⚠️ 以下所有内容总结都来自于 大语言模型的能力,如有错误,仅供参考,谨慎使用
🔴 请注意:千万不要用于严肃的学术场景,只能用于论文阅读前的初筛!
💗 如果您觉得我们的项目对您有帮助 ChatPaperFree ,还请您给我们一些鼓励!⭐️ HuggingFace免费体验
2025-03-27 更新
AvatarArtist: Open-Domain 4D Avatarization
Authors:Hongyu Liu, Xuan Wang, Ziyu Wan, Yue Ma, Jingye Chen, Yanbo Fan, Yujun Shen, Yibing Song, Qifeng Chen
This work focuses on open-domain 4D avatarization, with the purpose of creating a 4D avatar from a portrait image in an arbitrary style. We select parametric triplanes as the intermediate 4D representation and propose a practical training paradigm that takes advantage of both generative adversarial networks (GANs) and diffusion models. Our design stems from the observation that 4D GANs excel at bridging images and triplanes without supervision yet usually face challenges in handling diverse data distributions. A robust 2D diffusion prior emerges as the solution, assisting the GAN in transferring its expertise across various domains. The synergy between these experts permits the construction of a multi-domain image-triplane dataset, which drives the development of a general 4D avatar creator. Extensive experiments suggest that our model, AvatarArtist, is capable of producing high-quality 4D avatars with strong robustness to various source image domains. The code, the data, and the models will be made publicly available to facilitate future studies..
本文重点关注开放域4D化身技术,旨在从任意风格的肖像图像中创建4D化身。我们选择参数化三平面作为中间4D表示,并提出了一种实用的训练范式,该范式结合了生成对抗网络(GANs)和扩散模型。我们的设计灵感来自于一个观察结果,即4D GANs在无监督的情况下在桥接图像和三平面方面表现出色,但在处理多样数据分布时通常面临挑战。一个稳健的2D扩散先验作为一个解决方案出现,它可以帮助GAN在不同的领域之间转移其专业知识。这些专家之间的协同作用使得构建多域图像-三平面数据集成为可能,从而推动了通用4D化身创作者的发展。大量实验表明,我们的模型AvatarArtist能够生成高质量的4D化身,对各种源图像领域具有很强的稳健性。代码、数据和模型将公开提供,以方便未来研究。
论文及项目相关链接
PDF Accepted to CVPR 2025
摘要
本文关注开放域4D动态个性化角色的创建,旨在从任意风格的肖像图片生成4D动态个性化角色。研究采用参数化triplanes作为中间4D表示形式,并提出一种结合生成对抗网络(GANs)和扩散模型的实用训练范式。设计灵感来源于对GANs的观察,它能无监督地实现图像和triplanes之间的桥梁作用,但在处理多样数据分布时面临挑战。一个稳健的二维扩散先验成为解决方案,帮助GAN在不同领域之间转移知识。这些专家之间的协同作用使得构建多域图像-triplane数据集成为可能,从而推动了通用4D动态个性化角色创建器的发展。实验表明,AvatarArtist模型能够生成高质量的4D动态个性化角色,对各种源图像领域具有较强的鲁棒性。代码、数据和模型将公开提供,以便于后续研究。
关键见解
- 本研究关注开放域4D动态个性化角色的创建,旨在从肖像图片生成4D角色。
- 研究采用参数化triplanes作为中间形式进行表示。
- 研究结合了生成对抗网络(GANs)和扩散模型来构建训练范式。
- GAN在处理多样数据分布时存在挑战,提出利用稳健的二维扩散先验来帮助提高模型性能。
- 实现了不同领域的图像和triplanes之间的协同作用,推动了多域图像-triplane数据集的构建。
- 研究开发了名为AvatarArtist的模型,能够生成高质量的4D动态个性化角色。
点此查看论文截图
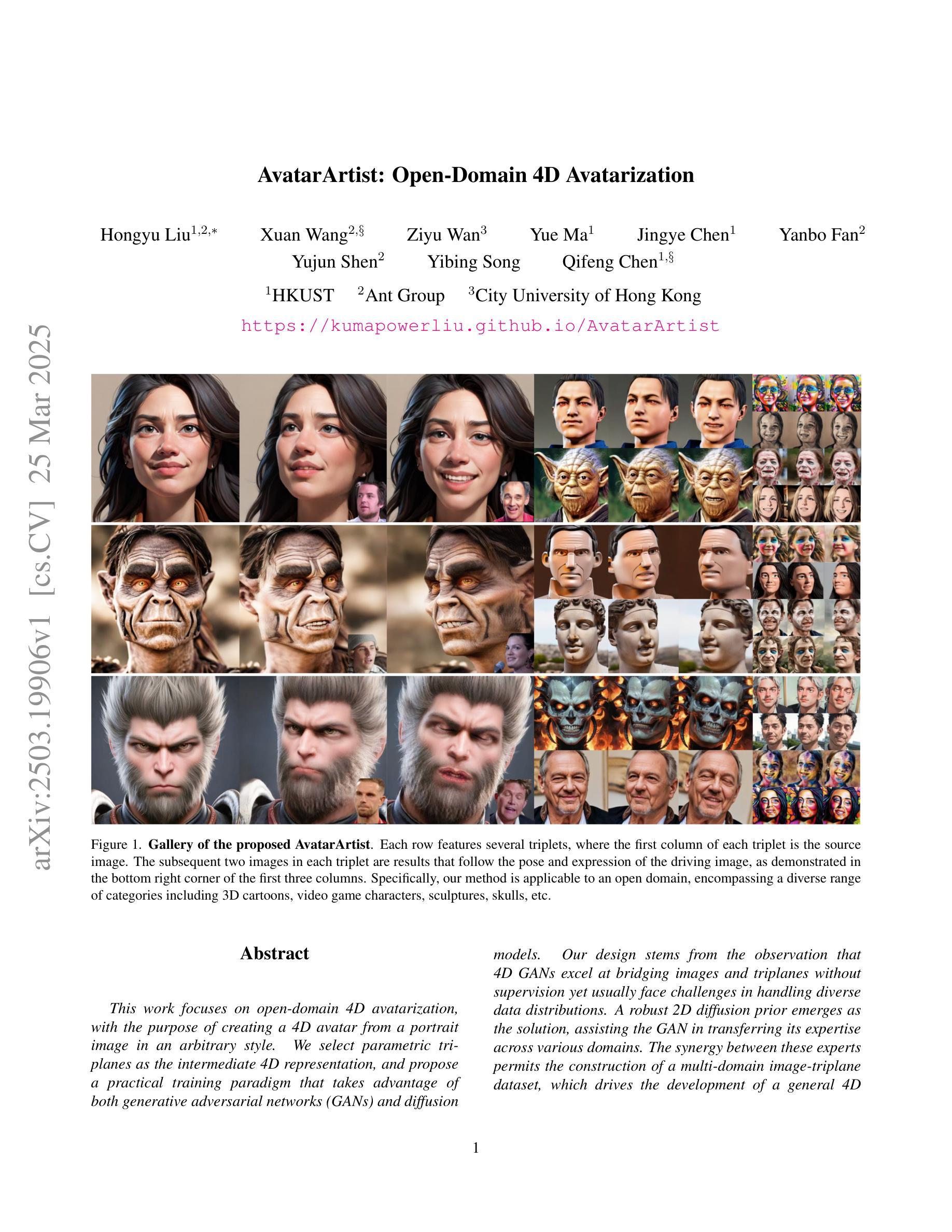
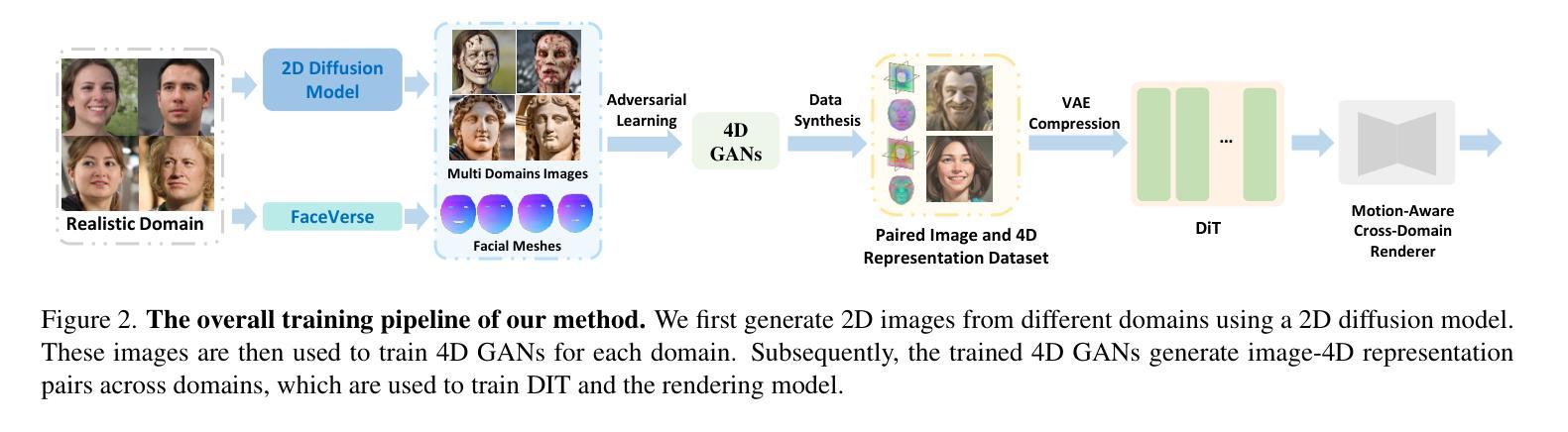
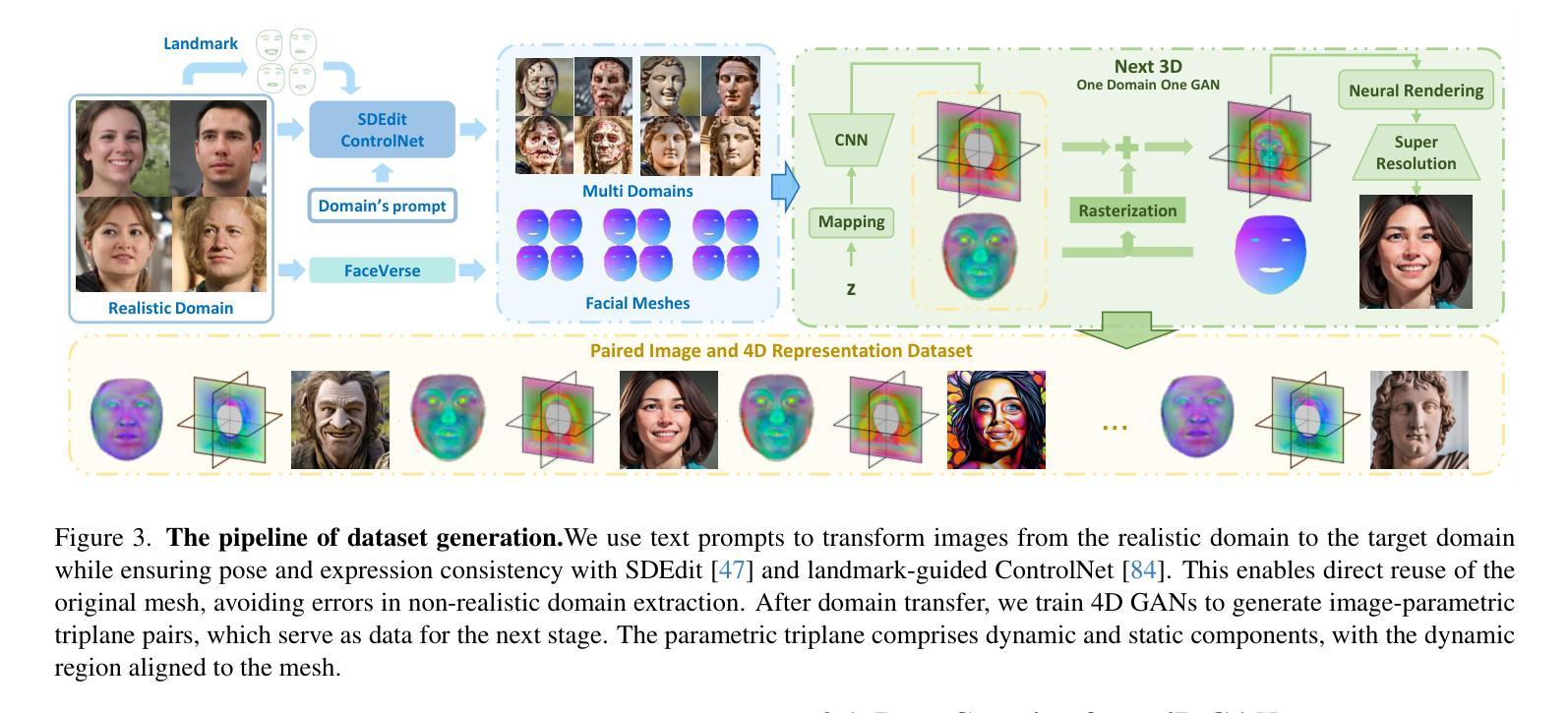
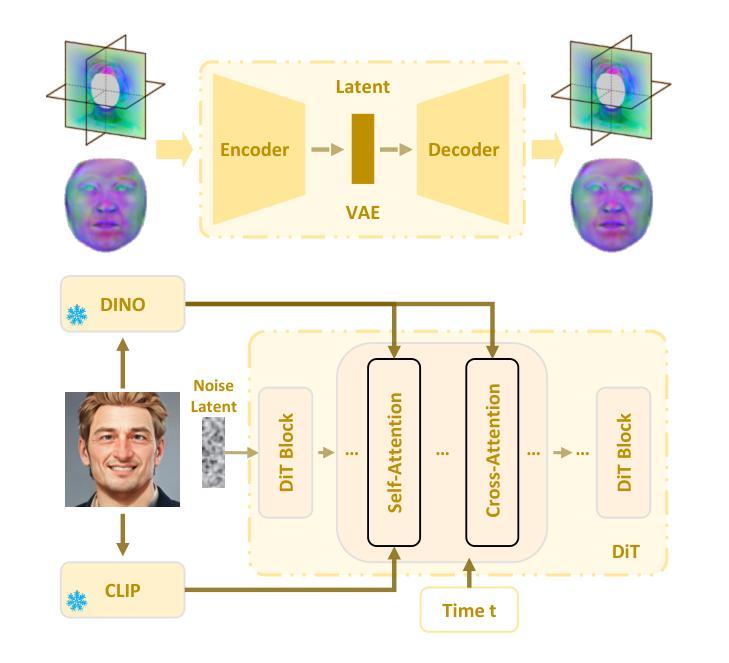
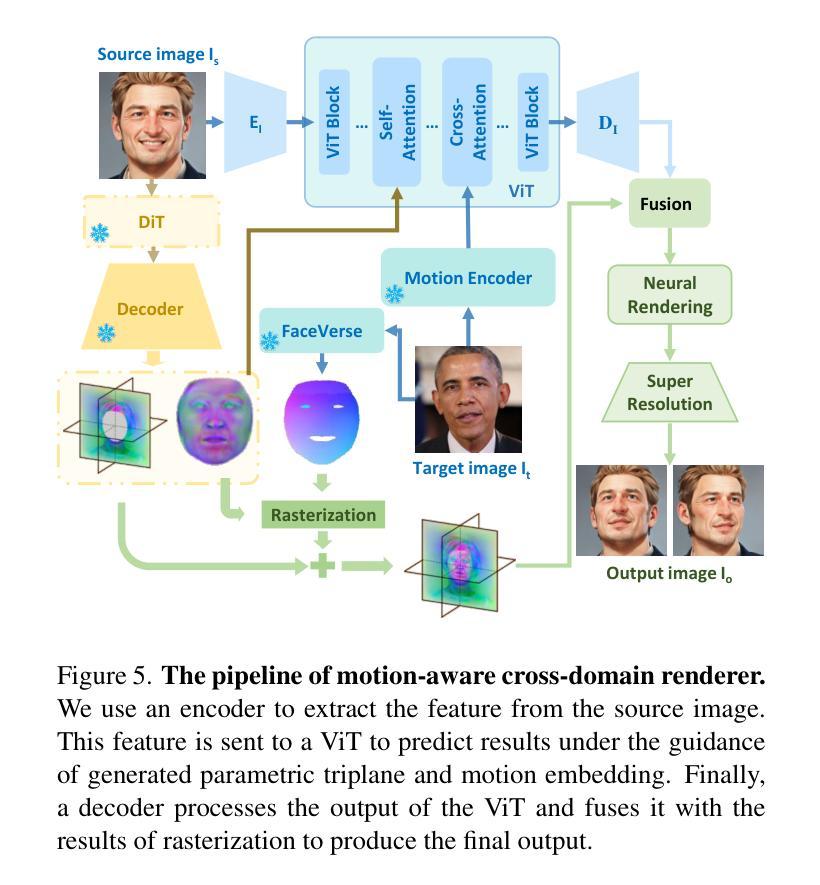
FRESA:Feedforward Reconstruction of Personalized Skinned Avatars from Few Images
Authors:Rong Wang, Fabian Prada, Ziyan Wang, Zhongshi Jiang, Chengxiang Yin, Junxuan Li, Shunsuke Saito, Igor Santesteban, Javier Romero, Rohan Joshi, Hongdong Li, Jason Saragih, Yaser Sheikh
We present a novel method for reconstructing personalized 3D human avatars with realistic animation from only a few images. Due to the large variations in body shapes, poses, and cloth types, existing methods mostly require hours of per-subject optimization during inference, which limits their practical applications. In contrast, we learn a universal prior from over a thousand clothed humans to achieve instant feedforward generation and zero-shot generalization. Specifically, instead of rigging the avatar with shared skinning weights, we jointly infer personalized avatar shape, skinning weights, and pose-dependent deformations, which effectively improves overall geometric fidelity and reduces deformation artifacts. Moreover, to normalize pose variations and resolve coupled ambiguity between canonical shapes and skinning weights, we design a 3D canonicalization process to produce pixel-aligned initial conditions, which helps to reconstruct fine-grained geometric details. We then propose a multi-frame feature aggregation to robustly reduce artifacts introduced in canonicalization and fuse a plausible avatar preserving person-specific identities. Finally, we train the model in an end-to-end framework on a large-scale capture dataset, which contains diverse human subjects paired with high-quality 3D scans. Extensive experiments show that our method generates more authentic reconstruction and animation than state-of-the-arts, and can be directly generalized to inputs from casually taken phone photos. Project page and code is available at https://github.com/rongakowang/FRESA.
