⚠️ 以下所有内容总结都来自于 大语言模型的能力,如有错误,仅供参考,谨慎使用
🔴 请注意:千万不要用于严肃的学术场景,只能用于论文阅读前的初筛!
💗 如果您觉得我们的项目对您有帮助 ChatPaperFree ,还请您给我们一些鼓励!⭐️ HuggingFace免费体验
2025-03-27 更新
NullSwap: Proactive Identity Cloaking Against Deepfake Face Swapping
Authors:Tianyi Wang, Harry Cheng, Xiao Zhang, Yinglong Wang
Suffering from performance bottlenecks in passively detecting high-quality Deepfake images due to the advancement of generative models, proactive perturbations offer a promising approach to disabling Deepfake manipulations by inserting signals into benign images. However, existing proactive perturbation approaches remain unsatisfactory in several aspects: 1) visual degradation due to direct element-wise addition; 2) limited effectiveness against face swapping manipulation; 3) unavoidable reliance on white- and grey-box settings to involve generative models during training. In this study, we analyze the essence of Deepfake face swapping and argue the necessity of protecting source identities rather than target images, and we propose NullSwap, a novel proactive defense approach that cloaks source image identities and nullifies face swapping under a pure black-box scenario. We design an Identity Extraction module to obtain facial identity features from the source image, while a Perturbation Block is then devised to generate identity-guided perturbations accordingly. Meanwhile, a Feature Block extracts shallow-level image features, which are then fused with the perturbation in the Cloaking Block