⚠️ 以下所有内容总结都来自于 大语言模型的能力,如有错误,仅供参考,谨慎使用
🔴 请注意:千万不要用于严肃的学术场景,只能用于论文阅读前的初筛!
💗 如果您觉得我们的项目对您有帮助 ChatPaperFree ,还请您给我们一些鼓励!⭐️ HuggingFace免费体验
2025-03-27 更新
Dance Like a Chicken: Low-Rank Stylization for Human Motion Diffusion
Authors:Haim Sawdayee, Chuan Guo, Guy Tevet, Bing Zhou, Jian Wang, Amit H. Bermano
Text-to-motion generative models span a wide range of 3D human actions but struggle with nuanced stylistic attributes such as a “Chicken” style. Due to the scarcity of style-specific data, existing approaches pull the generative prior towards a reference style, which often results in out-of-distribution low quality generations. In this work, we introduce LoRA-MDM, a lightweight framework for motion stylization that generalizes to complex actions while maintaining editability. Our key insight is that adapting the generative prior to include the style, while preserving its overall distribution, is more effective than modifying each individual motion during generation. Building on this idea, LoRA-MDM learns to adapt the prior to include the reference style using only a few samples. The style can then be used in the context of different textual prompts for generation. The low-rank adaptation shifts the motion manifold in a semantically meaningful way, enabling realistic style infusion even for actions not present in the reference samples. Moreover, preserving