我们提出了一种仅通过几张图片重建具有真实动画效果的个性化3D人类化身的新方法。由于人体形状、姿势和服装类型的巨大差异,现有方法大多需要在推理过程中对每个主题进行数小时的优化,这限制了它们的实际应用。相比之下,我们从数千名穿衣者身上学习通用先验知识,以实现即时前馈生成和零样本泛化。具体来说,我们不是用共享的蒙皮权重来搭建化身,而是联合推断个性化的化身形状、蒙皮权重和姿势相关的变形,这有效地提高了整体几何保真度并减少了变形伪影。此外,为了归一化姿势变化和解决规范形状和蒙皮权重之间的耦合模糊性,我们设计了一个3D规范化过程来生成像素对齐的初始条件,这有助于重建精细的几何细节。然后,我们提出了一种多帧特征聚合方法,以稳健地减少规范化过程中产生的伪影,并融合保留个人特定身份的可行化身。最后,我们在一个大规模捕获数据集上采用端到端框架进行模型训练,该数据集包含与高质量3D扫描配对的多样化人类主题。大量实验表明,我们的方法比最先进的技术生成更真实的重建和动画,并且可以直观地推广到来自随意拍摄的手机照片输入。项目页面和代码位于https://github.com/rongakowang/FRESA。
论文及项目相关链接
PDF Published in CVPR 2025
Summary
新一代个性化三维人类虚拟角色重建方法:采用深度学习技术,从少量图片中重建出具有高度真实感的动画角色。该方法通过通用先验学习,实现了即时前馈生成与零样本泛化,优化了角色形状、皮肤贴合权重和姿态变形联合推断,提高了几何精度并降低了变形失真。通过设计3D标准化流程解决姿态变化和耦合模糊问题,生成精细几何细节。使用多帧特征聚合技术降低标准化产生的伪影,并保持个性化身份。在大型捕捉数据集上进行端到端框架训练,与现有技术相比生成更真实的三维人类动画。
Key Takeaways
- 提出了一种新的个性化三维人类虚拟角色重建方法,能从少量图片中重建出真实感动画角色。
- 通过通用先验学习实现即时前馈生成与零样本泛化,提高了重建效率。
- 优化了角色形状、皮肤贴合权重和姿态变形的联合推断,提高了几何精度。
- 设计的3D标准化流程能解决姿态变化和耦合模糊问题,生成精细几何细节。
- 采用多帧特征聚合技术降低标准化产生的伪影,保持个性化身份。
- 在大型捕捉数据集上进行端到端框架训练,确保模型的准确性。
点此查看论文截图
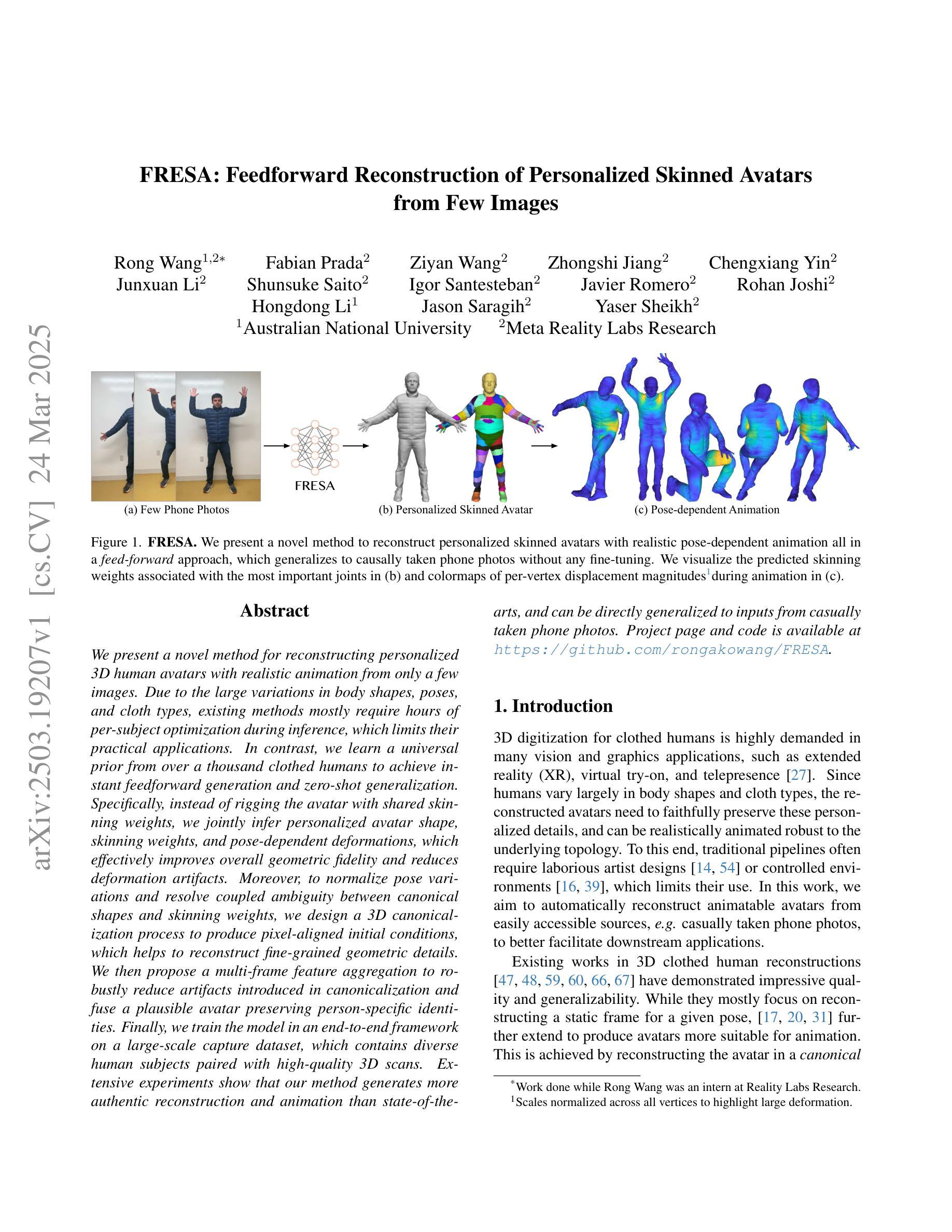
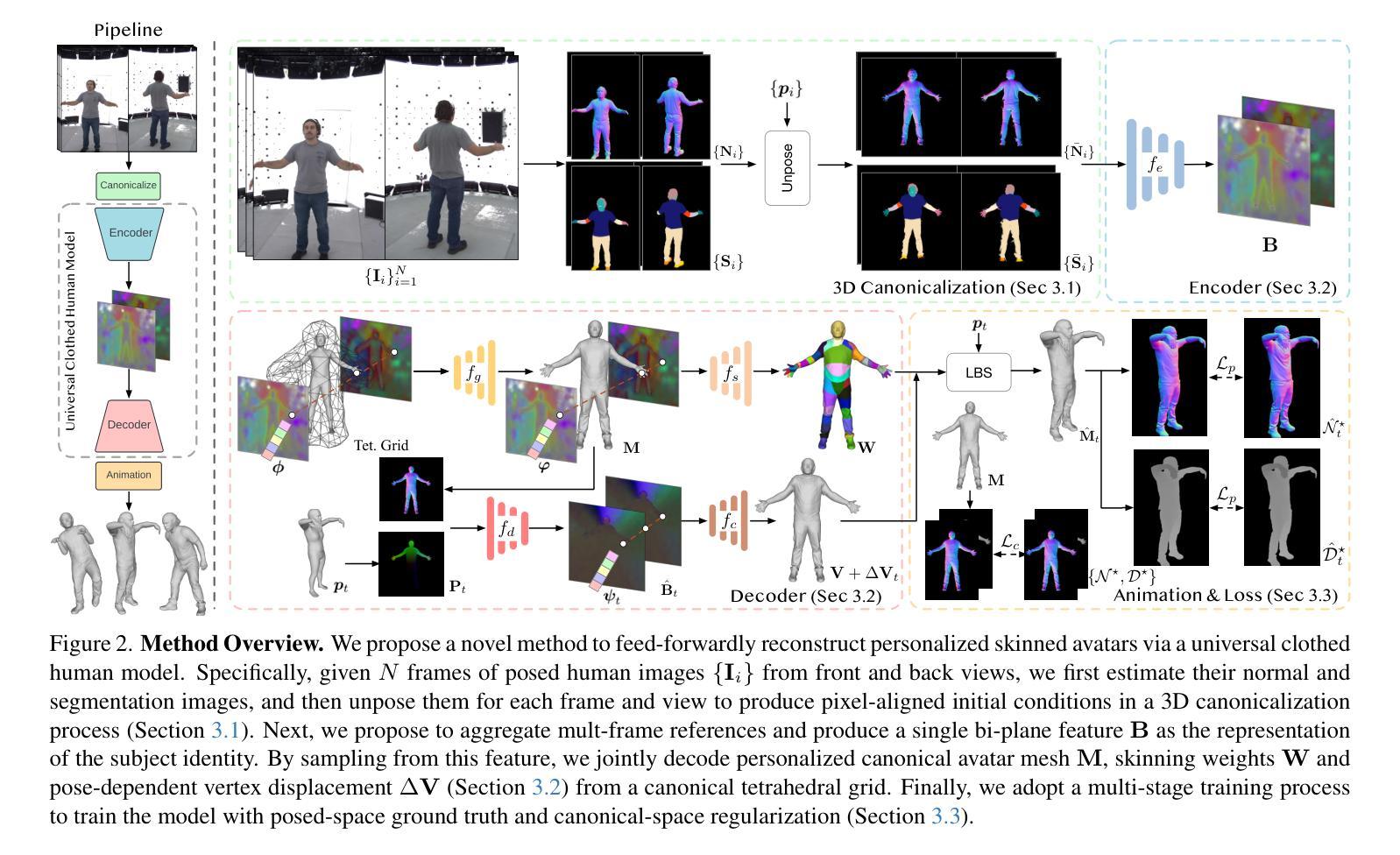
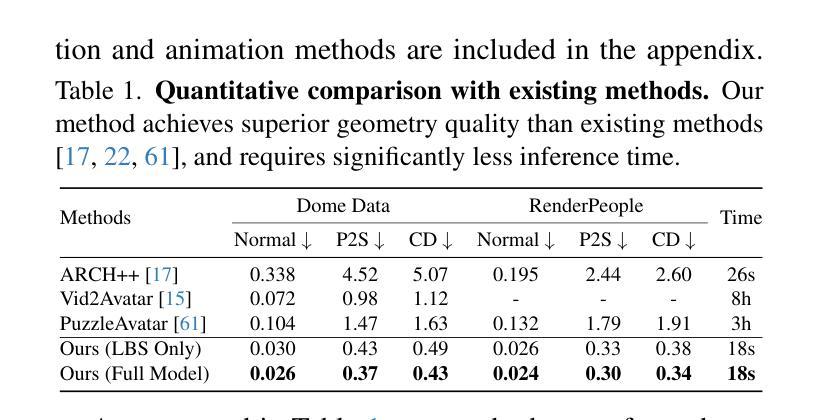
MetaSpatial: Reinforcing 3D Spatial Reasoning in VLMs for the Metaverse
Authors:Zhenyu Pan, Han Liu
We present MetaSpatial, the first reinforcement learning (RL)-based framework designed to enhance 3D spatial reasoning in vision-language models (VLMs), enabling real-time 3D scene generation without the need for hard-coded optimizations. MetaSpatial addresses two core challenges: (i) the lack of internalized 3D spatial reasoning in VLMs, which limits their ability to generate realistic layouts, and (ii) the inefficiency of traditional supervised fine-tuning (SFT) for layout generation tasks, as perfect ground truth annotations are unavailable. Our key innovation is a multi-turn RL-based optimization mechanism that integrates physics-aware constraints and rendered image evaluations, ensuring generated 3D layouts are coherent, physically plausible, and aesthetically consistent. Methodologically, MetaSpatial introduces an adaptive, iterative reasoning process, where the VLM refines spatial arrangements over multiple turns by analyzing rendered outputs, improving scene coherence progressively. Empirical evaluations demonstrate that MetaSpatial significantly enhances the spatial consistency and formatting stability of various scale models. Post-training, object placements are more realistic, aligned, and functionally coherent, validating the effectiveness of RL for 3D spatial reasoning in metaverse, AR/VR, digital twins, and game development applications. Our code, data, and training pipeline are publicly available at https://github.com/PzySeere/MetaSpatial.