for image reconstruction. Furthermore, to ensure adaptability across different identity extractors in face swapping algorithms, we propose Dynamic Loss Weighting to adaptively balance identity losses. Experiments demonstrate the outstanding ability of our approach to fool various identity recognition models, outperforming state-of-the-art proactive perturbations in preventing face swapping models from generating images with correct source identities.
在被动检测高质量深度伪造图像时,由于生成模型的进步,我们面临性能瓶颈问题。主动干扰通过在良性图像中插入信号来禁用深度伪造操作,这是一种前景广阔的方法。然而,现有的主动干扰方法在某些方面仍存在不足:1)由于直接逐元素加法导致的视觉质量下降;2)对面部交换操作的对抗效果有限;3)不可避免地在白盒和灰盒设置中依赖生成模型进行训练。本研究分析了深度伪造面部交换的本质,并主张保护源身份而非目标图像的重要性。我们提出了NullSwap,这是一种新的主动防御方法,能够在纯黑匣场景下隐藏源图像身份并消除面部交换。我们设计了一个身份提取模块,从源图像中获取面部身份特征,然后设计了一个扰动块,以生成相应的身份引导扰动。同时,特征块提取浅层图像特征,然后在遮蔽块中与扰动相融合进行图像重建。此外,为了确保在不同面部交换算法的身份提取器中的适应性,我们提出了动态损失加权,以自适应地平衡身份损失。实验表明,我们的方法在欺骗各种身份识别模型方面表现出卓越的能力,在防止面部交换模型生成具有正确源身份的图象方面优于最先进的主动扰动。
论文及项目相关链接
摘要
深度学习模型生成图像时面临性能瓶颈的问题,主动扰动是一种通过在良性图像中插入信号来阻止深度伪造操纵的具有前景的方法。然而现有主动扰动方法仍存在视觉退化、对换脸操纵有效性有限以及在训练和检测中无法回避依赖白盒和灰盒设置的缺点。本研究分析深度伪造换脸的本质,主张保护源身份而非目标图像的重要性,并提出NullSwap这一新型主动防御方法,可在纯黑箱场景下掩盖源图像身份并消除换脸。设计身份提取模块从源图像获取面部身份特征,同时开发扰动块生成相应的身份引导扰动。同时,特征块提取浅层图像特征,然后与遮挡块中的扰动相融合进行图像重建。此外,为确保在不同身份提取器中的换脸算法的可适应性,我们提出动态损失加权以自适应平衡身份损失。实验证明我们的方法能欺骗各种身份识别模型,在防止换脸模型生成具有正确源身份图像方面的性能优于最先进的主动扰动方法。
关键见解
- 现有主动扰动方法存在视觉退化问题,本研究提出NullSwap方法来解决这个问题。
- 本研究主张保护源身份而非目标图像的重要性,并提出NullSwap方法可在纯黑箱场景下掩盖源图像身份并消除换脸。
- 通过设计身份提取模块和扰动块,NullSwap可以从源图像中提取面部身份特征并生成相应的身份引导扰动。
- 特征块和遮挡块的融合技术帮助重建图像。