the distribution structure enables advanced operations such as style blending and motion editing. We compare LoRA-MDM to state-of-the-art stylized motion generation methods and demonstrate a favorable balance between text fidelity and style consistency.
文本到动作生成模型能够涵盖多种3D人类动作,但在细微的风格属性(如“鸡”风格)方面存在困难。由于特定风格的数据稀缺,现有方法往往将生成先验拉向参考风格,这通常会导致生成的结果分布外且质量低下。在这项工作中,我们引入了LoRA-MDM,这是一个轻量级的动作风格化框架,能够概括复杂动作并保持可编辑性。我们的关键见解是,适应生成先验以包含风格,同时保持其整体分布,这比在生成过程中修改每个单独的动作更有效。基于这一想法,LoRA-MDM学习适应先验以包含参考风格,仅使用少量样本。然后可以在不同的文本提示的上下文中使用该风格进行生成。低秩适应以语义上有意义的方式改变了动作流形,即使在参考样本中不存在的动作中也能实现逼真的风格融合。此外,保持分布结构可以进行更高级的操作,如风格混合和运动编辑。我们将LoRA-MDM与最新的风格化运动生成方法进行比较,并证明了在文本保真度和风格一致性之间的有利平衡。
论文及项目相关链接
PDF Project page at https://haimsaw.github.io/LoRA-MDM/
Summary
文本到动作生成模型涵盖多种3D人类动作,但在微妙的风格特征(如“鸡步”风格)方面存在挑战。由于特定风格的数据稀缺,现有方法往往将生成先验转向参考风格,这通常会导致生成的分布外、质量低下。本研究介绍了LoRA-MDM,一个用于动作风格化的轻量级框架,能够推广到复杂动作并保持可编辑性。我们的关键见解是,适应生成先验以包含风格,同时保持其整体分布,比在生成过程中修改每个单独的动作更有效。基于此想法,LoRA-MDM学习适应先验以包含参考风格,仅使用少数样本。然后,可以在不同的文本提示中使用这种风格进行生成。低秩适应在语义上有意义地改变运动流形,即使在参考样本中不存在的动作中也能实现逼真的风格融合。此外,保持分布结构可实现风格混合和运动编辑等高级操作。我们将LoRA-MDM与最新的风格化运动生成方法进行比较,并证明了其在文本保真度和风格一致性之间的有利平衡。
Key Takeaways
- 文本到动作生成模型在处理微妙风格特征(如特定动作风格)时面临挑战。
- 现有方法因缺乏特定风格数据,往往导致生成的分布外、质量低下。
- LoRA-MDM框架通过适应生成先验来包含参考风格,仅使用少量样本。
- LoRA-MDM能够在不同文本提示中使用特定风格进行生成。
- 低秩适应能够语义上改变运动流形,实现逼真的风格融合,即使对于参考样本中不存在的动作也是如此。
- 保持分布结构使高级操作(如风格混合和运动编辑)成为可能。
点此查看论文截图