我们推出MetaSpatial,这是首个基于强化学习(RL)的框架,旨在增强视觉语言模型(VLMs)的3D空间推理能力,实现无需硬编码优化的实时3D场景生成。MetaSpatial解决了两个核心挑战:(i)VLMs内部缺乏3D空间推理能力,限制了其生成真实布局的能力;(ii)对于布局生成任务,传统的监督微调(SFT)效率低下,因为完美的真实标注不可用。我们的关键创新在于采用基于多回合的强化学习优化机制,该机制结合了物理感知约束和渲染图像评估,确保生成的3D布局连贯、物理上合理且审美上一致。方法上,MetaSpatial引入了一种自适应的迭代推理过程,VLM通过分析渲染输出来完善空间布局,逐步改进场景的一致性。经验评估表明,MetaSpatial显著提高了各种规模模型的空间一致性和格式稳定性。训练后,对象放置更加真实、对齐且功能连贯,验证了强化学习在元宇宙、AR/VR、数字孪生和游戏开发应用程序中的3D空间推理的有效性。我们的代码、数据和训练管道可在https://github.com/PzySeere/MetaSpatial公开获取。
论文及项目相关链接
PDF Working Paper
Summary:
MetaSpatial是一个基于强化学习的框架,旨在提高视觉语言模型在元宇宙中的三维空间推理能力,实现实时三维场景生成,无需硬编码优化。它通过引入多回合的强化学习优化机制解决了两大难题,使得生成的三维布局更为连贯、物理可行且美学一致。这一框架在多种应用场景下均表现出卓越效果。
Key Takeaways:
- MetaSpatial是首个基于强化学习的框架,旨在增强视觉语言模型的三维空间推理能力。
- 该框架能够实现实时三维场景生成,无需硬编码优化。
- MetaSpatial解决了传统监督微调方法在处理布局生成任务时的两大难题。
- 强化学习优化机制确保了生成的三维布局的连贯性、物理可行性和美学一致性。
- 该框架引入了自适应的迭代推理过程,通过多次分析渲染输出来逐步改进场景的一致性。
- MetaSpatial在多种应用场景下表现出卓越的效果,如元宇宙、AR/VR、数字双胞胎和游戏开发等。
点此查看论文截图
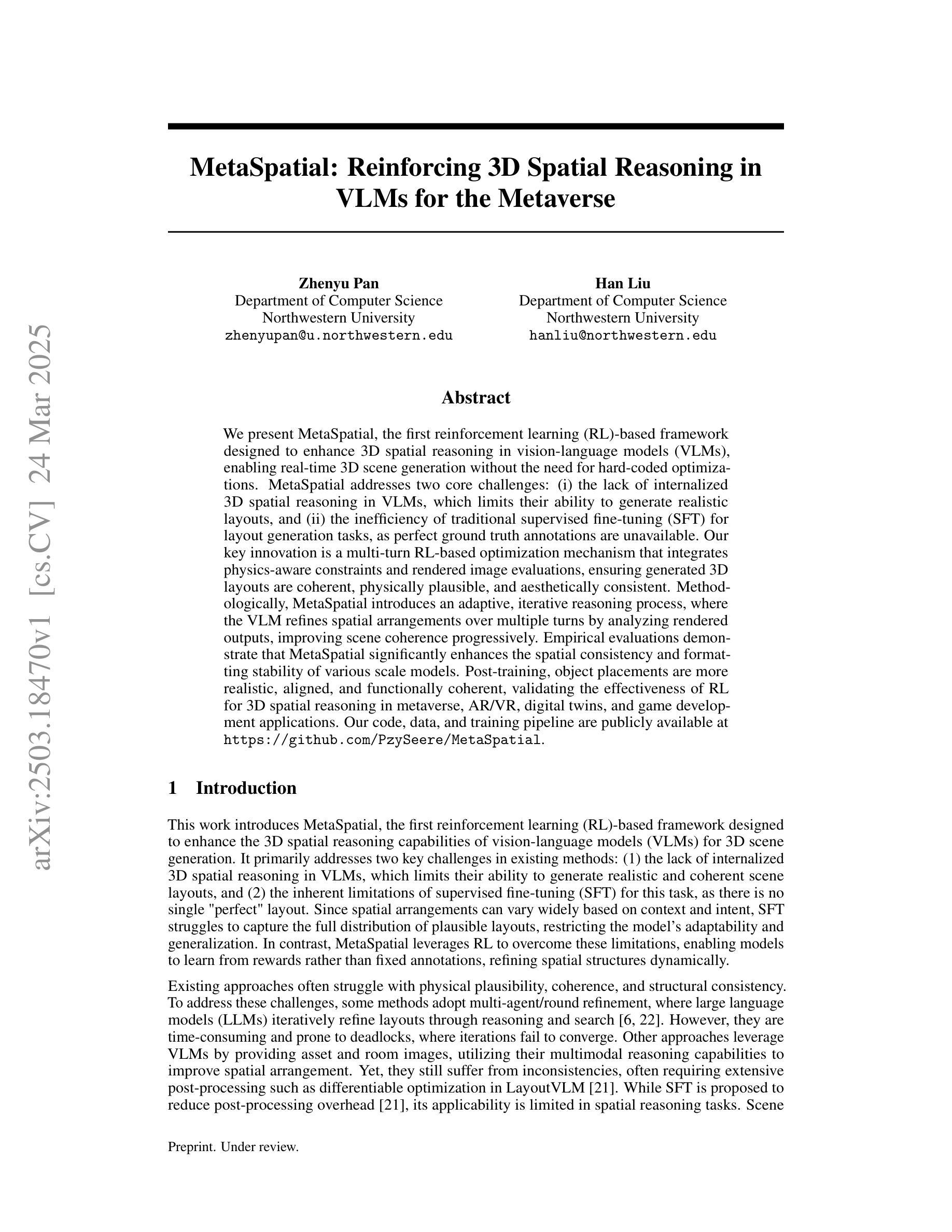
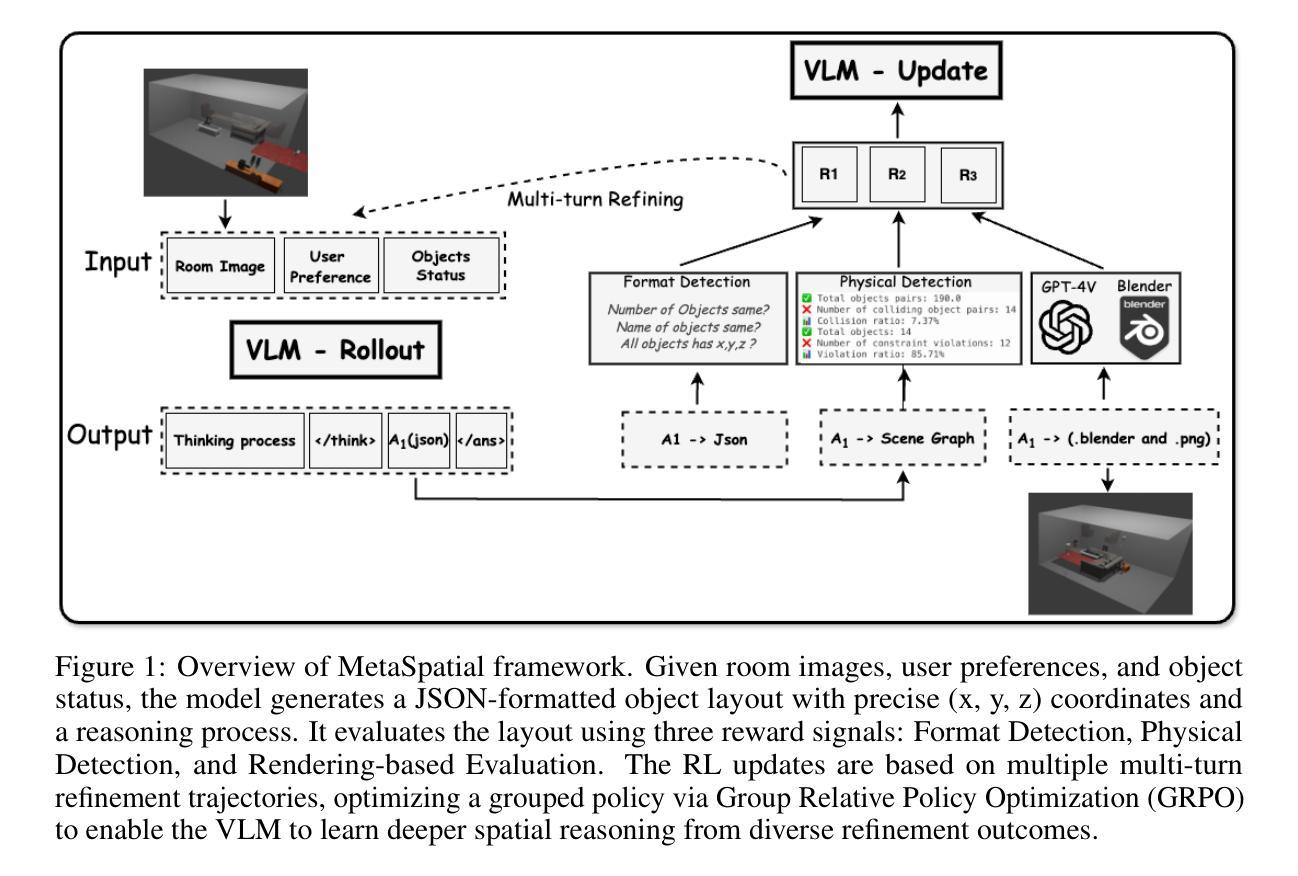
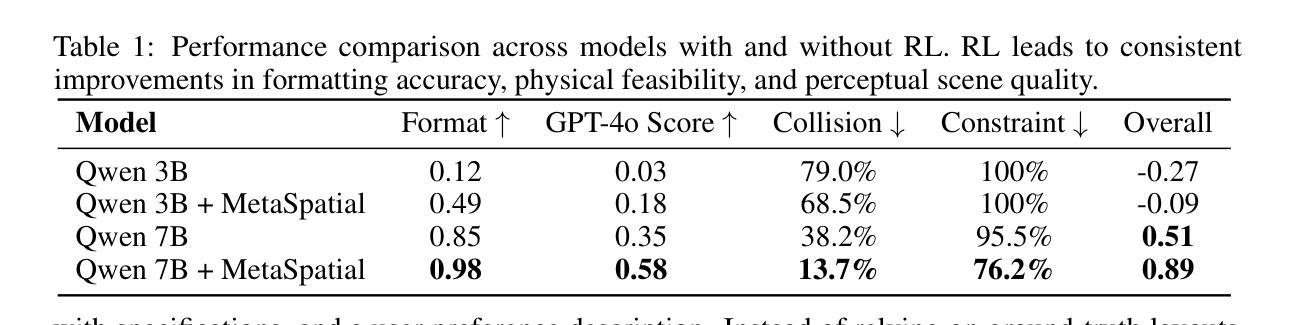
Fast and Physically-based Neural Explicit Surface for Relightable Human Avatars
Authors:Jiacheng Wu, Ruiqi Zhang, Jie Chen, Hui Zhang
Efficiently modeling relightable human avatars from sparse-view videos is crucial for AR/VR applications. Current methods use neural implicit representations to capture dynamic geometry and reflectance, which incur high costs due to the need for dense sampling in volume rendering. To overcome these challenges, we introduce Physically-based Neural Explicit Surface (PhyNES), which employs compact neural material maps based on the Neural Explicit Surface (NES) representation. PhyNES organizes human models in a compact 2D space, enhancing material disentanglement efficiency. By connecting Signed Distance Fields to explicit surfaces, PhyNES enables efficient geometry inference around a parameterized human shape model. This approach models dynamic geometry, texture, and material maps as 2D neural representations, enabling efficient rasterization. PhyNES effectively captures physical surface attributes under varying illumination, enabling real-time physically-based rendering. Experiments show that PhyNES achieves relighting quality comparable to SOTA methods while significantly improving rendering speed, memory efficiency, and reconstruction quality.
对于AR/VR应用而言,从稀疏视角的视频中有效地建模可重新照明的真实人类角色至关重要。当前的方法使用神经隐式表示来捕捉动态几何和反射,这由于在体积渲染中需要密集采样而产生高昂的成本。为了克服这些挑战,我们引入了基于物理的神经网络显式表面(PhyNES),它采用基于神经网络显式表面(NES)表示的紧凑神经网络材质图。PhyNES将人类模型组织在紧凑的二维空间中,提高了材质分离效率。通过将符号距离场与显式表面连接起来,PhyNES能够在参数化的人类形状模型周围实现有效的几何推断。这种方法将动态几何、纹理和材质图建模为二维神经表示,可实现高效的三角化处理。PhyNES能够捕获不同光照下的物理表面属性,从而实现基于物理的实时渲染。实验表明,PhyNES在实现重新照明质量方面与最先进的方法相当的同时,显著提高了渲染速度、内存效率和重建质量。
