⚠️ 以下所有内容总结都来自于 大语言模型的能力,如有错误,仅供参考,谨慎使用
🔴 请注意:千万不要用于严肃的学术场景,只能用于论文阅读前的初筛!
💗 如果您觉得我们的项目对您有帮助 ChatPaperFree ,还请您给我们一些鼓励!⭐️ HuggingFace免费体验
2025-03-27 更新
Exploring Hallucination of Large Multimodal Models in Video Understanding: Benchmark, Analysis and Mitigation
Authors:Hongcheng Gao, Jiashu Qu, Jingyi Tang, Baolong Bi, Yue Liu, Hongyu Chen, Li Liang, Li Su, Qingming Huang
The hallucination of large multimodal models (LMMs), providing responses that appear correct but are actually incorrect, limits their reliability and applicability. This paper aims to study the hallucination problem of LMMs in video modality, which is dynamic and more challenging compared to static modalities like images and text. From this motivation, we first present a comprehensive benchmark termed HAVEN for evaluating hallucinations of LMMs in video understanding tasks. It is built upon three dimensions, i.e., hallucination causes, hallucination aspects, and question formats, resulting in 6K questions. Then, we quantitatively study 7 influential factors on hallucinations, e.g., duration time of videos, model sizes, and model reasoning, via experiments of 16 LMMs on the presented benchmark. In addition, inspired by recent thinking models like OpenAI o1, we propose a video-thinking model to mitigate the hallucinations of LMMs via supervised reasoning fine-tuning (SRFT) and direct preference optimization (TDPO)– where SRFT enhances reasoning capabilities while TDPO reduces hallucinations in the thinking process. Extensive experiments and analyses demonstrate the effectiveness. Remarkably, it improves the baseline by 7.65% in accuracy on hallucination evaluation and reduces the bias score by 4.5%. The code and data are public at https://github.com/Hongcheng-Gao/HAVEN.
大型多模态模型(LMM)的幻觉问题,即提供看似正确但实际错误的回应,限制了其可靠性和适用性。本文针对视频模态的LMM幻觉问题进行研究,与图像和文本等静态模态相比,视频模态更加动态且更具挑战性。基于这一动机,我们首先提出了一个名为HAVEN的综合基准测试,用于评估视频理解任务中LMM的幻觉。它建立在幻觉原因、幻觉方面和问题格式三个维度上,形成了6000个问题。然后,我们通过在该基准测试上对16个LMM进行实验,定量研究了7个影响幻觉的因素,例如视频的持续时间、模型大小和模型推理等。此外,受到最近思考模型(如OpenAI o1)的启发,我们提出了一种视频思考模型,通过监督推理微调(SRFT)和直接偏好优化(TDPO)来缓解LMM的幻觉问题——其中SRFT增强推理能力,而TDPO减少思考过程中的幻觉。大量实验和分析证明了其有效性。值得注意的是,它在幻觉评估方面的准确率提高了7.65%,偏见分数降低了4.5%。代码和数据在https://github.com/Hongcheng-Gao/HAVEN公开。
论文及项目相关链接
Summary
本文研究了大型多模态模型(LMMs)在视频理解任务中的幻觉问题。为评估LMMs在视频模态的幻觉,建立了一个综合基准测试HAVEN。通过实验研究,定量分析了7个影响幻觉的因素,并提出了一种视频思考模型,通过监督推理微调(SRFT)和直接偏好优化(TDPO)来缓解LMMs的幻觉问题。这提高了基准测试评估幻觉的准确性,并降低了偏见分数。
Key Takeaways
- 大型多模态模型(LMMs)在视频理解任务中存在幻觉问题,影响其可靠性和适用性。
- 建立了HAVEN基准测试,用于评估LMMs在视频模态的幻觉表现。
- HAVEN基准测试包含三个维度:幻觉原因、幻觉方面和问题格式。
- 通过实验定量研究了7个影响幻觉的因素,包括视频持续时间、模型大小和模型推理等。
- 提出了一种视频思考模型,通过监督推理微调(SRFT)和直接偏好优化(TDPO)来缓解LMMs的幻觉问题。
- 视频思考模型在幻觉评估方面提高了基线准确性7.65%,并降低了偏见分数4.5%。
点此查看论文截图
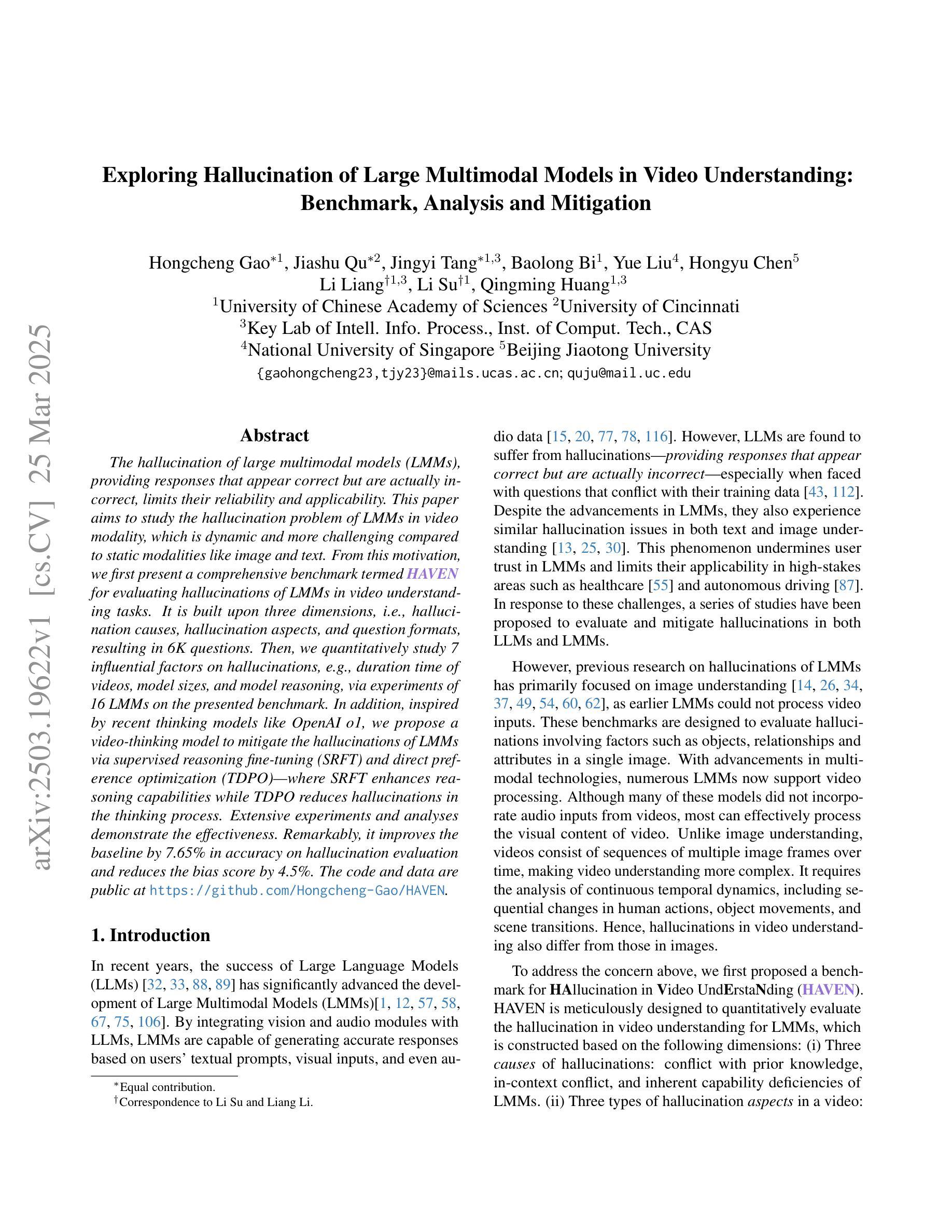
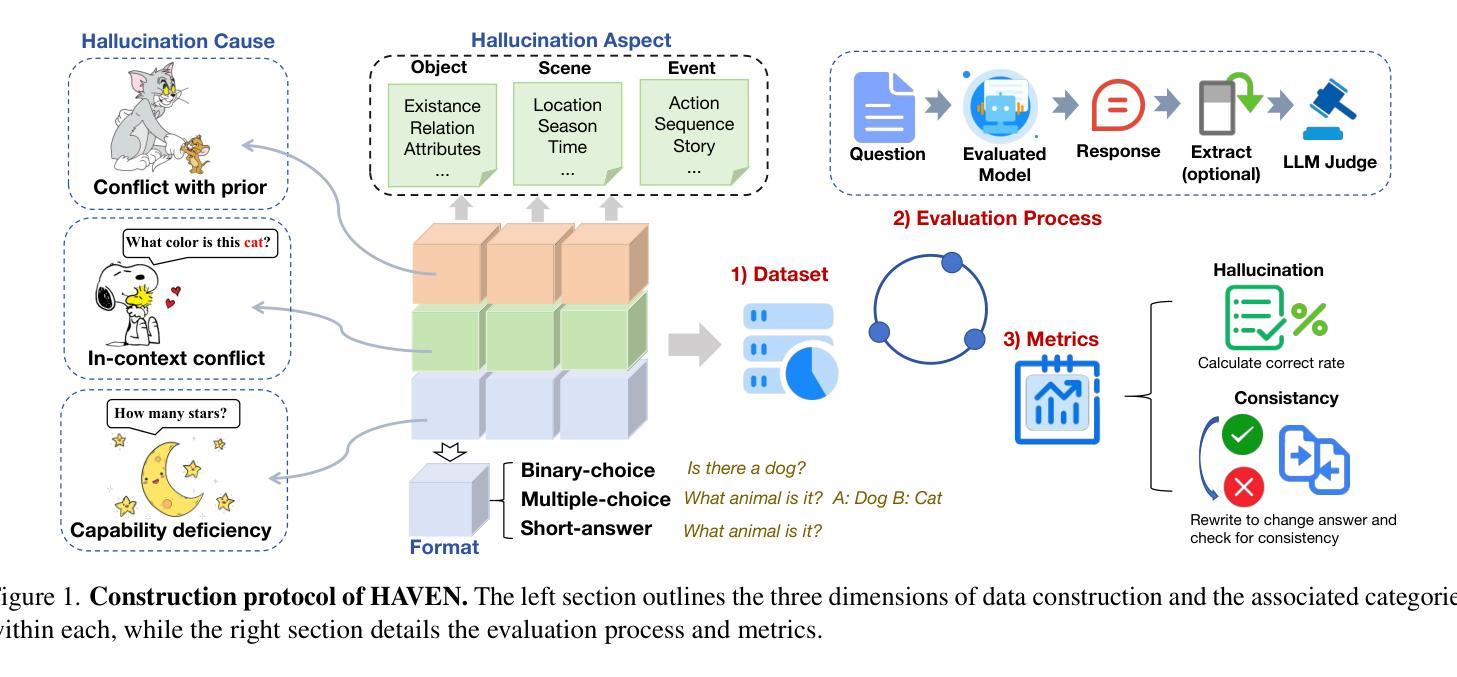
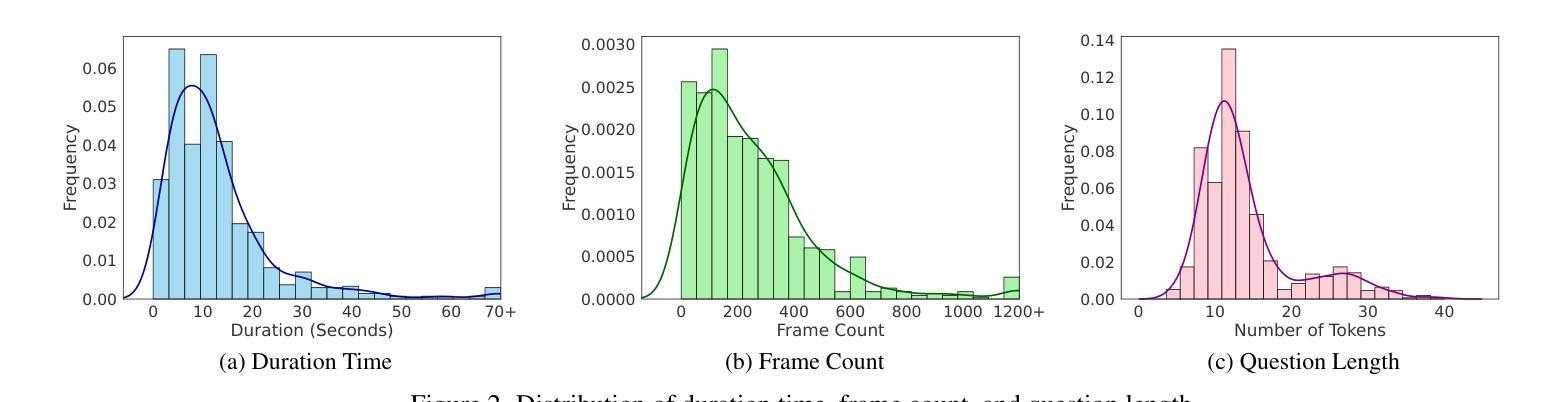
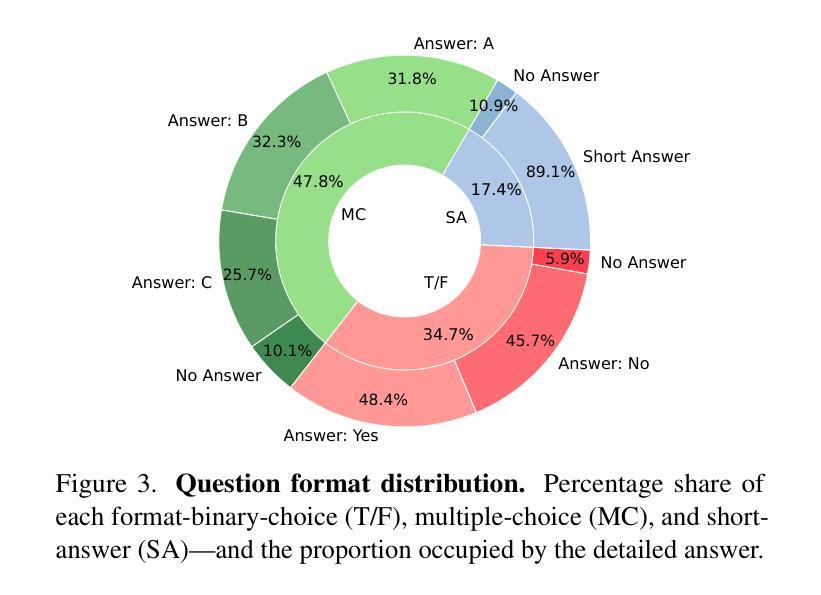
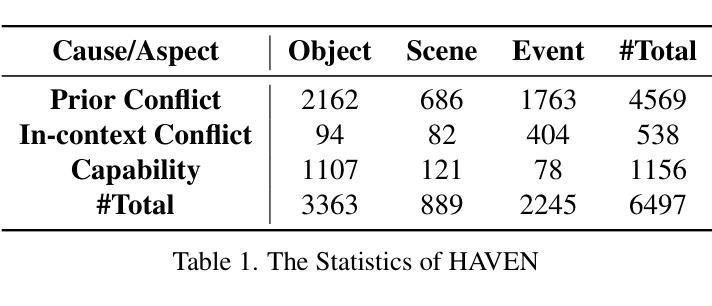
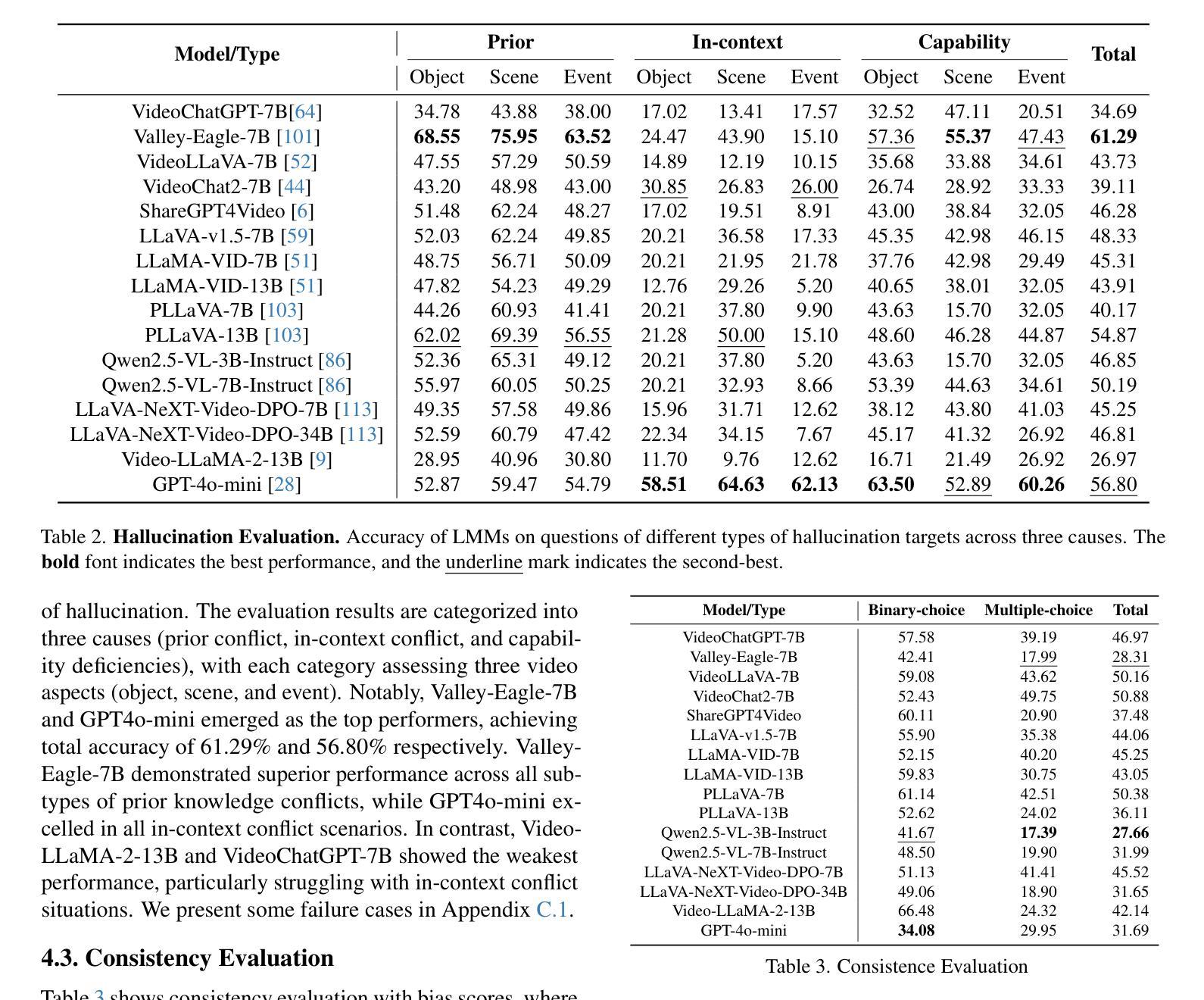
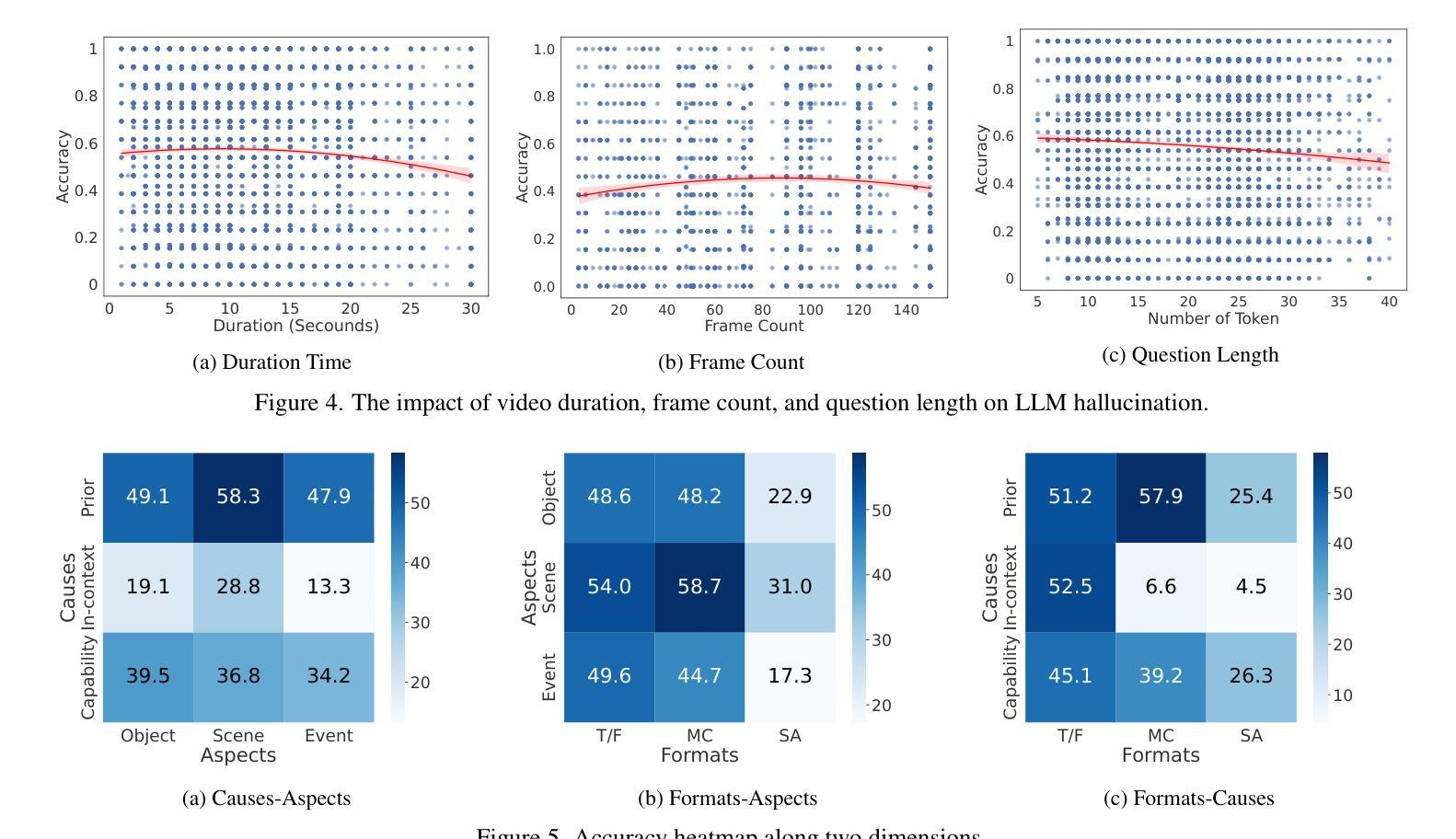
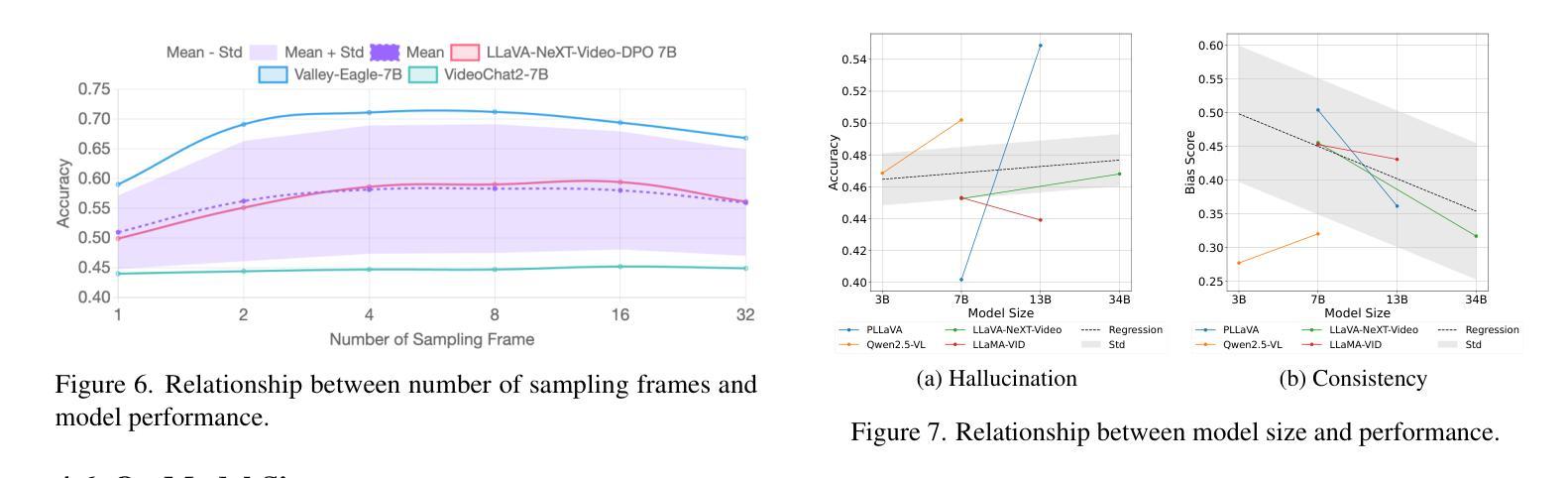
SlowFast-LLaVA-1.5: A Family of Token-Efficient Video Large Language Models for Long-Form Video Understanding
Authors:Mingze Xu, Mingfei Gao, Shiyu Li, Jiasen Lu, Zhe Gan, Zhengfeng Lai, Meng Cao, Kai Kang, Yinfei Yang, Afshin Dehghan
We introduce SlowFast-LLaVA-1.5 (abbreviated as SF-LLaVA-1.5), a family of video large language models (LLMs) offering a token-efficient solution for long-form video understanding. This model family employs the two-stream SlowFast mechanism, enabling efficient modeling of long-range temporal context to meet the demand for lightweight, mobile-friendly Video LLMs. We provide models ranging from 1B to 7B parameters, optimized through a streamlined training pipeline and a high-quality data mixture composed of publicly available datasets. Experimental results demonstrate that SF-LLaVA-1.5 achieves competitive performance on a wide range of video and image benchmarks, with robust results across all model sizes. Notably, SF-LLaVA-1.5 achieves state-of-the-art results in long-form video understanding (e.g., LongVideoBench and MLVU) and excels at small scales (1B and 3B) across various video benchmarks.