- 动态损失加权机制确保在不同身份提取器中的换脸算法的可适应性。
- 实验证明NullSwap方法在防止换脸模型生成具有正确源身份图像方面的性能优于其他方法。
- NullSwap能够欺骗各种身份识别模型。
点此查看论文截图
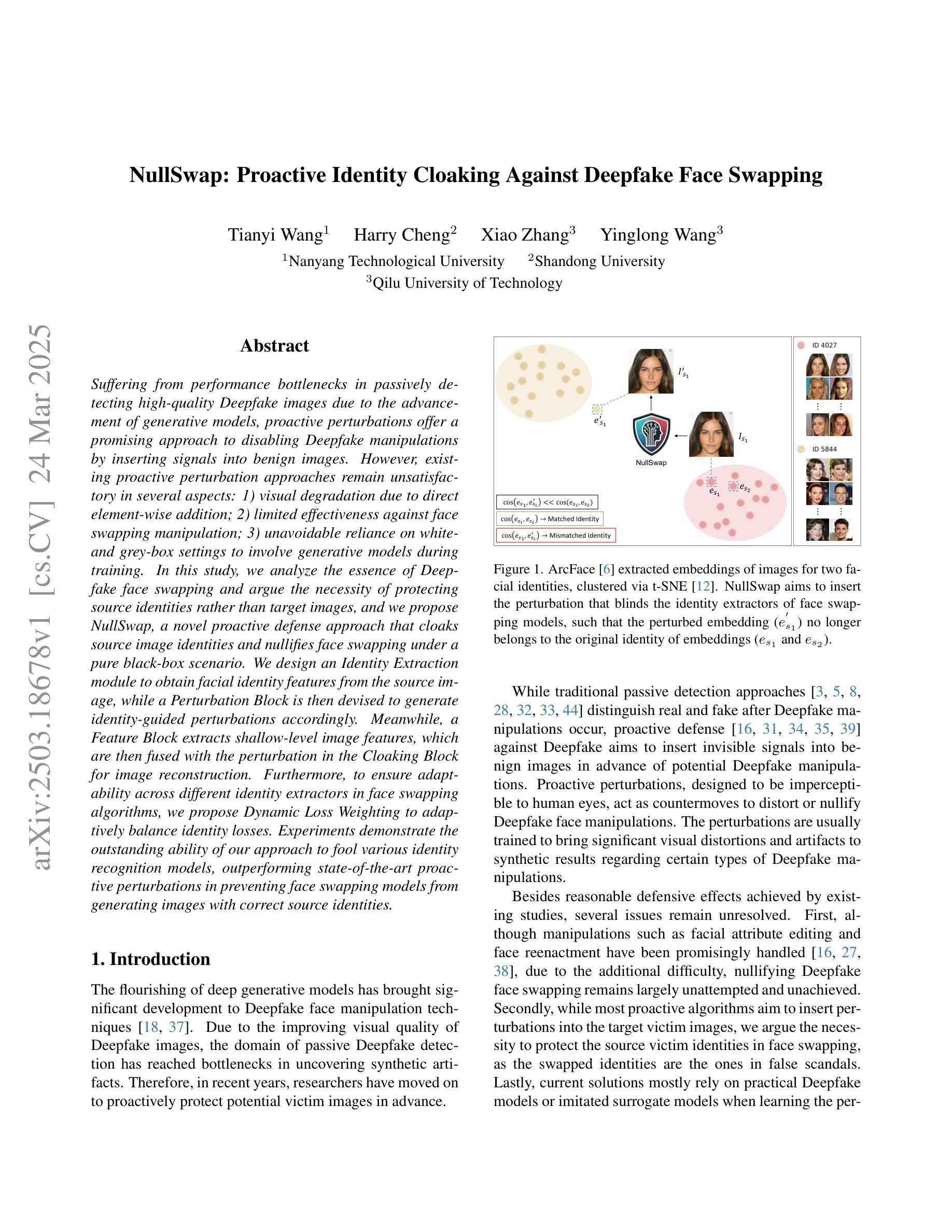
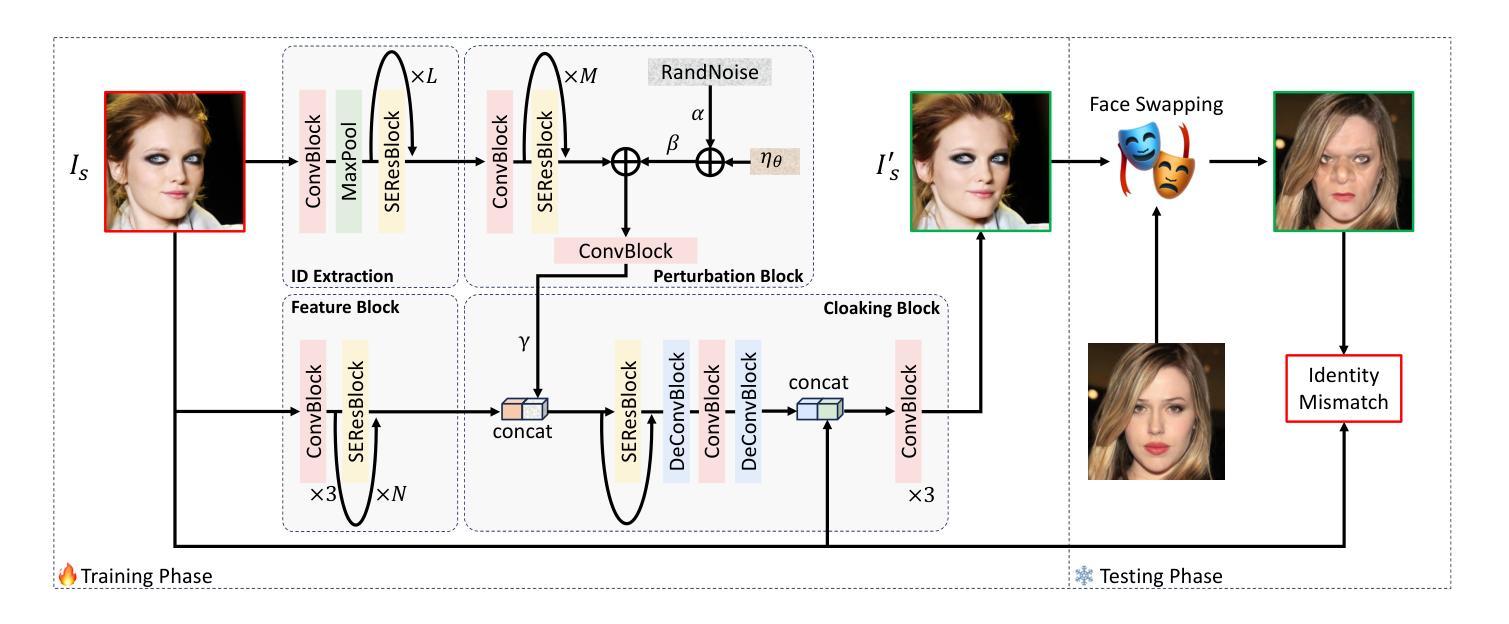
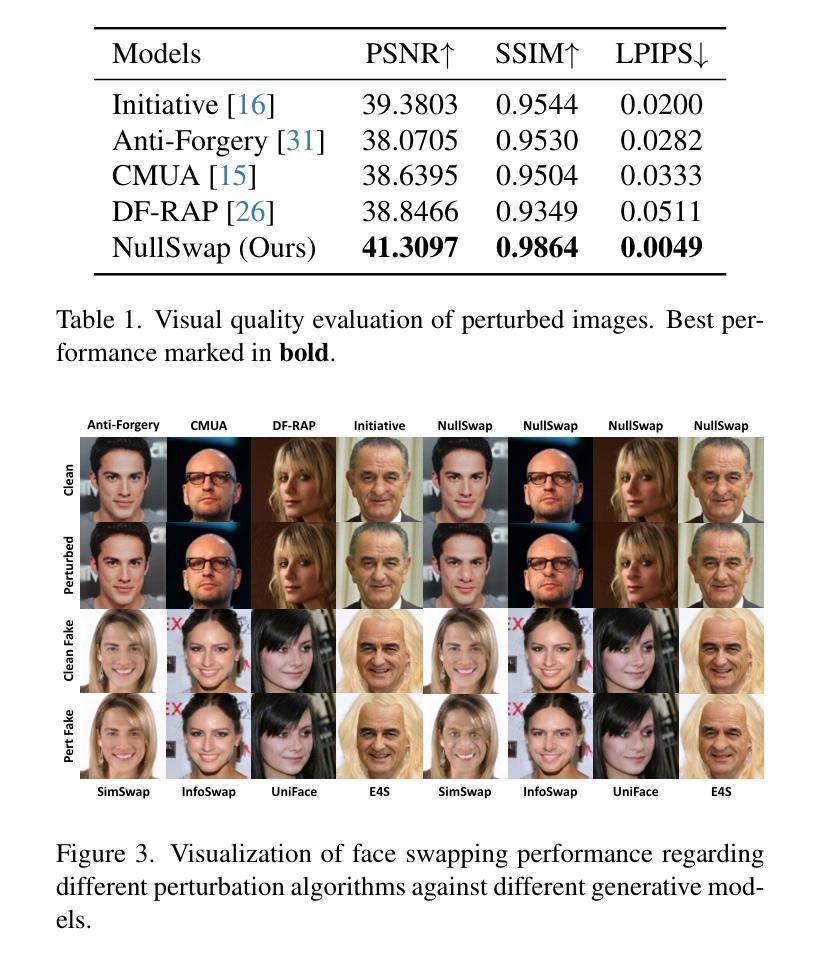
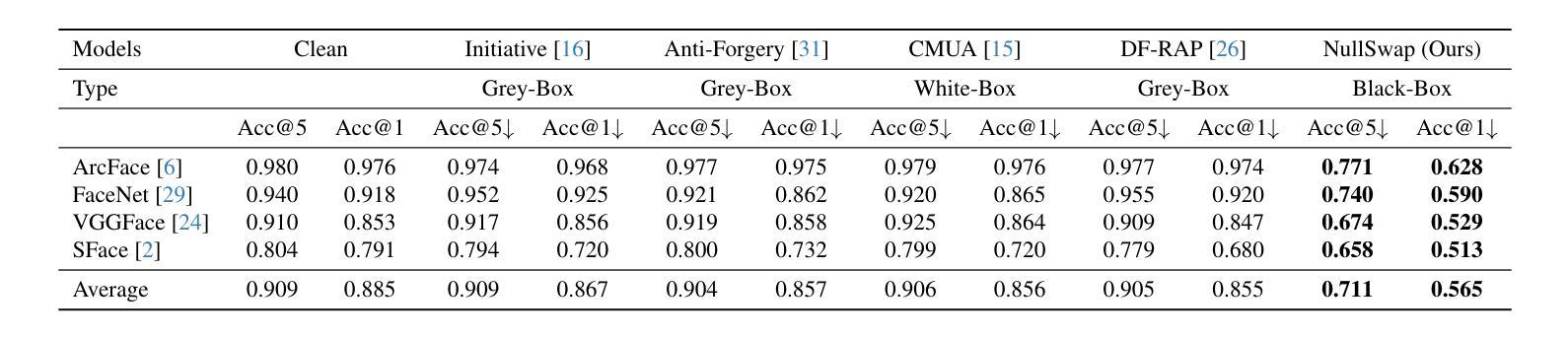
Zero-Shot Head Swapping in Real-World Scenarios
Authors:Taewoong Kang, Sohyun Jeong, Hyojin Jang, Jaegul Choo
With growing demand in media and social networks for personalized images, the need for advanced head-swapping techniques, integrating an entire head from the head image with the body from the body image, has increased. However, traditional head swapping methods heavily rely on face-centered cropped data with primarily frontal facing views, which limits their effectiveness