论文及项目相关链接
Summary
本文介绍了一种基于物理的神经网络显式表面(PhyNES)建模方法,用于从稀疏视角的视频中高效地模拟可重新照明的动态人类角色。该方法采用紧凑的神经网络材质映射,结合神经网络显式表面(NES)表示,通过连接符号距离场到显式表面,实现高效几何推断。此方法将动态几何、纹理和材质映射为二维神经网络表示,实现高效渲染。实验表明,PhyNES在重新照明质量方面达到了最先进的方法水平,同时显著提高了渲染速度、内存效率和重建质量。
Key Takeaways
- PhyNES方法能够高效建模可重新照明的动态人类角色,适用于AR/VR应用。
- 采用紧凑的神经网络材质映射和神经网络显式表面表示。
- 通过连接符号距离场到显式表面,实现高效几何推断。
- 将动态几何、纹理和材质映射为二维神经网络表示,实现高效渲染。
- PhyNES能有效捕捉物理表面属性在不同照明条件下的变化。
- 实现实时物理渲染。
点此查看论文截图
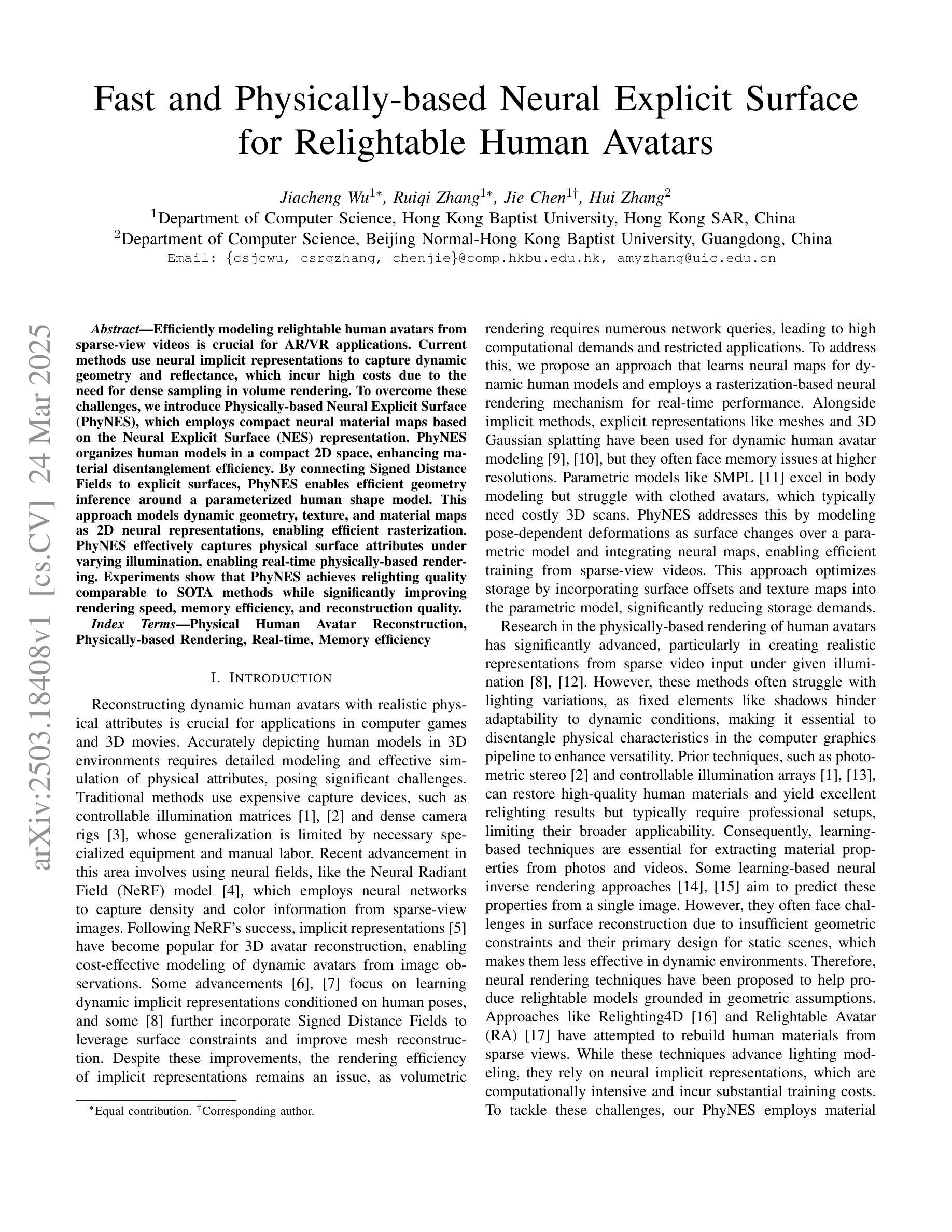
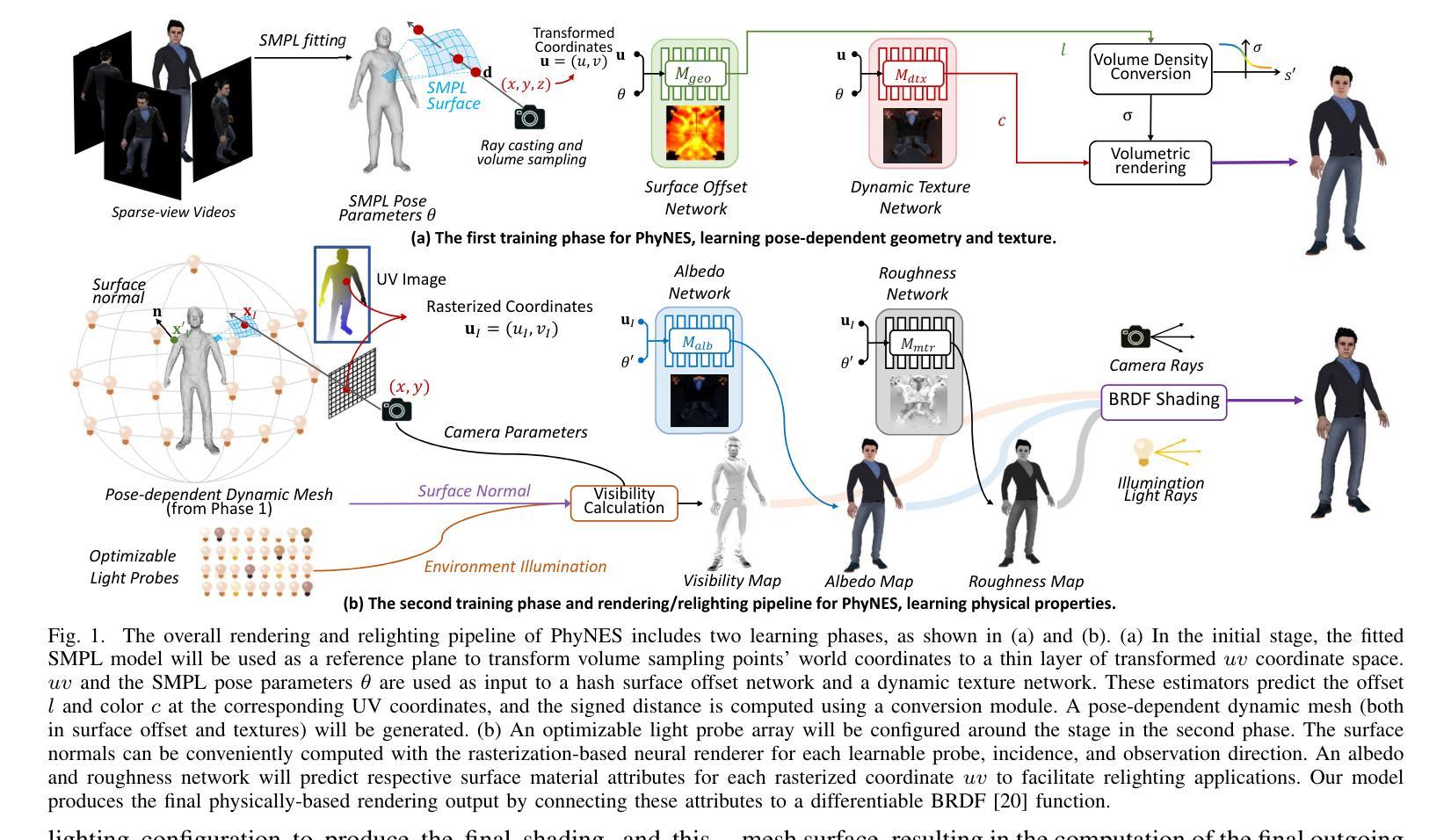
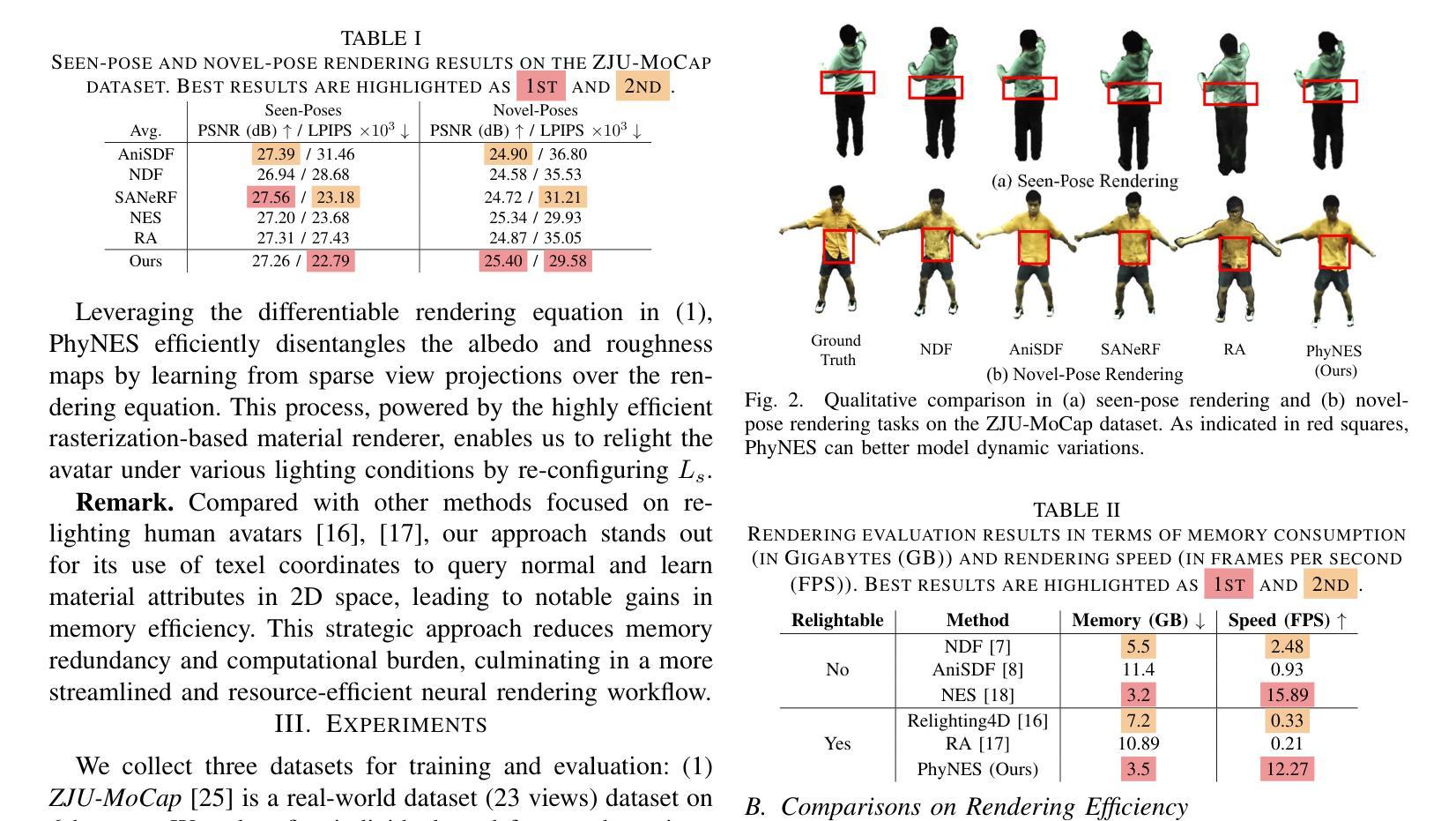
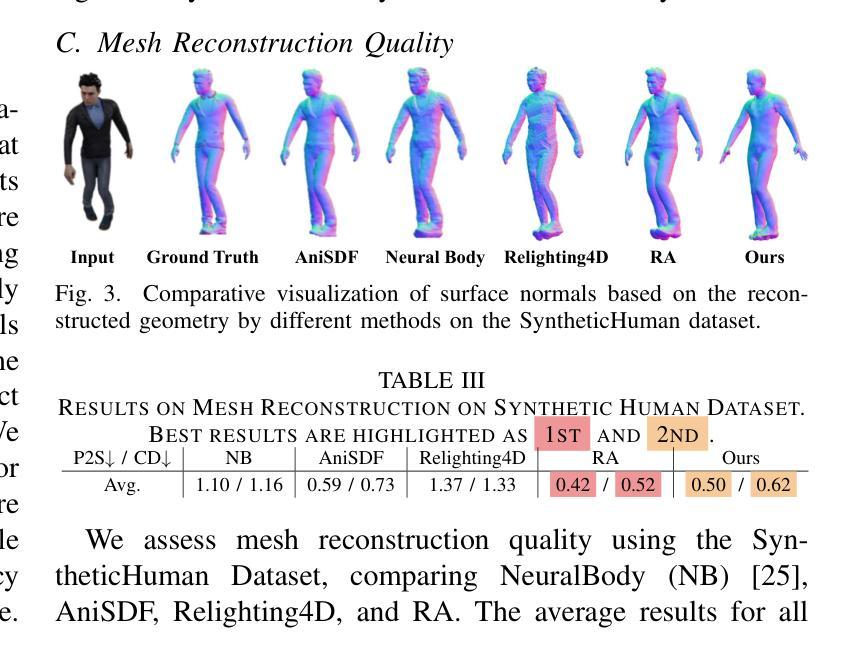
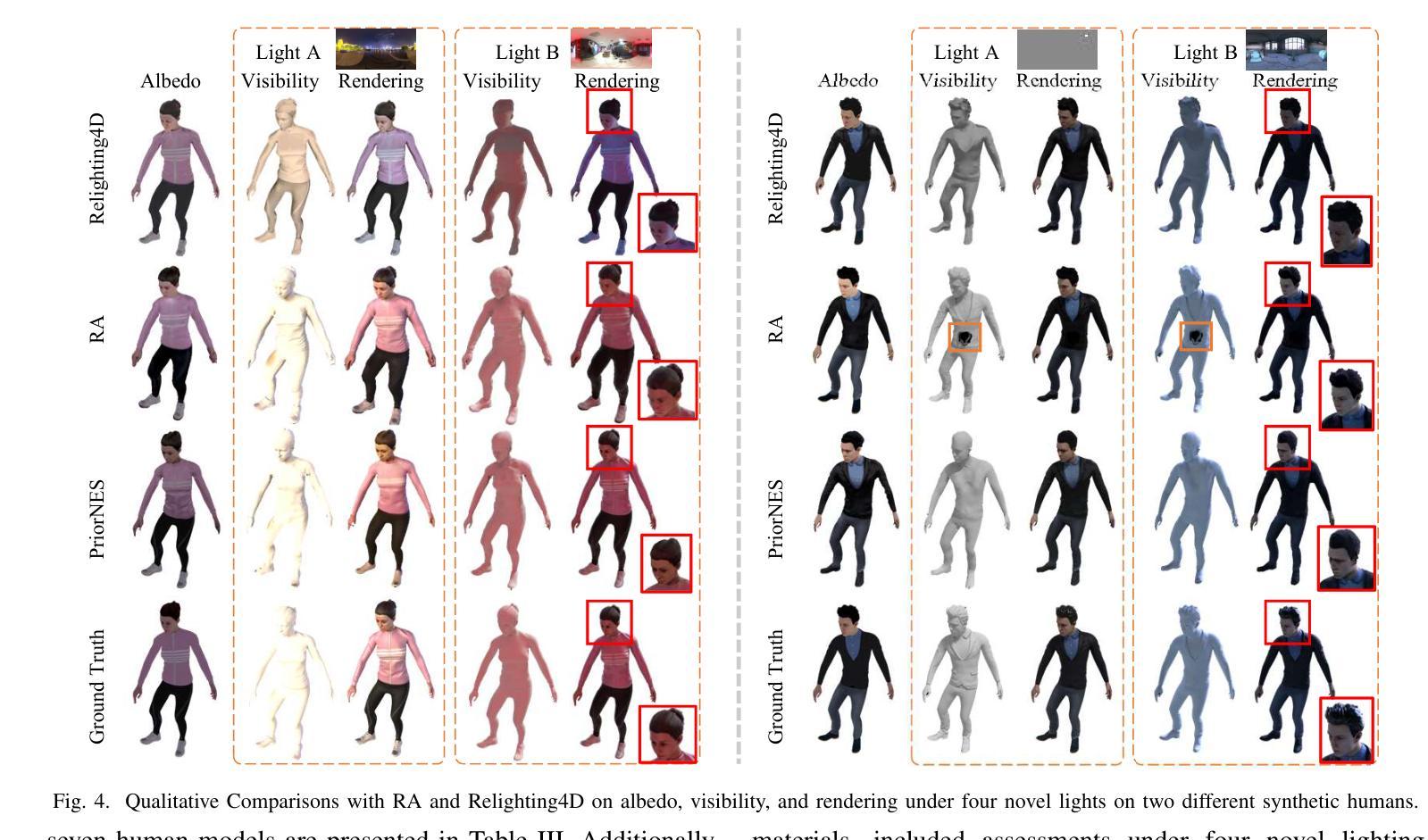
PRIMAL: Physically Reactive and Interactive Motor Model for Avatar Learning
Authors:Yan Zhang, Yao Feng, Alpár Cseke, Nitin Saini, Nathan Bajandas, Nicolas Heron, Michael J. Black
To build a motor system of the interactive avatar, it is essential to develop a generative motion model drives the body to move through 3D space in a perpetual, realistic, controllable, and responsive manner. Although motion generation has been extensively studied, most methods do not support embodied intelligence'' due to their offline setting, slow speed, limited motion lengths, or unnatural movements. To overcome these limitations, we propose PRIMAL, an autoregressive diffusion model that is learned with a two-stage paradigm, inspired by recent advances in foundation models. In the pretraining stage, the model learns motion dynamics from a large number of sub-second motion segments, providing
motor primitives’’ from which more complex motions are built. In the adaptation phase, we employ a ControlNet-like adaptor to fine-tune the motor control for semantic action generation and spatial target reaching. Experiments show that physics effects emerge from our training. Given a single-frame initial state, our model not only generates unbounded, realistic, and controllable motion, but also enables the avatar to be responsive to induced impulses in real time. In addition, we can effectively and efficiently adapt our base model to few-shot personalized actions and the task of spatial control. Evaluations show that our proposed method outperforms state-of-the-art baselines. We leverage the model to create a real-time character animation system in Unreal Engine that is highly responsive and natural. Code, models, and more results are available at: https://yz-cnsdqz.github.io/eigenmotion/PRIMAL