我们推出了SlowFast-LLaVA-1.5(简称SF-LLaVA-1.5),这是一款针对长视频理解的高效标记视频大型语言模型(LLM)系列。该模型系列采用双流SlowFast机制,能够高效建模长时序上下文,满足轻量级、移动友好的视频大型语言模型的需求。我们提供了从1B到7B参数的模型,通过简化的训练管道和由公开数据集组成的高质量数据混合进行优化。实验结果表明,SF-LLaVA-1.5在广泛的视频和图像基准测试中表现出竞争力,且各种模型大小的结果都很稳健。值得注意的是,SF-LLaVA-1.5在长视频理解方面达到了最新水平(例如在LongVideoBench和MLVU上),并在各种视频基准测试中在小规模(1B和3B)上表现出色。
论文及项目相关链接
PDF Technical report
Summary
SF-LLaVA-1.5系列视频大语言模型采用两流快慢机制,实现高效建模,满足轻量级移动友好型视频大语言模型的需求。该模型家族具有多种参数规模,从1B到7B不等,优化训练流程和高质量数据混合。实验结果表明,SF-LLaVA-1.5在视频和图像基准测试中表现优异,特别是长视频理解方面达到业界领先水平。
Key Takeaways
- SF-LLaVA-1.5是一种针对长形式视频理解的视频大语言模型家族。
- 采用两流快慢机制,实现高效建模长距离时间上下文。
- 模型系列提供从1B到7B参数的多种选择。
- 通过优化训练流程和使用高质量数据混合来提高模型性能。
- 在各种视频基准测试中表现强劲,特别是长视频理解方面表现突出。
- 在不同的模型规模下均表现出稳健的性能。
点此查看论文截图
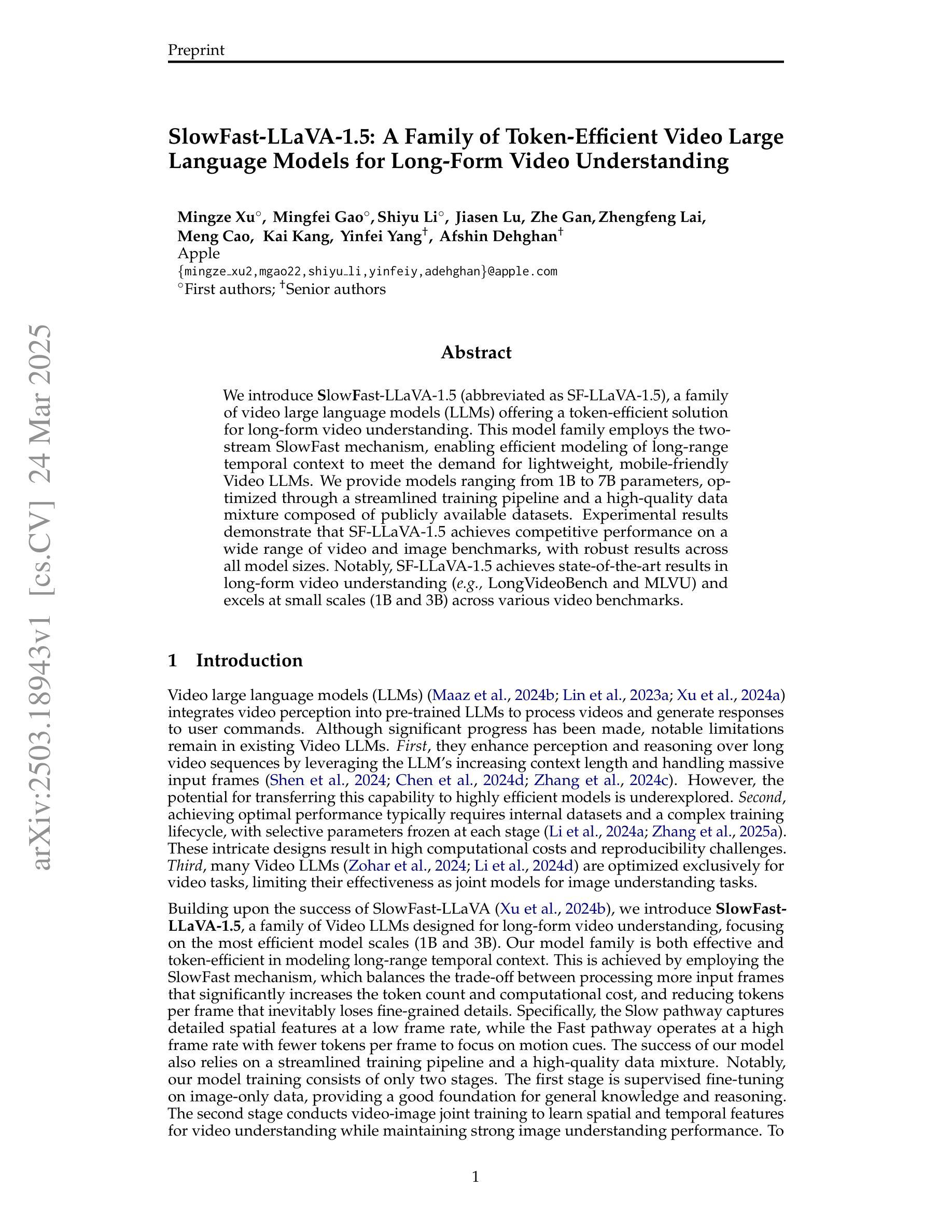
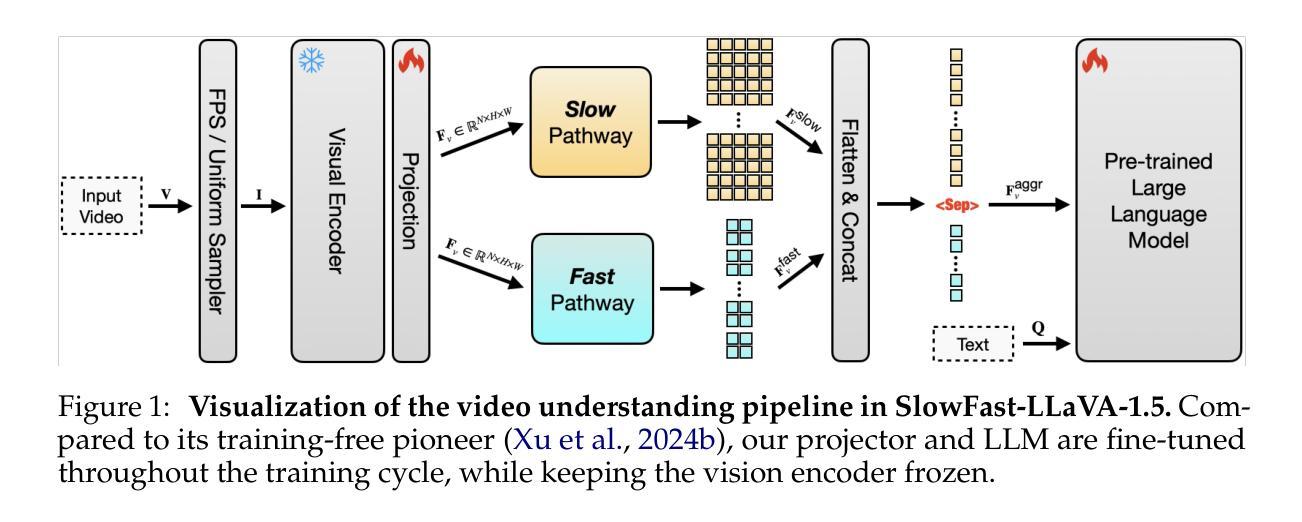
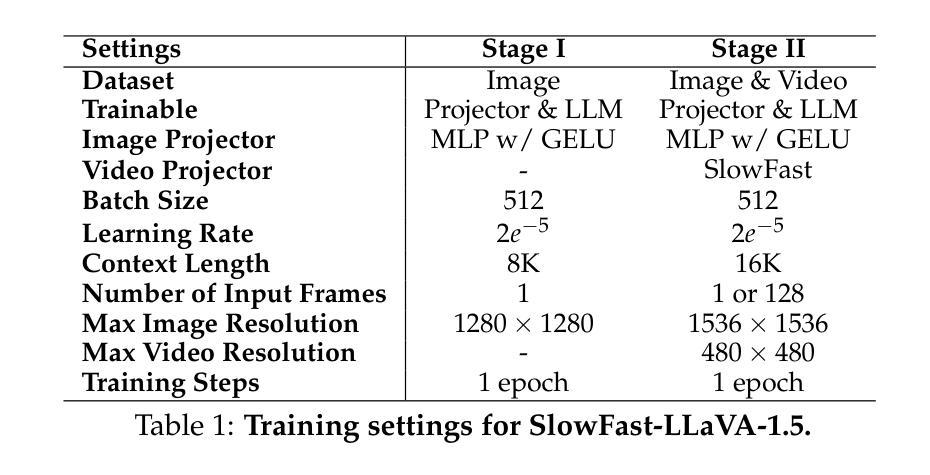
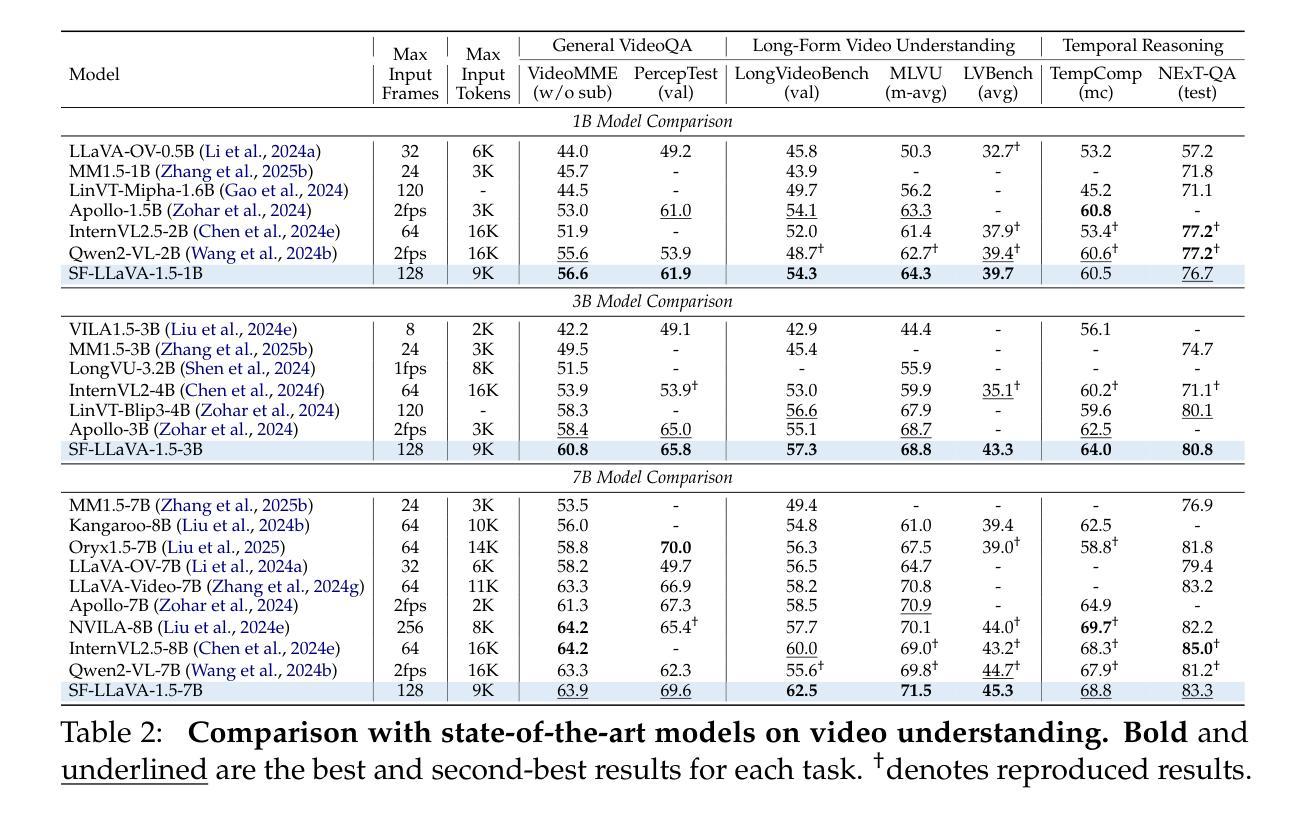
Video-XL-Pro: Reconstructive Token Compression for Extremely Long Video Understanding
Authors:Xiangrui Liu, Yan Shu, Zheng Liu, Ao Li, Yang Tian, Bo Zhao
Despite advanced token compression techniques, existing multimodal large language models (MLLMs) still struggle with hour-long video understanding. In this work, we propose Video-XL-Pro, an efficient method for extremely long video understanding, built upon Reconstructive Compression of Tokens (ReCoT), a learnable module that leverages self-supervised learning to generate comprehensive and compact video tokens. ReCoT introduces two key components: (i) Dynamic Token Synthesizer (DTS): DTS generates pseudo-video tokens from static image tokens by learning intra-token relationships, which are then used in masked video modeling. (ii) Semantic-Guided Masking (SGM): SGM adaptively masks redundant visual tokens to facilitate more effective reconstructive learning. To improve training efficiency in MLLMs fine-tuning, we introduce a video-specific dataset pruning strategy and design a simple yet Query-aware Selector that enables the model to precisely locate query-relevant video tokens. With only 3B parameters, Video-XL-Pro outperforms most 7B models trained on larger datasets across multiple long video understanding benchmarks. Moreover, it can process over 8K frames on a single A100 GPU while maintaining high-quality performance.
尽管有先进的令牌压缩技术,现有的多模态大型语言模型(MLLMs)在理解长达数小时的视频时仍然面临挑战。在这项工作中,我们提出了Video-XL-Pro,这是一种基于令牌重建压缩(ReCoT)的极端长视频理解的有效方法。ReCoT是一个可学习的模块,它利用自监督学习生成全面且紧凑的视频令牌。ReCoT引入了两个关键组件:(i)动态令牌合成器(DTS):DTS通过学习令牌内部关系从静态图像令牌生成伪视频令牌,然后用于遮罩视频建模。(ii)语义引导遮罩(SGM):SGM自适应地遮罩冗余的视觉令牌,以促进更有效的重建学习。为了提高MLLM微调中的训练效率,我们采用了一种针对视频特定的数据集修剪策略,并设计了一个简单但查询感知的选择器,使模型能够精确定位与查询相关的视频令牌。仅使用3B参数,Video-XL-Pro在多个长视频理解基准测试中表现出超过大多数在更大数据集上训练的7B模型的效果。此外,它可以在单个A100 GPU上处理超过8K帧的同时保持高质量的性能。
简化解释
论文及项目相关链接
Summary
本文提出了Video-XL-Pro模型,该模型采用重建令牌压缩技术(ReCoT),能够实现对超长视频的高效理解。ReCoT包含动态令牌合成器和语义引导遮蔽技术,能够生成伪视频令牌并自适应地遮蔽冗余视觉令牌以促进重建学习。此外,为了提高多模态大型语言模型的微调效率,还引入了视频特定数据集修剪策略和查询感知选择器。Video-XL-Pro在多个长视频理解基准测试中表现优异,能够处理单个A100 GPU上的超过8K帧,并保持高质量性能。
Key Takeaways
- Video-XL-Pro利用重建令牌压缩技术(ReCoT)实现了对超长视频的高效理解。
- ReCoT包含动态令牌合成器(DTS)和语义引导遮蔽技术(SGM),用于生成伪视频令牌和自适应遮蔽冗余视觉令牌。
- Video-XL-Pro在多个长视频理解基准测试中表现优异,甚至超越了使用更大数据集训练的模型。
- 该模型具有高效的训练效率,能够处理单个A100 GPU上的大量视频帧。
- Video-XL-Pro采用了视频特定数据集修剪策略,以提高训练效率。
点此查看论文截图
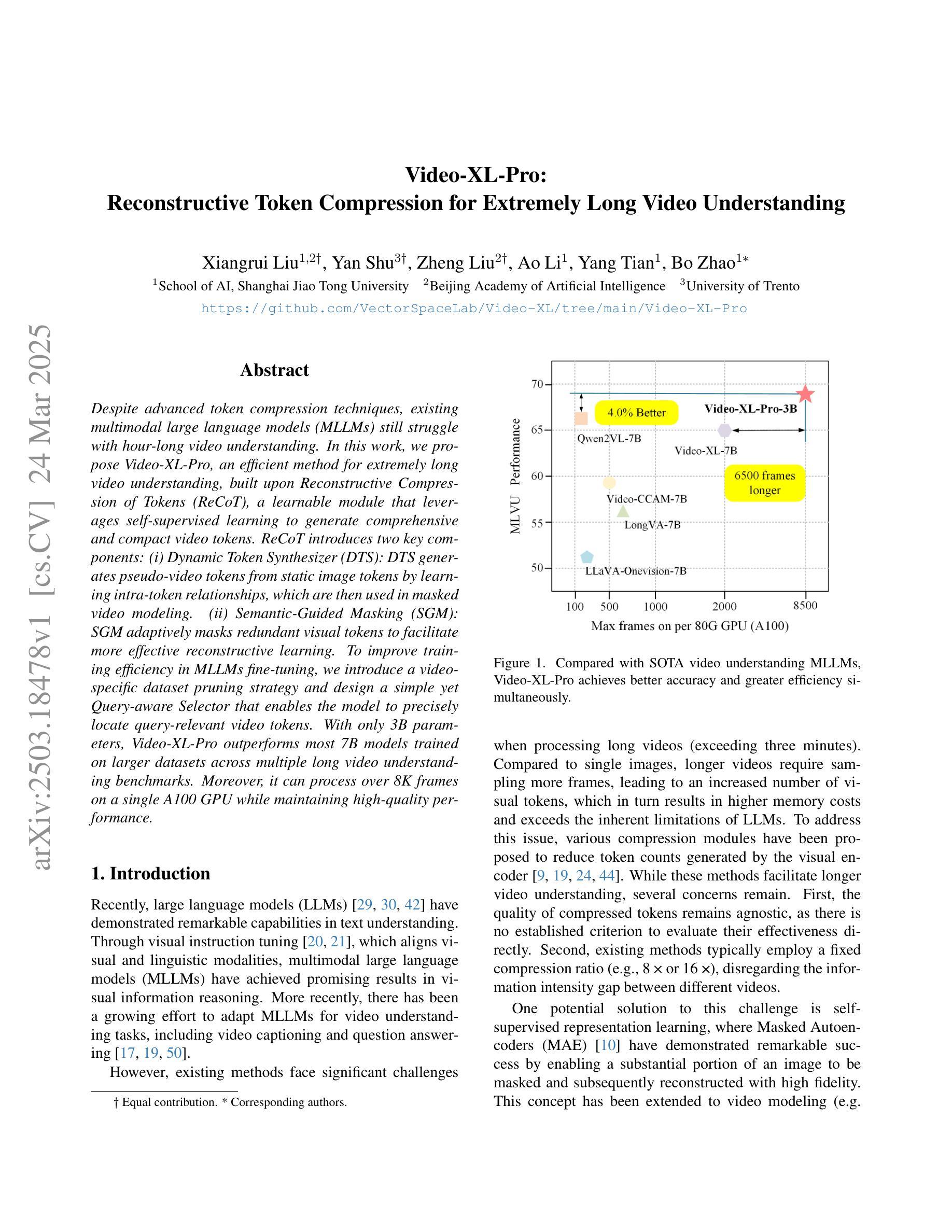
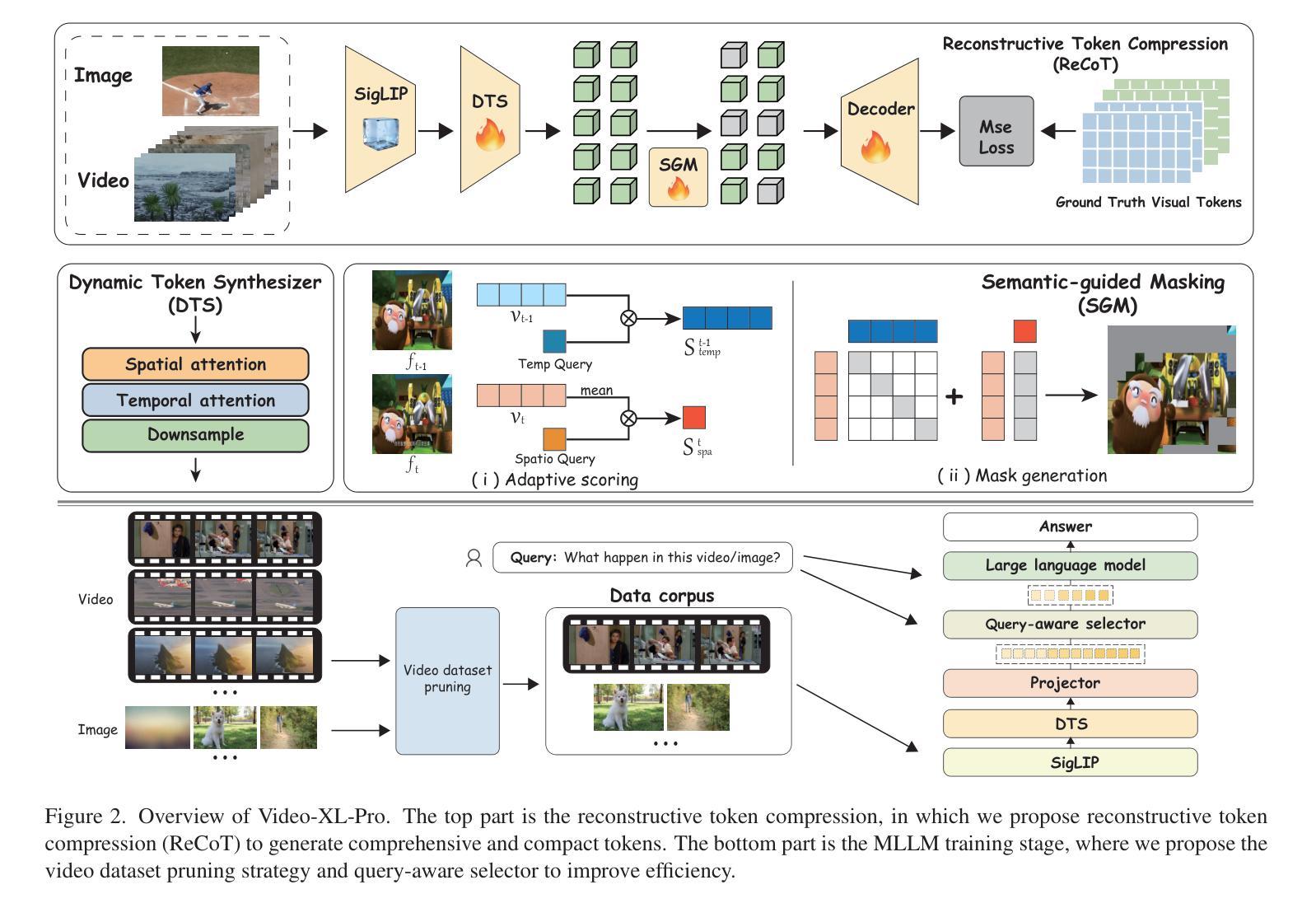
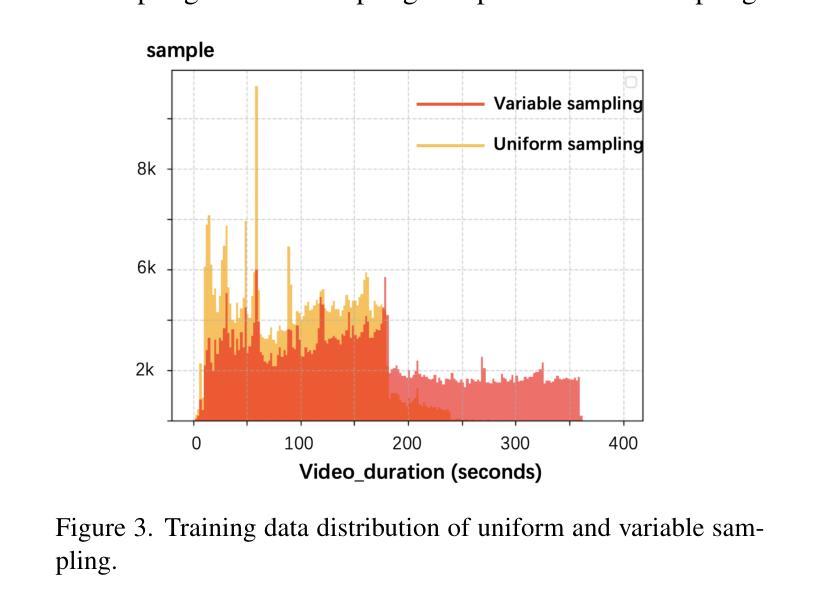
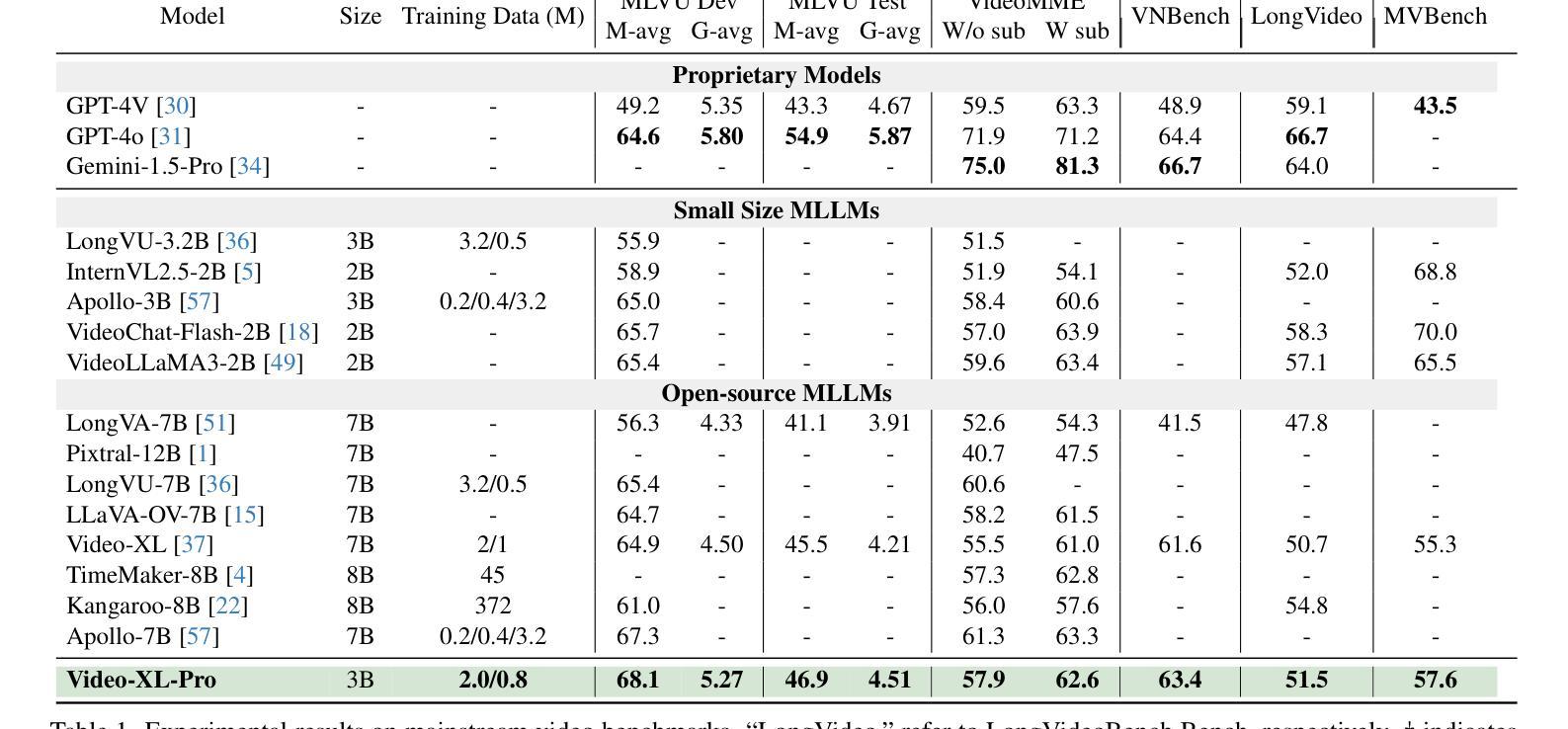
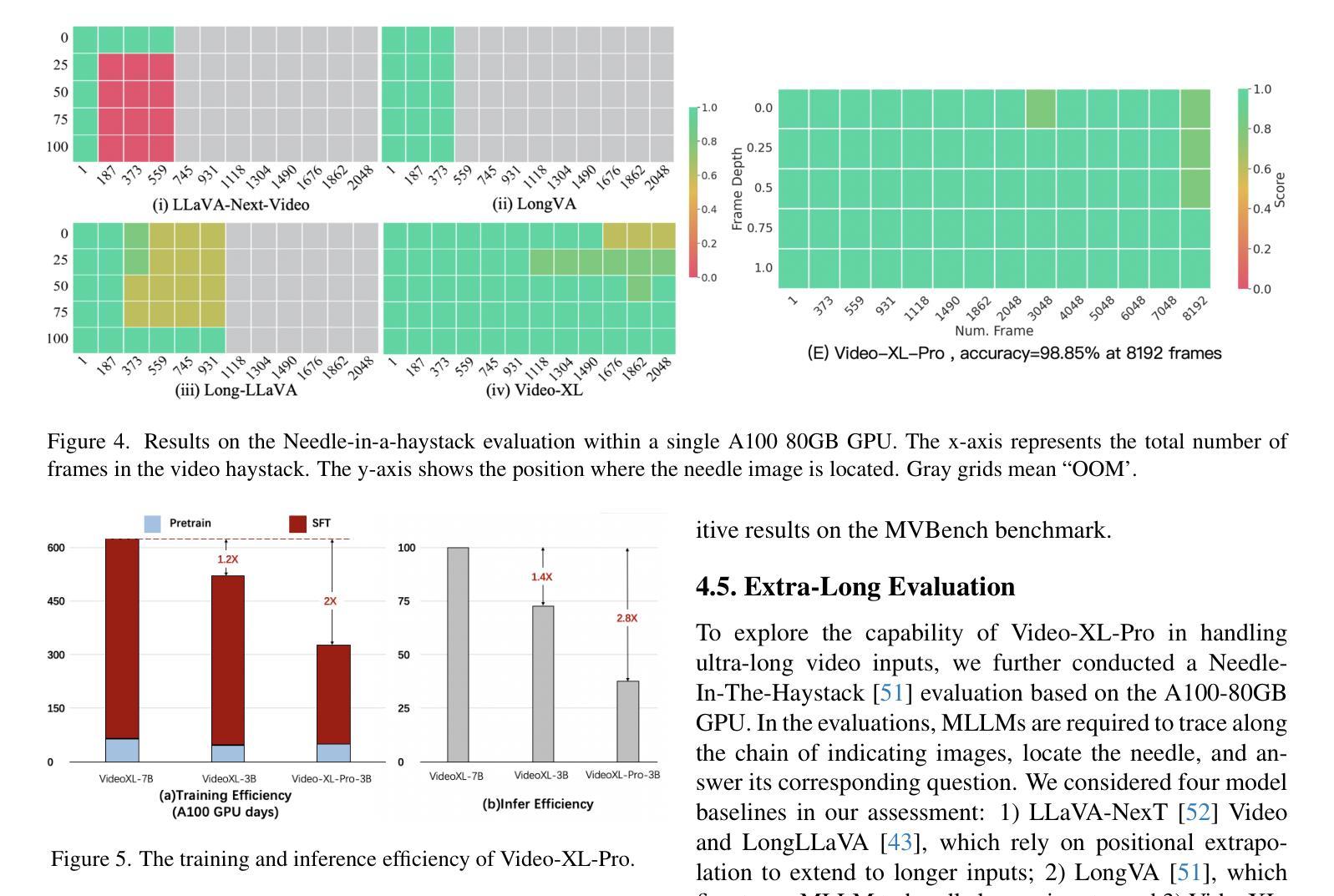
V2P-Bench: Evaluating Video-Language Understanding with Visual Prompts for Better Human-Model Interaction
Authors:Yiming Zhao, Yu Zeng, Yukun Qi, YaoYang Liu, Lin Chen, Zehui Chen, Xikun Bao, Jie Zhao, Feng Zhao
Large Vision-Language Models (LVLMs) have made significant progress in the field of video understanding recently. However, current benchmarks uniformly lean on text prompts for evaluation, which often necessitate complex referential language and fail to provide precise spatial and temporal references. This limitation diminishes the experience and efficiency of human-model interaction. To address this limitation, we propose the Video Visual Prompt Benchmark(V2P-Bench), a comprehensive benchmark specifically designed to evaluate LVLMs’ video understanding capabilities in multimodal human-model interaction scenarios. V2P-Bench includes 980 unique videos and 1,172 QA pairs, covering 5 main tasks and 12 dimensions, facilitating instance-level fine-grained understanding aligned with human cognition. Benchmarking results reveal that even the most powerful models perform poorly on V2P-Bench (65.4% for GPT-4o and 67.9% for Gemini-1.5-Pro), significantly lower than the human experts’ 88.3%, highlighting the current shortcomings of LVLMs in understanding video visual prompts. We hope V2P-Bench will serve as a foundation for advancing multimodal human-model interaction and video understanding evaluation. Project page: https://github.com/gaotiexinqu/V2P-Bench.