in real world applications. Additionally, their masking methods, designed to indicate regions requiring editing, are optimized for these types of dataset but struggle to achieve seamless blending in complex situations, such as when the original data includes features like long hair extending beyond the masked area. To overcome these limitations and enhance adaptability in diverse and complex scenarios, we propose a novel head swapping method, HID, that is robust to images including the full head and the upper body, and handles from frontal to side views, while automatically generating context aware masks. For automatic mask generation, we introduce the IOMask, which enables seamless blending of the head and body, effectively addressing integration challenges. We further introduce the hair injection module to capture hair details with greater precision. Our experiments demonstrate that the proposed approach achieves state-of-the-art performance in head swapping, providing visually consistent and realistic results across a wide range of challenging conditions.
随着媒体和社交网络中对个性化图像需求的增长,对高级换头技术的需求也在增加。这种技术需要整合头像的头和全身图像的身体部分。然而,传统的换头方法严重依赖于以面部为中心的裁剪数据,主要是正面面部视图,这在现实世界的实际应用中限制了其有效性。此外,他们的遮罩方法是为了指示需要编辑的区域而设计的,对于这类数据集进行了优化,但在复杂情况下实现无缝融合时遇到了困难,例如当原始数据包括超出遮罩区域的长头发等特征时。为了克服这些局限性,并在各种复杂场景中提高适应性,我们提出了一种新型的换头方法HID,它对包含整个头部和上半身的图像具有鲁棒性,能够处理从正面到侧面的视图,并自动生成上下文感知遮罩。为了自动生成遮罩,我们引入了IOMask,它能够无缝融合头部和躯体,有效解决融合挑战。我们还进一步引入了头发注入模块,以更精确地捕捉头发细节。我们的实验表明,所提出的方法在换头方面达到了最先进的性能,在广泛的条件下提供了视觉上一致和真实的结果。