要构建交互式虚拟角色的运动系统,关键在于开发一个生成运动模型,使身体能够以持续、真实、可控和响应迅速的方式在三维空间内移动。虽然运动生成已经得到了广泛的研究,但大多数方法并不支持“嵌入智能”,因为它们是在离线设置下运行的、速度慢、运动长度有限或不自然。为了克服这些局限性,我们提出了PRIMA,这是一种基于自回归扩散模型的,受到最新基础模型启发的两阶段学习范式。在预训练阶段,模型从大量次秒运动片段中学习运动动力学,提供“运动原始数据”,从中构建更复杂的运动。在适应阶段,我们采用类似ControlNet的适配器对运动控制进行微调,以生成语义动作和目标空间到达。实验表明,我们的训练产生了物理效果。给定单帧初始状态,我们的模型不仅生成无界限、真实和可控的运动,还使虚拟角色能够实时响应产生的脉冲。此外,我们可以有效且高效地调整我们的基础模型进行个性化动作和少量空间控制任务。评估表明,我们提出的方法优于最新的基线方法。我们利用该模型在Unreal Engine中创建了一个实时角色动画系统,具有高度响应性和自然性。代码、模型和更多结果可在:https://yz-cnsdqz.github.io/eigenmotion/PRIMA上找到。
论文及项目相关链接
PDF 18 pages
Summary
该文介绍了构建交互式虚拟角色的运动系统的重要性,并指出传统方法存在局限性。为此,提出了基于生成扩散模型的新方法PRIMAL,包含预训练阶段和自适应阶段。该模型能从大量次秒级运动片段中学习运动动力学,生成复杂运动,并能实时响应外部刺激。同时,该模型还能高效适应个性化动作和空间控制任务,优于现有方法。
Key Takeaways
- 交互式虚拟角色的运动系统需要能生成持续、真实、可控并响应外部刺激的动态运动。
- 现有方法存在离线设置、速度慢、运动长度有限或不自然等问题,无法实现“内在智能”。
- 提出新方法PRIMAL,结合预训练阶段和自适应阶段,从大量运动片段中学习运动动力学。
- 预训练阶段提供“运动原语”,构建复杂运动;自适应阶段通过ControlNet-like适配器精细调整运动控制,实现语义动作生成和空间目标达成。
- 模型能生成无界限、真实、可控的运动,并实时响应外部刺激。
- 模型能高效适应个性化动作和空间控制任务。
点此查看论文截图
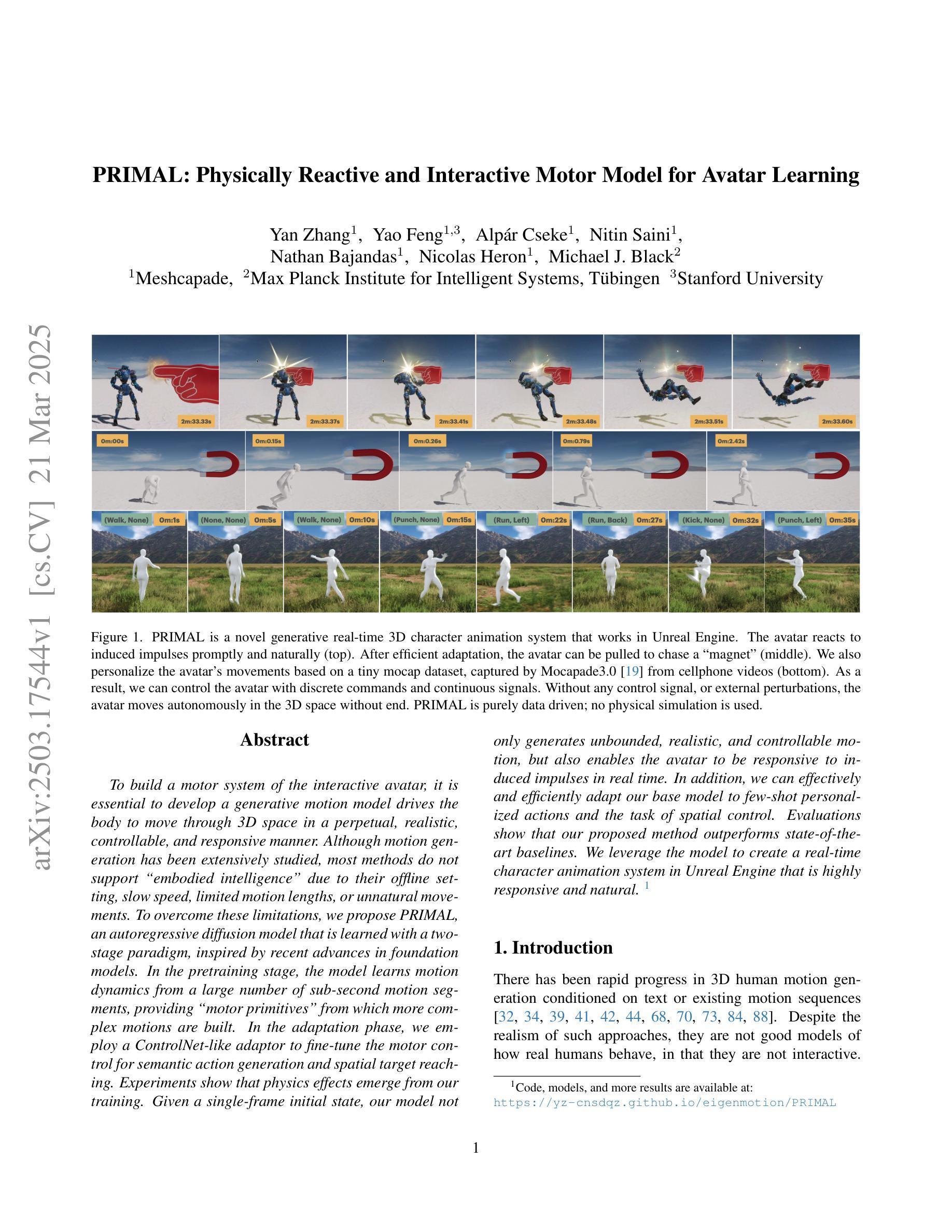
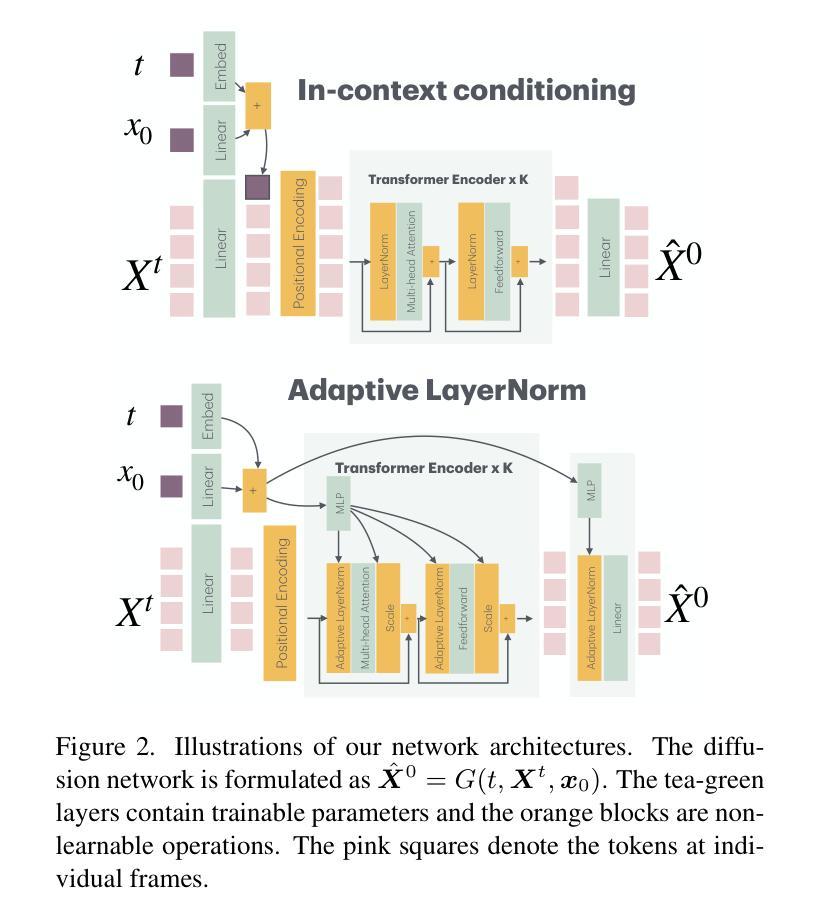
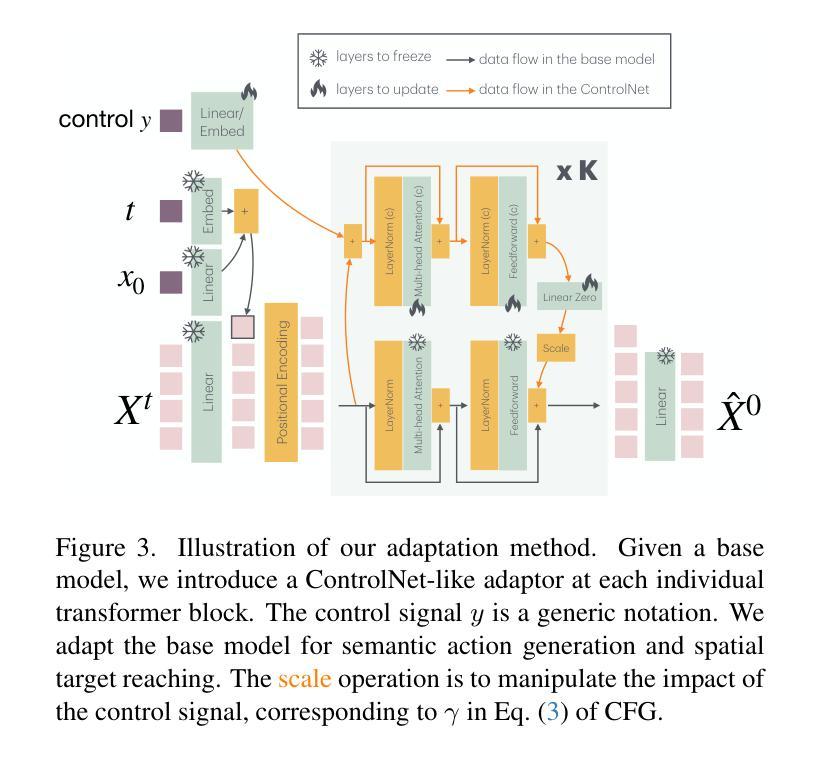
TaoAvatar: Real-Time Lifelike Full-Body Talking Avatars for Augmented Reality via 3D Gaussian Splatting
Authors:Jianchuan Chen, Jingchuan Hu, Gaige Wang, Zhonghua Jiang, Tiansong Zhou, Zhiwen Chen, Chengfei Lv
Realistic 3D full-body talking avatars hold great potential in AR, with applications ranging from e-commerce live streaming to holographic communication. Despite advances in 3D Gaussian Splatting (3DGS) for lifelike avatar creation, existing methods struggle with fine-grained control of facial expressions and body movements in full-body talking tasks. Additionally, they often lack sufficient details and cannot run in real-time on mobile devices. We present TaoAvatar, a high-fidelity, lightweight, 3DGS-based full-body talking avatar driven by various signals. Our approach starts by creating a personalized clothed human parametric template that binds Gaussians to represent appearances. We then pre-train a StyleUnet-based network to handle complex pose-dependent non-rigid deformation, which can capture high-frequency appearance details but is too resource-intensive for mobile devices. To overcome this, we “bake” the non-rigid deformations into a lightweight MLP-based network using a distillation technique and develop blend shapes to compensate for details. Extensive experiments show that TaoAvatar achieves state-of-the-art rendering quality while running in real-time across various devices, maintaining 90 FPS on high-definition stereo devices such as the Apple Vision Pro.