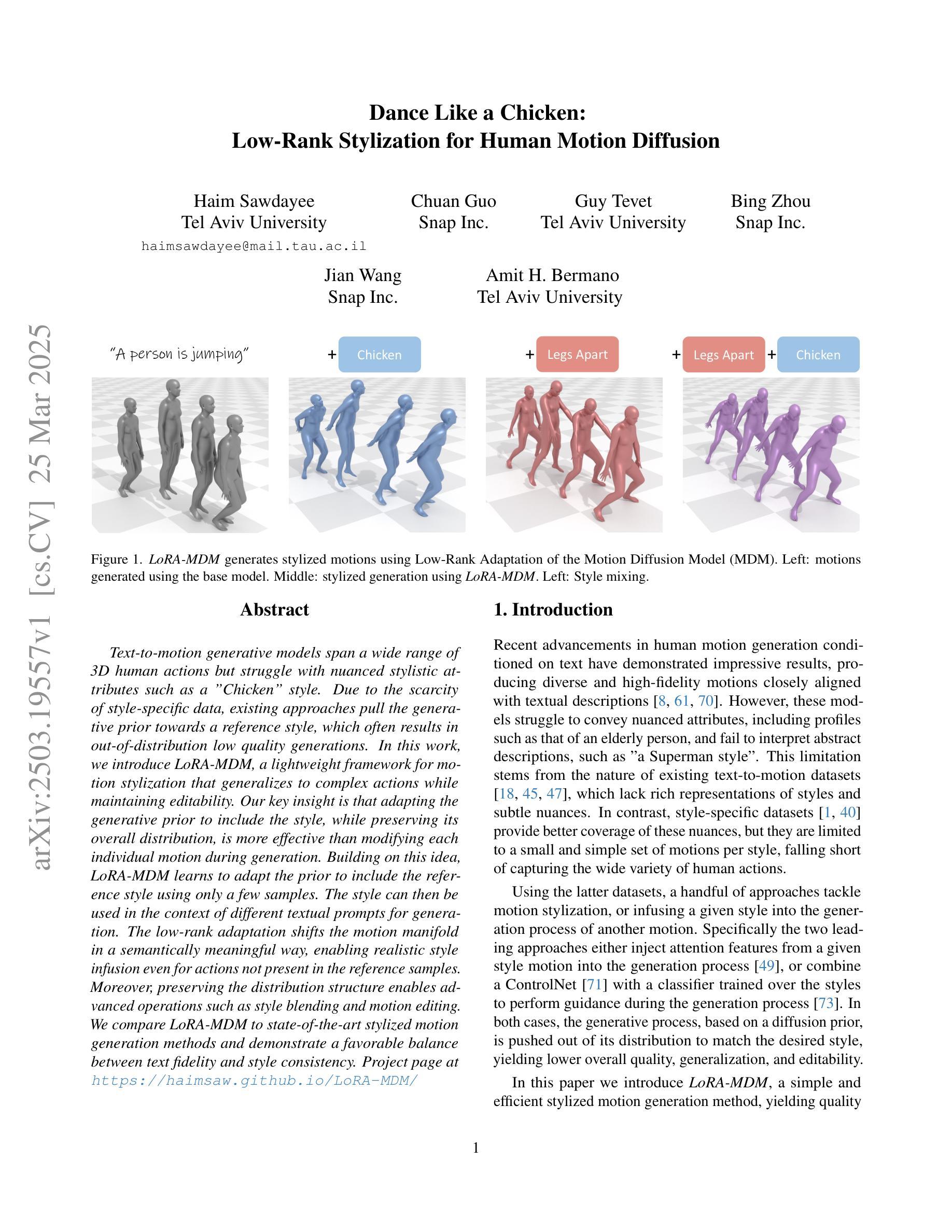
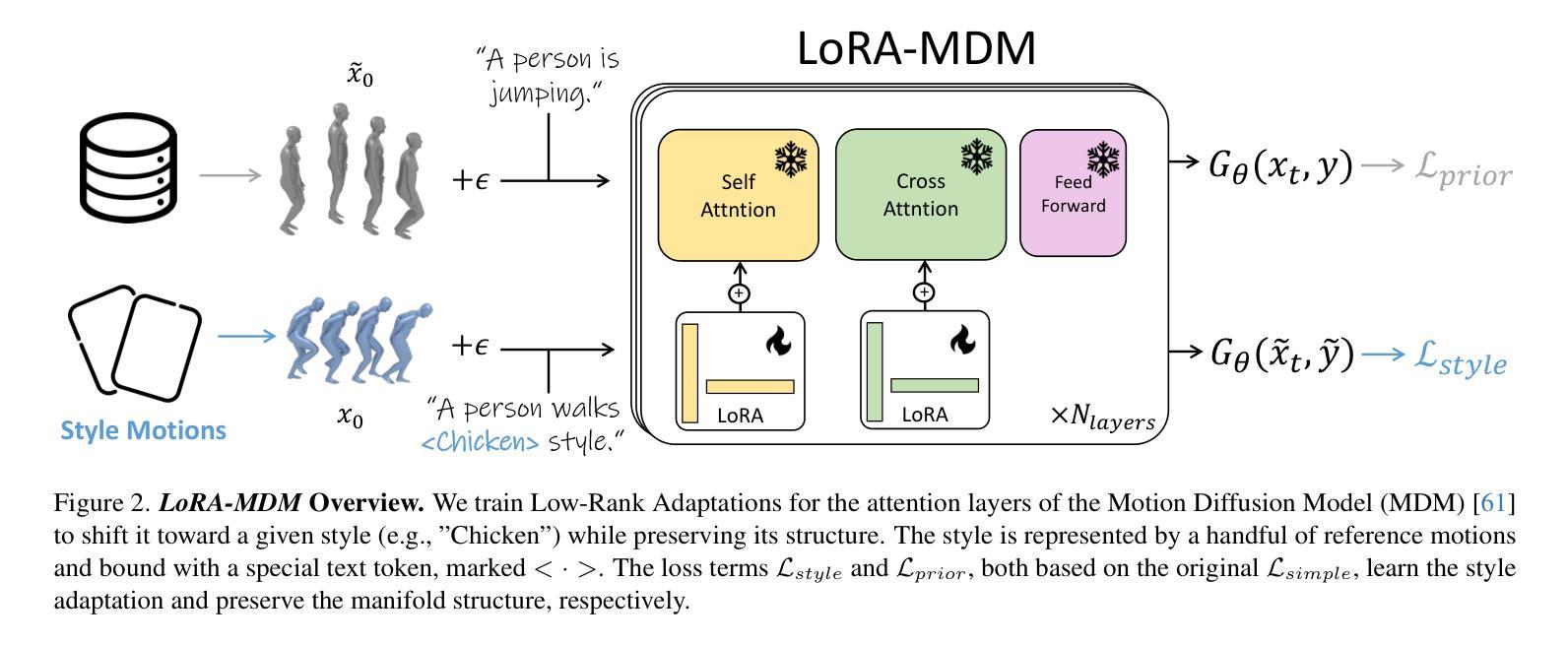
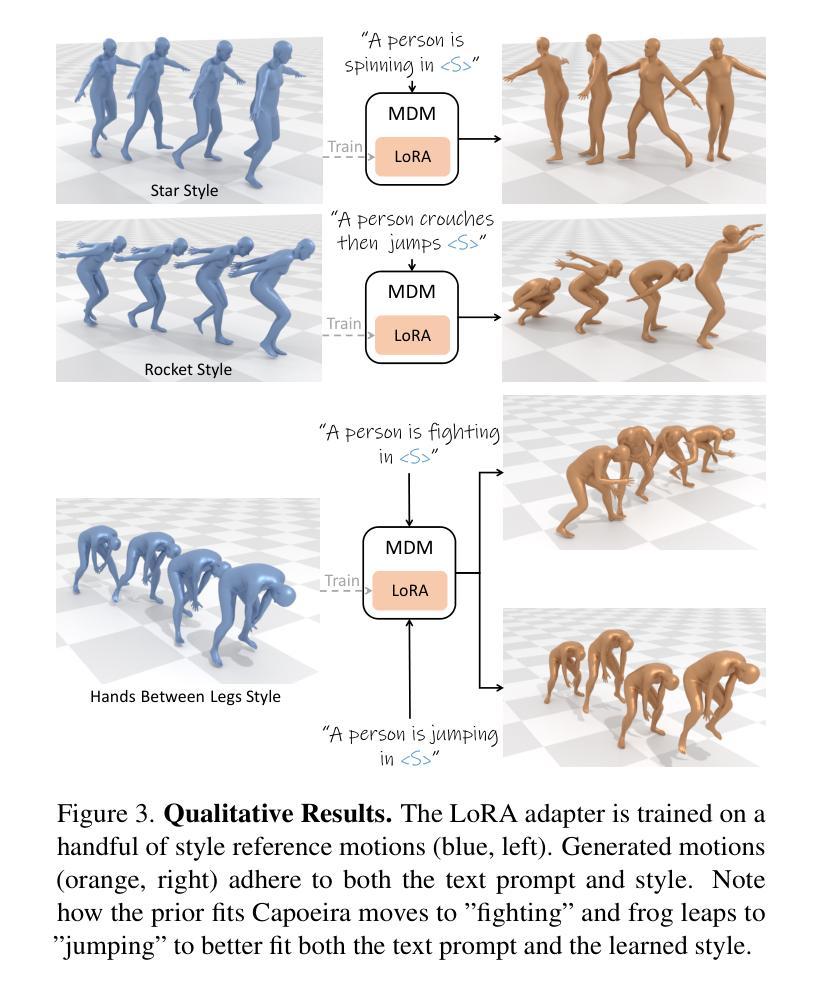
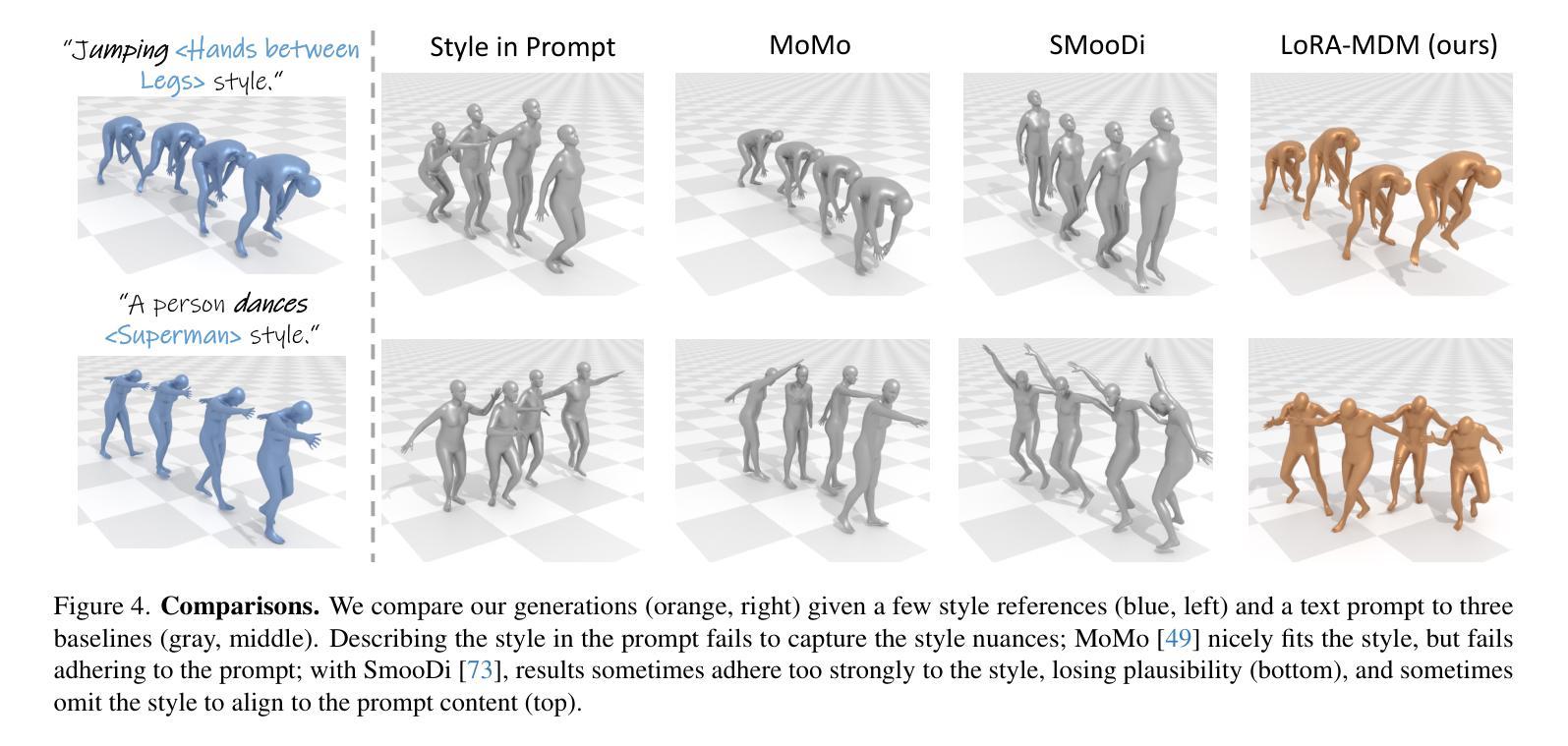
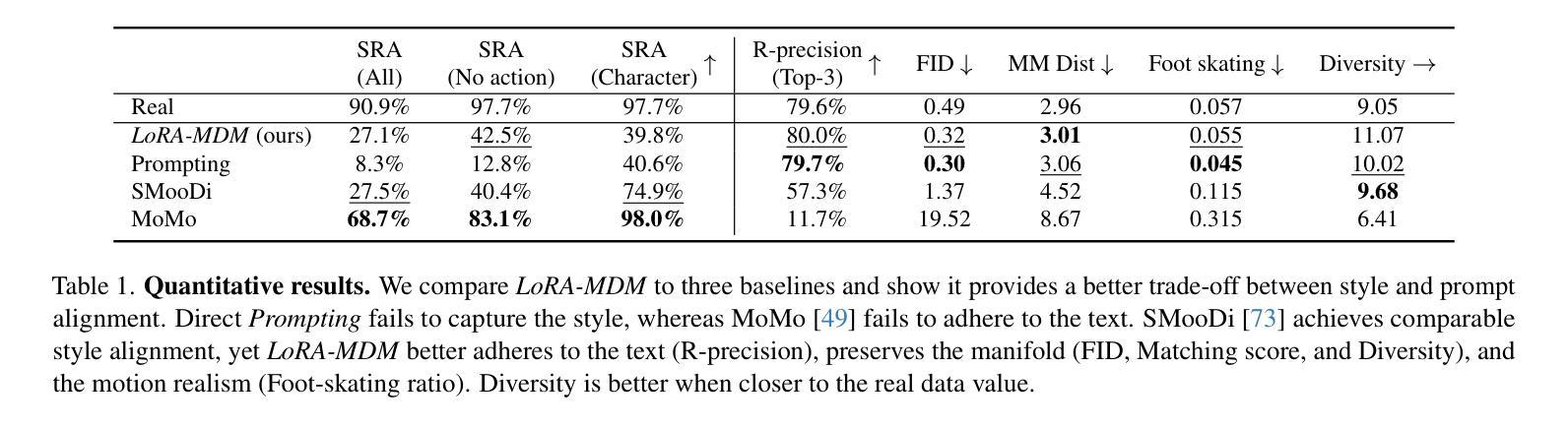
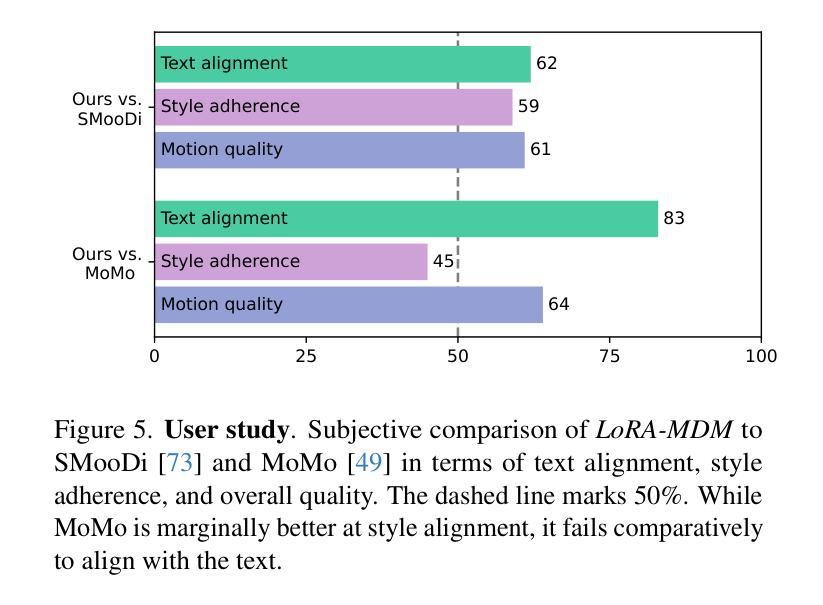
Human Motion Unlearning
Authors:Edoardo De Matteis, Matteo Migliarini, Alessio Sampieri, Indro Spinelli, Fabio Galasso
We introduce the task of human motion unlearning to prevent the synthesis of toxic animations while preserving the general text-to-motion generative performance. Unlearning toxic motions is challenging as those can be generated from explicit text prompts and from implicit toxic combinations of safe motions (e.g., kicking" is
loading and swinging a leg”). We propose the first motion unlearning benchmark by filtering toxic motions from the large and recent text-to-motion datasets of HumanML3D and Motion-X. We propose baselines, by adapting state-of-the-art image unlearning techniques to process spatio-temporal signals. Finally, we propose a novel motion unlearning model based on Latent Code Replacement, which we dub LCR. LCR is training-free and suitable to the discrete latent spaces of state-of-the-art text-to-motion diffusion models. LCR is simple and consistently outperforms baselines qualitatively and quantitatively. Project page: \href{https://www.pinlab.org/hmu}{https://www.pinlab.org/hmu}.