大型视觉语言模型(LVLMs)在视频理解领域取得了显著进展。然而,当前的基准测试普遍倾向于使用文本提示进行评估,这通常需要复杂的指代语言,并且无法提供精确的空间和时间参考。这一局限性降低了人与模型互动的体验和效率。为了解决这一局限性,我们提出了视频视觉提示基准测试(V2P-Bench),这是一个专门设计的全面基准测试,用于评估LVLMs在多媒体人机互动场景中的视频理解能力。V2P-Bench包括980个独特视频和1172个问答对,涵盖5个主要任务和12个维度,促进与人类认知相一致的实例级精细粒度理解。基准测试结果表明,即使在V2P-Bench上,最强大的模型表现也不佳(GPT-4o为65.4%,Gemini-1.5-Pro为67.9%),远低于人类专家的88.3%,这凸显了LVLMs在理解视频视觉提示方面的当前不足。我们希望V2P-Bench能成为推进多媒体人机互动和视频理解评估的基础。项目页面:[https://github.com/gaotiexinqu/V2P-Bench。](请点击链接查看详细信息)
论文及项目相关链接
Summary:
大型视觉语言模型(LVLMs)在视频理解领域取得显著进展,但现有评估基准倾向于使用文本提示,这常常需要复杂的指代语言,无法提供精确的空间和时间参考,限制了人与模型的互动体验与效率。为此,我们提出视频视觉提示基准测试(V2P-Bench),专门评估LVLMs在多模态人机互动场景中的视频理解能力。V2P-Bench包含980个独特视频和1172个问答对,涵盖5个主要任务和12个维度,促进与人类认知相一致的实例级精细理解。评估结果显示,最强大的模型在V2P-Bench上的表现仍然不佳(GPT-4o为65.4%,Gemini-1.5-Pro为67.9%),远低于人类专家的88.3%,突显出LVLMs在理解视频视觉提示方面的当前不足。我们期望V2P-Bench能为推进多模态人机互动和视频理解评估奠定基础。
Key Takeaways:
- 大型视觉语言模型(LVLMs)在视频理解领域有重要进展。
- 现有评估基准倾向于使用文本提示,存在复杂指代语言和缺乏精确空间时间参考的问题。
- 为解决此问题,提出视频视觉提示基准测试(V2P-Bench)。
- V2P-Bench包含980个视频和1172个问答对,涵盖5个任务和12个维度。
- 评估显示,现有强大模型在V2P-Bench上的表现仍低于人类专家,突显LVLMs在理解视频视觉提示方面的不足。
- V2P-Bench为推进多模态人机互动和视频理解评估奠定了基础。
点此查看论文截图
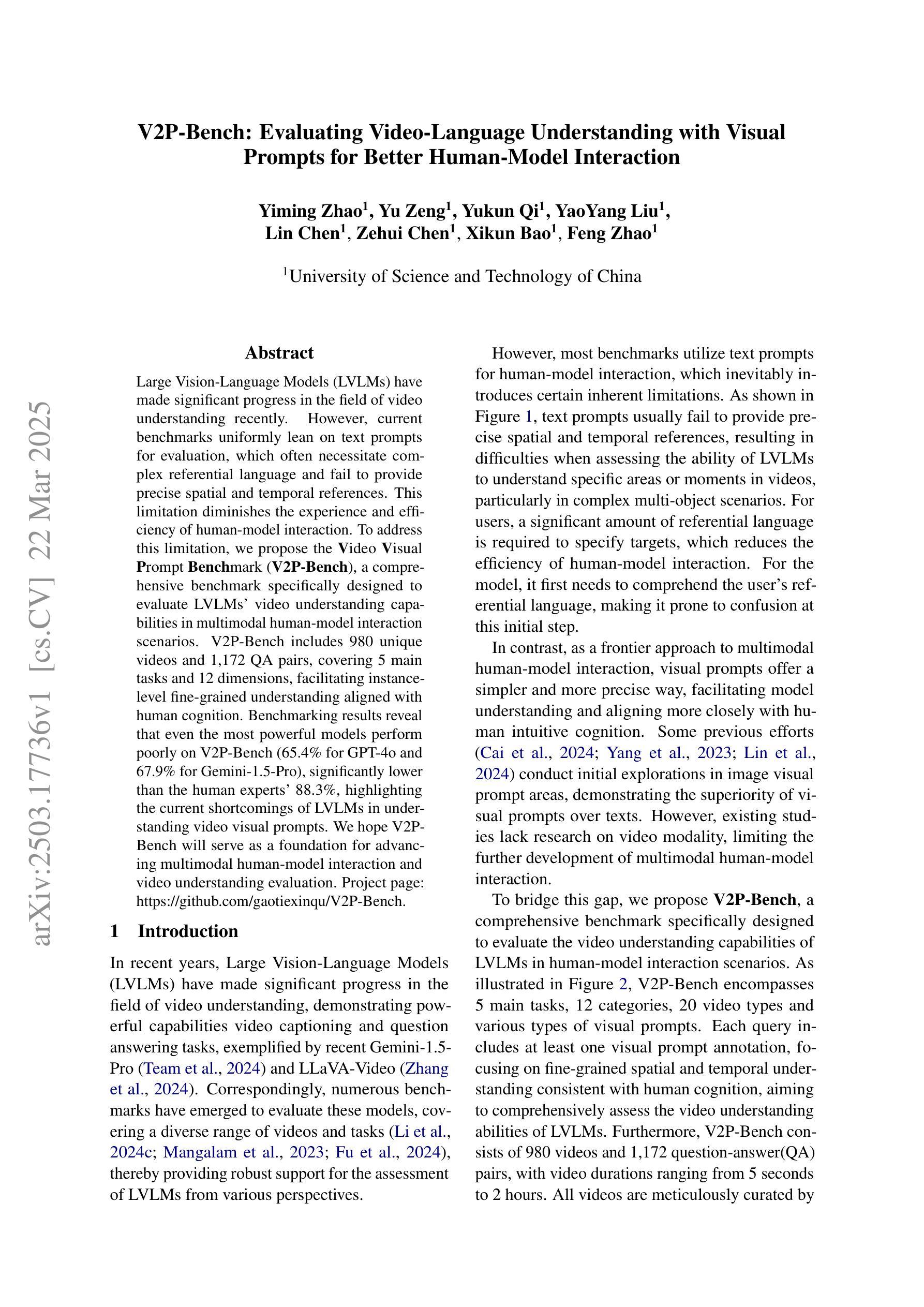
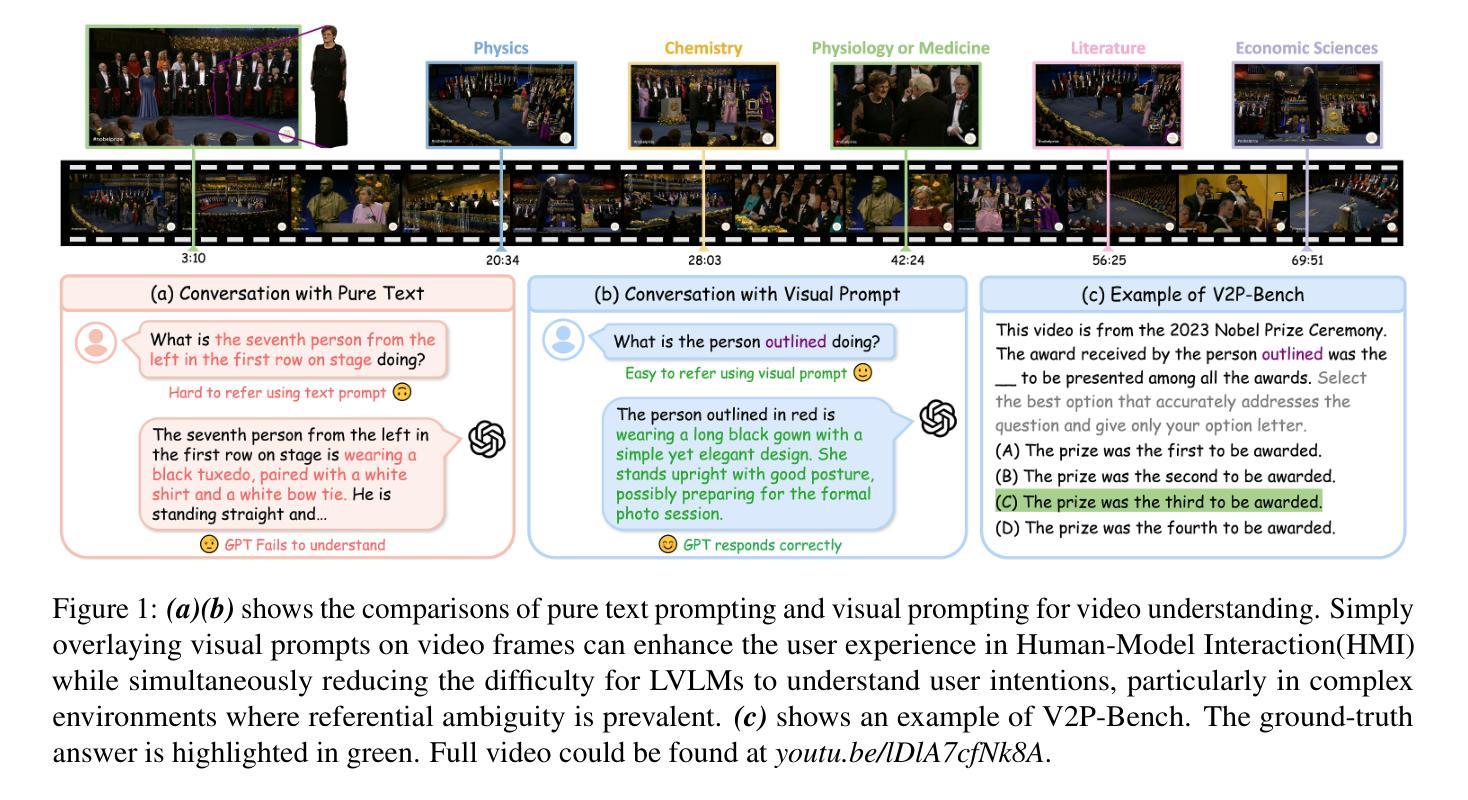
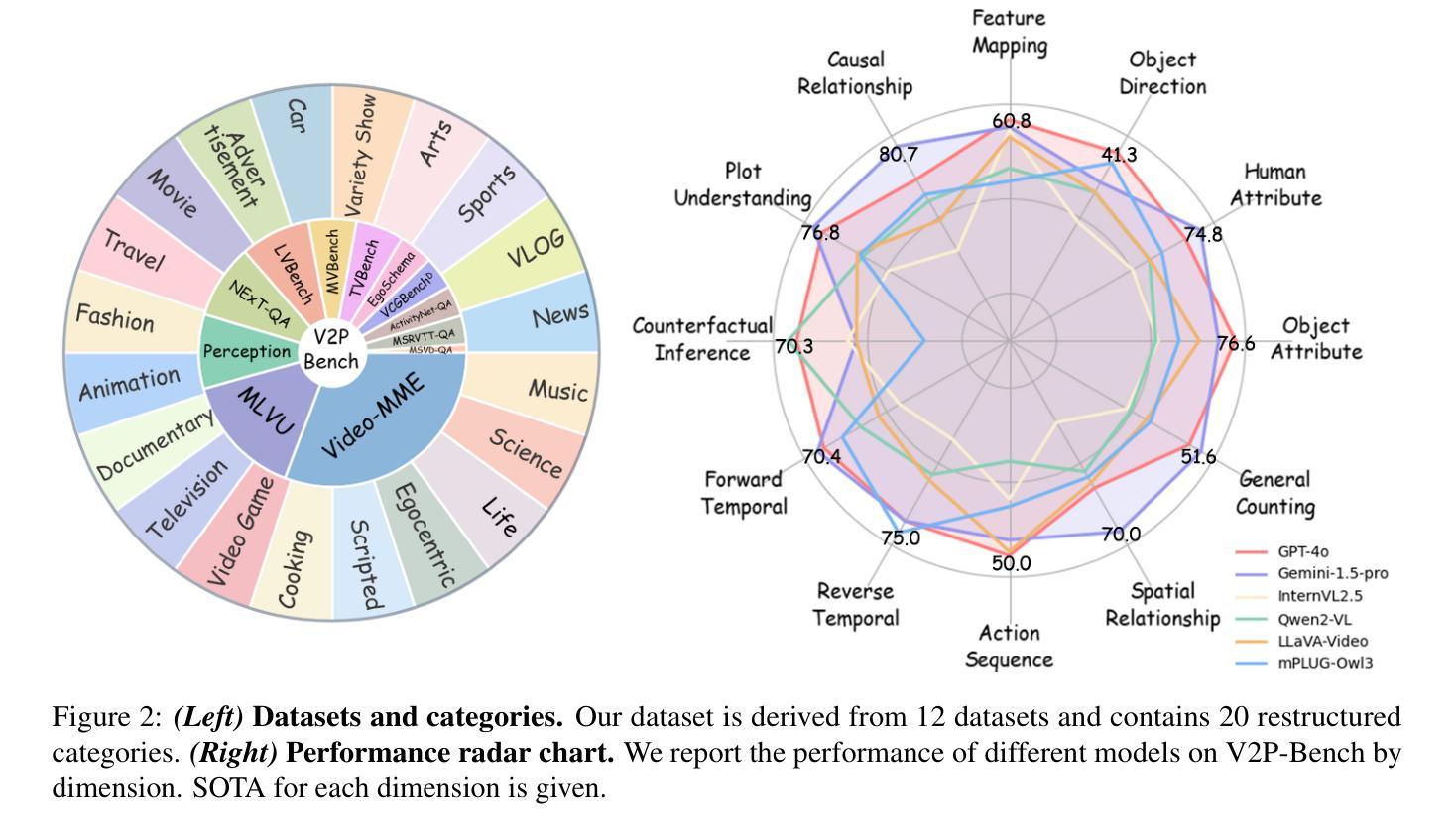
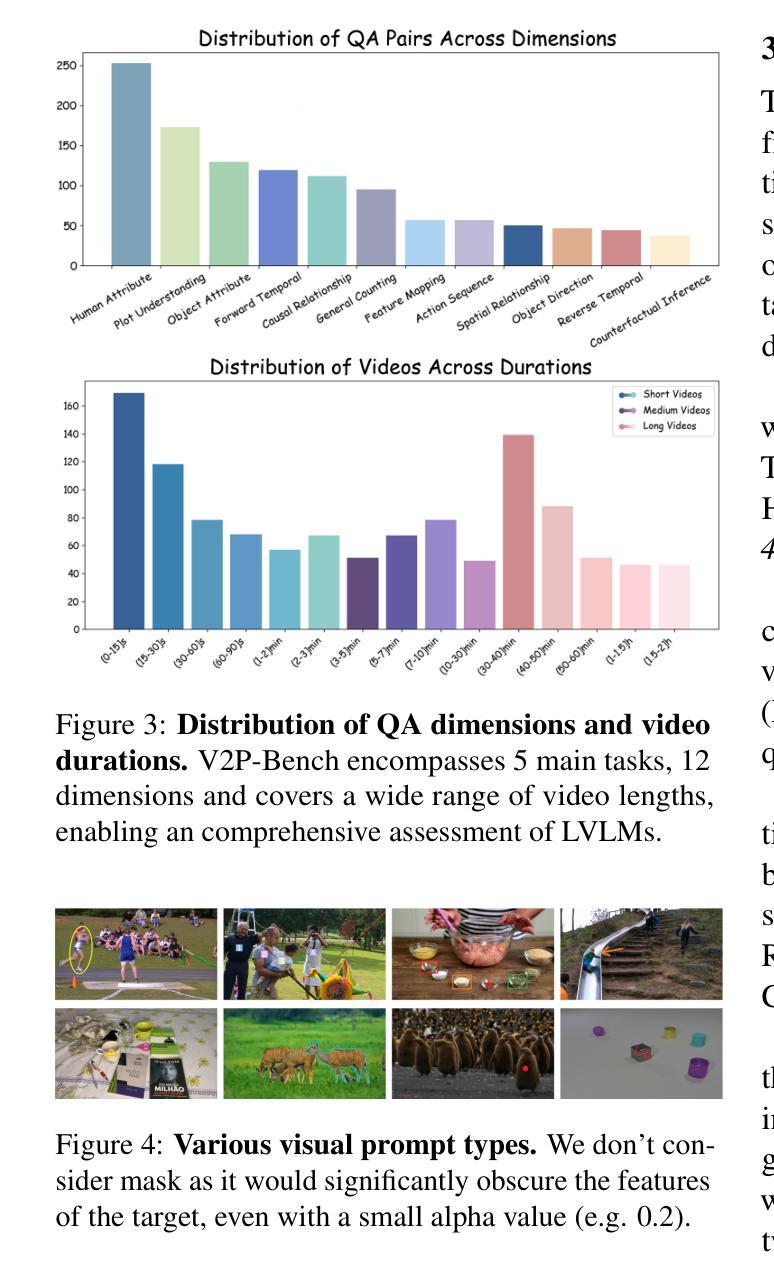
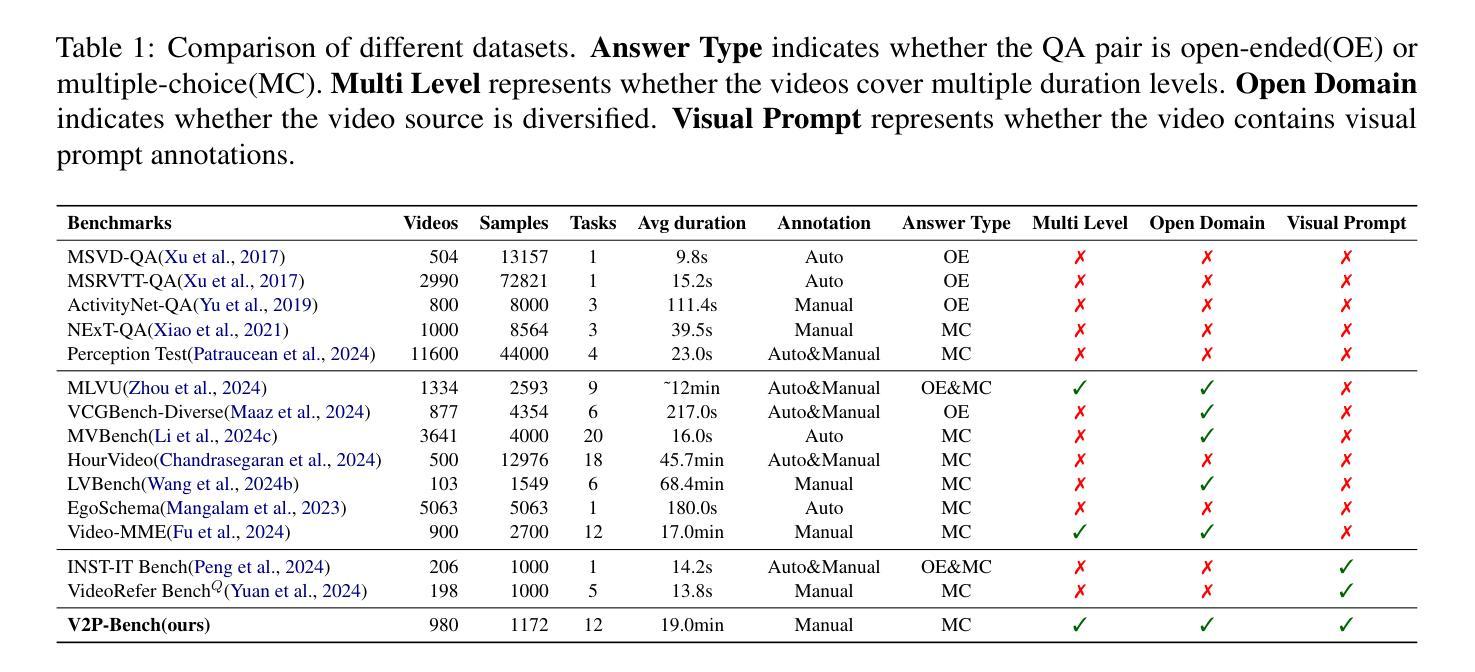
STOP: Integrated Spatial-Temporal Dynamic Prompting for Video Understanding
Authors:Zichen Liu, Kunlun Xu, Bing Su, Xu Zou, Yuxin Peng, Jiahuan Zhou
Pre-trained on tremendous image-text pairs, vision-language models like CLIP have demonstrated promising zero-shot generalization across numerous image-based tasks. However, extending these capabilities to video tasks remains challenging due to limited labeled video data and high training costs. Recent video prompting methods attempt to adapt CLIP for video tasks by introducing learnable prompts, but they typically rely on a single static prompt for all video sequences, overlooking the diverse temporal dynamics and spatial variations that exist across frames. This limitation significantly hinders the model’s ability to capture essential temporal information for effective video understanding. To address this, we propose an integrated Spatial-TempOral dynamic Prompting (STOP) model which consists of two complementary modules, the intra-frame spatial prompting and inter-frame temporal prompting. Our intra-frame spatial prompts are designed to adaptively highlight discriminative regions within each frame by leveraging intra-frame attention and temporal variation, allowing the model to focus on areas with substantial temporal dynamics and capture fine-grained spatial details. Additionally, to highlight the varying importance of frames for video understanding, we further introduce inter-frame temporal prompts, dynamically inserting prompts between frames with high temporal variance as measured by frame similarity. This enables the model to prioritize key frames and enhances its capacity to understand temporal dependencies across sequences. Extensive experiments on various video benchmarks demonstrate that STOP consistently achieves superior performance against state-of-the-art methods. The code is available at https://github.com/zhoujiahuan1991/CVPR2025-STOP.