真实3D全身对话虚拟人在增强现实领域具有巨大潜力,应用范围从电子商务直播到全息通信。尽管在用于创建逼真虚拟人的3D高斯拼贴(3DGS)技术方面取得了进展,但现有方法在全身对话任务中的面部表情和身体动作的精细控制方面仍存在困难。此外,它们通常缺乏足够的细节,无法在移动设备上实时运行。我们推出了TaoAvatar,一种高保真、轻量级的3DGS全身对话虚拟人,由各种信号驱动。我们的方法首先创建一个个性化的穿衣人类参数模板,将高斯绑定以表示外观。然后,我们预训练一个基于StyleUnet的网络来处理复杂的姿势相关的非刚性变形,该网络能够捕获高频外观细节,但对于移动设备来说资源过于密集。为了克服这一点,我们使用蒸馏技术将非刚性变形“烘焙”到基于MLP的轻量级网络中,并开发混合形状来补偿细节。大量实验表明,TaoAvatar在保持实时运行的同时实现了最先进的渲染质量,在各种设备上都能保持90帧的帧率,如在苹果视界专业等高分辨率立体声设备上。
论文及项目相关链接
PDF Accepted by CVPR 2025, project page: https://PixelAI-Team.github.io/TaoAvatar
Summary:
本文介绍了基于实时动态信号的逼真的全身交互式化身TaoAvatar的研发与应用。它采用了创新的基于3D高斯图像平铺技术来创建个性化模板,并采用预训练的StyleUnet网络处理复杂姿态的非刚性变形。同时,利用蒸馏技术优化模型以在移动设备上实现实时运行和高帧率输出。
Key Takeaways:
- TaoAvatar是基于增强现实技术的全身交互式化身系统,可用于电商直播和全息通信等多种应用。
- TaoAvatar利用个性化的人类参数化模板创建化身的外观。这种模型采用了先进的实时信号驱动技术,支持高度真实的动态表达和运动控制。
- 采用StyleUnet网络处理复杂的姿态依赖的非刚性变形,能够捕捉高频率的外观细节。然而,该网络在移动设备上的资源消耗较大。
- 为了解决这一问题,采用了蒸馏技术来“烘焙”非刚性变形数据,并利用神经网络推理轻量化方法使其在移动设备上进行实时运行成为可能。此外,还开发了补偿细节的混合形状技术。
点此查看论文截图
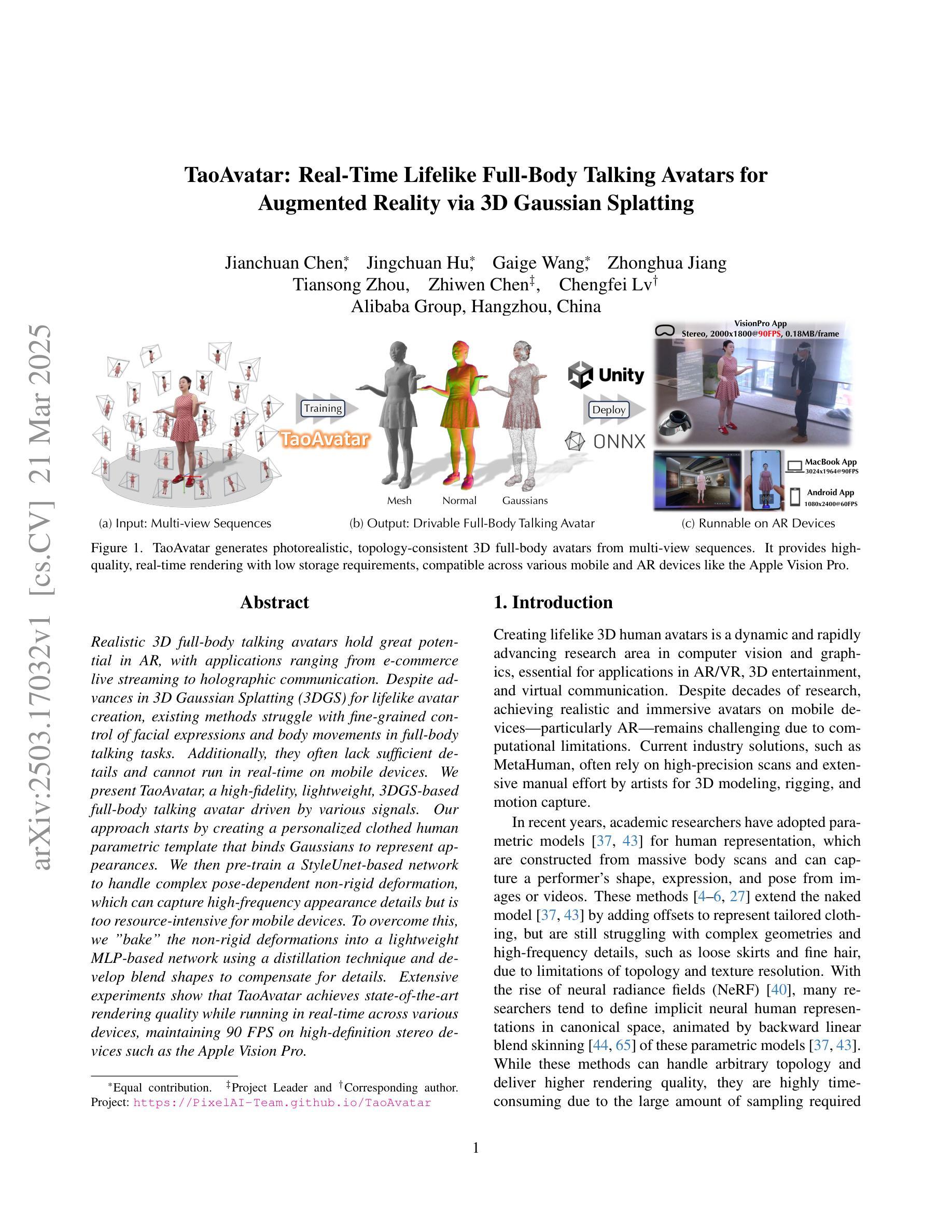
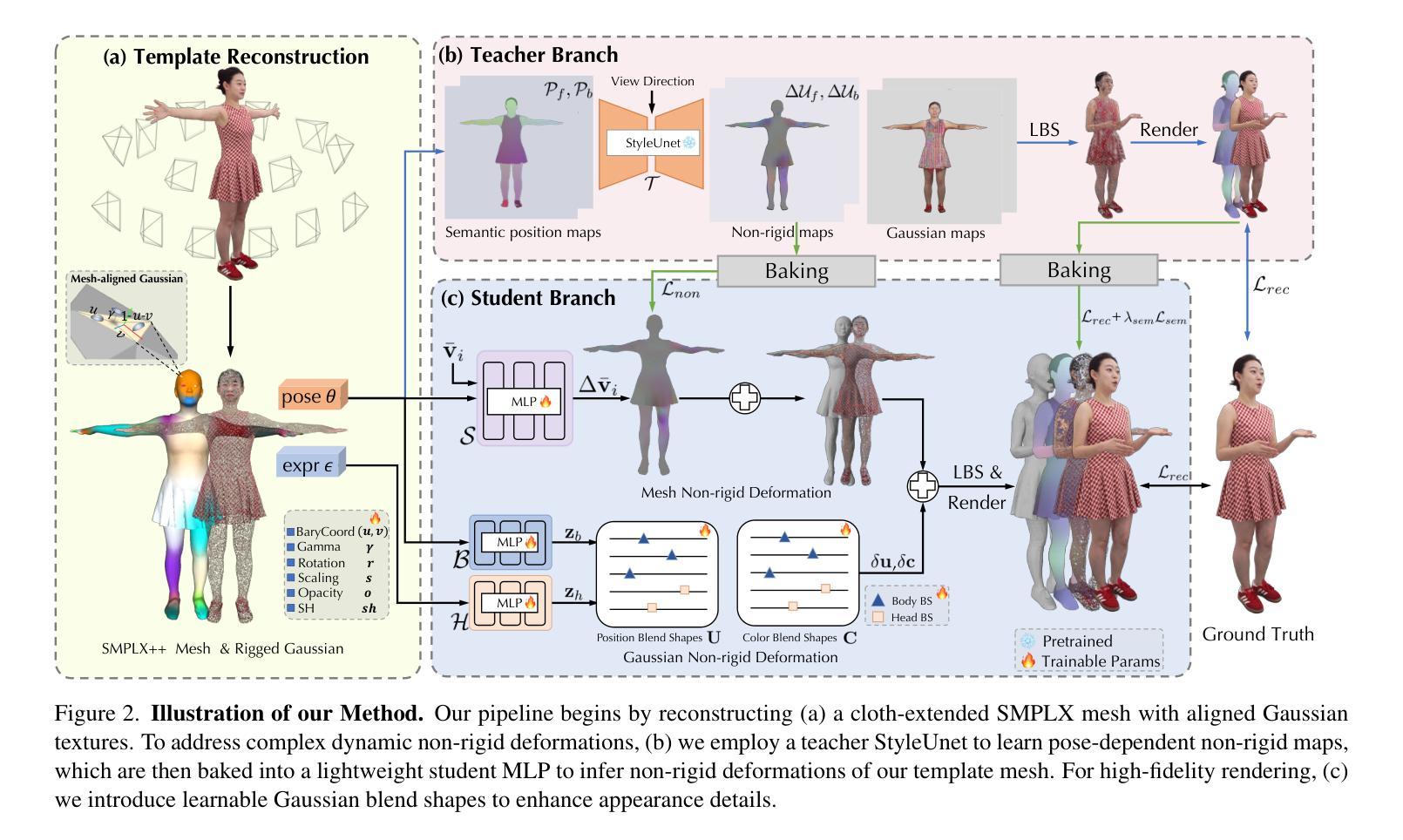
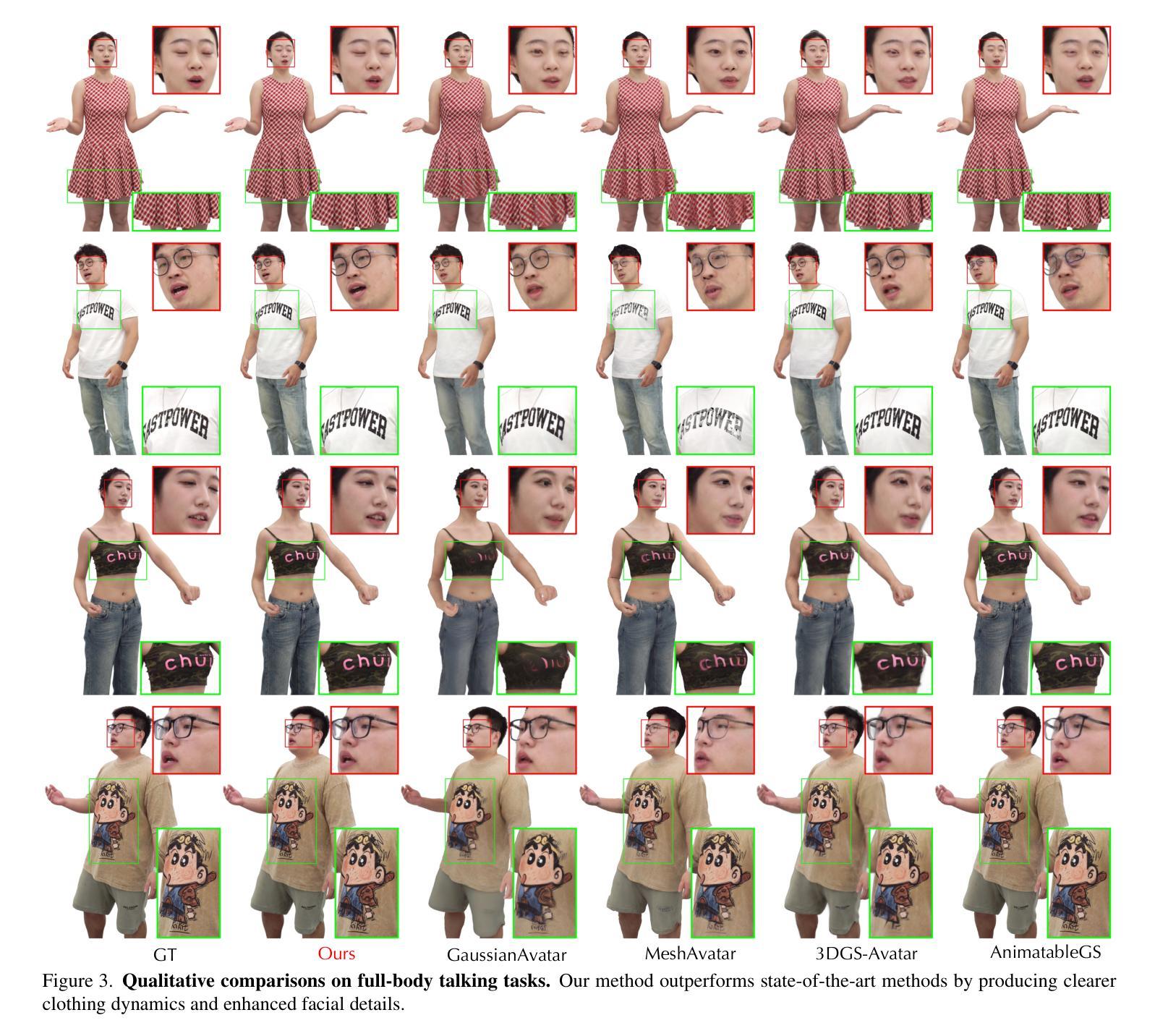
Zero-1-to-A: Zero-Shot One Image to Animatable Head Avatars Using Video Diffusion
Authors:Zhenglin Zhou, Fan Ma, Hehe Fan, Tat-Seng Chua
Animatable head avatar generation typically requires extensive data for training. To reduce the data requirements, a natural solution is to leverage existing data-free static avatar generation methods, such as pre-trained diffusion models with score distillation sampling (SDS), which align avatars with pseudo ground-truth outputs from the diffusion model. However, directly distilling 4D avatars from video diffusion often leads to over-smooth results due to spatial and temporal inconsistencies in the generated video. To address this issue, we propose Zero-1-to-A, a robust method that synthesizes a spatial and temporal consistency dataset for 4D avatar reconstruction using the video diffusion model. Specifically, Zero-1-to-A iteratively constructs video datasets and optimizes animatable avatars in a progressive manner, ensuring that avatar quality increases smoothly and consistently throughout the learning process. This progressive learning involves two stages: (1) Spatial Consistency Learning fixes expressions and learns from front-to-side views, and (2) Temporal Consistency Learning fixes views and learns from relaxed to exaggerated expressions, generating 4D avatars in a simple-to-complex manner. Extensive experiments demonstrate that Zero-1-to-A improves fidelity, animation quality, and rendering speed compared to existing diffusion-based methods, providing a solution for lifelike avatar creation. Code is publicly available at: https://github.com/ZhenglinZhou/Zero-1-to-A.
