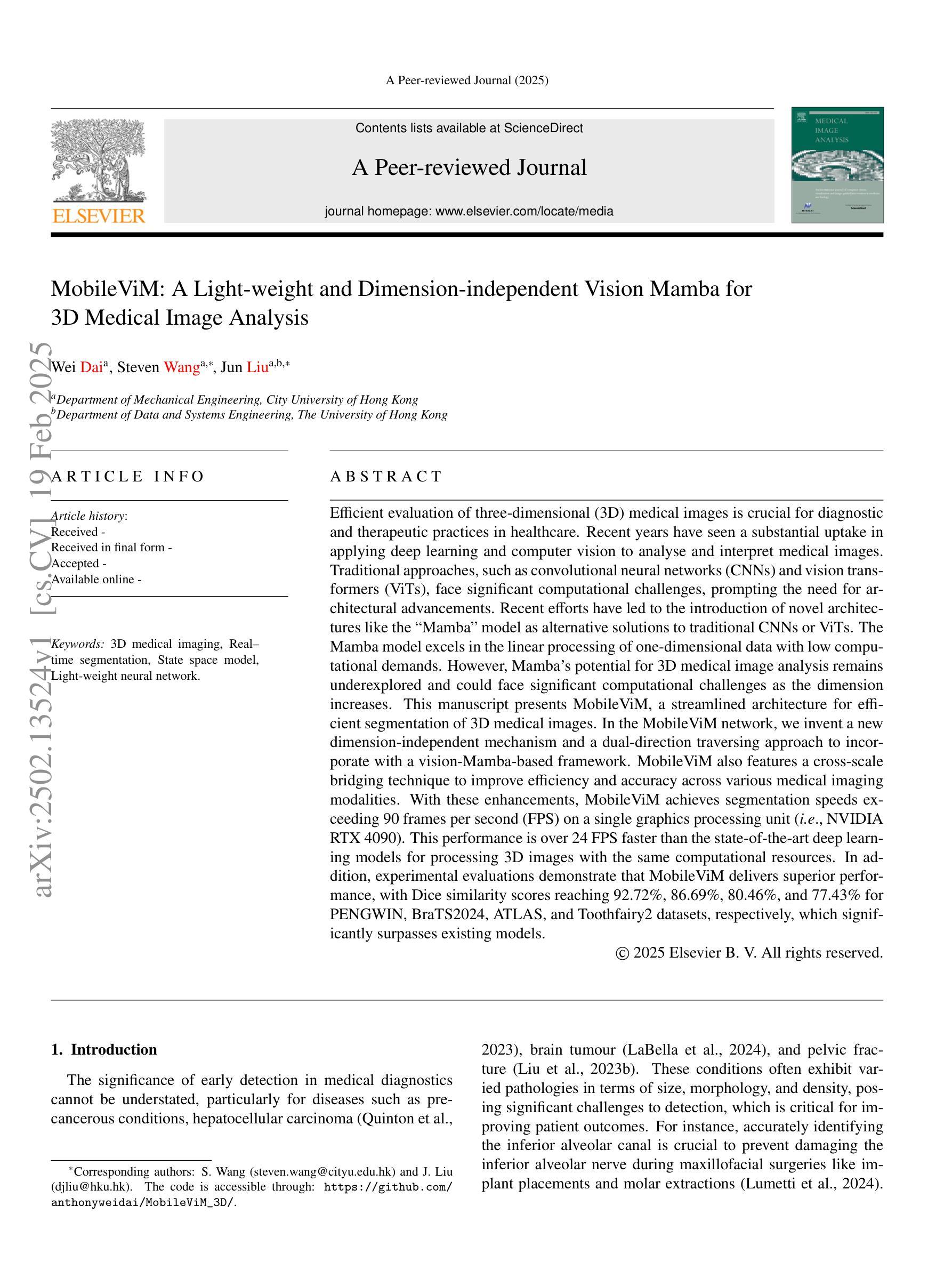
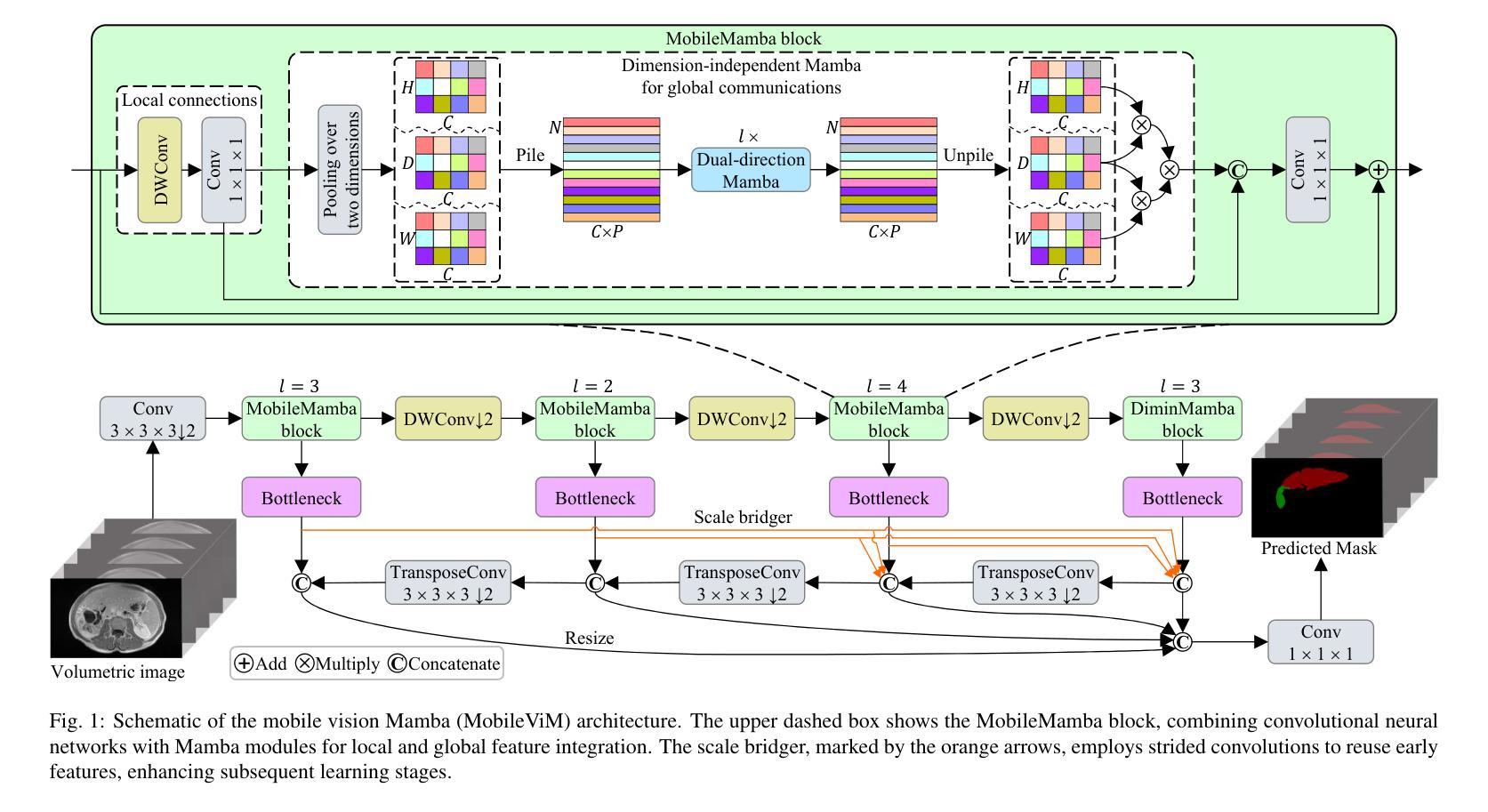
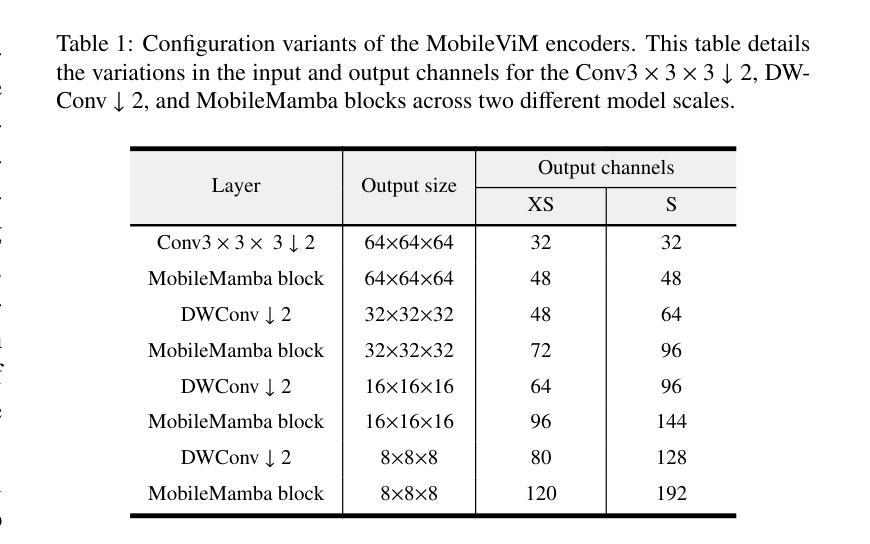
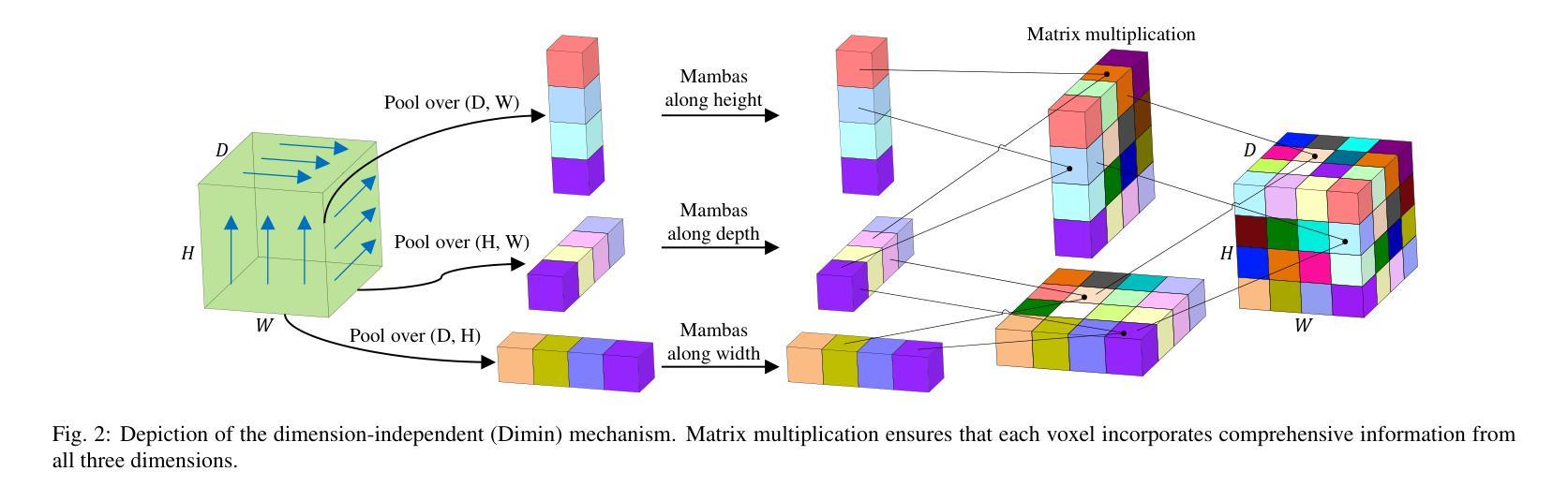
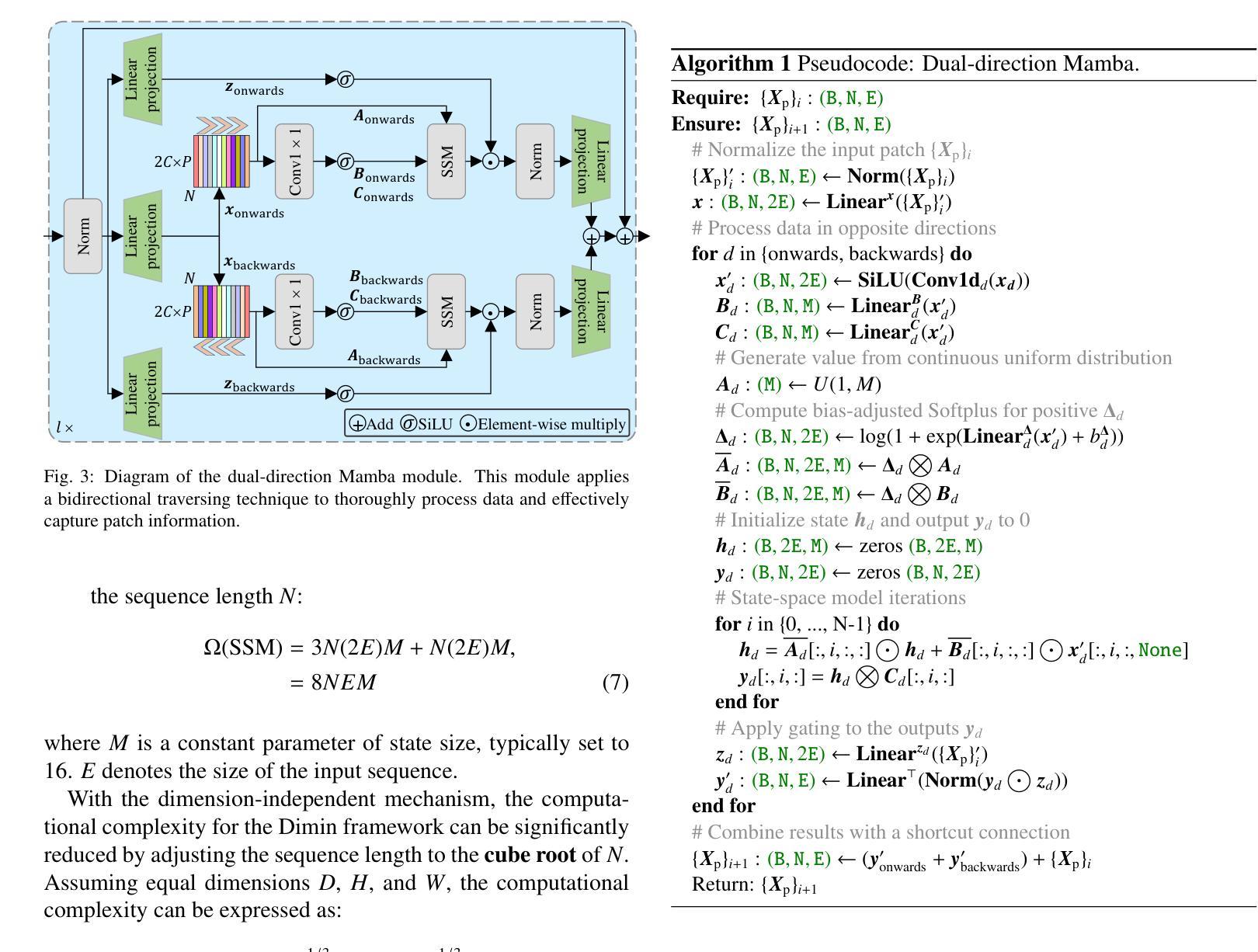
Multiview Equivariance Improves 3D Correspondence Understanding with Minimal Feature Finetuning
Authors:Yang You, Yixin Li, Congyue Deng, Yue Wang, Leonidas Guibas
Vision foundation models, particularly the ViT family, have revolutionized image understanding by providing rich semantic features. However, despite their success in 2D comprehension, their abilities on grasping 3D spatial relationships are still unclear. In this work, we evaluate and enhance the 3D awareness of ViT-based models. We begin by systematically assessing their ability to learn 3D equivariant features, specifically examining the consistency of semantic embeddings across different viewpoints. Our findings indicate that improved 3D equivariance leads to better performance on various downstream tasks, including pose estimation, tracking, and semantic transfer. Building on this insight, we propose a simple yet effective finetuning strategy based on 3D correspondences, which significantly enhances the 3D correspondence understanding of existing vision models. Remarkably, finetuning on a single object for one iteration results in substantial gains. Our code is available at https://github.com/qq456cvb/3DCorrEnhance.