预训练于海量的图像文本对上,视觉语言模型如CLIP在众多的图像任务中展现出了有前景的零样本泛化能力。然而,将这些能力扩展到视频任务仍然具有挑战性,因为标注的视频数据有限且训练成本高昂。最近的视频提示方法试图通过引入可学习的提示来适应CLIP进行视频任务,但它们通常使用单一静态提示来处理所有视频序列,忽视了跨帧存在的各种时间动态和空间变化。这一局限性显著阻碍了模型捕捉有效视频理解所需的关键时间信息的能力。为了解决这一问题,我们提出了一种集成时空动态提示(STOP)模型,该模型由两个互补模块组成:帧内空间提示和帧间时间提示。我们的帧内空间提示旨在利用帧内注意力和时间变化来动态突出显示每个帧中的判别区域,使模型能够关注具有重大时间动态的区域并捕捉精细的空间细节。此外,为了突出不同帧对视频理解的重要性,我们进一步引入了帧间时间提示,根据帧相似性测量在具有高时间方差的帧之间动态插入提示。这使得模型能够优先处理关键帧并增强其理解序列中时间依赖性的能力。在各种视频基准测试上的大量实验表明,STOP始终实现了对最先进方法的优越性能。代码可在https://github.com/zhoujiahuan1991/CVPR2025-STOP上找到。
论文及项目相关链接
Summary
针对视频任务,CLIP等视觉语言模型的零样本泛化能力因缺少标注视频数据和训练成本高昂而受到挑战。为应对此问题,研究人员提出了引入可学习提示的视频提示方法,但现有方法忽视了视频帧间的多样性和时空动态变化。为此,我们提出了集成时空动态提示(STOP)模型,包括帧内空间提示和帧间时间提示两个互补模块。STOP模型能自适应地突出关键帧和精细的空间细节,并在各种视频基准测试中表现优异。
Key Takeaways
- CLIP等视觉语言模型在图像任务上表现出良好的零样本泛化能力,但在视频任务上仍面临挑战。
- 当前视频提示方法主要依赖单一静态提示,忽略了视频的时空动态变化。
- STOP模型通过帧内空间提示和帧间时间提示两个互补模块,自适应地突出关键帧和精细的空间细节。
- STOP模型利用帧内注意力和时间变化设计帧内空间提示。
- STOP模型通过测量帧相似性,动态地在帧间插入提示,以突出不同帧的重要性。
- STOP模型在各种视频基准测试中的表现优于现有方法。
点此查看论文截图
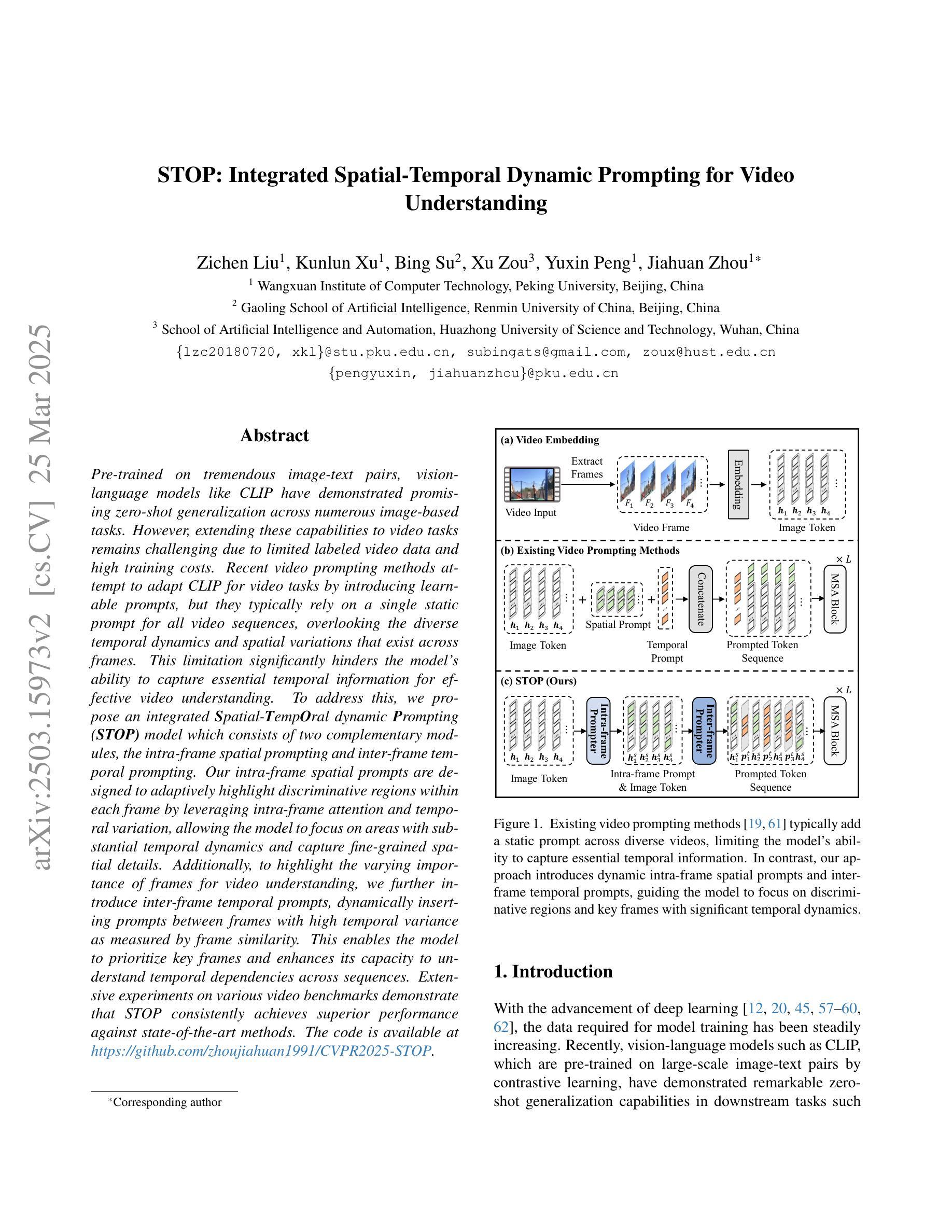
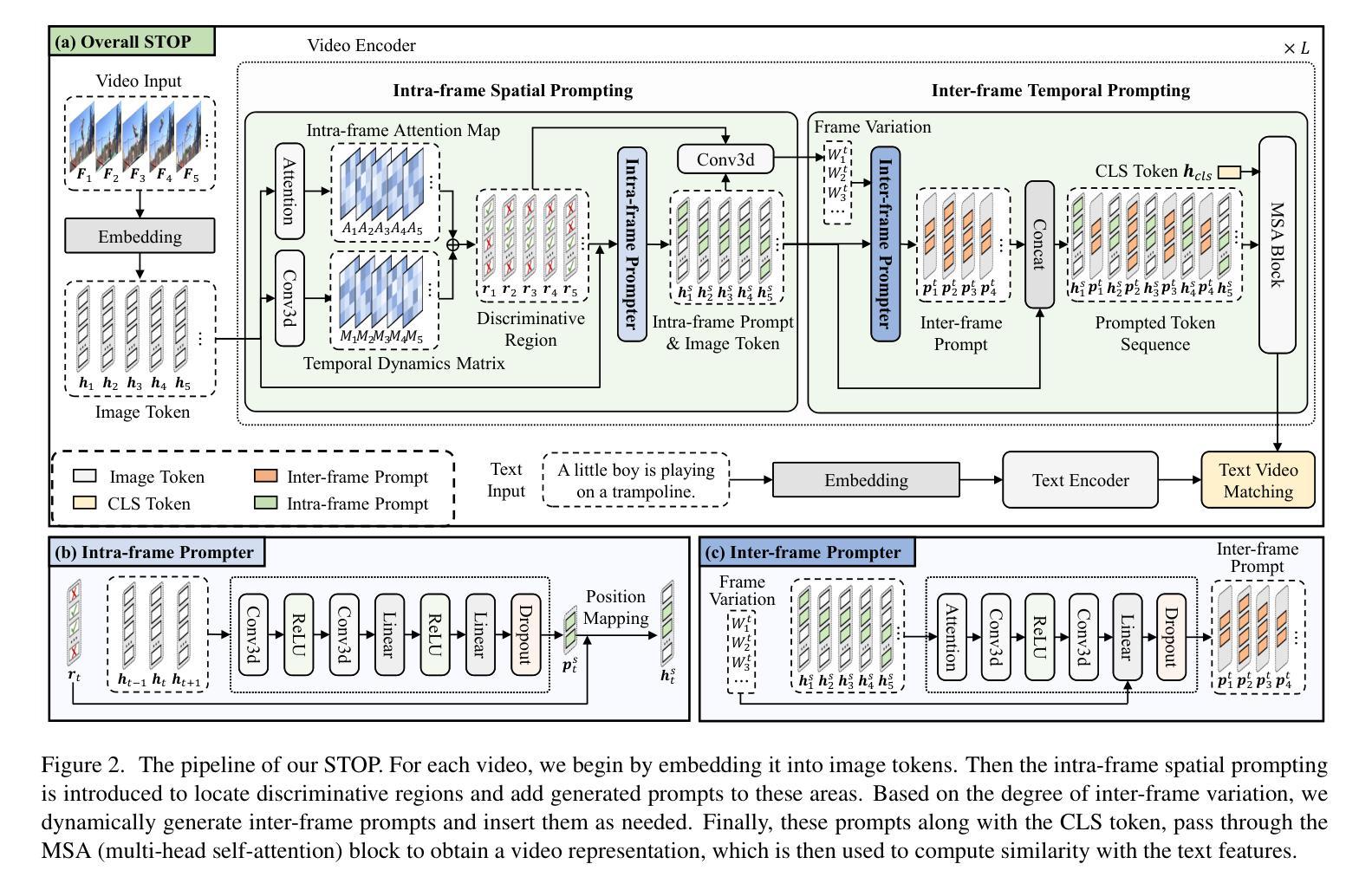
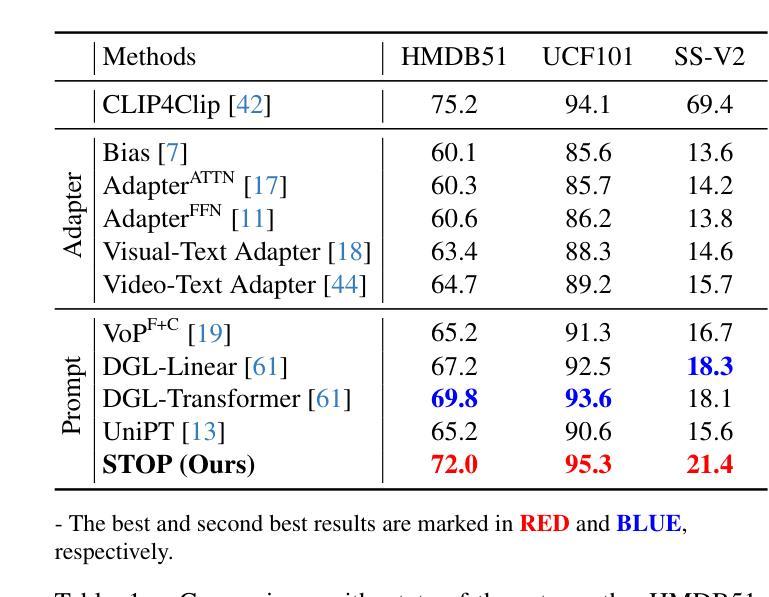
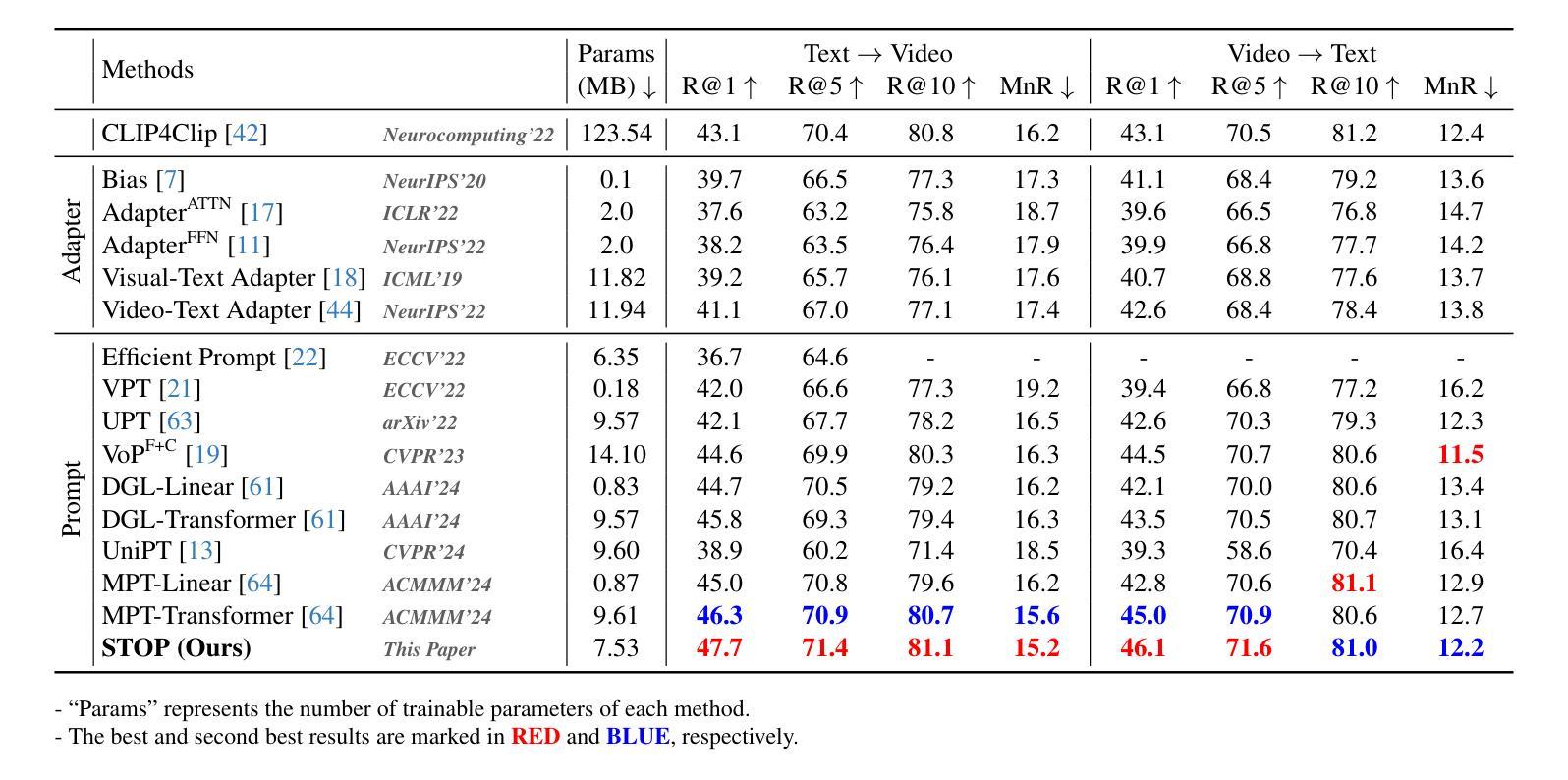
Omni-RGPT: Unifying Image and Video Region-level Understanding via Token Marks
Authors:Miran Heo, Min-Hung Chen, De-An Huang, Sifei Liu, Subhashree Radhakrishnan, Seon Joo Kim, Yu-Chiang Frank Wang, Ryo Hachiuma
We present Omni-RGPT, a multimodal large language model designed to facilitate region-level comprehension for both images and videos. To achieve consistent region representation across spatio-temporal dimensions, we introduce Token Mark, a set of tokens highlighting the target regions within the visual feature space. These tokens are directly embedded into spatial regions using region prompts (e.g., boxes or masks) and simultaneously incorporated into the text prompt to specify the target, establishing a direct connection between visual and text tokens. To further support robust video understanding without requiring tracklets, we introduce an auxiliary task that guides Token Mark by leveraging the consistency of the tokens, enabling stable region interpretation across the video. Additionally, we introduce a large-scale region-level video instruction dataset (RegVID-300k). Omni-RGPT achieves state-of-the-art results on image and video-based commonsense reasoning benchmarks while showing strong performance in captioning and referring expression comprehension tasks.
我们推出了Omni-RGPT,这是一款多模态大型语言模型,旨在促进图像和视频的区域级理解。为了实现时空维度上的一致区域表示,我们引入了Token Mark,这是一组突出显示目标区域的令牌,直接嵌入到空间区域中使用区域提示(例如,盒子或蒙版),并同时纳入文本提示以指定目标,从而在视觉令牌和文本令牌之间建立直接联系。为了进一步支持不需要轨迹的稳健视频理解,我们引入了一个辅助任务,通过利用令牌的一致性来指导Token Mark,实现在视频中的稳定区域解释。此外,我们还引入了一个大规模的区域级视频指令数据集(RegVID-300k)。Omni-RGPT在图像和基于视频的常识推理基准测试上达到了最新技术水准,同时在描述和指代表达理解任务中表现出强劲性能。
论文及项目相关链接
PDF CVPR 2025, Project page: https://miranheo.github.io/omni-rgpt/
Summary:
我们提出了Omni-RGPT,这是一款多模态大型语言模型,旨在实现图像和视频的区域级别理解。通过引入Token Mark,该模型能在时空维度上实现一致的区域表示。Token Mark是一组标记目标区域的令牌,它们通过区域提示(如框或掩膜)直接嵌入到空间区域中,并通过文本提示指定目标,从而建立视觉和文本令牌之间的直接联系。为了支持不需要轨迹的稳健视频理解,我们引入了一个辅助任务,通过利用令牌的一致性来引导Token Mark,实现在视频中的稳定区域解释。此外,我们还引入了一个大规模的区域级别视频指令数据集(RegVID-300k)。Omni-RGPT在图像和基于视频的常识推理基准测试上达到了最先进的成果,同时在描述和引用表达理解任务中表现出强大的性能。
Key Takeaways:
- Omni-RGPT是一个多模态大型语言模型,旨在促进图像和视频的区域级别理解。
- Token Mark是Omni-RGPT的核心组件,它通过区域提示和文本提示建立视觉和文本令牌之间的直接联系。
- 引入了一个辅助任务来支持稳定的区域解释,可以在视频中进行一致的理解。
- Token Mark通过在时空维度上实现一致的区域表示,实现图像和视频理解的一致性。
- 引入的大规模区域级别视频指令数据集RegVID-300k对Omni-RGPT的性能提升起到了重要作用。
- Omni-RGPT在图像和基于视频的常识推理基准测试中取得了最先进的成果。
点此查看论文截图
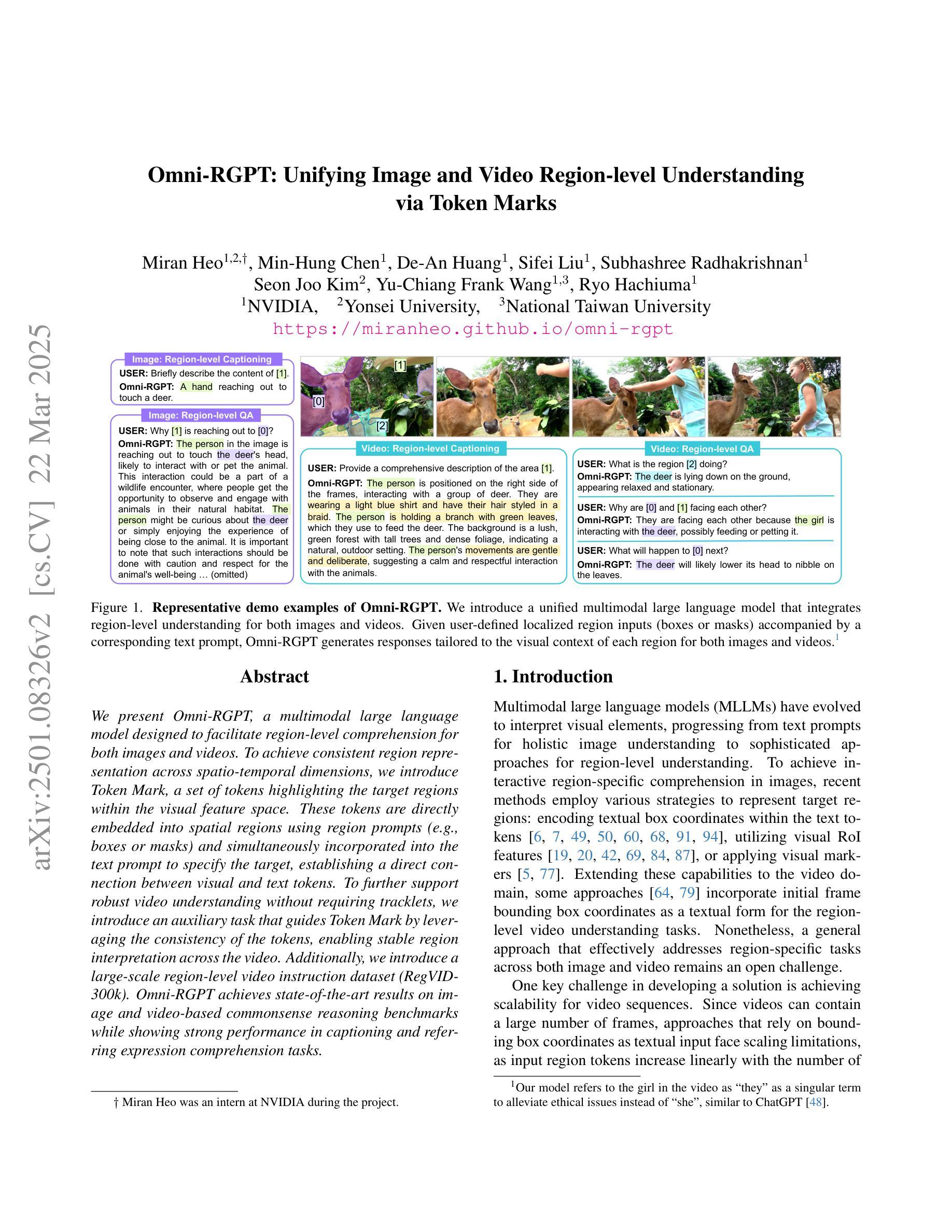
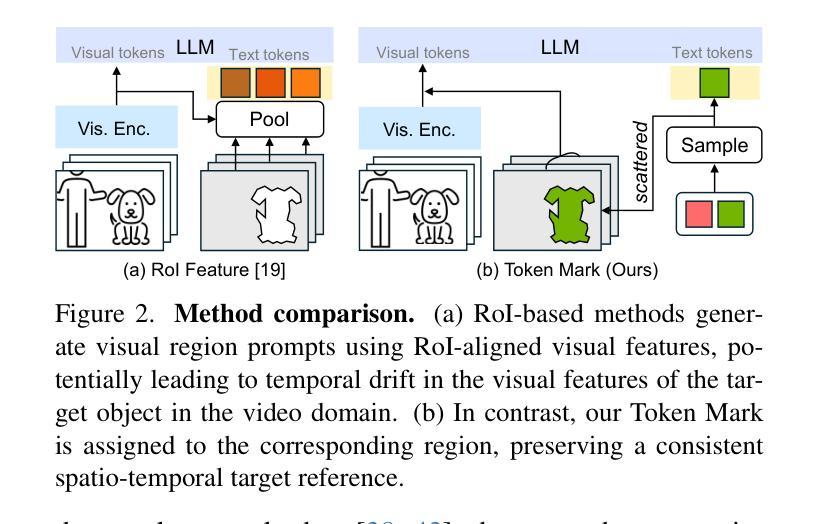
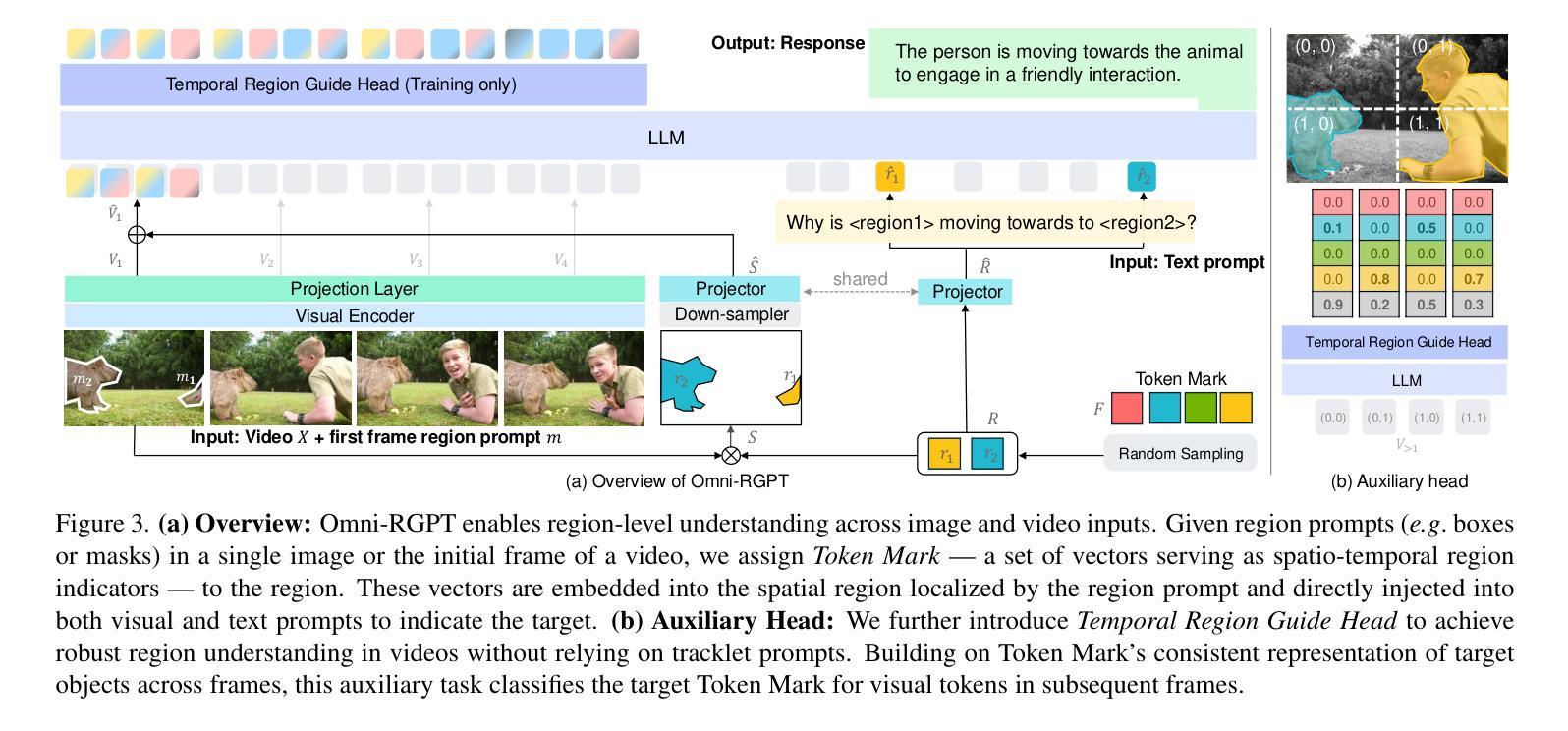
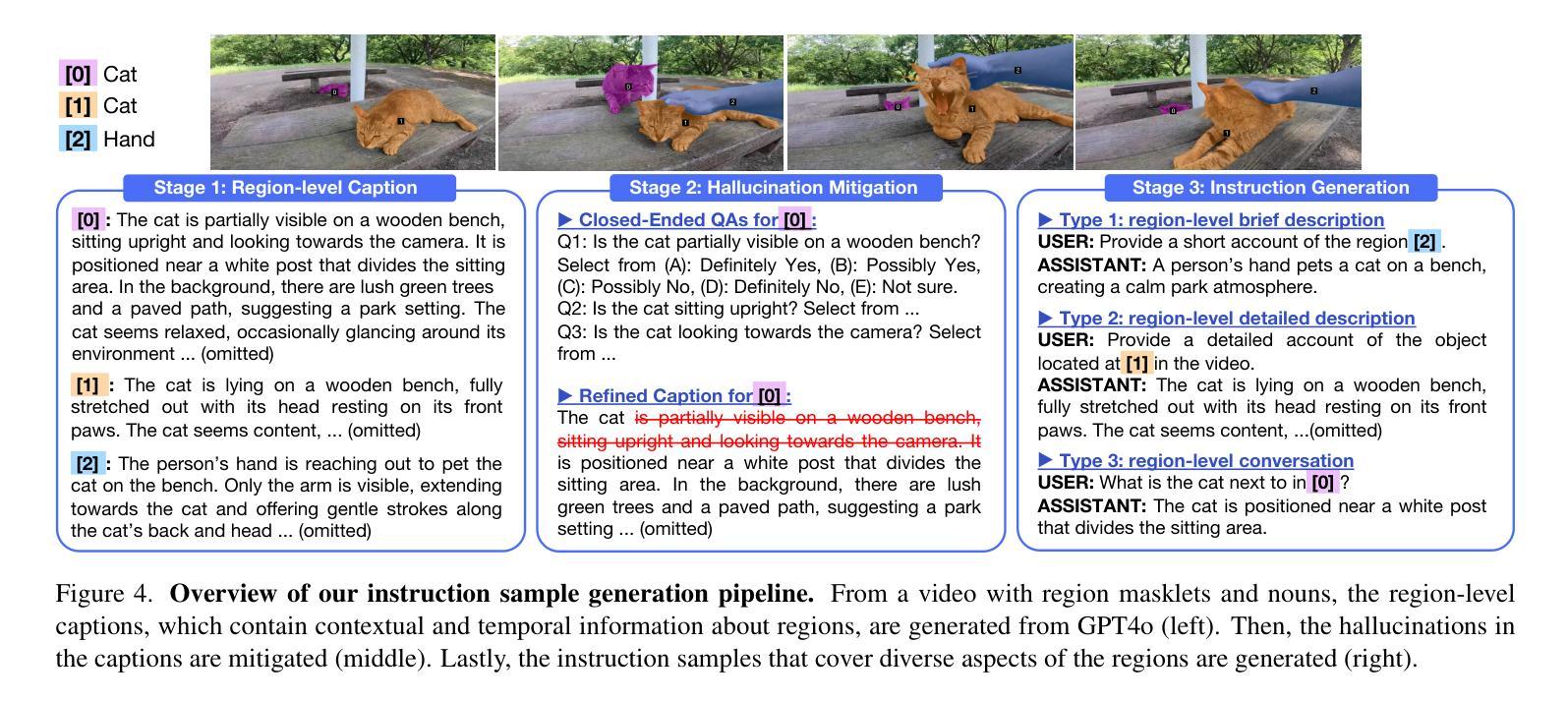
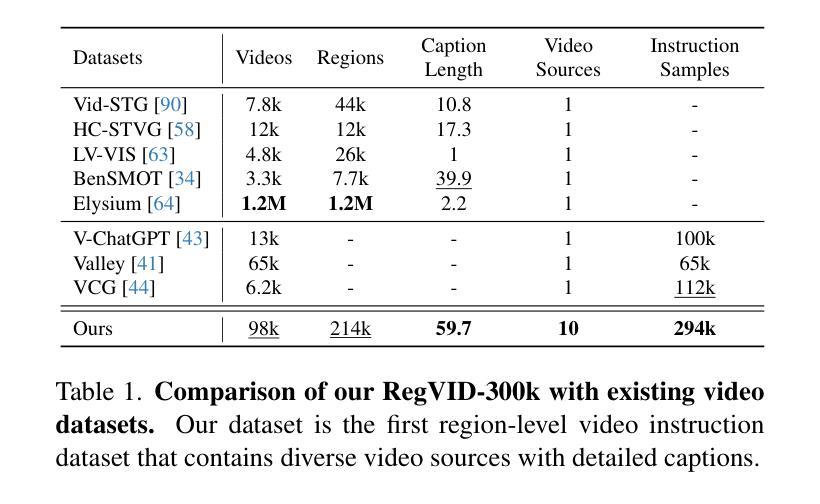
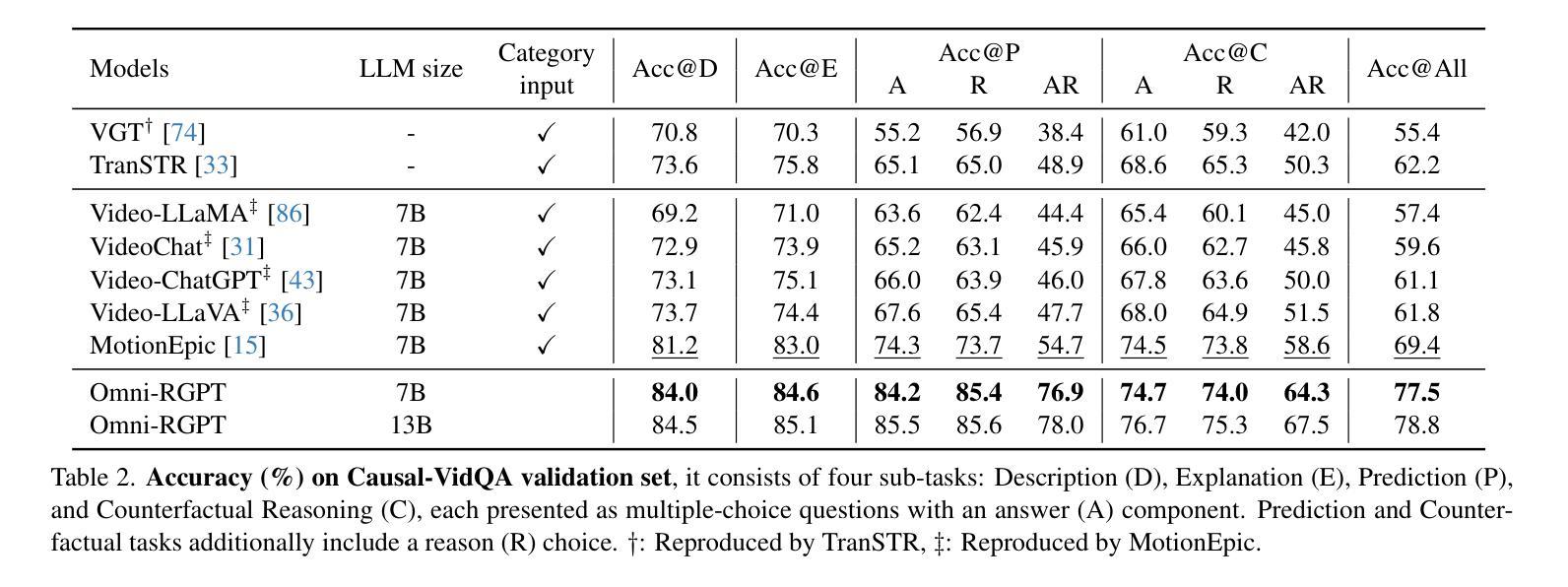
ReTaKe: Reducing Temporal and Knowledge Redundancy for Long Video Understanding
Authors:Xiao Wang, Qingyi Si, Jianlong Wu, Shiyu Zhu, Li Cao, Liqiang Nie
Video Large Language Models (VideoLLMs) have made significant strides in video understanding but struggle with long videos due to the limitations of their backbone LLMs. Existing solutions rely on length extrapolation, which is memory-constrained, or visual token compression, which primarily leverages low-level temporal redundancy while overlooking the more effective high-level knowledge redundancy. To address this, we propose $\textbf{ReTaKe}$, a training-free method with two novel modules DPSelect and PivotKV, to jointly reduce both temporal visual redundancy and knowledge redundancy for video compression. To align with the way of human temporal perception, DPSelect identifies keyframes based on inter-frame distance peaks. To leverage LLMs’ learned prior knowledge, PivotKV marks the keyframes as pivots and compress non-pivot frames by pruning low-attention tokens in their KV cache. ReTaKe enables VideoLLMs to process 8 times longer frames (up to 2048), outperforming similar-sized models by 3-5% and even rivaling much larger ones on VideoMME, MLVU, LongVideoBench, and LVBench. Moreover, by overlapping compression operations with prefilling, ReTaKe introduces only ~10% prefilling latency overhead while reducing decoding latency by ~20%. Our code is available at https://github.com/SCZwangxiao/video-ReTaKe.