动画头像生成通常需要大量的数据进行训练。为了减少数据需求,一种自然的解决方案是利用无数据的静态头像生成方法,例如使用带有得分蒸馏采样(SDS)的预训练扩散模型,将头像与扩散模型的伪地面真实输出对齐。然而,直接从视频扩散中提取4D头像往往会导致结果过于平滑,这是由于生成视频的空间和时间不一致性所致。为了解决这个问题,我们提出了Zero-1-to-A方法,这是一种使用视频扩散模型为4D头像重建合成空间和时间一致性数据集的有效方法。具体来说,Zero-1-to-A通过迭代构建视频数据集和以渐进的方式优化动画头像,确保在学习过程中头像质量平稳且一致地提高。这种渐进学习涉及两个阶段:(1)空间一致性学习修正表情并从正面到侧面进行学习;(2)时间一致性学习修正视图,并从轻松到夸张的表情进行学习,以简单到复杂的方式生成4D头像。大量实验表明,与现有的扩散方法相比,Zero-1-to-A提高了保真度、动画质量和渲染速度,为逼真头像的创作提供了解决方案。代码公开可用:https://github.com/ZhenglinZhou/Zero-1-to-A。
论文及项目相关链接
PDF Accepted by CVPR 2025, project page: https://zhenglinzhou.github.io/Zero-1-to-A/
Summary
动画头像生成通常需要大量数据进行训练。为减少数据需求,可利用无需数据的静态头像生成方法,如预训练的扩散模型与评分蒸馏采样(SDS)。然而,直接从视频扩散中蒸馏四维头像往往会导致结果过于平滑,因为生成视频的空间和时间不一致性。为解决这一问题,我们提出Zero-1-to-A方法,利用视频扩散模型合成一个具有空间和时间一致性的数据集,用于四维头像重建。该方法通过两个阶段的渐进学习,确保头像质量在学习过程中平稳提高。首先是空间一致性学习,修复表情并从正面到侧面进行学习;然后是时间一致性学习,修复视角并从轻松到夸张的表情进行学习,以简单到复杂的方式生成四维头像。实验表明,Zero-1-to-A提高了保真度、动画质量和渲染速度,为创建逼真的头像提供了解决方案。
Key Takeaways
- 动画头像生成需要大数据训练,为降低数据需求,可利用预训练的扩散模型与评分蒸馏采样方法。
- 直接从视频扩散中蒸馏四维头像会导致结果过于平滑,因为生成视频的空间和时间不一致性。
- 提出Zero-1-to-A方法,通过合成具有空间和时间一致性的数据集来解决这一问题。
- Zero-1-to-A方法包含两个阶段的渐进学习:空间一致性学习和时间一致性学习。
- 空间一致性学习修复表情并从多个视角进行学习。
- 时间一致性学习修复视角并从轻松到夸张的表情进行学习。
点此查看论文截图
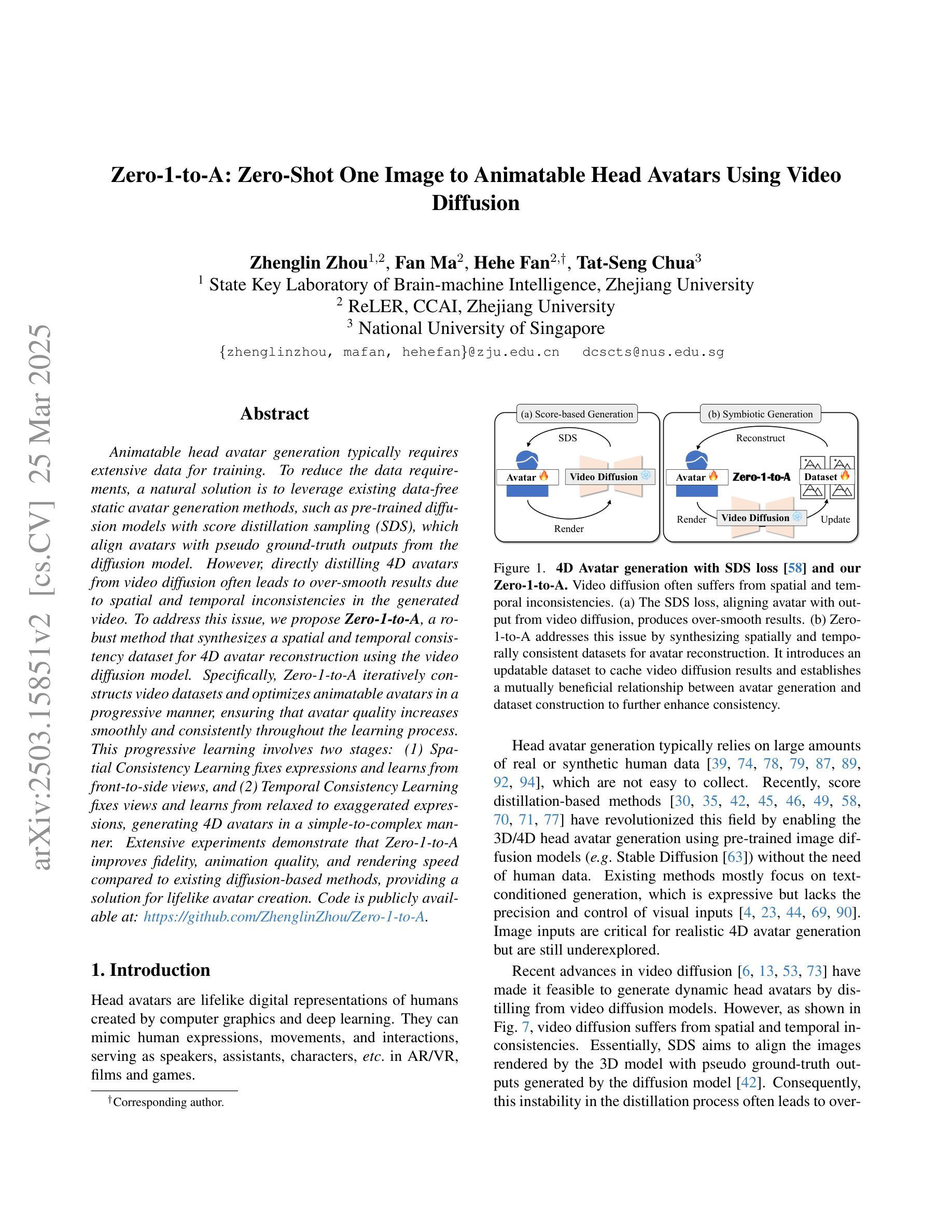
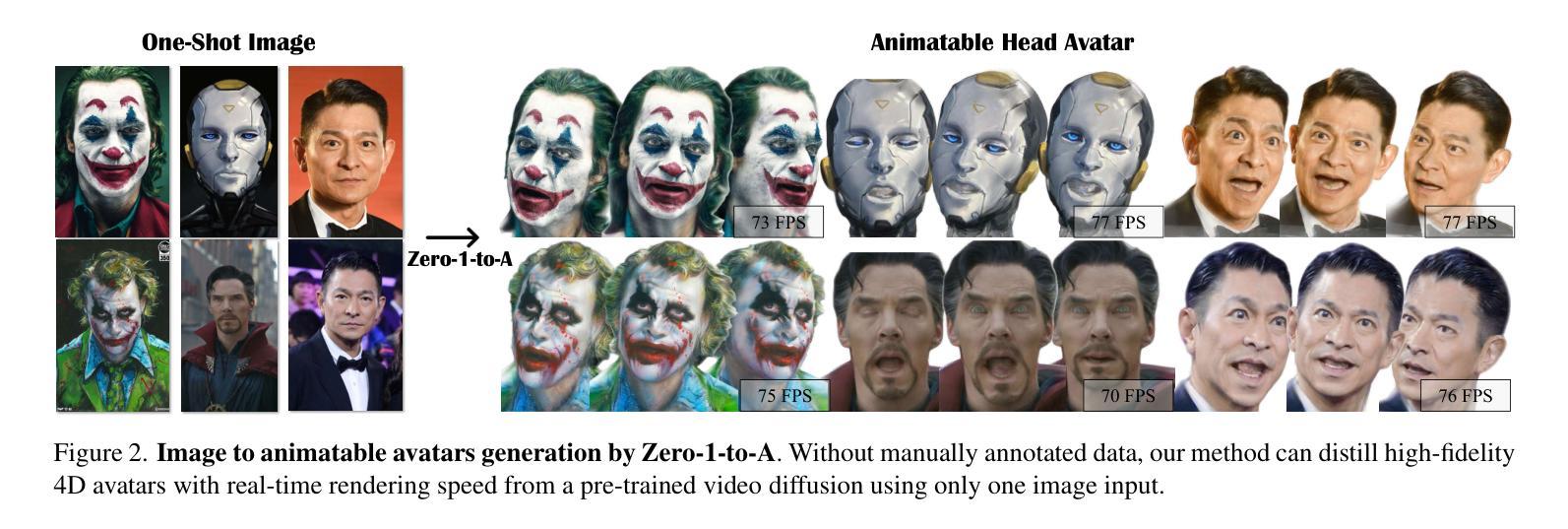
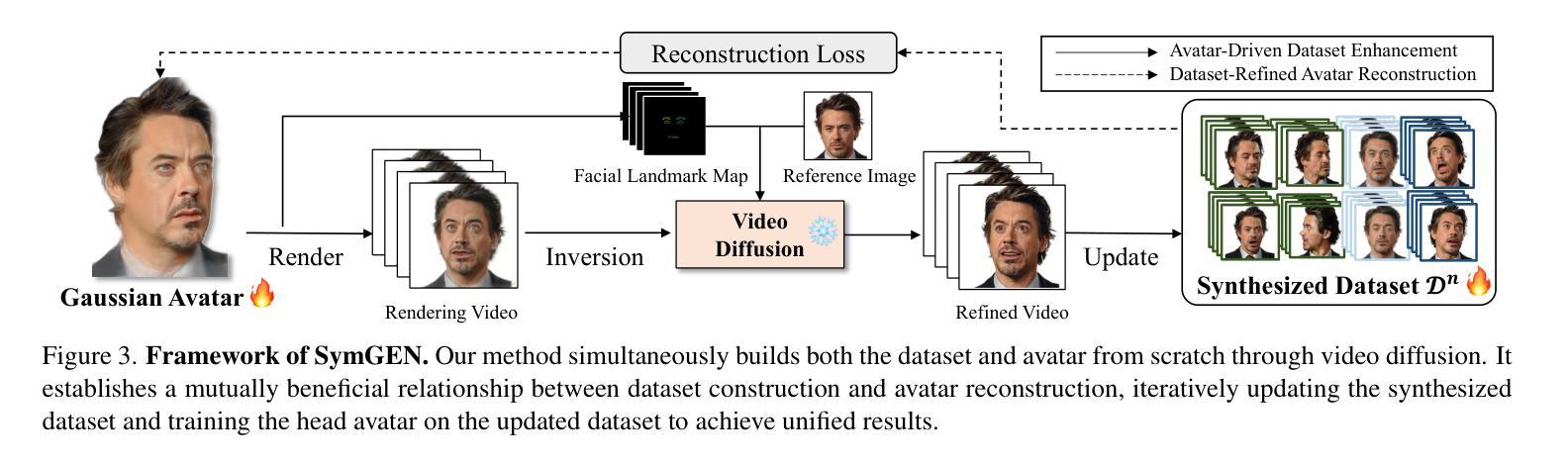
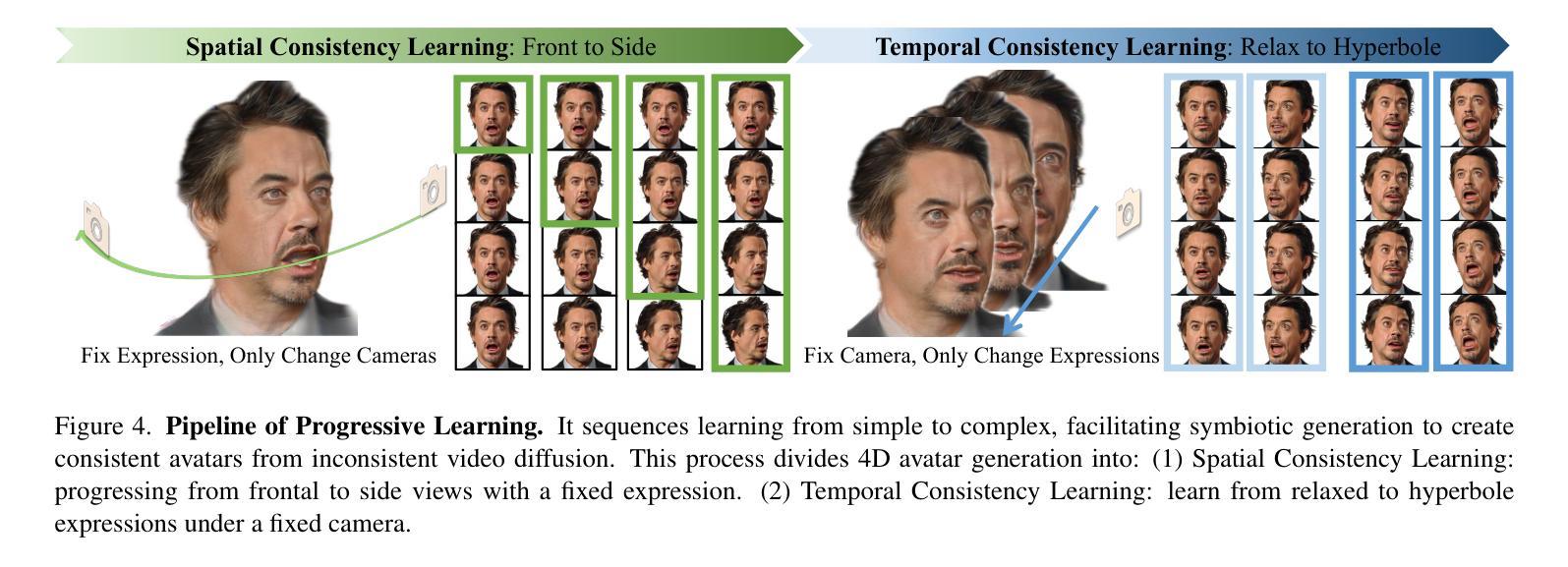
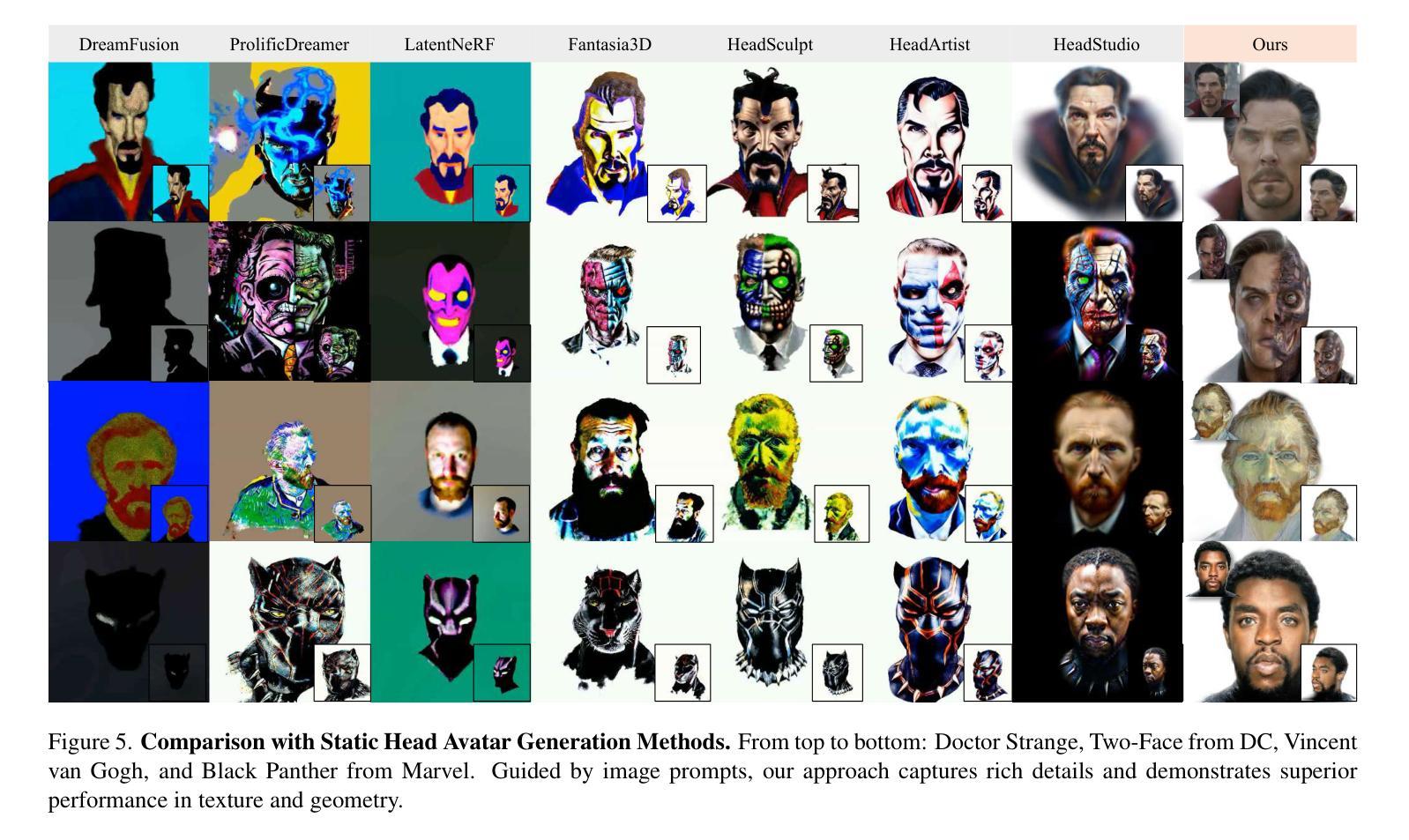
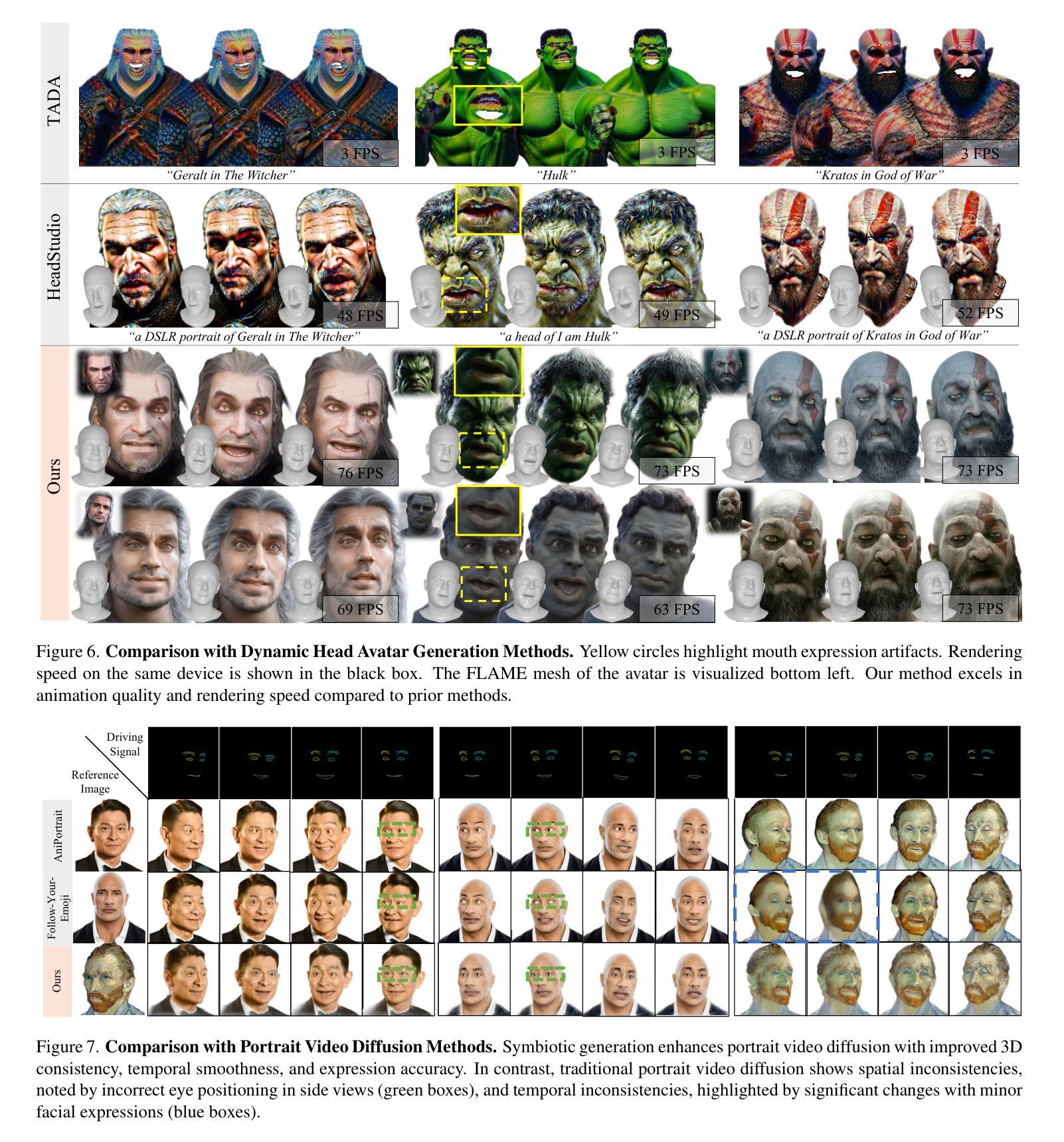
FATE: Full-head Gaussian Avatar with Textural Editing from Monocular Video
Authors:Jiawei Zhang, Zijian Wu, Zhiyang Liang, Yicheng Gong, Dongfang Hu, Yao Yao, Xun Cao, Hao Zhu
Reconstructing high-fidelity, animatable 3D head avatars from effortlessly captured monocular videos is a pivotal yet formidable challenge. Although significant progress has been made in rendering performance and manipulation capabilities, notable challenges remain, including incomplete reconstruction and inefficient Gaussian representation. To address these challenges, we introduce FATE, a novel method for reconstructing an editable full-head avatar from a single monocular video. FATE integrates a sampling-based densification strategy to ensure optimal positional distribution of points, improving rendering efficiency. A neural baking technique is introduced to convert discrete Gaussian representations into continuous attribute maps, facilitating intuitive appearance editing. Furthermore, we propose a universal completion framework to recover non-frontal appearance, culminating in a 360$^\circ$-renderable 3D head avatar. FATE outperforms previous approaches in both qualitative and quantitative evaluations, achieving state-of-the-art performance. To the best of our knowledge, FATE is the first animatable and 360$^\circ$ full-head monocular reconstruction method for a 3D head avatar.