论文及项目相关链接
PDF CVPR’25
Summary
随着媒体和社交网络中对个性化图像需求的增长,需要更先进的换头技术,即将头部图像中的整个头部与身体图像中的身体部分进行集成。然而,传统的换头方法主要依赖于以面部为中心的裁剪数据,并且主要处理正面视图,这在现实世界的实际应用中限制了其有效性。本文提出了一种新型的换头方法HID,该方法对包含整个头部和上半身的图像具有强大的适应性,可以处理从正面到侧面的各种视图,并自动生成上下文感知的掩膜。引入的IOMask能够实现头部和身体的无缝融合,有效解决了集成挑战。此外,还引入了头发注入模块以更精确地捕捉头发细节。实验表明,该方法在换头术中达到了最先进的性能,在广泛的各种条件下都能产生视觉一致且逼真的结果。
Key Takeaways
- 随着媒体和社交网络中对个性化图像需求的增长,先进的换头技术变得至关重要。
- 传统换头方法主要依赖面部为中心的裁剪数据,并局限于正面视图,限制了其在现实应用中的有效性。
- 新型换头方法HID适应于包含整个头部和上半身的图像,并能处理各种视图。
- HID方法自动生成上下文感知的掩膜,实现了头部和身体的无缝融合。
- IOMask的引入为头部和身体的融合提供了更精细的掩膜生成技术。
- 头发注入模块的引入提高了捕捉头发细节的精度。
点此查看论文截图
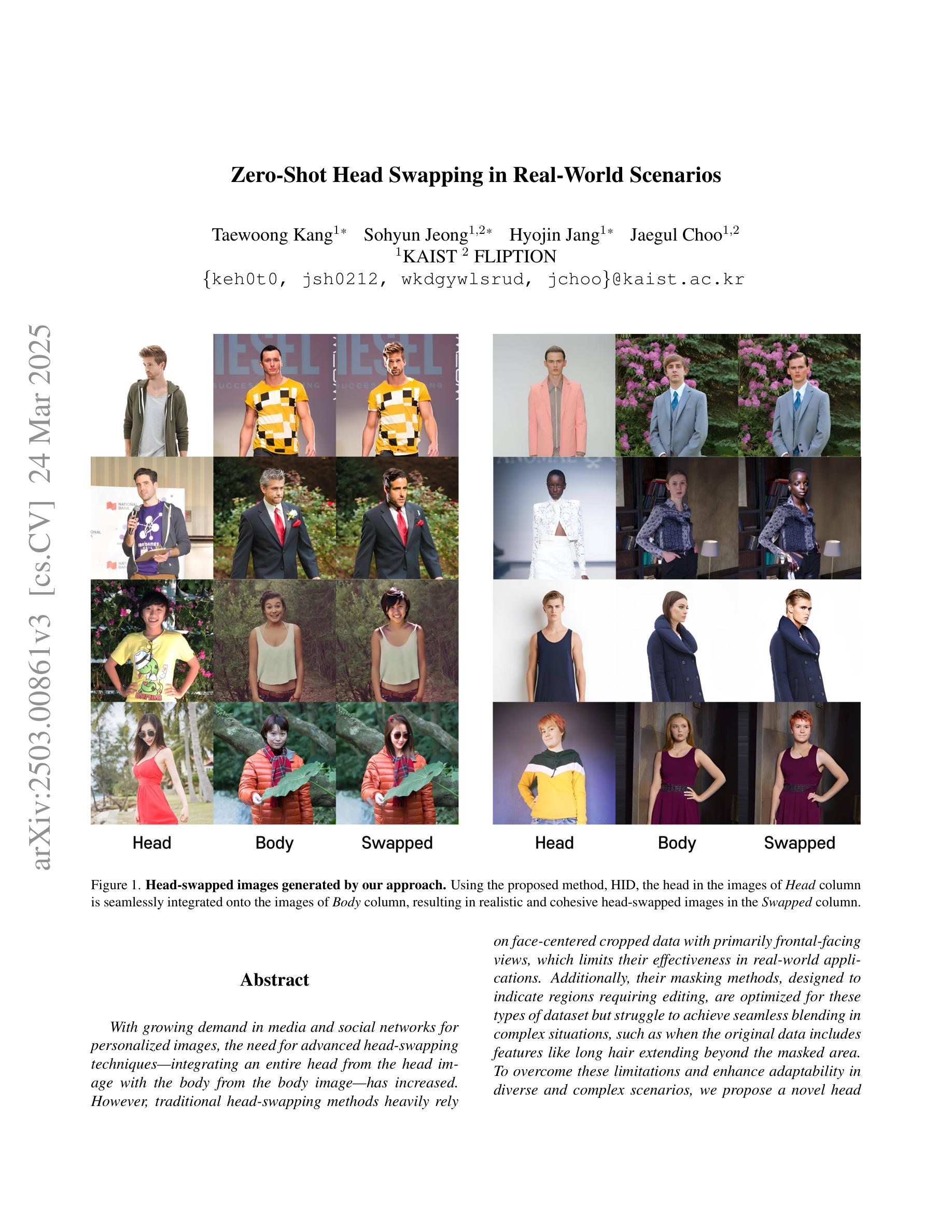
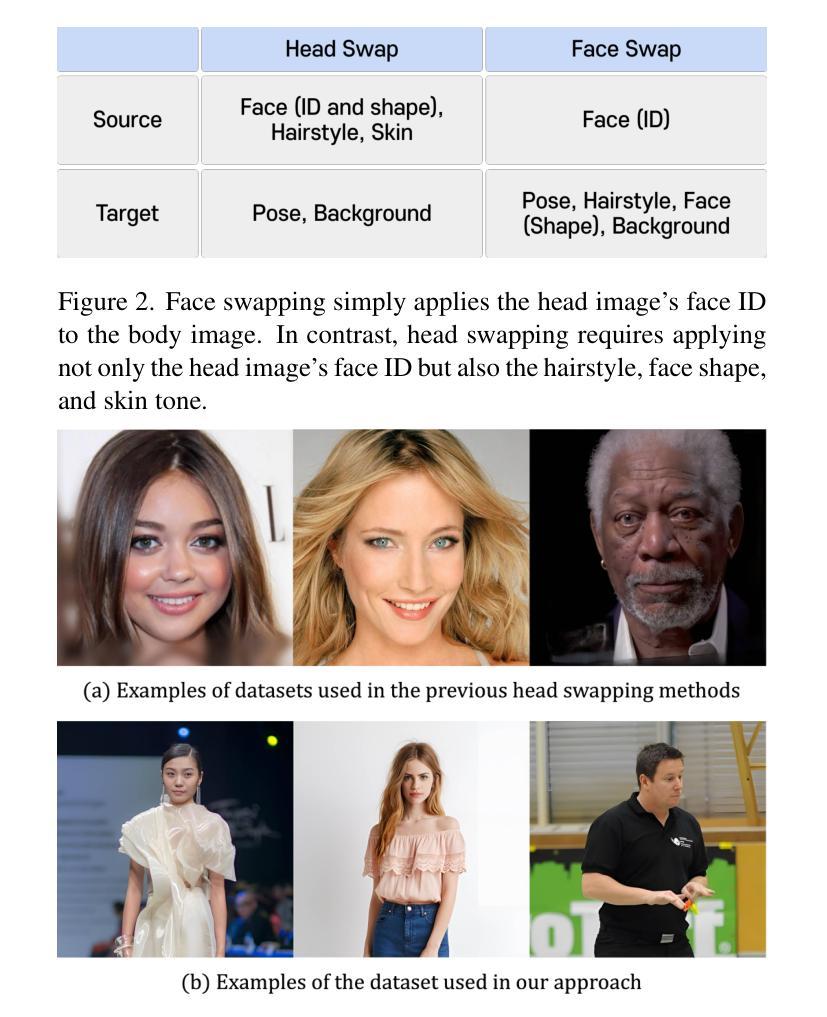
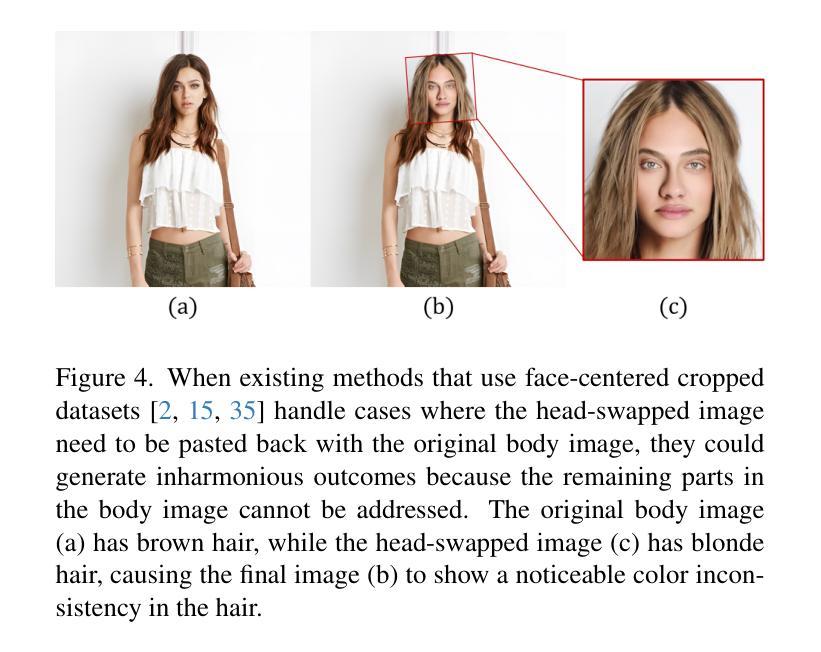
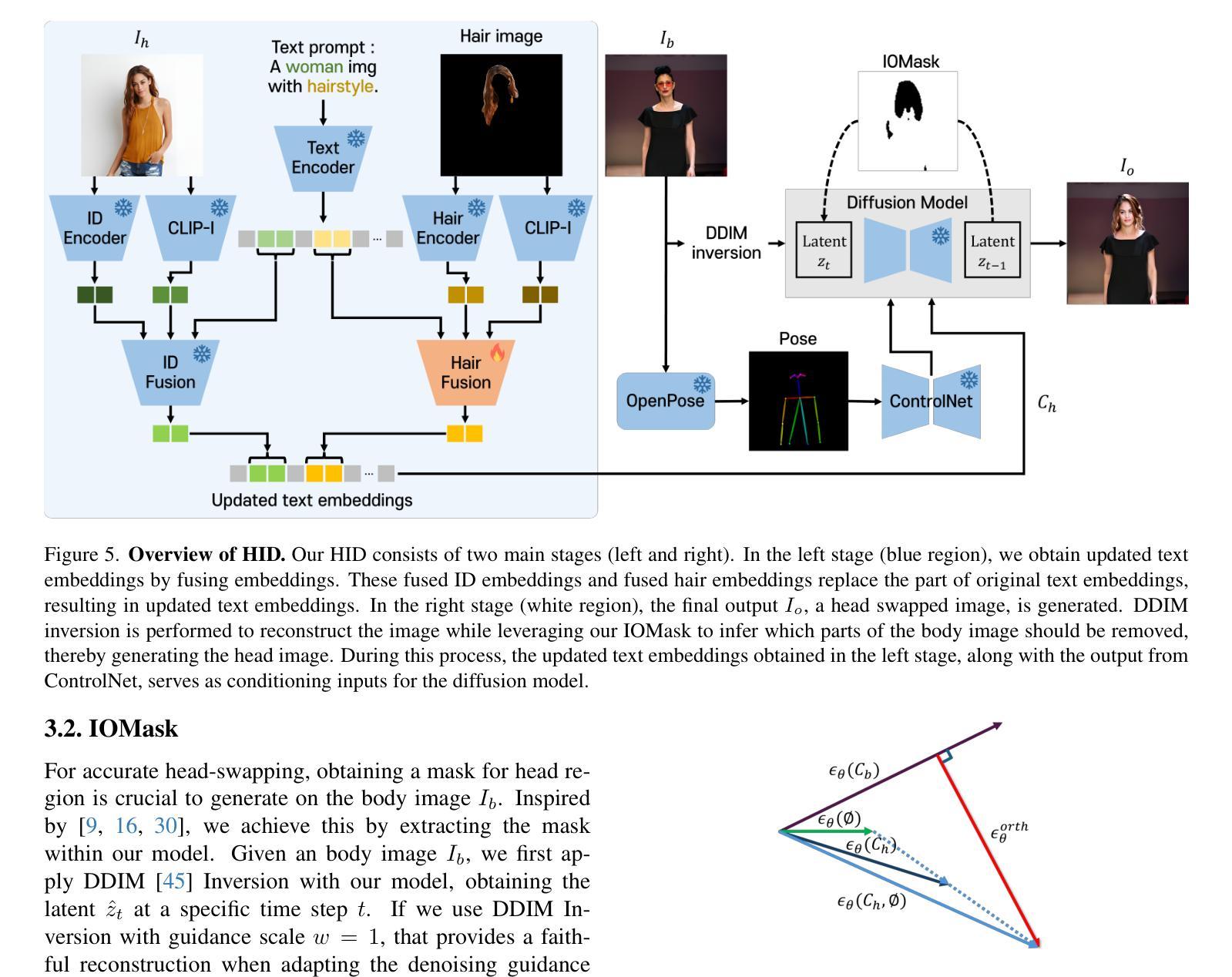