视频大语言模型(VideoLLMs)在视频理解方面取得了显著进展,但由于其背后的大语言模型的局限性,在处理长视频时面临困难。现有解决方案依赖于内存受限的长度扩展或视觉令牌压缩,后者主要利用低级的时序冗余,而忽略了更高效的高级知识冗余。为了解决这个问题,我们提出了无需训练的ReTaKe方法,该方法包含两个新颖模块DPSelect和PivotKV,可联合减少时序视觉冗余和知识冗余,用于视频压缩。为了与人类的时间感知方式保持一致,DPSelect基于帧间距离峰值识别关键帧。为了利用LLMs的先验知识,PivotKV将关键帧标记为枢轴,并通过删除其KV缓存中的低关注令牌来压缩非枢轴帧。ReTaKe使VideoLLMs能够处理8倍更长的帧(最多可达2048帧),在VideoMME、MLVU、LongVideoBench和LVBench上的表现优于类似规模的模型3-5%,甚至与更大的模型相抗衡。此外,通过压缩操作与预填充的重叠,ReTaKe仅引入约10%的预填充延迟开销,同时减少约20%的解码延迟。我们的代码可用在https://github.com/SCZwangxiao/video-ReTaKe。
论文及项目相关链接
PDF Rewrite the methods section. Add more ablation studies and results in LongVideoBench. Update metadata
摘要
视频大型语言模型(VideoLLMs)在视频理解方面取得了显著进展,但由于其骨干LLMs的局限性,在处理长视频时面临挑战。现有解决方案通常采用内存受限的长度扩展或主要利用低级别时间冗余的视觉令牌压缩,忽视了更高效的高级知识冗余。为解决这一问题,我们提出了无需训练的ReTaKe方法,包含两个新颖模块DPSelect和PivotKV,可联合减少时间视觉冗余和知识冗余,实现视频压缩。DPSelect基于帧间距离峰值识别关键帧,与人的时间感知方式相符。PivotKV将关键帧标记为支点,并利用LLMs的先验知识压缩非支点帧,通过删除低关注令牌来减少其KV缓存。ReTaKe使VideoLLMs能够处理长达8倍的视频帧(最多达2048帧),在VideoMME、MLVU、LongVideoBench和LVBench上的性能优于同类模型3-5%,甚至可与更大的模型相抗衡。此外,通过压缩操作与预填充的重叠,ReTaKe仅引入约10%的预填充延迟开销,同时降低约20%的解码延迟。我们的代码可在https://github.com/SCZwangxiao/video-ReTaKe获取。
要点
- VideoLLMs在视频理解上表现出显著进步,但在处理长视频时因骨干LLMs的局限性而面临挑战。
- 现有解决方案主要依赖内存受限的长度扩展或仅利用低级别时间冗余的视觉令牌压缩。
- ReTaKe方法通过两个新颖模块DPSelect和PivotKV联合减少时间视觉冗余和知识冗余,实现视频压缩。
- DPSelect根据帧间距离峰值识别关键帧,与人的时间感知相符。
- PivotKV利用LLMs的先验知识压缩非支点帧。
- ReTaKe提升了VideoLLMs处理长视频的能力,最多可处理长达两倍的视频长度(即最多达2048帧)。
点此查看论文截图
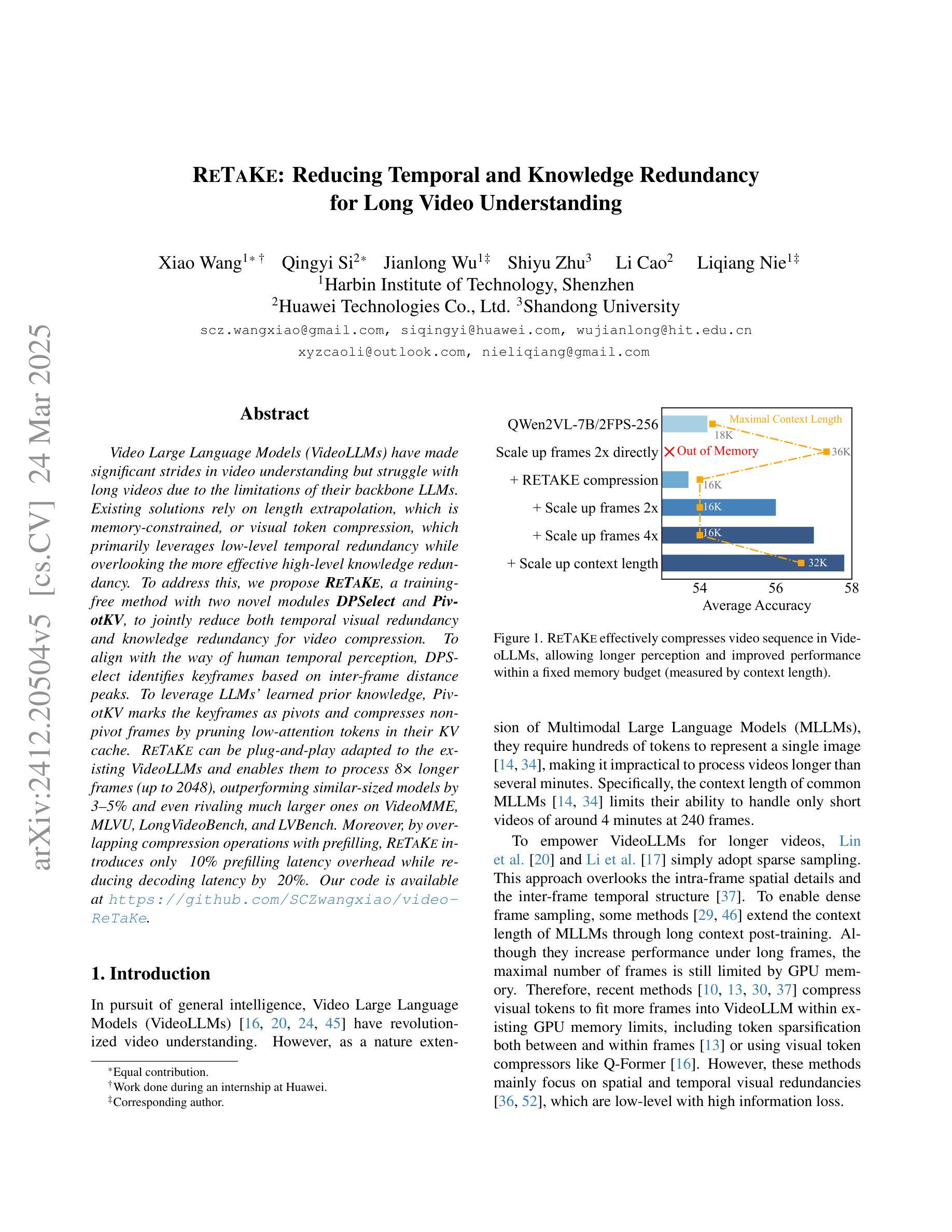
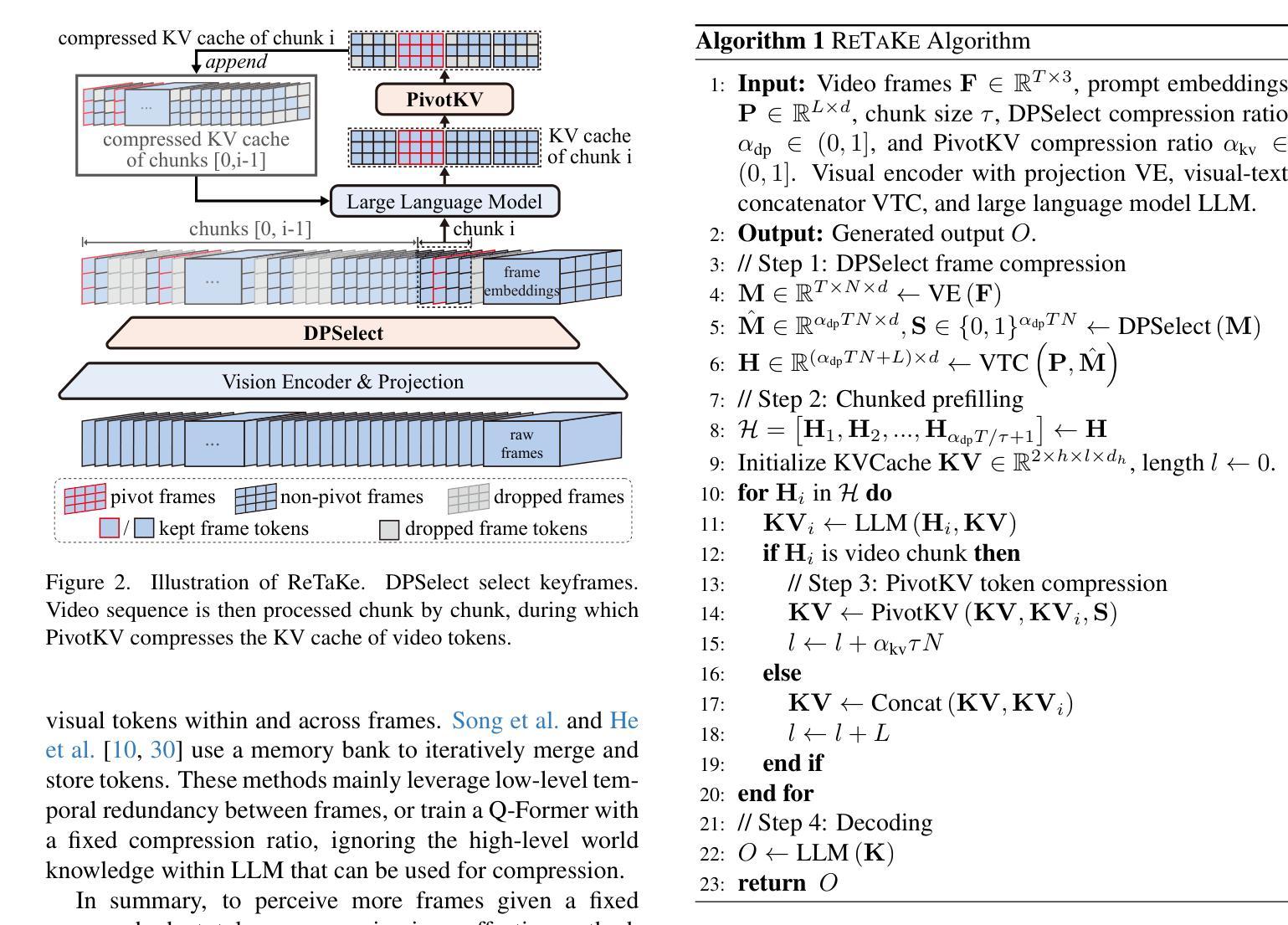
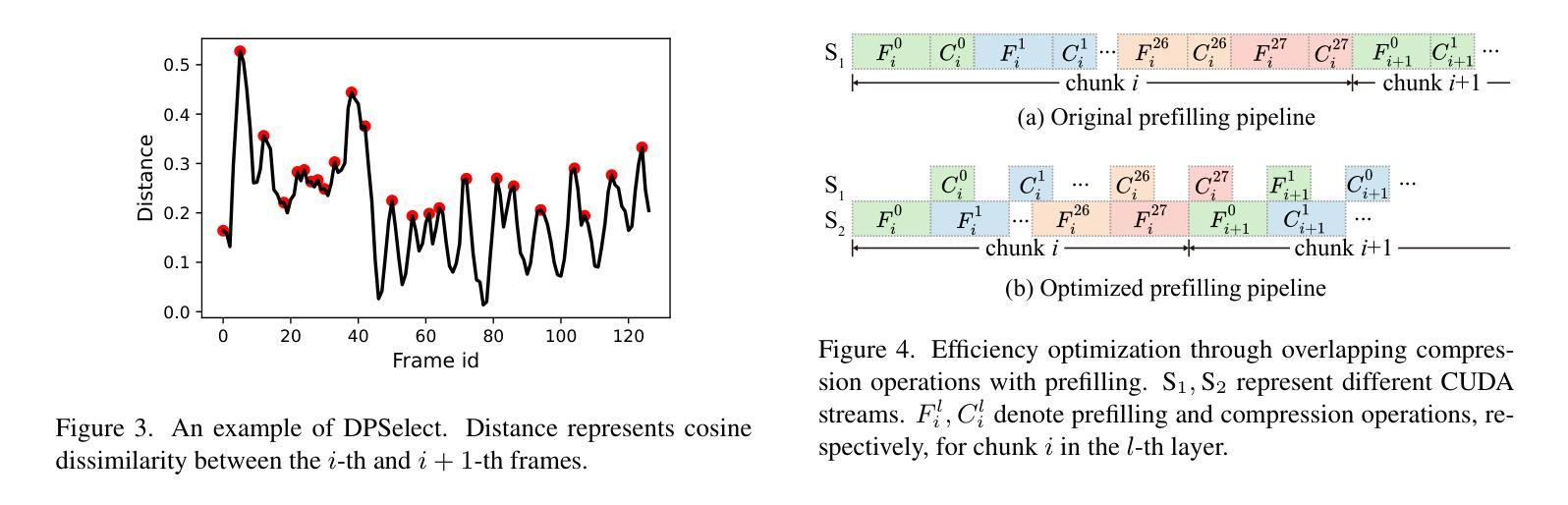
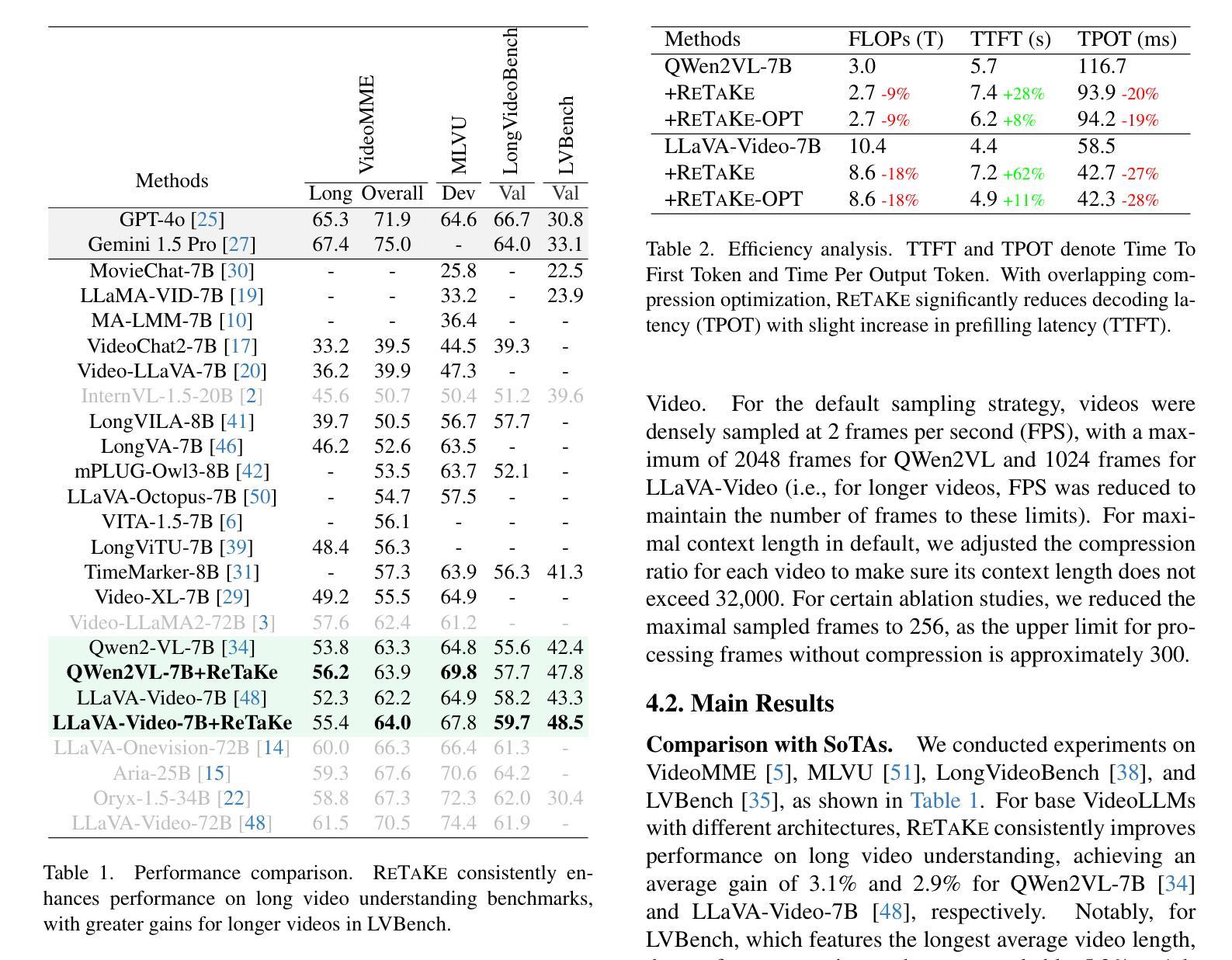
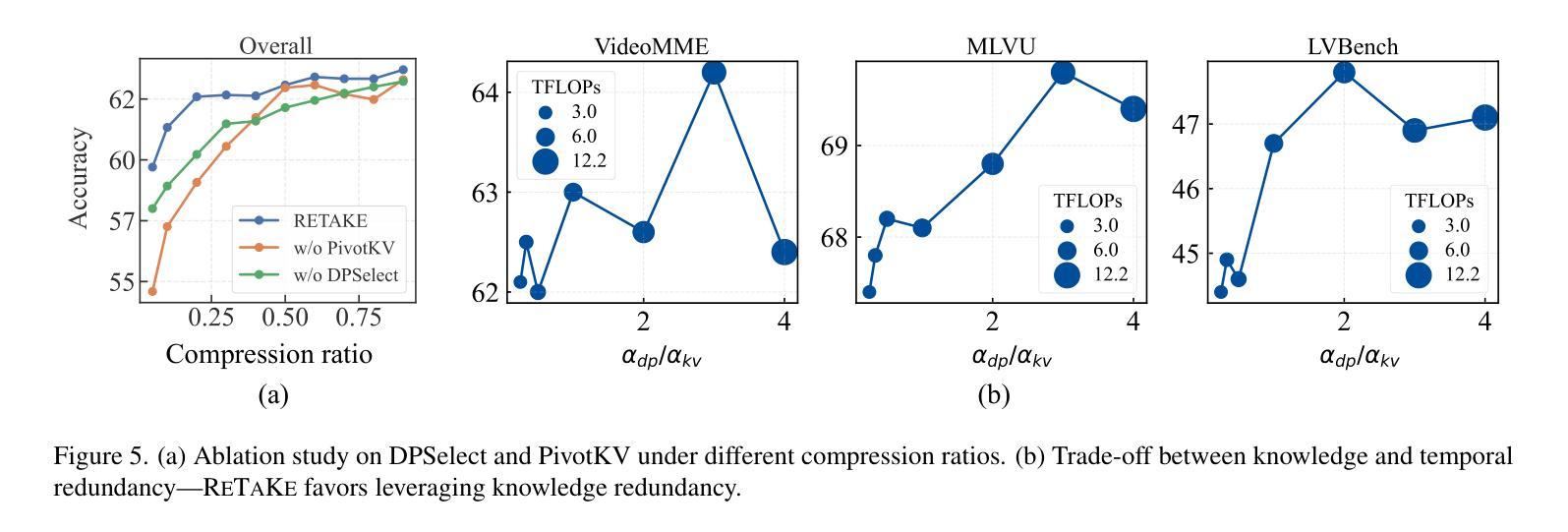

Towards Universal Soccer Video Understanding
Authors:Jiayuan Rao, Haoning Wu, Hao Jiang, Ya Zhang, Yanfeng Wang, Weidi Xie
As a globally celebrated sport, soccer has attracted widespread interest from fans all over the world. This paper aims to develop a comprehensive multi-modal framework for soccer video understanding. Specifically, we make the following contributions in this paper: (i) we introduce SoccerReplay-1988, the largest multi-modal soccer dataset to date, featuring videos and detailed annotations from 1,988 complete matches, with an automated annotation pipeline; (ii) we present an advanced soccer-specific visual encoder, MatchVision, which leverages spatiotemporal information across soccer videos and excels in various downstream tasks; (iii) we conduct extensive experiments and ablation studies on event classification, commentary generation, and multi-view foul recognition. MatchVision demonstrates state-of-the-art performance on all of them, substantially outperforming existing models, which highlights the superiority of our proposed data and model. We believe that this work will offer a standard paradigm for sports understanding research.