视觉基础模型,特别是ViT系列,通过提供丰富的语义特征,已经实现了对图像理解的革新。然而,尽管它们在2D理解方面取得了成功,但在把握3D空间关系方面的能力仍不明确。在这项工作中,我们评估并提高了基于ViT模型的3D意识。首先,我们系统地评估了它们学习3D等价特征的能力,特别检查了不同观点下语义嵌入的一致性。我们的研究结果表明,提高3D等价性有助于提高各种下游任务的性能,包括姿态估计、跟踪和语义转移。基于这一发现,我们提出了一种简单有效的基于3D对应的微调策略,这显著提高了现有视觉模型的3D对应理解。值得注意的是,对单个对象进行单次迭代的微调即可实现显著的提升。我们的代码可在https://github.com/qq456cvb/3DCorrEnhance找到。
论文及项目相关链接
PDF 10 pages; Accepted to ICLR 2025
Summary
ViT家族模型在图像理解方面表现出强大的语义特征提取能力,但在3D空间关系理解方面仍存在不足。本文旨在评估和提升ViT模型的3D感知能力,通过系统研究其在不同视角下的语义嵌入一致性来验证模型的3D等变性。研究发现,提高模型的3D等变性有助于提高姿态估计、跟踪和语义迁移等下游任务的表现。