从轻松捕获的单目视频中重建高保真、可动画的3D头像是一项至关重要但艰巨的挑战。虽然在渲染性能和操作功能上取得了显著进展,但仍存在显著挑战,包括重建不完整和高斯表示效率低下。为了解决这些挑战,我们引入了FATE,这是一种从单目视频重建可编辑的全头像的新方法。FATE采用基于采样的密集化策略,确保点的最优位置分布,提高渲染效率。引入了一种神经烘焙技术,将离散高斯表示转换为连续属性图,便于直观的外貌编辑。此外,我们提出了一个通用的完成框架,以恢复非正面的外观,最终生成一个可旋转360度的3D头像。FATE在定性和定量评估中都优于以前的方法,达到了最先进的性能。据我们所知,FATE是第一个可用于3D头像的可动画和360度全头单目重建方法。
论文及项目相关链接
PDF Accepted by CVPR2025
Summary
新一代三维头像重建技术突破:单目视频捕捉,全头可编辑。采用采样加密策略优化点分布,提升渲染效率;引入神经网络烘焙技术,将离散高斯表示转为连续属性图,便于直观编辑外观;提出通用补全框架,实现非正面视角的渲染,生成360°可渲染的三维头像。FATE技术超越现有方法,达到领先水平。
Key Takeaways
- FATE技术能够从单目视频中重建出可编辑的全头三维头像。
- 采用采样加密策略优化点的位置分布,提升渲染效率。
- 引入神经网络烘焙技术,将离散的高斯表示转换为连续属性图。
- 提出通用补全框架,用于恢复非正面外观。
- 生成的三维头像可以实现360°渲染。
- FATE技术在定性和定量评估中都表现出卓越性能。
点此查看论文截图
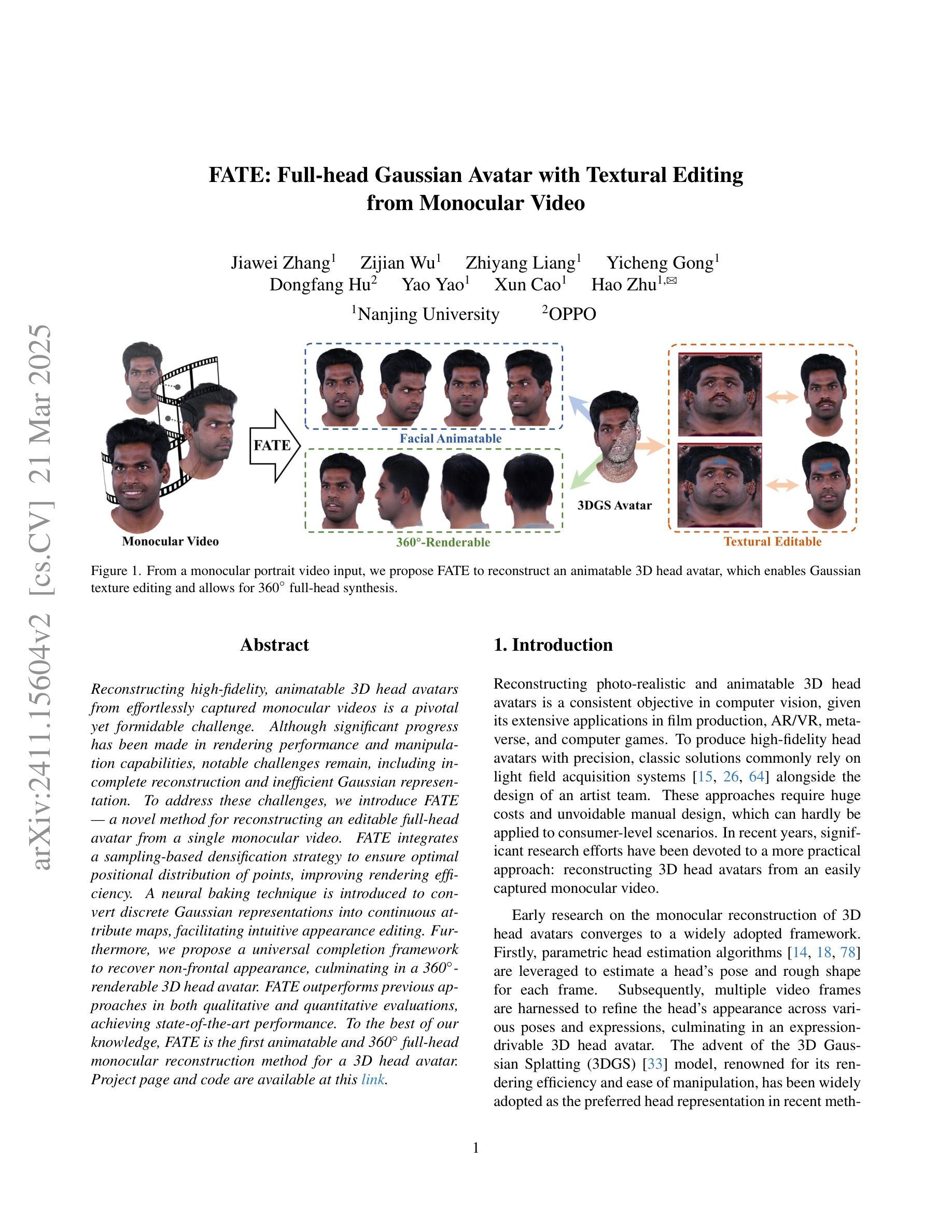
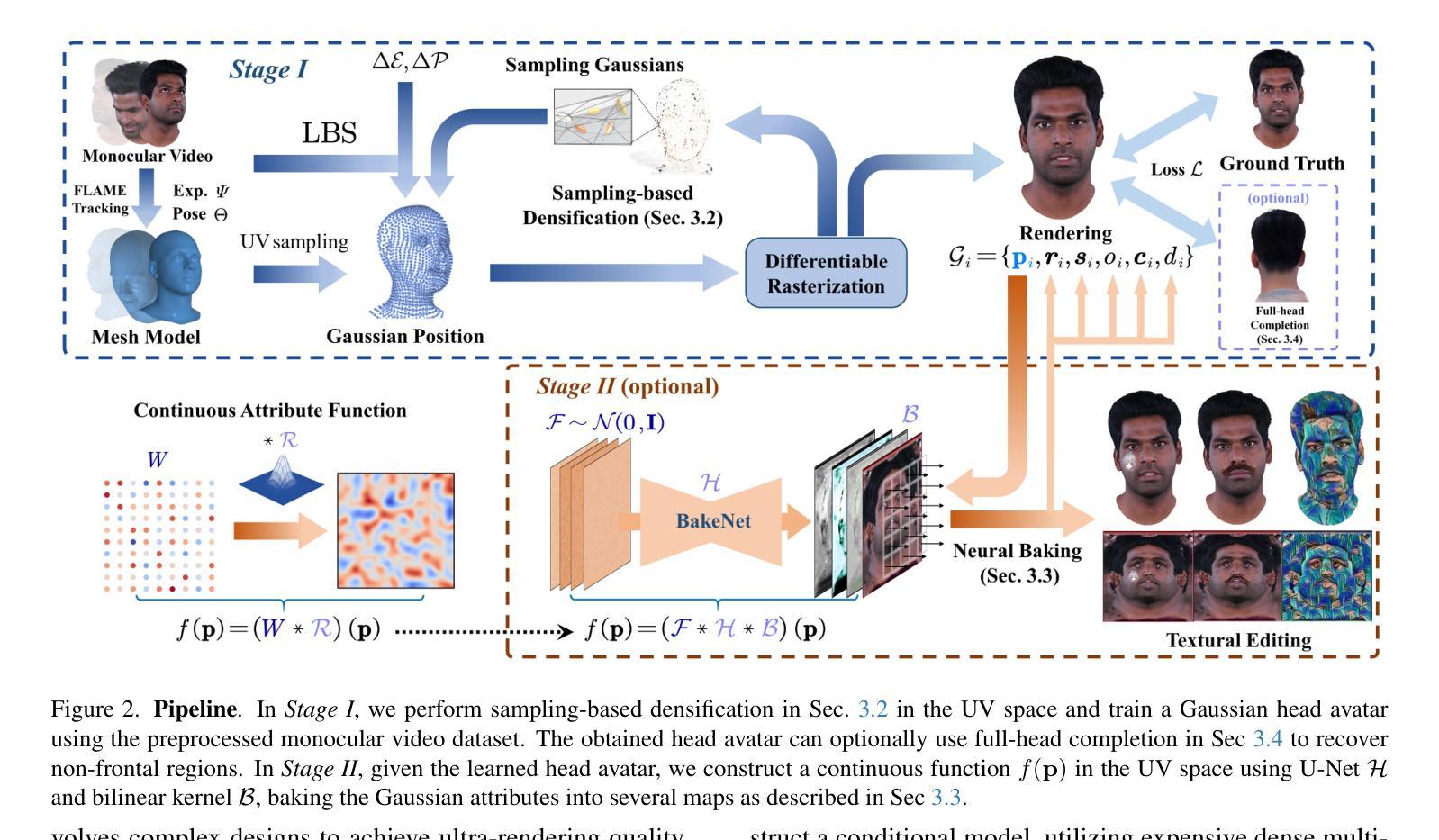
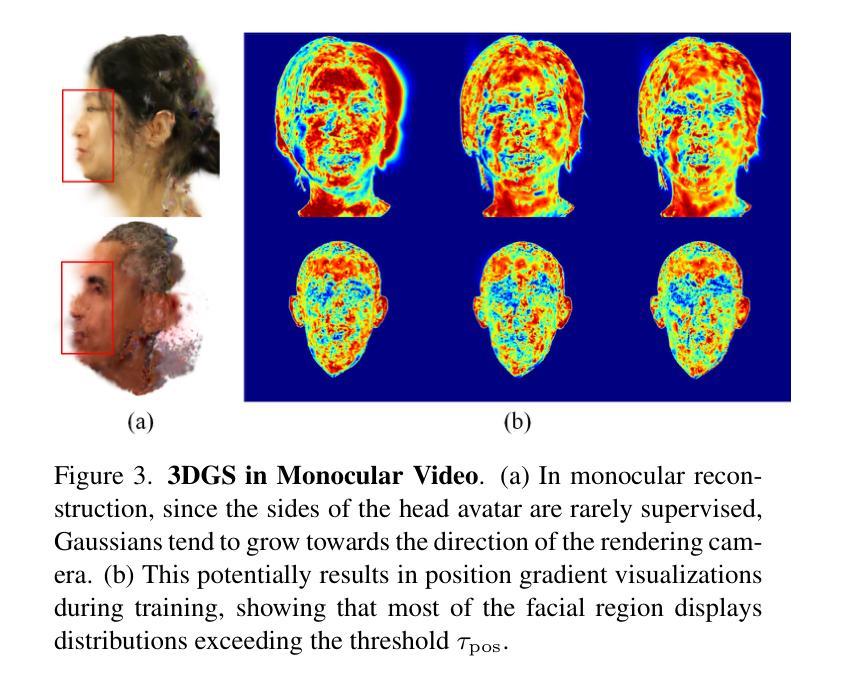
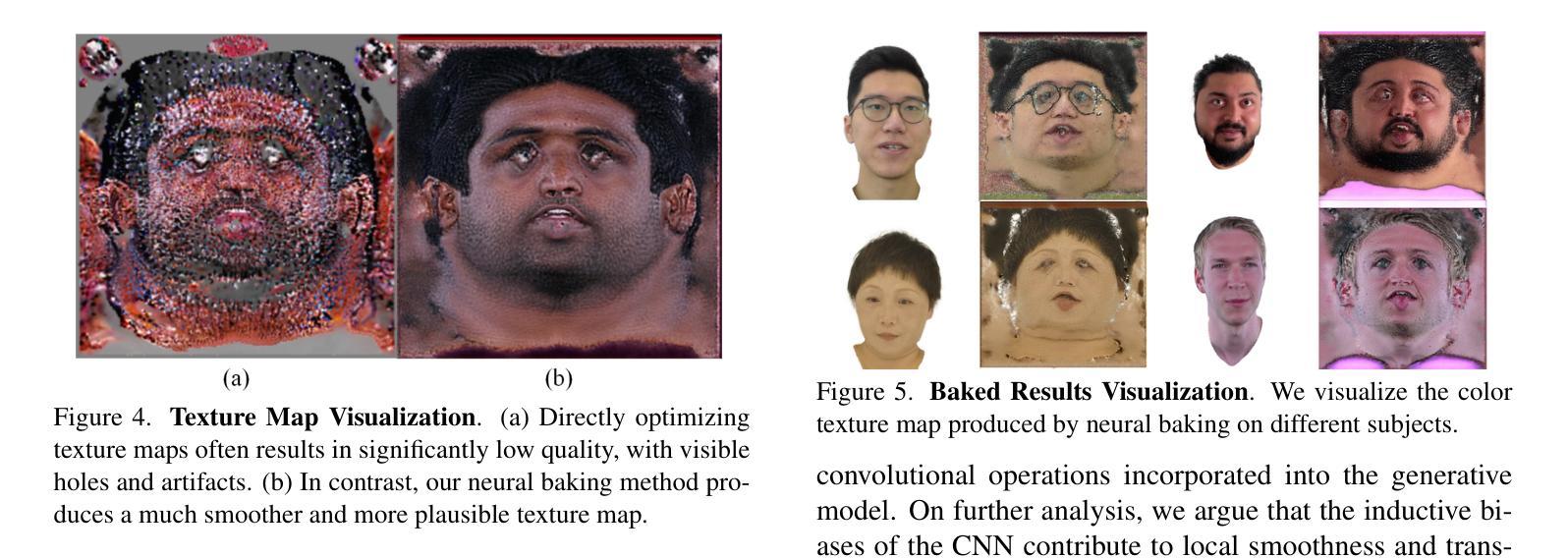
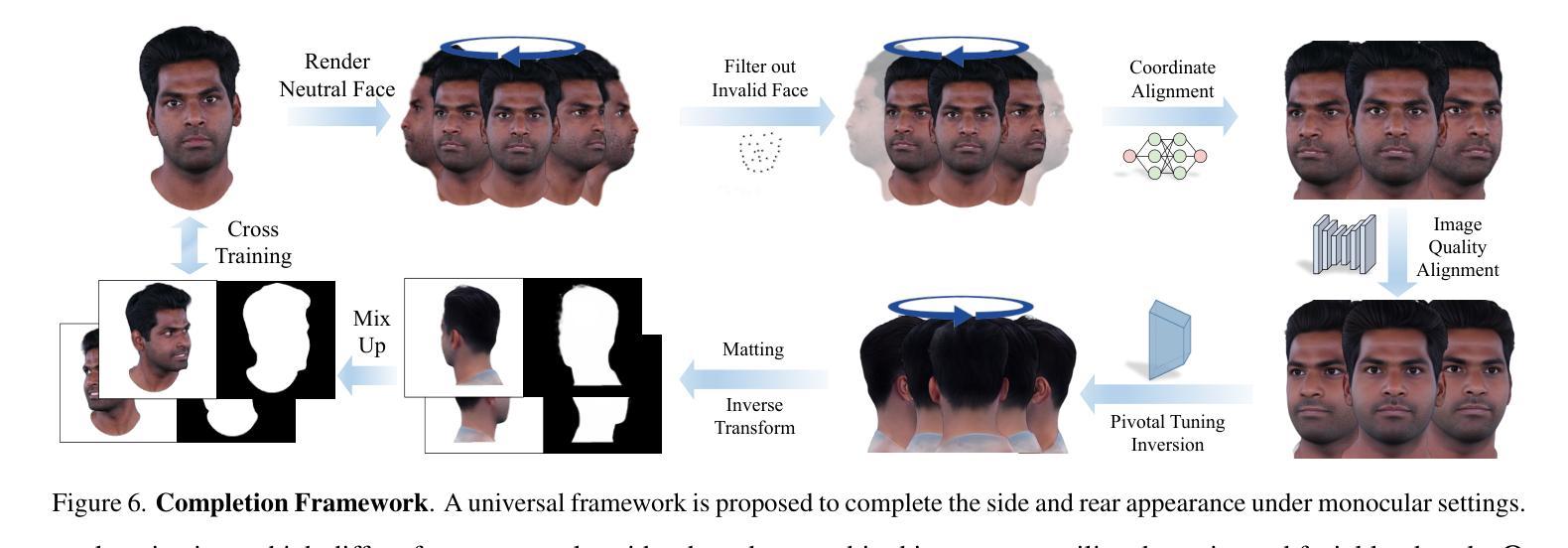