作为一项全球盛行的运动,足球吸引了世界各地球迷的广泛关注。本文旨在开发一个用于足球视频理解的全面多模态框架。具体来说,本文做出了以下贡献:(i)我们介绍了SoccerReplay-1988,这是迄今为止最大的多模态足球数据集,包含1988场完整比赛的视频和详细注释,采用自动化注释管道;(ii)我们提出了一种先进的足球特定视觉编码器MatchVision,它利用足球视频中的时空信息,在各种下游任务中表现出色;(iii)我们在事件分类、评论生成和多视角犯规识别等方面进行了大量实验和消融研究。MatchVision在所有任务上均表现出卓越的性能,显著优于现有模型,这凸显了我们提出的数据和模型的优越性。我们相信,这项工作将为体育理解研究提供标准范式。
论文及项目相关链接
PDF CVPR 2025; Project Page: https://jyrao.github.io/UniSoccer/
Summary
该论文旨在开发一个用于足球视频理解的全面多模态框架。其贡献包括:引入迄今为止最大的多模态足球数据集SoccerReplay-1988,包含1988场完整比赛的视频和详细注释;提出先进的足球特定视觉编码器MatchVision,能利用足球视频中的时空信息,并在各种下游任务中表现出卓越性能;进行了关于事件分类、评论生成和多视角犯规识别的大量实验和消融研究,MatchVision在所有这些任务上都展示了最先进的性能。
Key Takeaways
- 引入了一个大规模的多模态足球数据集SoccerReplay-1988,包含自动化注释管道的视频和详细注释。
- 提出了一种先进的足球特定视觉编码器MatchVision,利用时空信息,并在多种下游任务中表现优秀。
- 通过广泛实验和消融研究验证了MatchVision在各种任务上的优越性。
- MatchVision在事件分类、评论生成和多视角犯规识别方面表现出卓越性能。
- 该工作提供了对体育理解研究的新视角和方法论基础。
- 该论文提出的多模态框架可为未来的体育视频分析提供有力支持。
点此查看论文截图
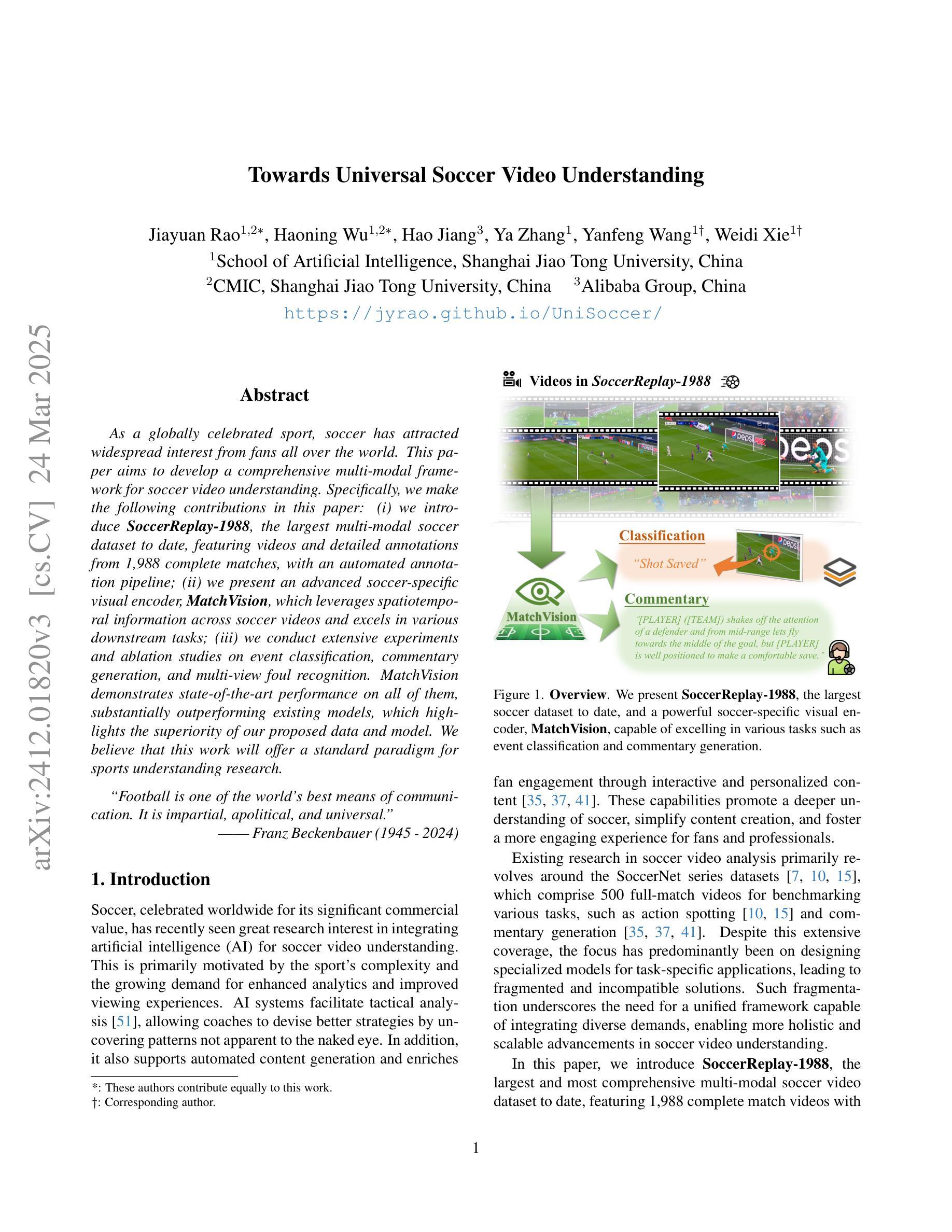
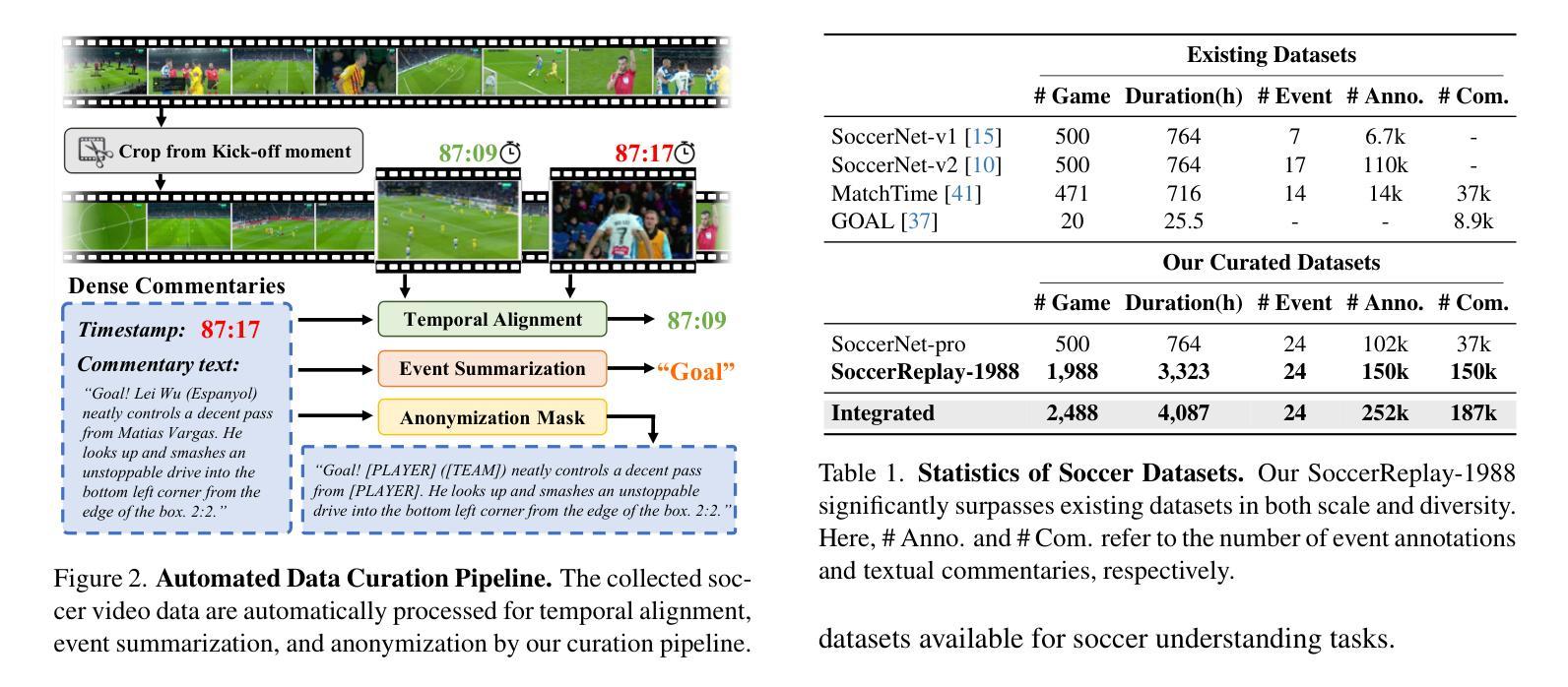
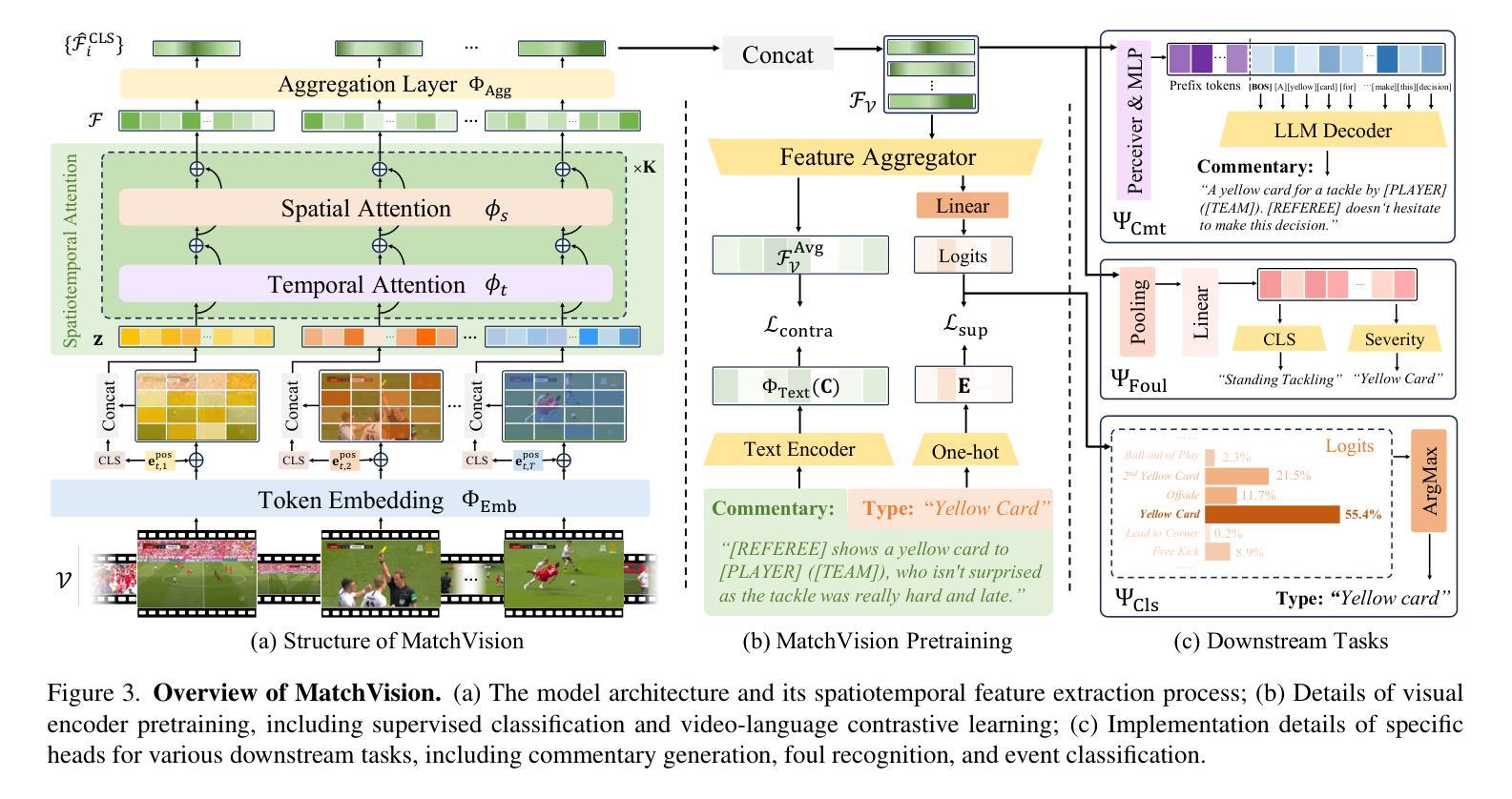
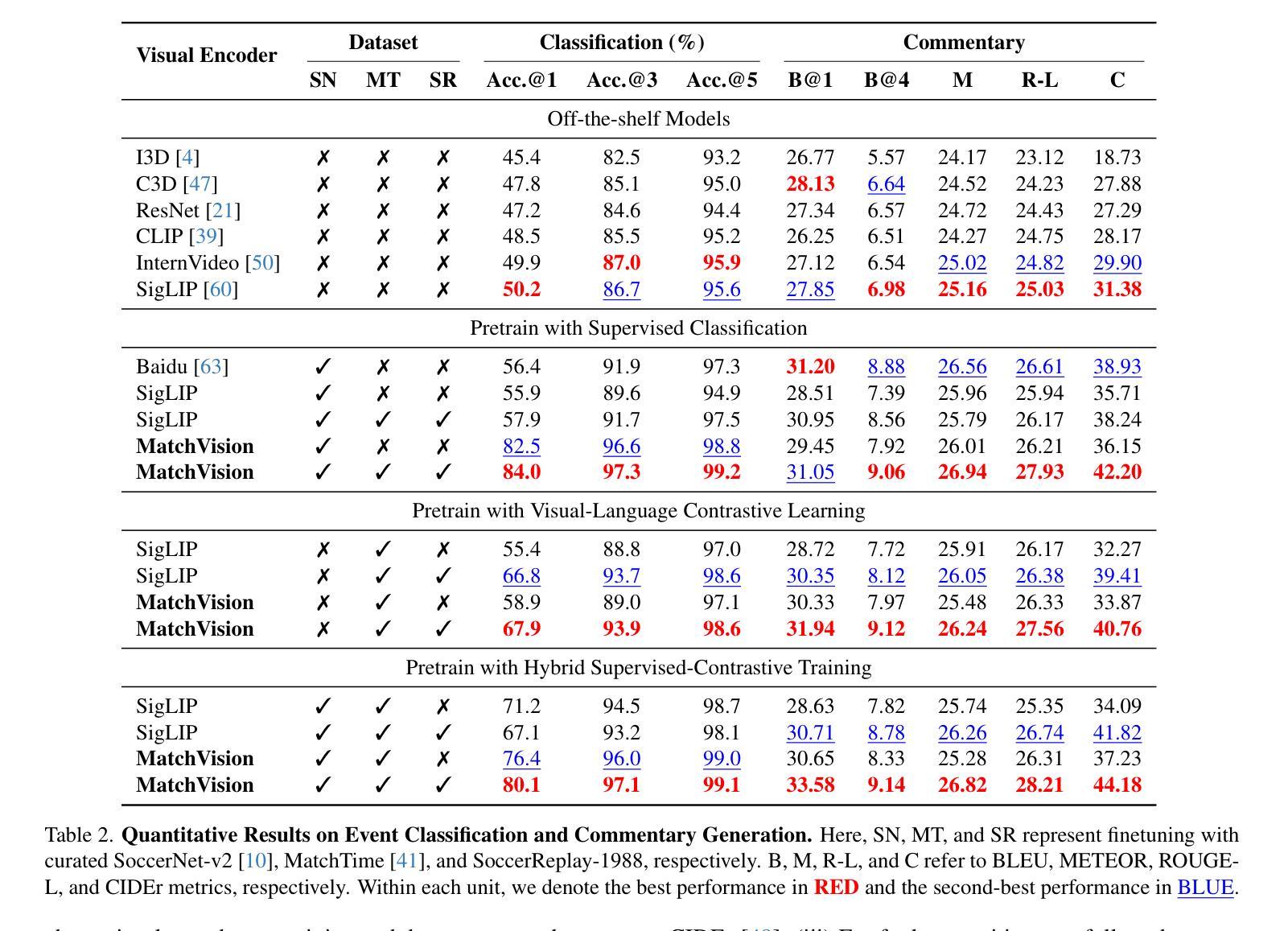
Beyond Training: Dynamic Token Merging for Zero-Shot Video Understanding
Authors:Yiming Zhang, Zhuokai Zhao, Zhaorun Chen, Zenghui Ding, Xianjun Yang, Yining Sun
Recent advancements in multimodal large language models (MLLMs) have opened new avenues for video understanding. However, achieving high fidelity in zero-shot video tasks remains challenging. Traditional video processing methods rely heavily on fine-tuning to capture nuanced spatial-temporal details, which incurs significant data and computation costs. In contrast, training-free approaches, though efficient, often lack robustness in preserving context-rich features across complex video content. To this end, we propose DYTO, a novel dynamic token merging framework for zero-shot video understanding that adaptively optimizes token efficiency while preserving crucial scene details. DYTO integrates a hierarchical frame selection and a bipartite token merging strategy to dynamically cluster key frames and selectively compress token sequences, striking a balance between computational efficiency with semantic richness. Extensive experiments across multiple benchmarks demonstrate the effectiveness of DYTO, achieving superior performance compared to both fine-tuned and training-free methods and setting a new state-of-the-art for zero-shot video understanding.
最近的多模态大型语言模型(MLLMs)的进步为视频理解开辟了新的途径。然而,在零样本视频任务中实现高保真仍然具有挑战性。传统视频处理方法严重依赖于微调来捕捉细微的空间时间细节,这产生了大量的数据和计算成本。相比之下,无训练的方法虽然效率高,但在保留复杂视频内容的丰富上下文特征方面往往缺乏稳健性。为此,我们提出了DYTO,这是一种用于零样本视频理解的新型动态令牌合并框架,它自适应地优化令牌效率,同时保留关键的场景细节。DYTO结合了分层帧选择和二分令牌合并策略,以动态地聚类关键帧并选择性压缩令牌序列,在计算效率和语义丰富性之间取得平衡。在多个基准测试上的广泛实验证明了DYTO的有效性,与经过精细调整和无训练的方法相比取得了优越的性能,为零样本视频理解创造了新的技术水平。
论文及项目相关链接
PDF Code is available at https://github.com/Jam1ezhang/DYTO
Summary
多媒体模态大型语言模型(MLLMs)的最新进展为视频理解开辟了新途径,但仍存在零样本视频任务中保真度不高的问题。传统视频处理方法依赖于微调以捕捉细微的时空细节,这带来了大量的数据和计算成本。本文提出了一种新型的动态令牌合并框架DYTO,它能在保持关键场景细节的同时自适应地优化令牌效率,以实现零样本视频理解。DYTO结合了分层帧选择和二分令牌合并策略,以动态聚类关键帧和选择性地压缩令牌序列,在计算效率和语义丰富性之间取得了平衡。实验证明,DYTO在多个基准测试上表现出卓越的性能,相较于微调方法和无训练方法都有显著优势,为零样本视频理解领域树立了新的里程碑。
Key Takeaways
- 多模态大型语言模型(MLLMs)的进展推动了视频理解的新方向。
- 零样本视频任务中的保真度问题仍是挑战。
- 传统视频处理方法依赖微调,导致高数据计算成本。
- DYTO框架实现了零样本视频理解中的动态令牌管理。
- DYTO结合了分层帧选择和二分令牌合并策略。
- DYTO在多个基准测试上表现出卓越性能,优于现有方法。
点此查看论文截图
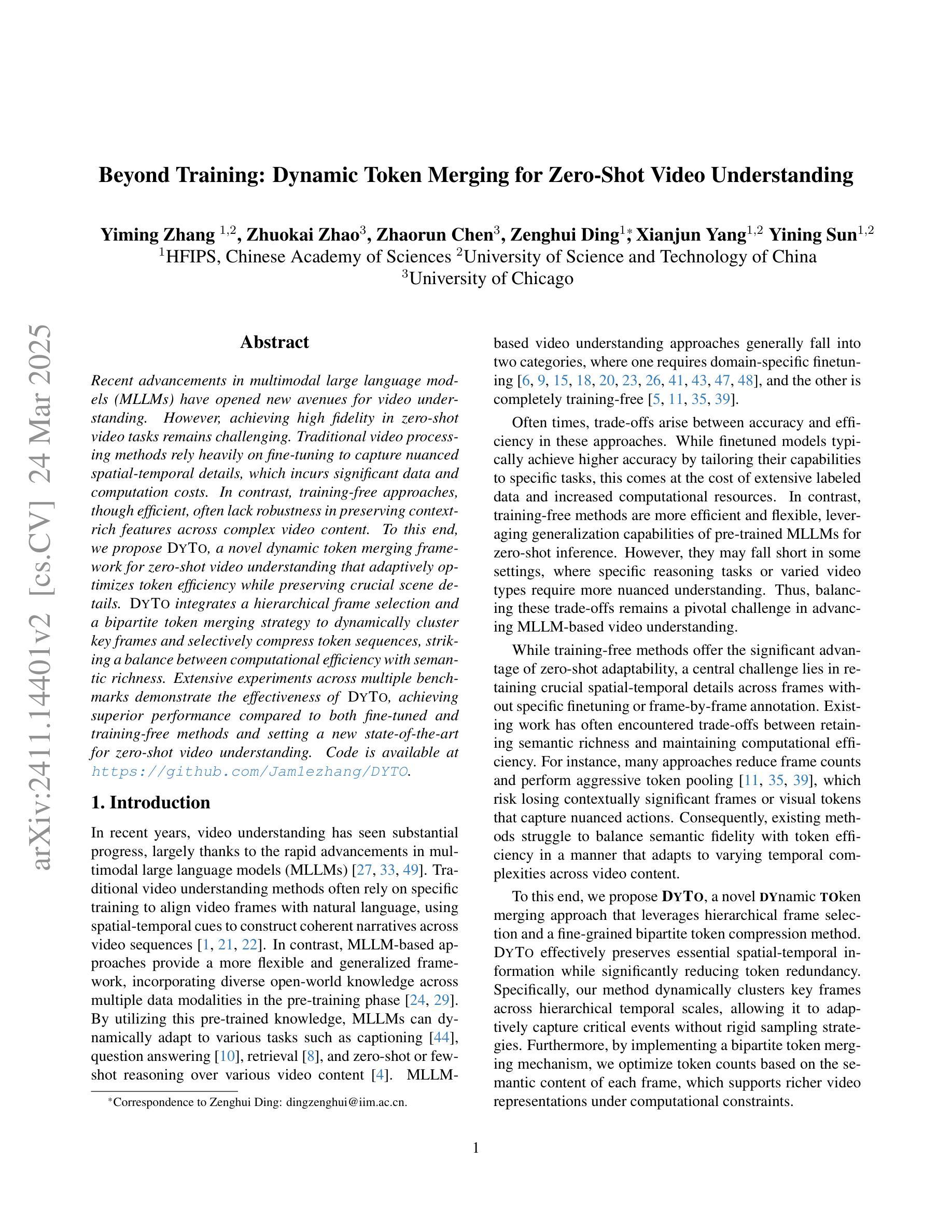
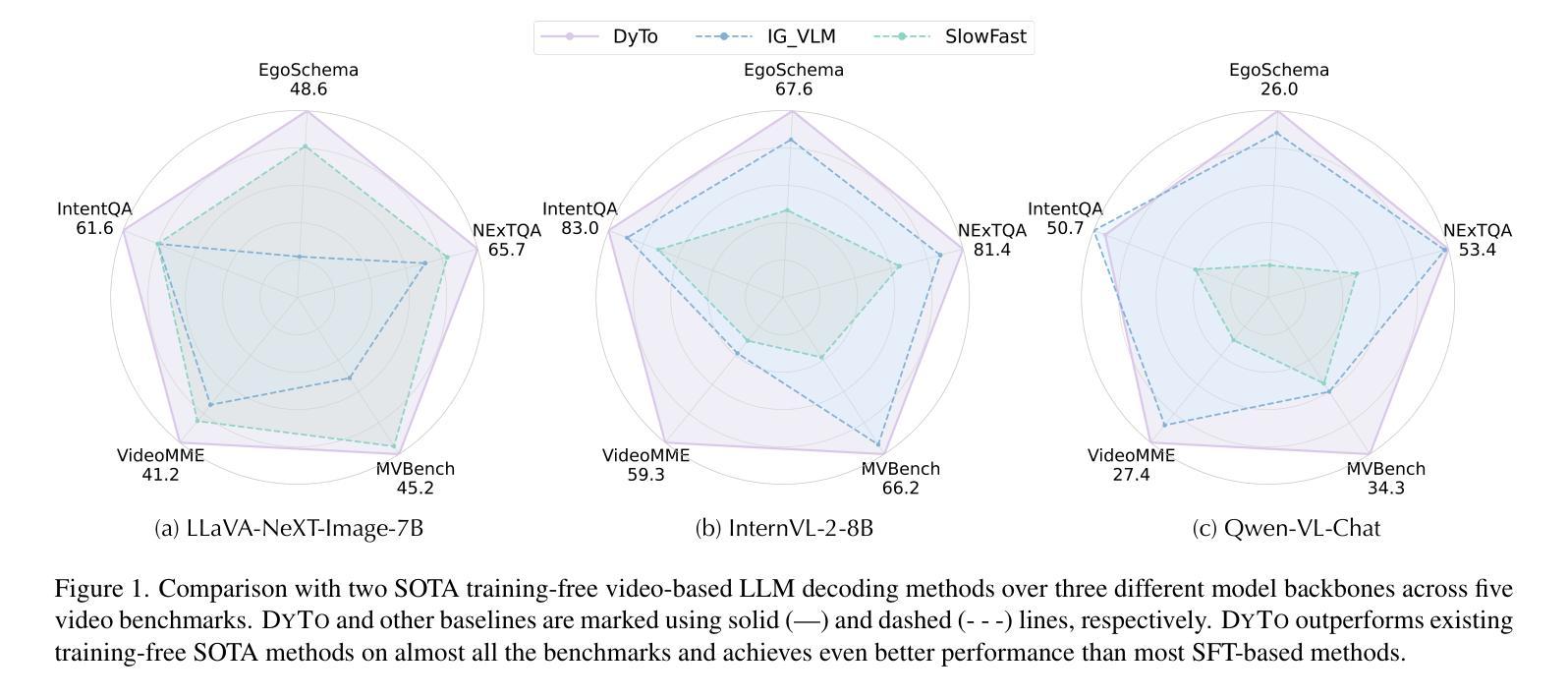
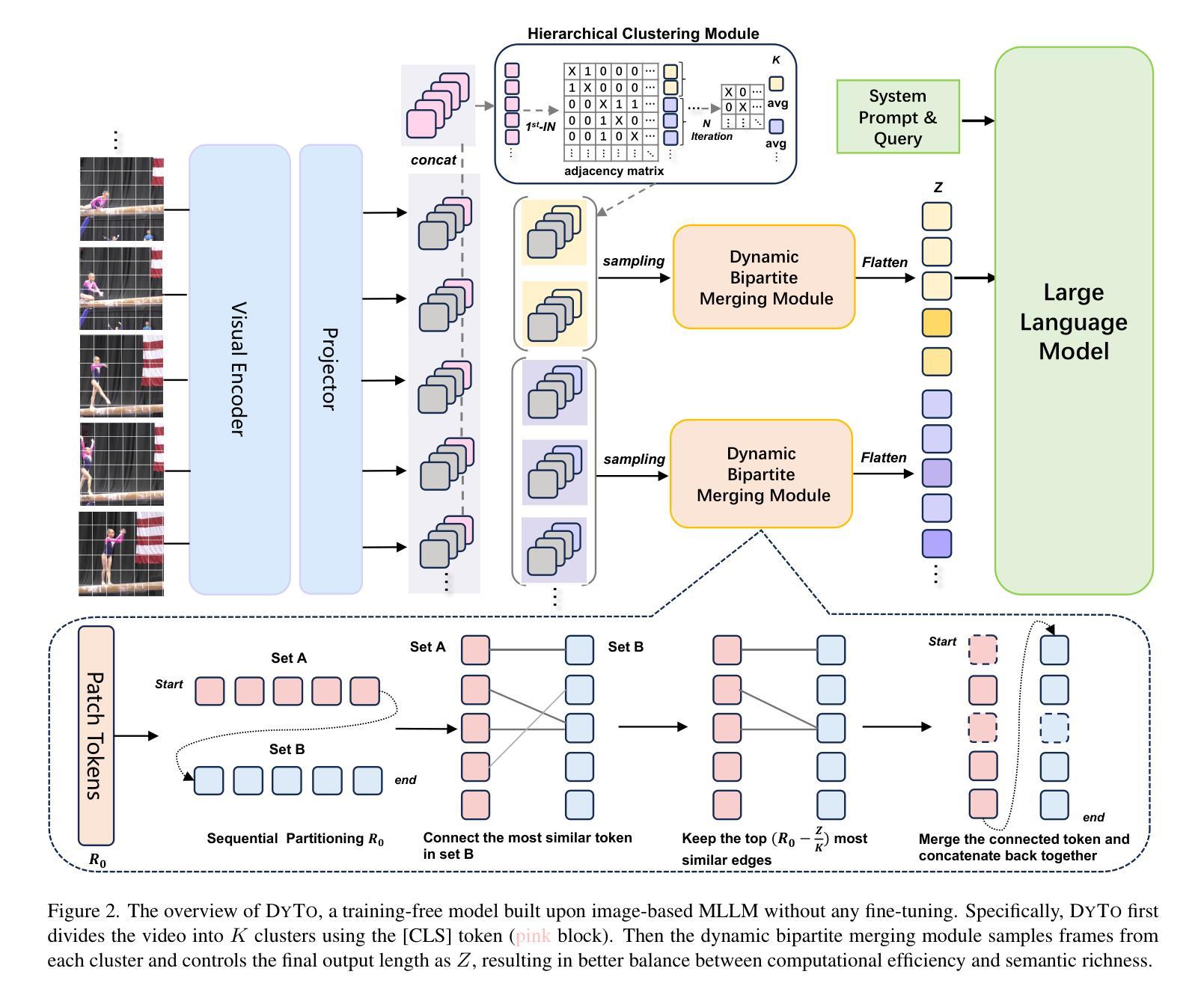
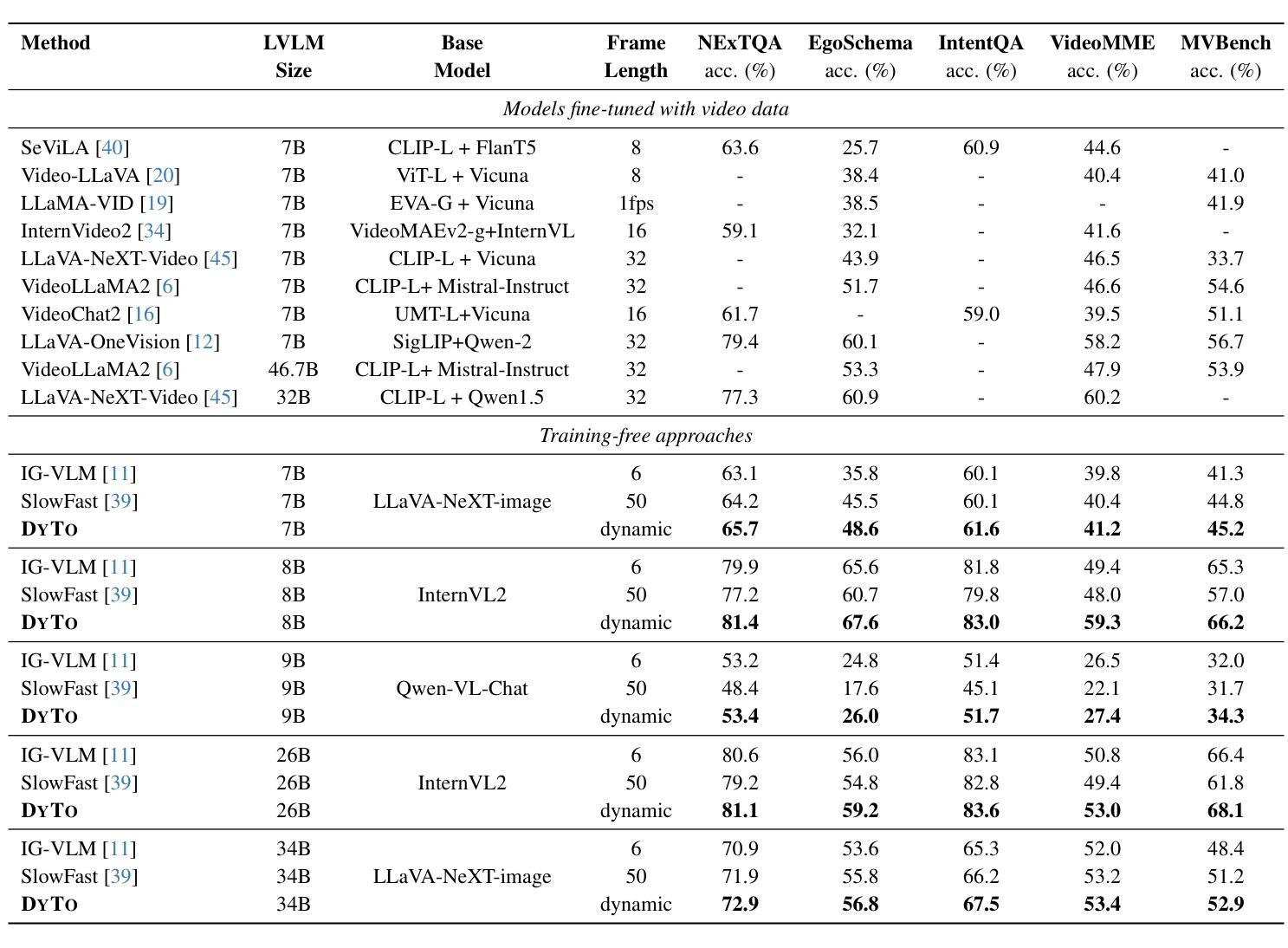
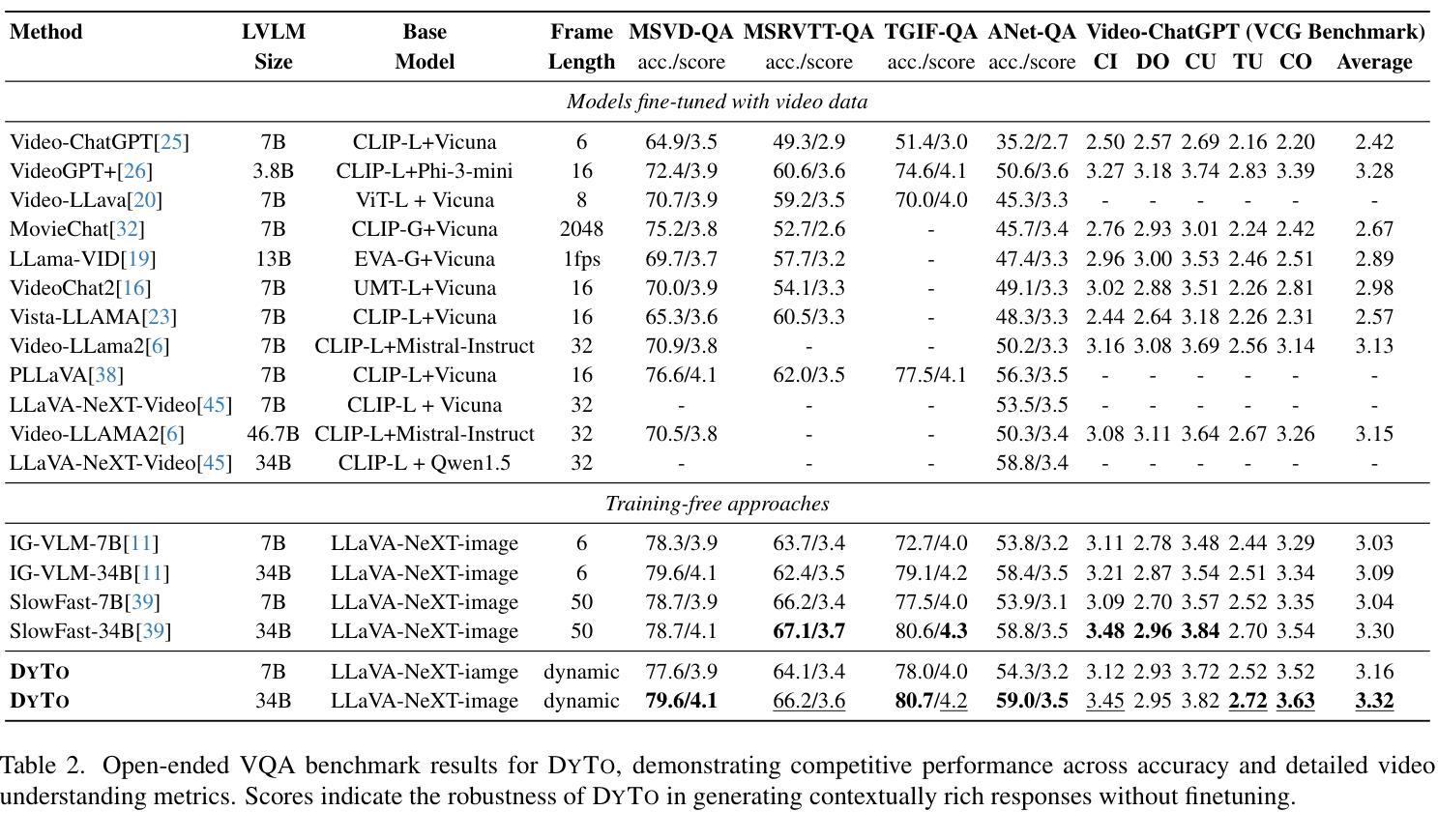
Principles of Visual Tokens for Efficient Video Understanding
Authors:Xinyue Hao, Gen Li, Shreyank N Gowda, Robert B Fisher, Jonathan Huang, Anurag Arnab, Laura Sevilla-Lara
Video understanding has made huge strides in recent years, relying largely on the power of transformers. As this architecture is notoriously expensive and video data is highly redundant, research into improving efficiency has become particularly relevant. Some creative solutions include token selection and merging. While most methods succeed in reducing the cost of the model and maintaining accuracy, an interesting pattern arises: most methods do not outperform the baseline of randomly discarding tokens. In this paper we take a closer look at this phenomenon and observe 5 principles of the nature of visual tokens. For example, we observe that the value of tokens follows a clear Pareto-distribution where most tokens have remarkably low value, and just a few carry most of the perceptual information. We build on these and further insights to propose a lightweight video model, LITE, that can select a small number of tokens effectively, outperforming state-of-the-art and existing baselines across datasets (Kinetics-400 and Something-Something-V2) in the challenging trade-off of computation (GFLOPs) vs accuracy. Experiments also show that LITE generalizes across datasets and even other tasks without the need for retraining.