基于此,本文提出了一种基于3D对应关系的简单有效的微调策略,能显著提升现有视觉模型的3D对应理解能力。仅对一个物体进行一次迭代微调即可实现显著的提升。
Key Takeaways
- ViT模型在图像理解方面具有强大的语义特征提取能力,但在处理3D空间关系时表现不足。
- 系统评估了ViT模型在不同视角下的语义嵌入一致性,验证了模型的3D等变性。
- 提高模型的3D等变性有助于提高姿态估计、跟踪和语义迁移等下游任务的表现。
- 提出了一种基于3D对应关系的简单有效的微调策略,增强现有视觉模型的3D理解能力。
- 通过对单一物体的一次迭代微调,即可实现显著的性能提升。
- 模型的改进和研究成果在公开代码库(https://github.com/qq456cvb/3DCorrEnhance)中可供查阅和使用。
点此查看论文截图
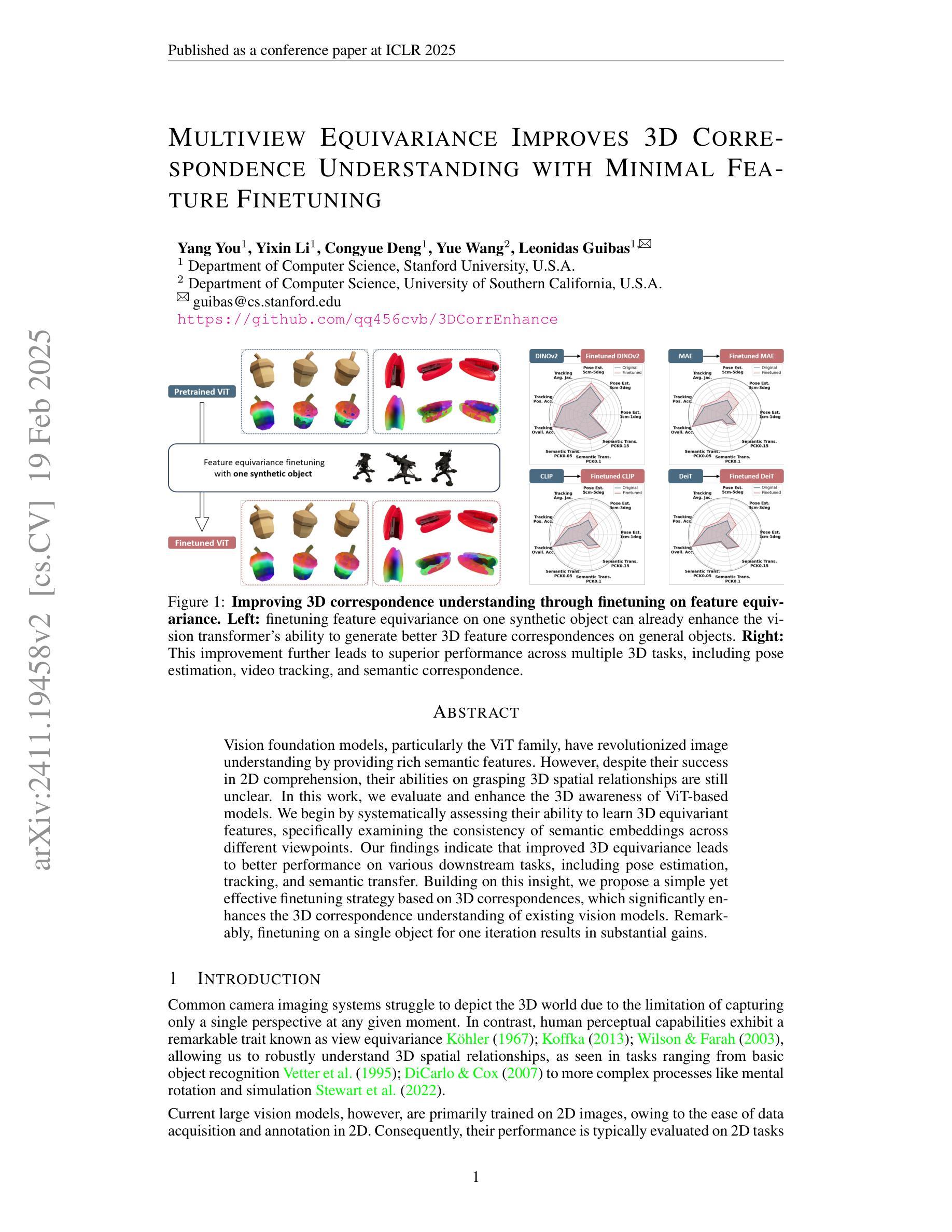
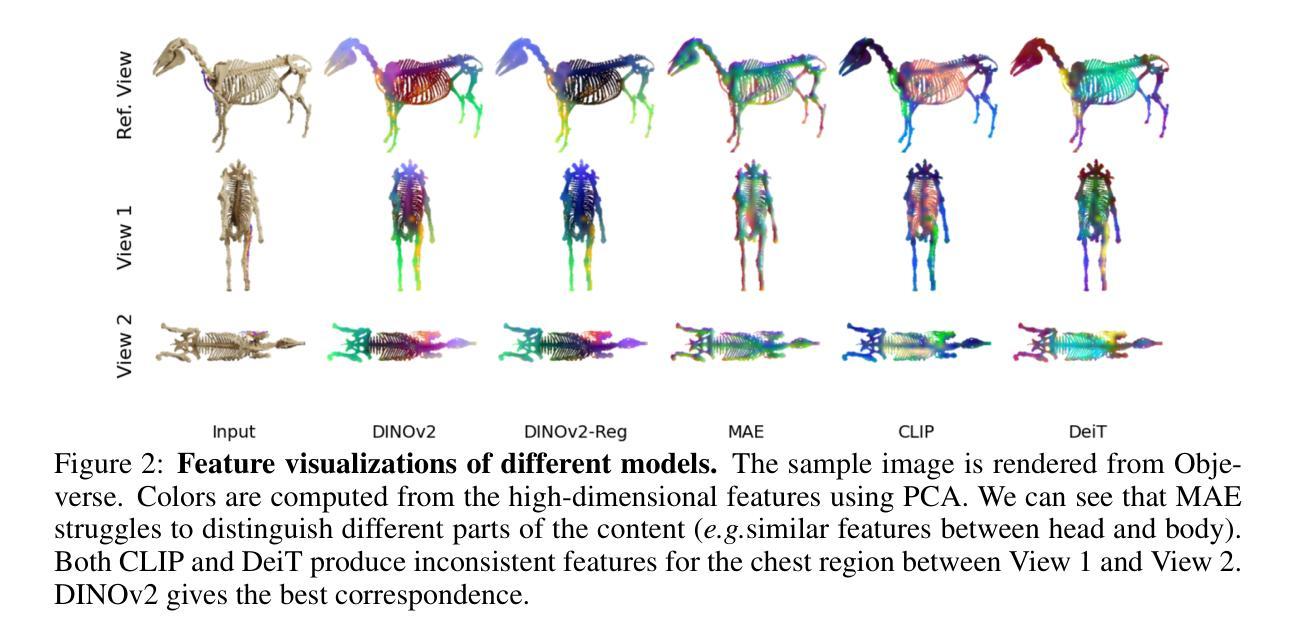
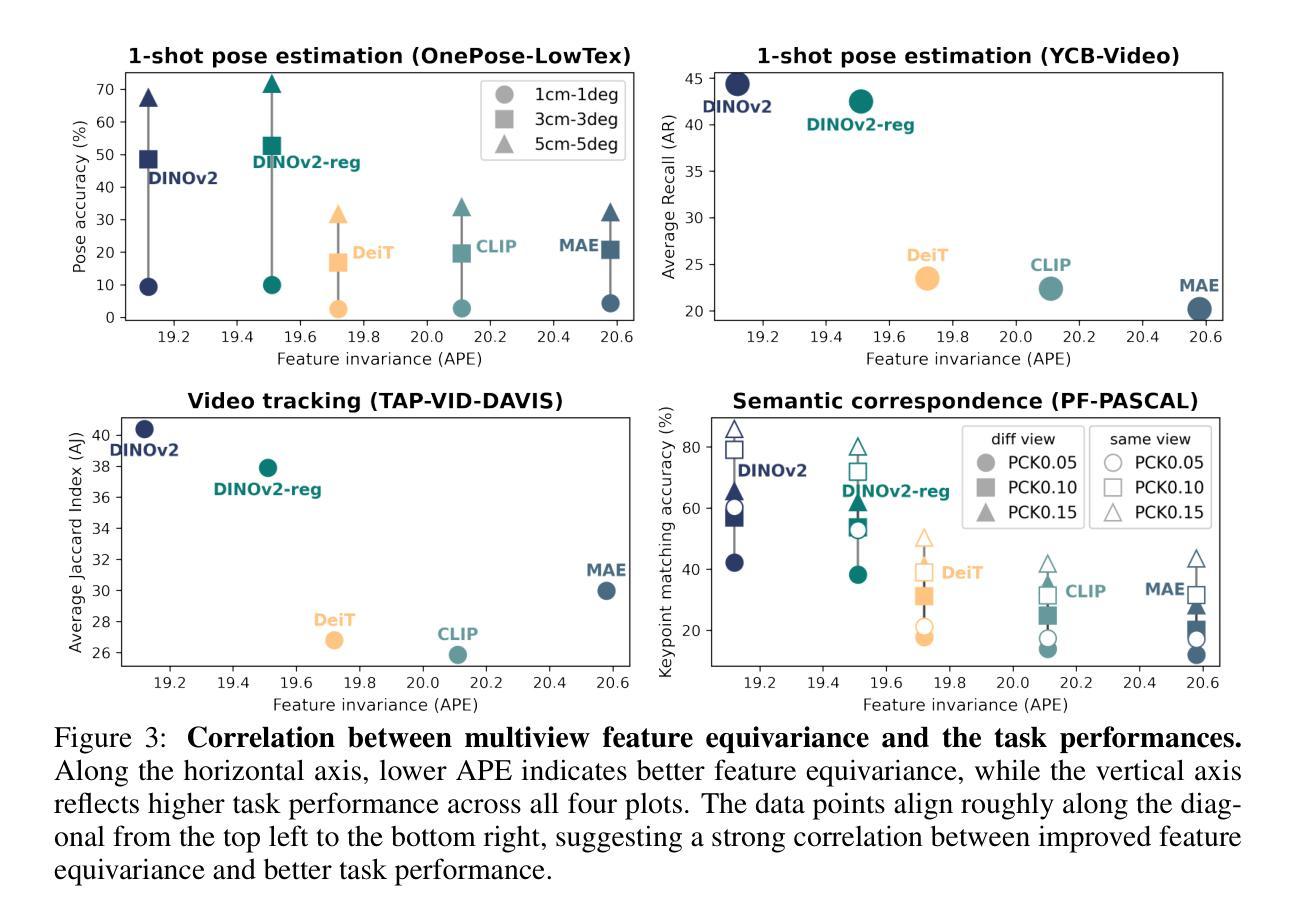

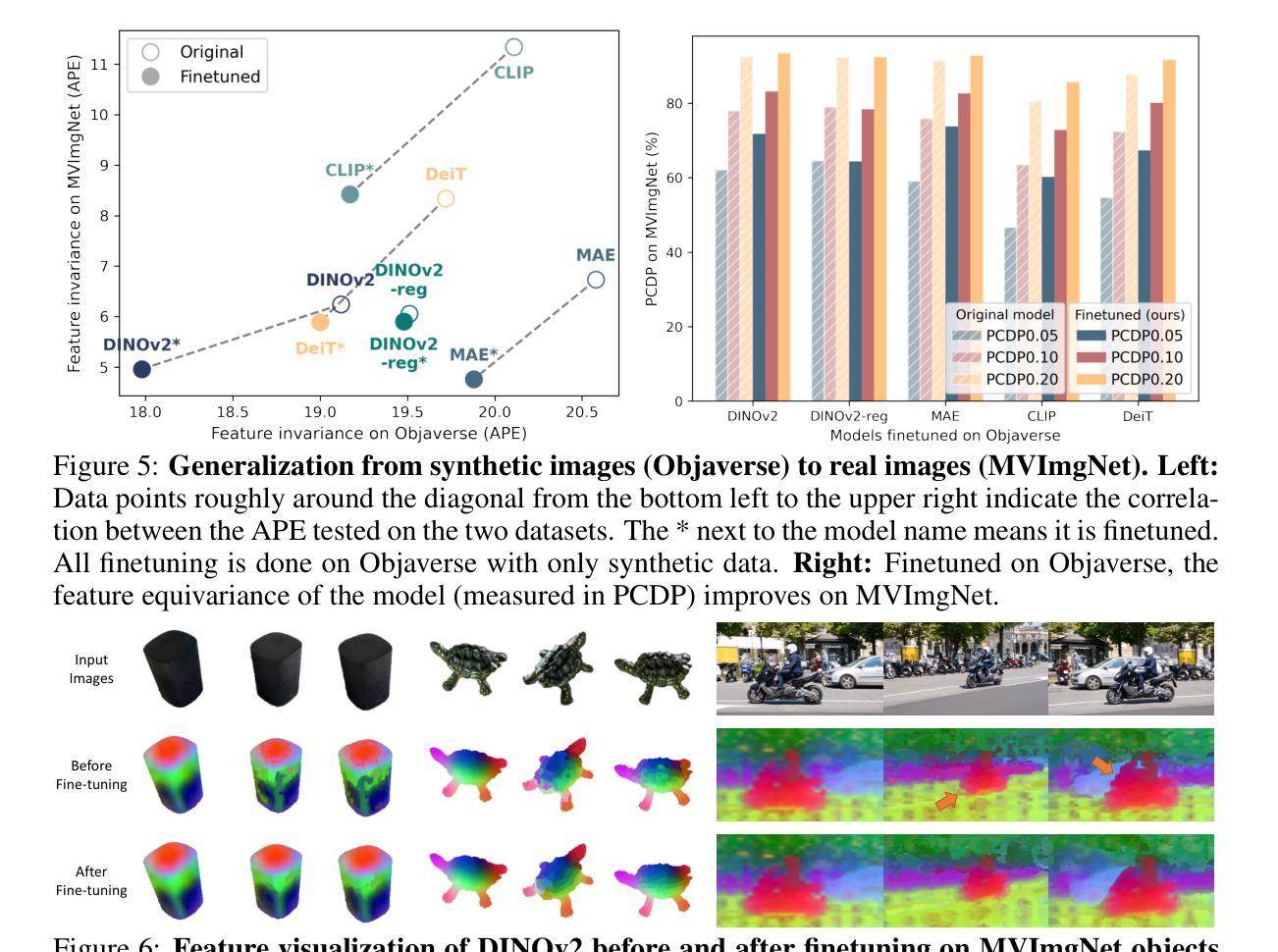