视频理解近年来取得了巨大进展,这主要得益于变压器的强大功能。由于这种架构非常昂贵且视频数据高度冗余,因此研究提高效率变得尤为重要。一些创意解决方案包括令牌选择和合并。虽然大多数方法在降低模型成本的同时保持了准确性,但出现了一个有趣的现象:大多数方法并不优于随机丢弃令牌的基本线。在本文中,我们仔细观察了这一现象,并观察了视觉令牌性质的5条原则。例如,我们发现令牌的值遵循清晰的帕累托分布,其中大多数令牌的价值非常低,只有少数令牌携带大部分感知信息。我们基于这些见解和其他进一步见解,提出了一个轻量级的视频模型LITE,它能够有效地选择少量令牌,在数据集(Kinetics-400和Something-Something-V2)的计算(GFLOPs)与准确性之间的权衡上优于最新技术和现有基线。实验还表明,LITE可以在不需要重新训练的情况下跨数据集甚至其他任务进行推广。
论文及项目相关链接
Summary
视频理解领域近年来取得了巨大进展,主要依赖于变压器架构的威力。由于该架构成本高昂且视频数据高度冗余,提高效率的研究变得尤为重要。本文深入探讨了视频理解中视觉令牌的本质特征,提出了一个轻量级的视频模型LITE,能够有效地选择少量的令牌并优于现有的最佳模型和其他基线模型,能够在计算和准确度之间实现更好的权衡。该模型还能够跨数据集甚至跨任务进行泛化而无需重新训练。
Key Takeaways
- 视频理解领域依赖变压器架构取得显著进展,但该架构成本高昂。
- 视频数据存在高度冗余性。
- 令牌价值遵循帕累托分布,大部分令牌价值较低,只有少数令牌携带大部分感知信息。
- LITE模型是一个轻量级视频模型,能有效选择重要令牌。
- LITE模型在多个数据集上表现优于现有最佳模型和其他基线模型。
- LITE模型在计算和准确度之间实现了良好的权衡。
点此查看论文截图
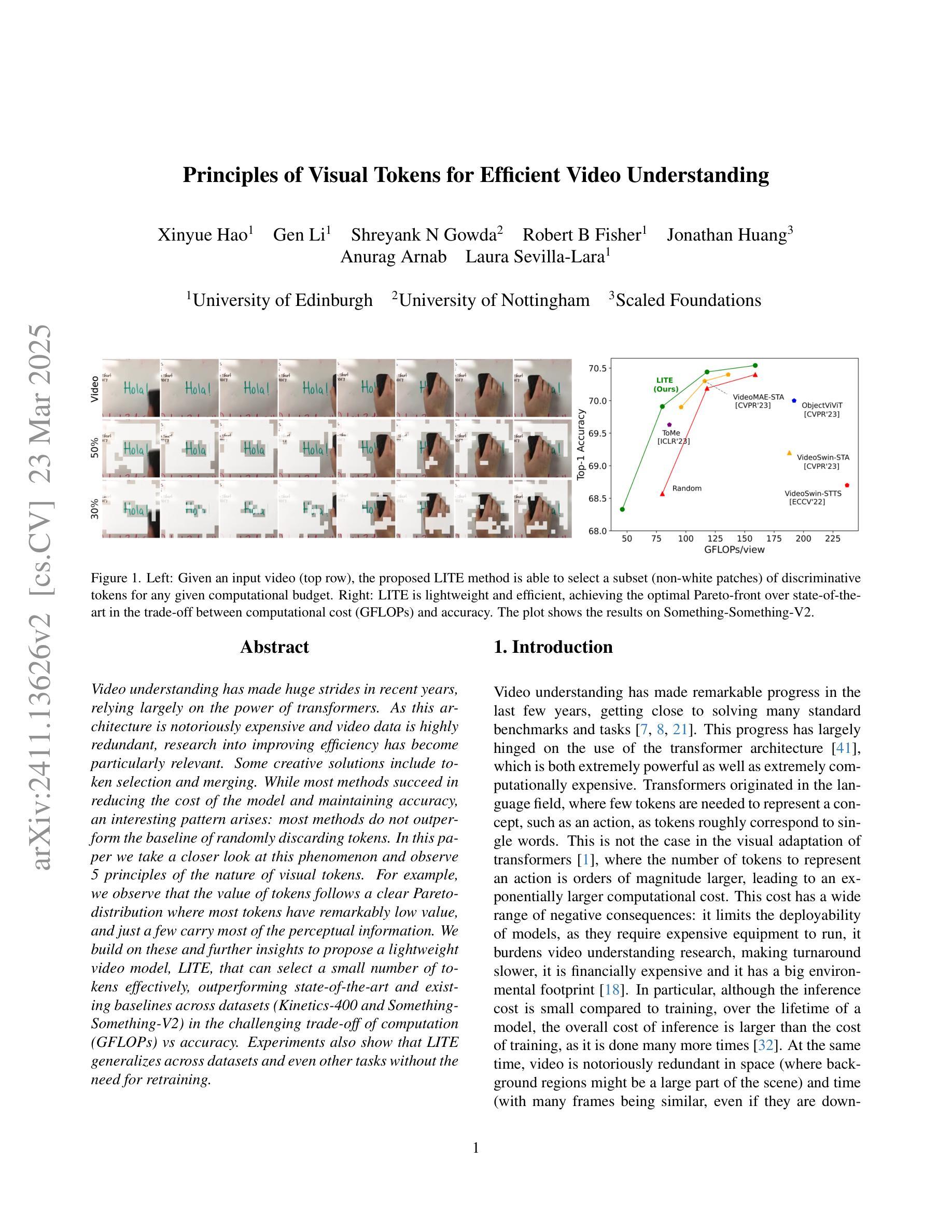
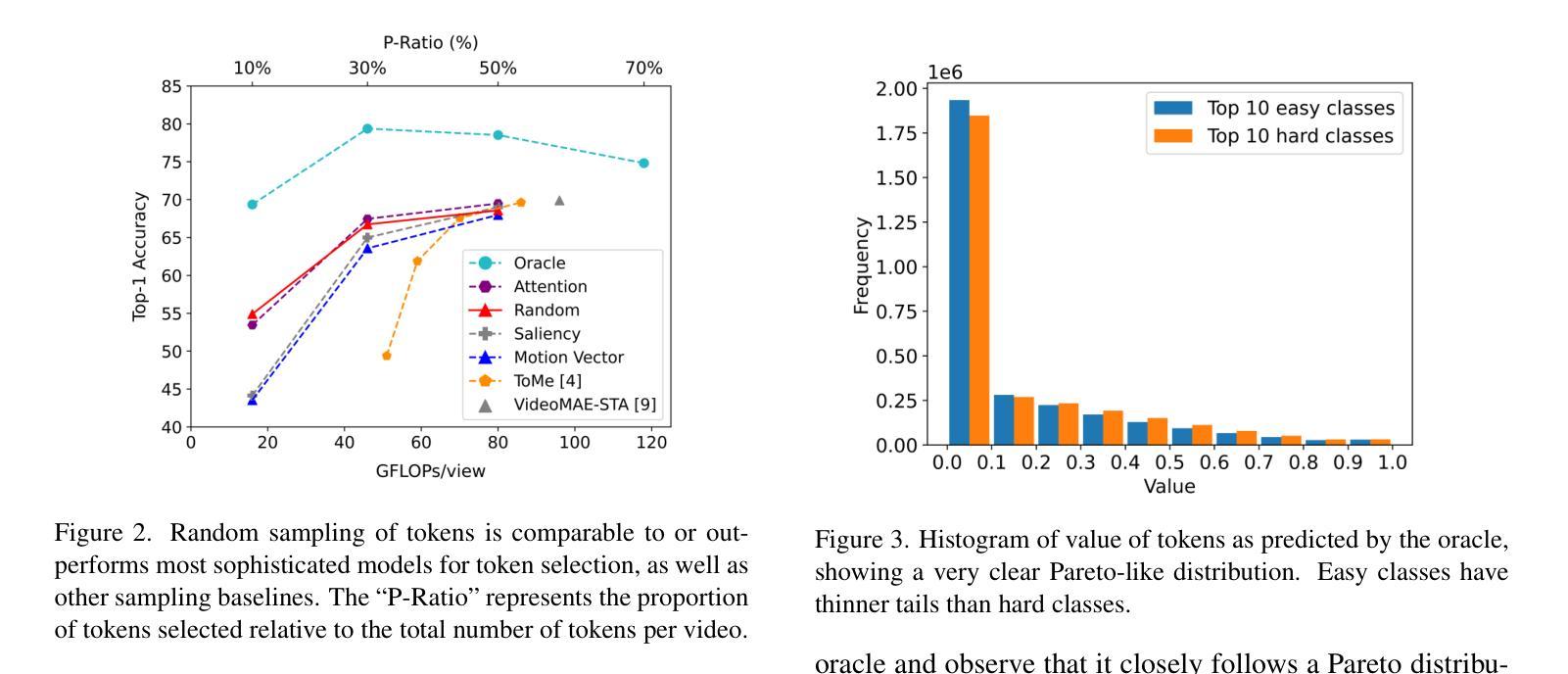
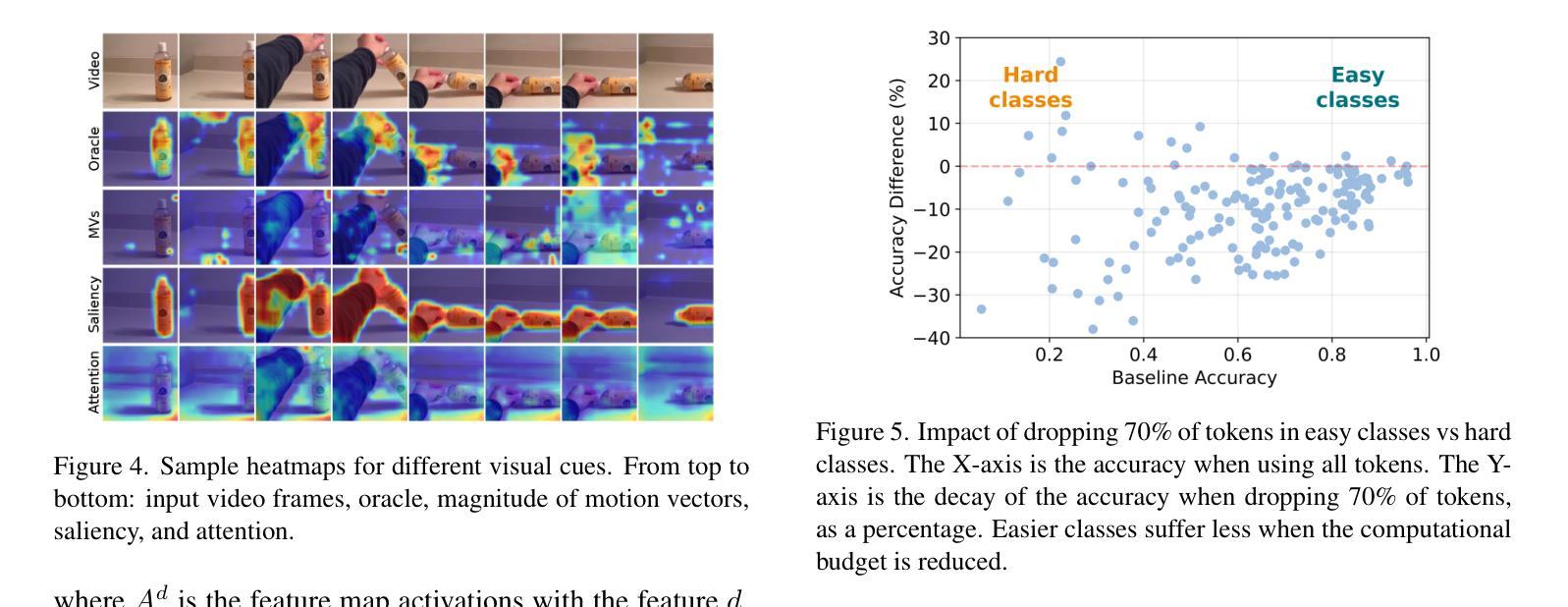
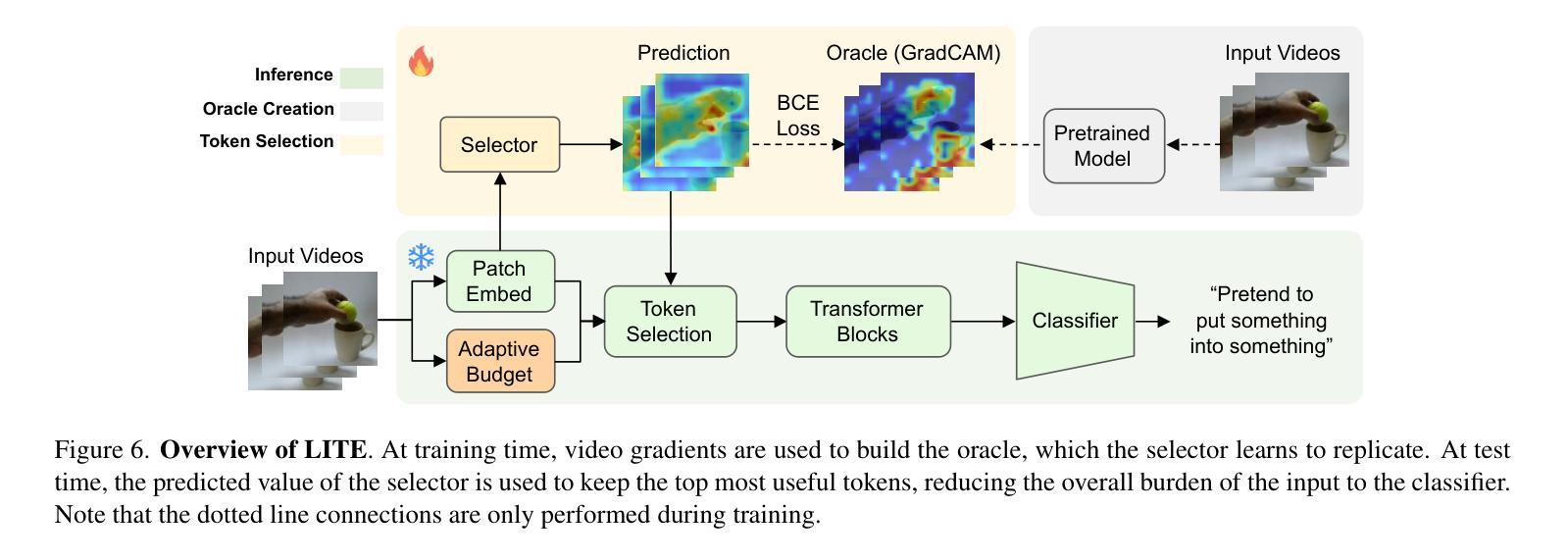
DynFocus: Dynamic Cooperative Network Empowers LLMs with Video Understanding
Authors:Yudong Han, Qingpei Guo, Liyuan Pan, Liu Liu, Yu Guan, Ming Yang
The challenge in LLM-based video understanding lies in preserving visual and semantic information in long videos while maintaining a memory-affordable token count. However, redundancy and correspondence in videos have hindered the performance potential of existing methods. Through statistical learning on current datasets, we observe that redundancy occurs in both repeated and answer-irrelevant frames, and the corresponding frames vary with different questions. This suggests the possibility of adopting dynamic encoding to balance detailed video information preservation with token budget reduction. To this end, we propose a dynamic cooperative network, DynFocus, for memory-efficient video encoding in this paper. Specifically, i) a Dynamic Event Prototype Estimation (DPE) module to dynamically select meaningful frames for question answering; (ii) a Compact Cooperative Encoding (CCE) module that encodes meaningful frames with detailed visual appearance and the remaining frames with sketchy perception separately. We evaluate our method on five publicly available benchmarks, and experimental results consistently demonstrate that our method achieves competitive performance.
基于大型语言模型(LLM)的视频理解挑战在于在长视频中保留视觉和语义信息的同时,保持可承受的令牌计数。然而,视频中的冗余和对应关系阻碍了现有方法的性能潜力。通过对当前数据集进行统计学习,我们发现冗余现象既出现在重复帧中也出现在与答案无关的帧中,并且对应帧会因不同问题而变化。这提示我们有可能采用动态编码来平衡保留详细的视频信息与减少令牌预算。为此,本文提出了一种用于内存高效视频编码的动态协同网络DynFocus。具体来说,一是对动态事件原型估计(DPE)模块进行动态选择有意义的帧用于问答;二是紧凑协同编码(CCE)模块,该模块对有意义的帧进行详细视觉外观编码,对剩余帧进行草图感知编码。我们在五个公开可用的基准测试集上评估了我们的方法,实验结果一致表明,我们的方法具有竞争力。
论文及项目相关链接
PDF Accepted by CVPR 25
摘要
基于LLM的视频理解挑战在于在长视频中保留视觉和语义信息的同时,保持可记忆的标记计数合理。冗余和视频的对应关系阻碍了现有方法的性能潜力。通过对当前数据集的统计学习,我们发现冗余出现在重复和与答案无关的帧中,并且对应的帧因问题的不同而变化。这提示我们可能采用动态编码来平衡保留详细的视频信息和减少标记预算。为此,本文提出了一种用于内存高效视频编码的动态协同网络DynFocus。具体来说,一、动态事件原型估计(DPE)模块,用于动态选择对问答有意义的帧;二、紧凑协同编码(CCE)模块,对有意义的帧进行详细视觉外观编码,对剩余帧进行草图感知编码。我们在五个公开的基准测试集上评估了我们的方法,实验结果表明,我们的方法具有竞争力。
要点
- LLM在视频理解中面临挑战,需要在保留视觉和语义信息的同时控制标记计数。
- 视频冗余现象影响现有方法的性能,包括重复和与答案无关的帧。
- 不同问题对应的帧不同,表明需要动态选择重要帧进行处理。
- 提出DynFocus动态协同网络进行内存高效视频编码。
- DynFocus包含动态事件原型估计(DPE)模块,用于选择重要帧。
- DynFocus还包含紧凑协同编码(CCE)模块,可以对有意义的帧进行详细编码,而对其他帧进行简化编码。
点此查看论文截图
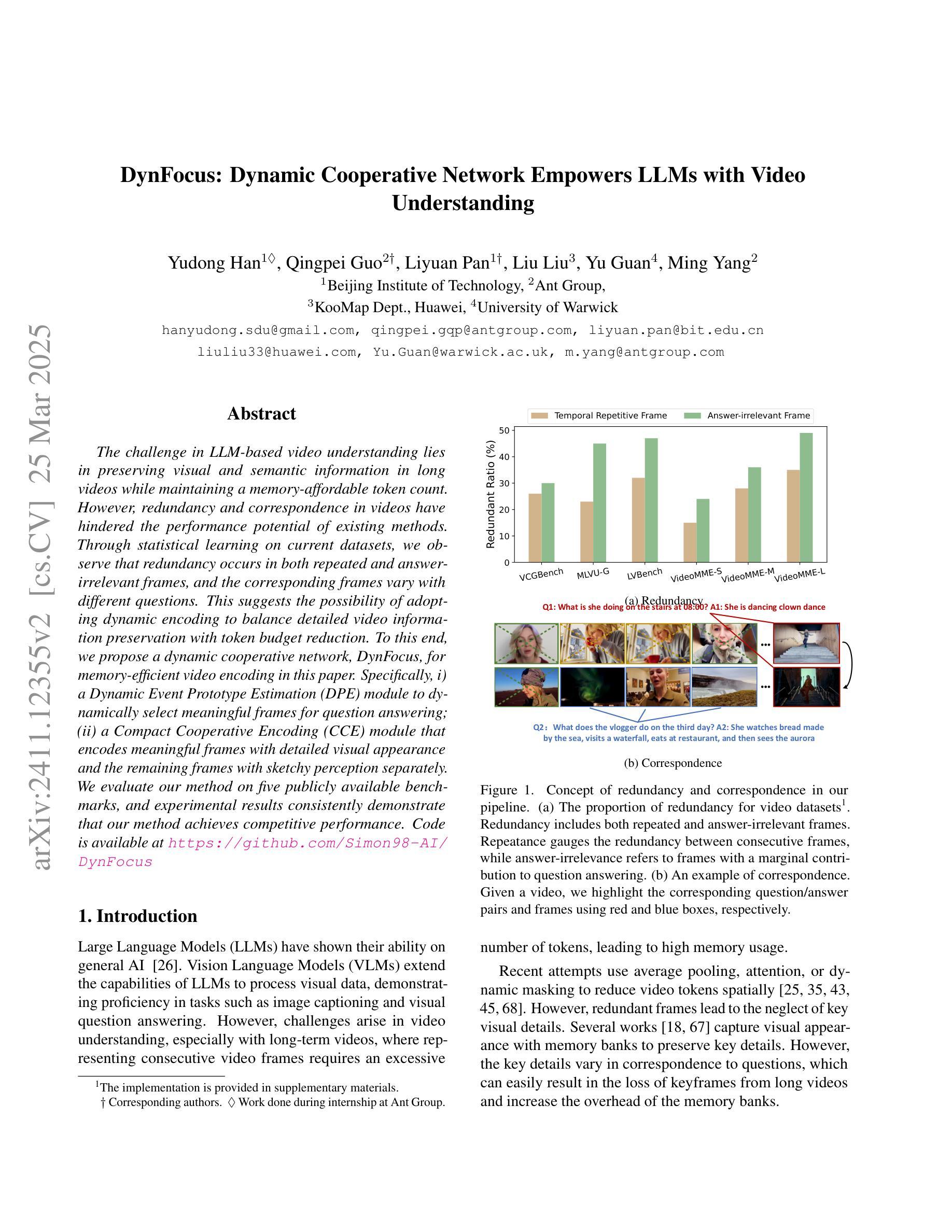
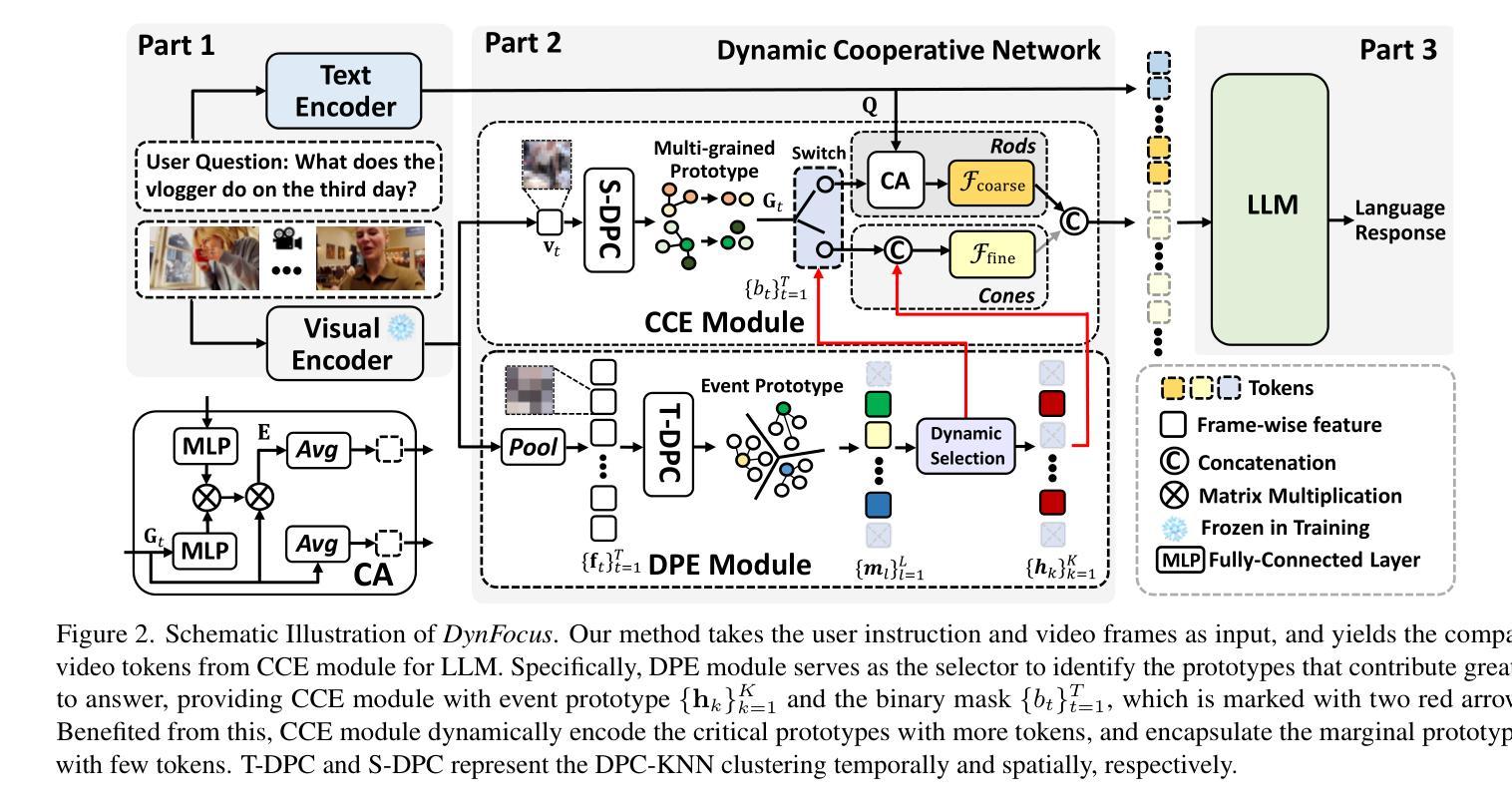
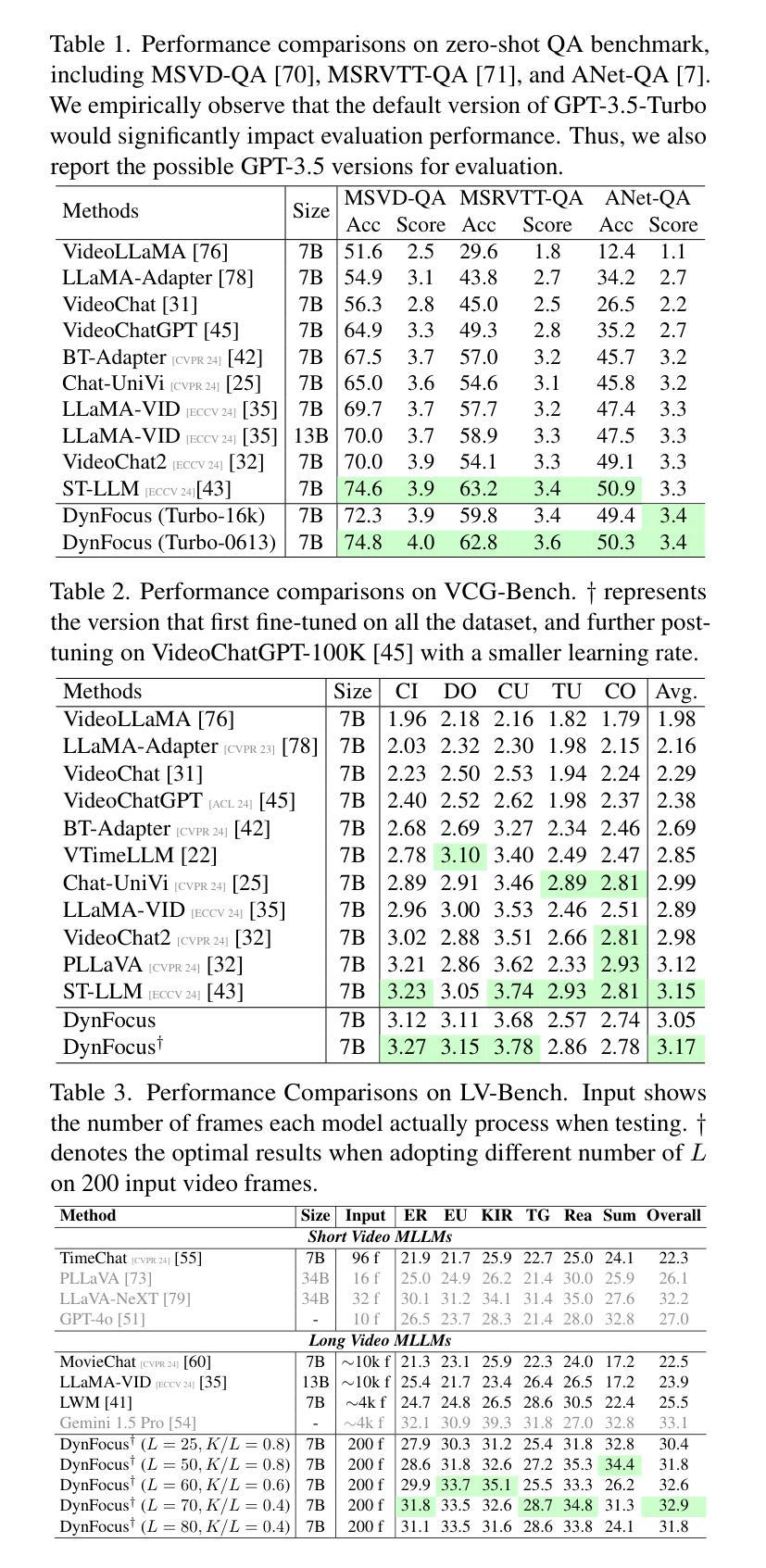
GUI-World: A Video Benchmark and Dataset for Multimodal GUI-oriented Understanding
Authors:Dongping Chen, Yue Huang, Siyuan Wu, Jingyu Tang, Liuyi Chen, Yilin Bai, Zhigang He, Chenlong Wang, Huichi Zhou, Yiqiang Li, Tianshuo Zhou, Yue Yu, Chujie Gao, Qihui Zhang, Yi Gui, Zhen Li, Yao Wan, Pan Zhou, Jianfeng Gao, Lichao Sun
Recently, Multimodal Large Language Models (MLLMs) have been used as agents to control keyboard and mouse inputs by directly perceiving the Graphical User Interface (GUI) and generating corresponding commands. However, current agents primarily demonstrate strong understanding capabilities in static environments and are mainly applied to relatively simple domains, such as Web or mobile interfaces. We argue that a robust GUI agent should be capable of perceiving temporal information on the GUI, including dynamic Web content and multi-step tasks. Additionally, it should possess a comprehensive understanding of various GUI scenarios, including desktop software and multi-window interactions. To this end, this paper introduces a new dataset, termed GUI-World, which features meticulously crafted Human-MLLM annotations, extensively covering six GUI scenarios and eight types of GUI-oriented questions in three formats. We evaluate the capabilities of current state-of-the-art MLLMs, including Image LLMs and Video LLMs, in understanding various types of GUI content, especially dynamic and sequential content. Our findings reveal that current models struggle with dynamic GUI content without manually annotated keyframes or operation history. On the other hand, Video LLMs fall short in all GUI-oriented tasks given the sparse GUI video dataset. Therefore, we take the initial step of leveraging a fine-tuned Video LLM, GUI-Vid, as a GUI-oriented assistant, demonstrating an improved understanding of various GUI tasks. However, due to the limitations in the performance of base LLMs, we conclude that using video LLMs as GUI agents remains a significant challenge. We believe our work provides valuable insights for future research in dynamic GUI content understanding. All the dataset and code are publicly available at: https://gui-world.github.io.
近期,多模态大型语言模型(MLLMs)被用作通过直接感知图形用户界面(GUI)并生成相应命令来控制键盘和鼠标输入的代理。然而,当前的代理主要表现出在静态环境中的强大理解能力,并主要应用于相对简单的领域,如网页或移动界面。我们认为,一个稳健的GUI代理应该能够感知GUI上的时间信息,包括动态Web内容和多步骤任务。此外,它还应全面理解各种GUI场景,包括桌面软件和多窗口交互。为此,本文引入了一个新数据集,名为GUI-World,它具有精心制作的人类-MLLM注释,广泛涵盖了六种GUI场景和三种格式中的八种面向GUI的问题。我们评估了当前最先进的MLLMs,包括图像LLMs和视频LLMs,在理解各种类型的GUI内容,尤其是动态和顺序内容方面的能力。我们的研究发现,当前模型在处理动态GUI内容时,如果没有手动注释的关键帧或操作历史,会遇到困难。另一方面,由于GUI视频数据集较为稀疏,视频LLMs在所有面向GUI的任务中都表现不足。因此,我们采取了利用精细调整的视频LLM——GUI-Vid作为面向GUI的助手的初步措施,展示了对各种GUI任务的改进理解。然而,由于基础LLMs的性能限制,我们使用视频LLMs作为GUI代理仍然存在重大挑战。我们相信我们的工作为未来的动态GUI内容理解研究提供了有价值的见解。所有数据集和代码可在https://gui-world.github.io上公开找到。
论文及项目相关链接
PDF Accepted by ICLR 2025
Summary
基于文本的讨论,本文主要介绍了多模态大型语言模型(MLLMs)作为图形用户界面(GUI)代理的应用情况。当前模型主要理解静态环境,并应用于简单的领域如网页或移动界面。作者主张一个强大的GUI代理应该能够感知GUI上的时间信息,包括动态网页内容和多步骤任务,并全面理解各种GUI场景。为此,引入了新的数据集GUI-World,用于评估当前先进的MLLMs(包括图像LLMs和视频LLMs)理解GUI内容的能力。研究发现,当前模型在处理动态GUI内容时面临挑战,而视频LLMs在所有GUI导向的任务中都表现不佳。作者尝试使用精细调整的视频LLM(GUI-Vid)作为GUI导向的助理,但仍然存在挑战。
Key Takeaways
- 当前的多模态大型语言模型(MLLMs)主要用作图形用户界面(GUI)代理,主要理解静态环境并应用于简单领域。
- 一个强大的GUI代理应能感知GUI上的时间信息,包括动态内容和多步骤任务。
- 引入的新数据集GUI-World旨在评估LLMs在理解各种GUI内容方面的能力,特别是动态和顺序内容。
- 当前模型在处理动态GUI内容时遇到困难,需要手动注释的关键帧或操作历史。
- 视频LLMs在所有GUI导向的任务中都表现不佳,尽管尝试使用精细调整的视频LLM(GUI-Vid)作为助理,但仍存在挑战。
- 使用视频LLMs作为GUI代理仍然是一个重大挑战,这显示了该领域未来研究的价值。
点此查看论文截图
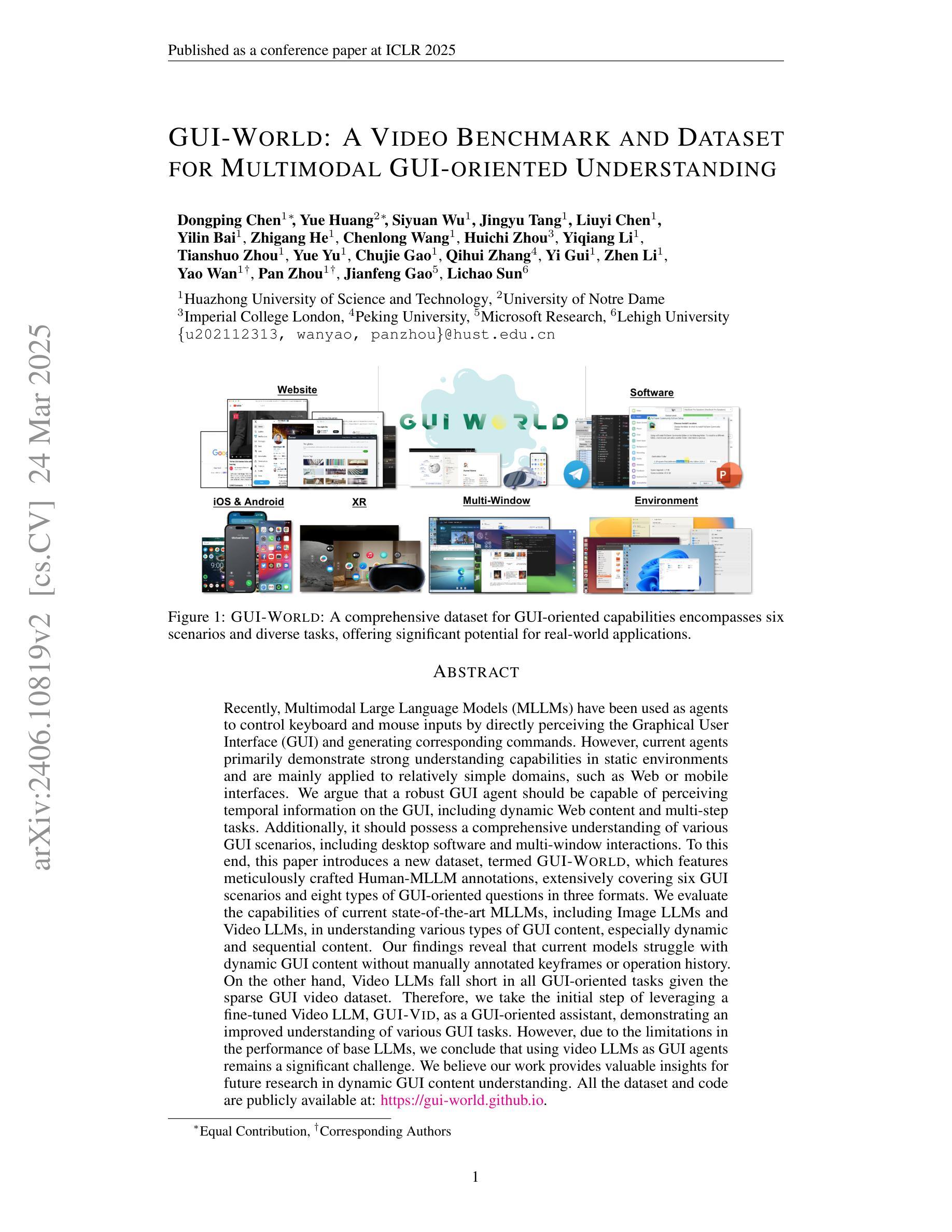
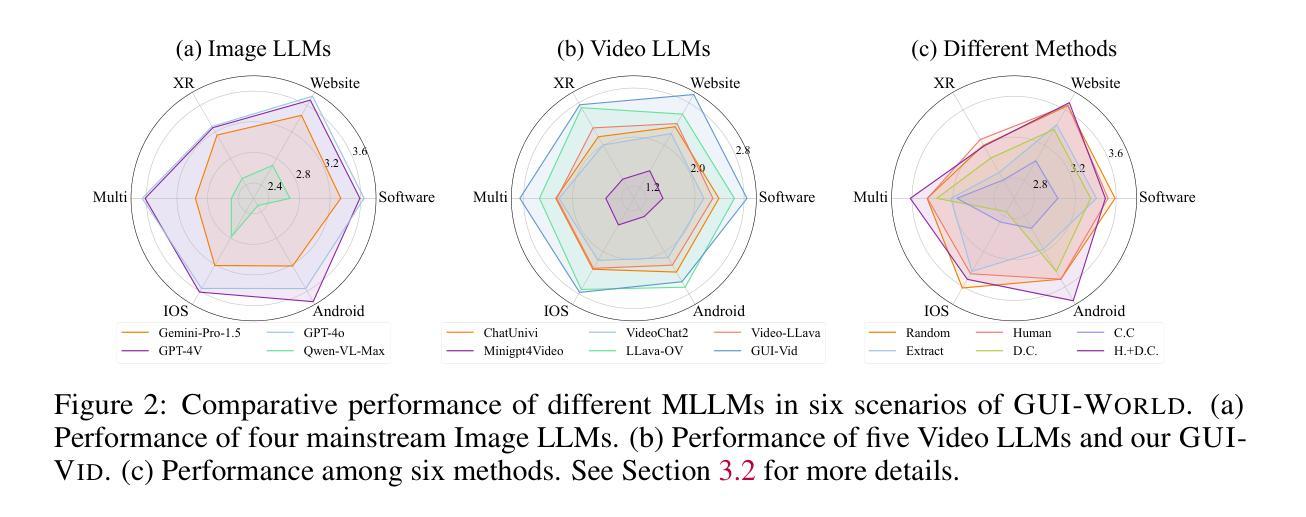
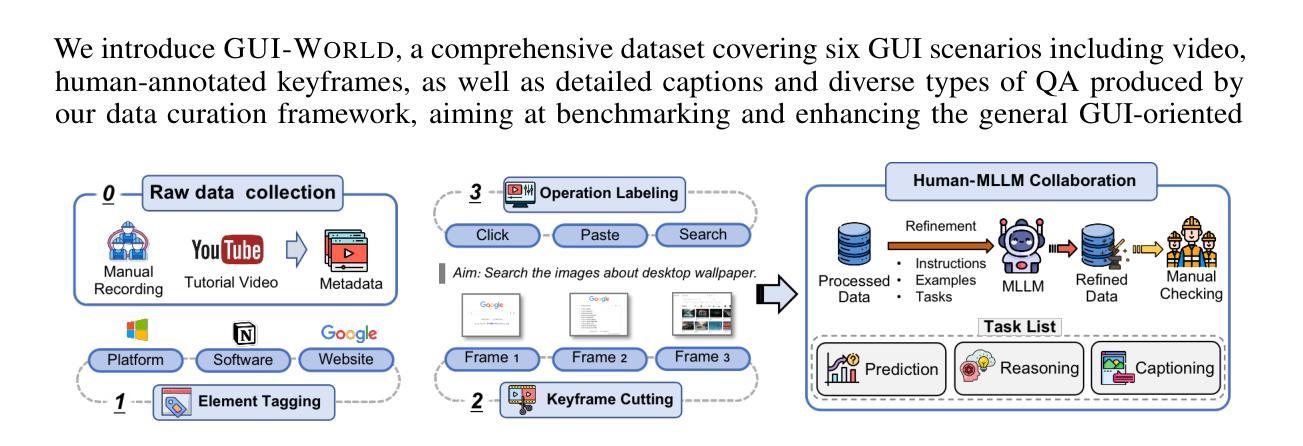
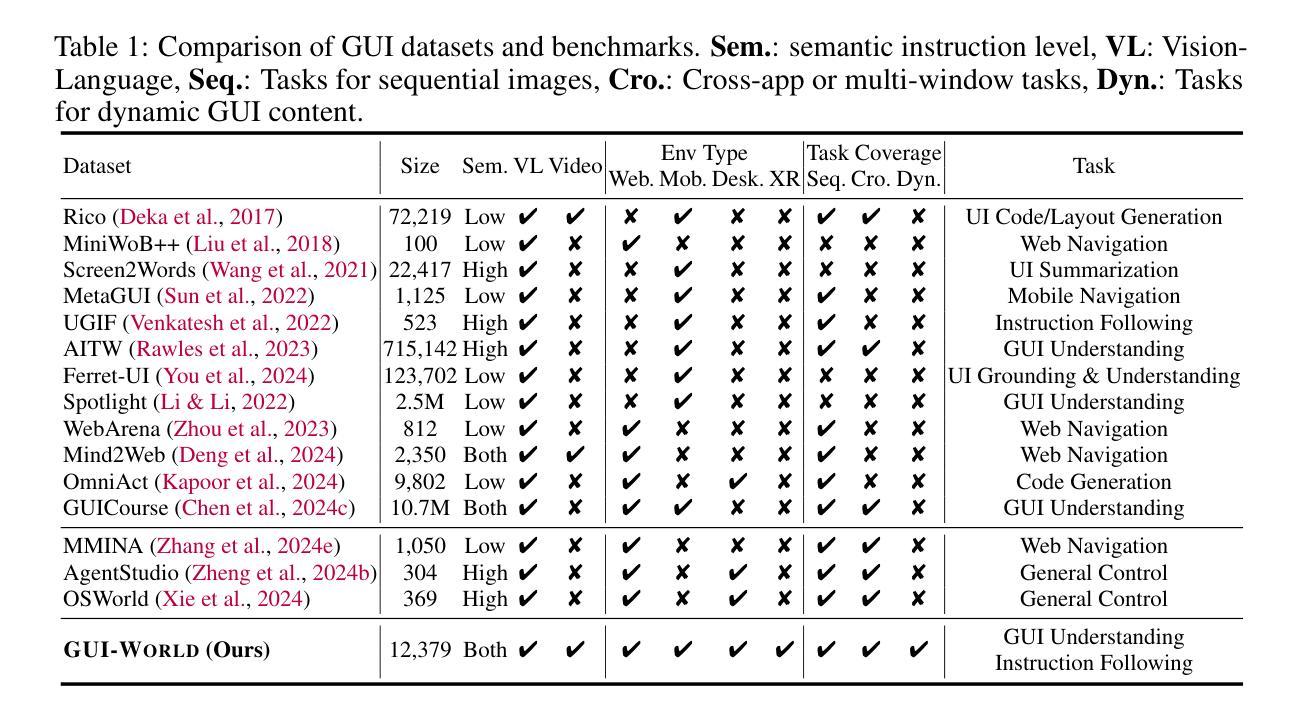
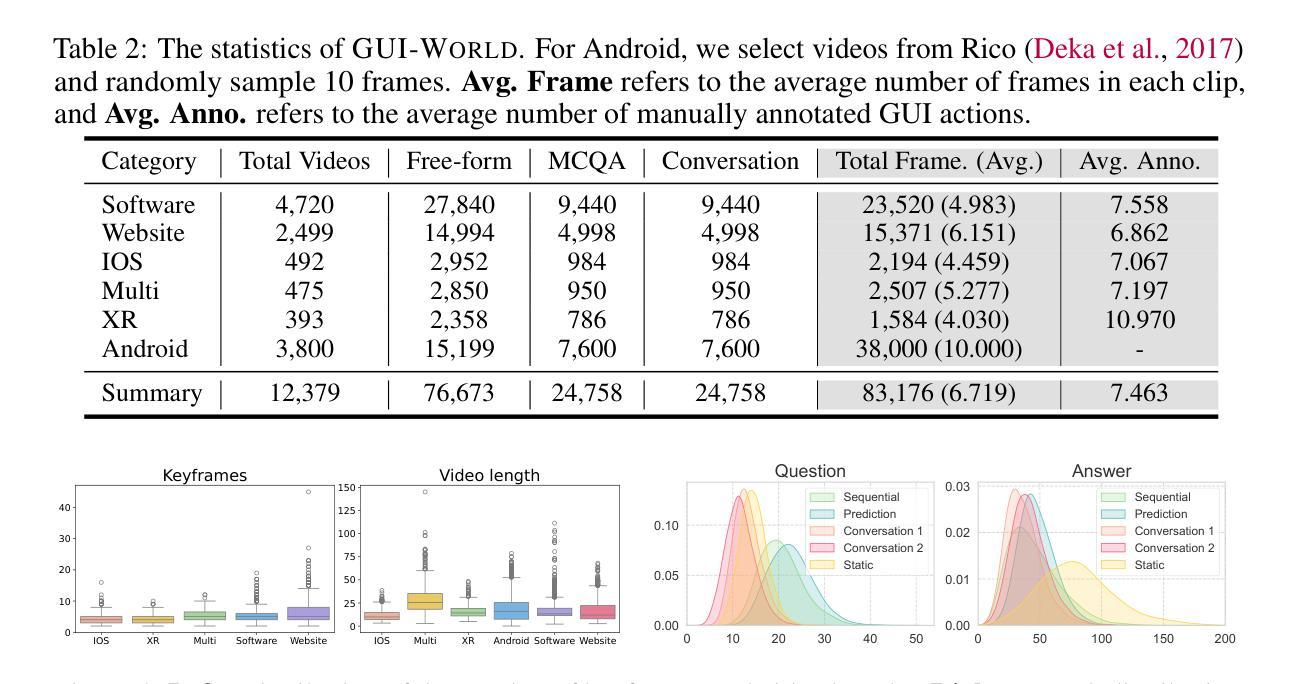
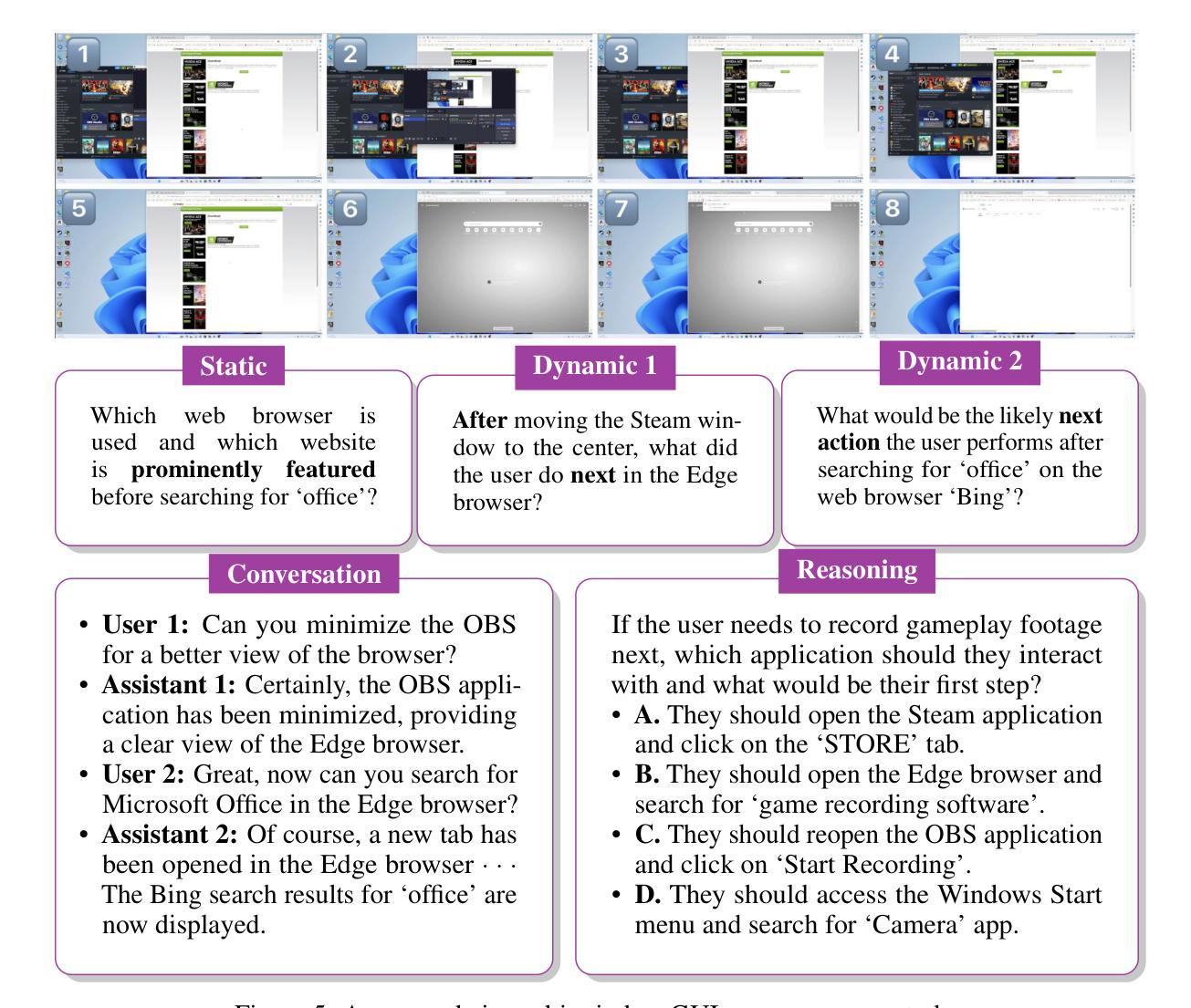
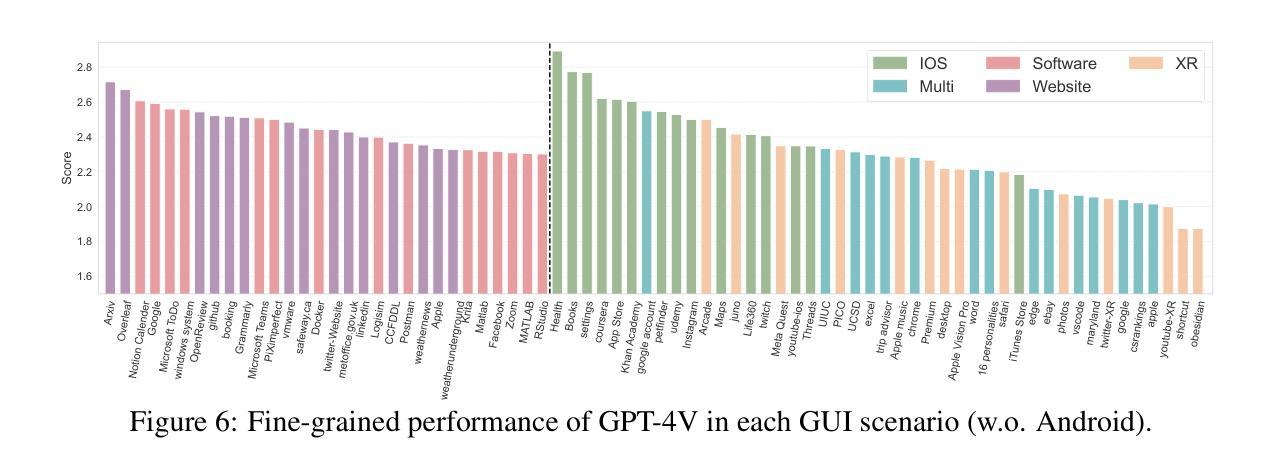