我们引入人类运动遗忘任务,旨在防止有毒动画的合成,同时保留一般的文本到运动的生成性能。遗忘有毒运动具有挑战性,因为这些运动可能源于明确的文本提示和安全的运动组合产生的隐含毒性(例如,“踢”是“加载和摆动腿”)。我们从HumanML3D和Motion-X的大型最新文本到运动数据集中过滤有毒运动,从而提出第一个运动遗忘基准测试。我们通过对先进的图像遗忘技术进行改编,提出基线水平处理时空信号。最后,我们提出了一种基于潜在代码替换的新型运动遗忘模型,我们称之为LCR。LCR无需训练,适用于最新的文本到运动扩散模型的离散潜在空间。LCR简单且定性定量上均始终优于基线。项目页面:https://www.pinlab.org/hmu。
论文及项目相关链接
Summary
本文介绍了人类运动“去学习”的任务,旨在防止有毒动画的合成,同时保留一般的文本到运动的生成性能。文章提出了一个运动去学习的基准测试,通过对HumanML3D和Motion-X等大型文本到运动数据集进行有毒运动的过滤来实现。同时,提出了基于图像去学习方法基线的一种自适应运动去学习方法,并提出了一种新的基于潜在代码替换的运动学习方法——LCR。LCR是一种无训练的方式,适用于当前先进的文本到运动扩散模型的离散潜在空间。总体而言,LCR简单且一致地在质量和数量上超越了基线方法。
Key Takeaways
- 引入人类运动去学习的任务,旨在避免合成有毒动画并保留文本到运动的生成性能。
- 提出首个运动去学习的基准测试,通过过滤大型文本到运动数据集中的有毒运动来实现。
- 介绍了使用基于图像去学习方法的基线进行运动去学习的自适应方法。
- 提出了一种名为LCR的运动学习方法,基于潜在代码替换且无需训练。
- LCR适用于当前流行的文本到运动扩散模型的离散潜在空间。
- LCR简单且有效地超越基线方法在处理文本到运动数据的性能。
点此查看论文截图
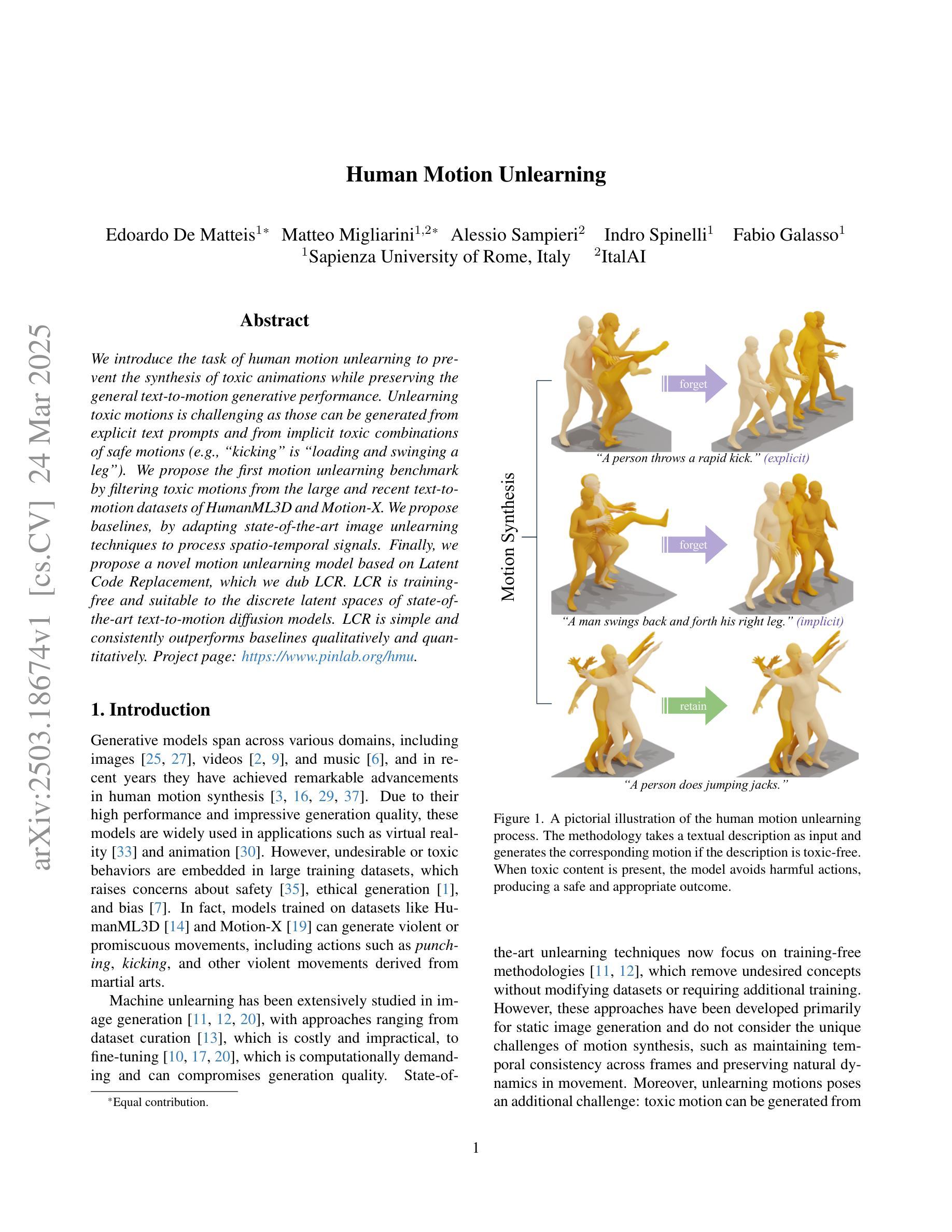
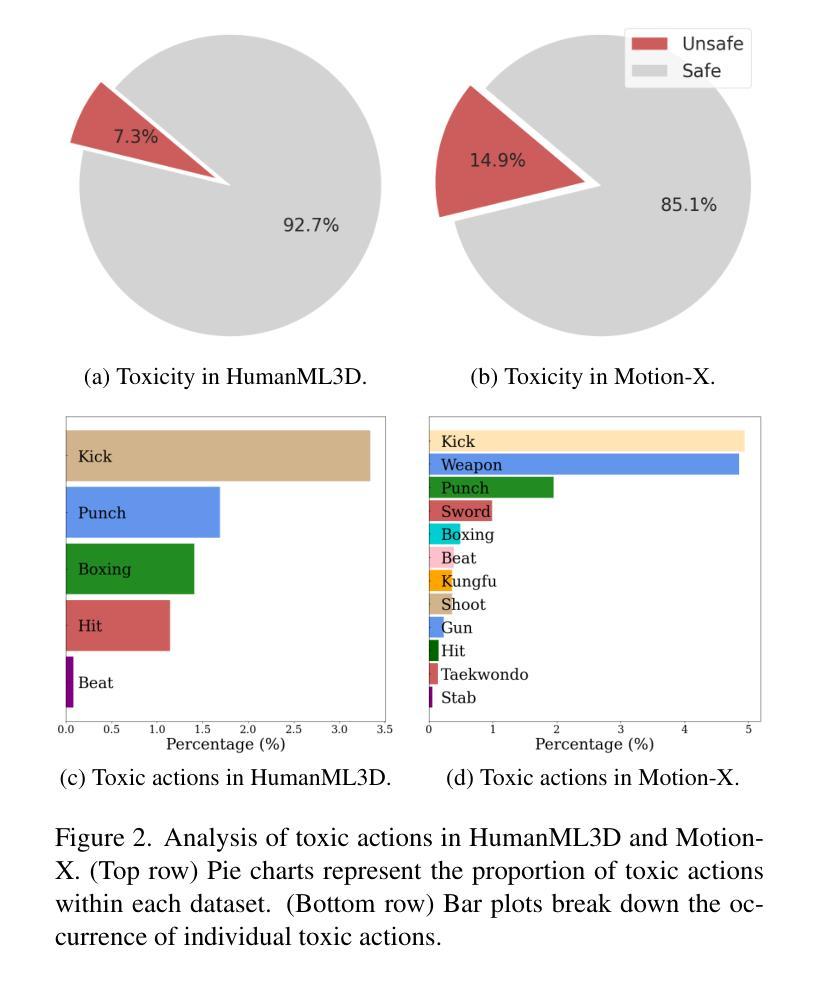
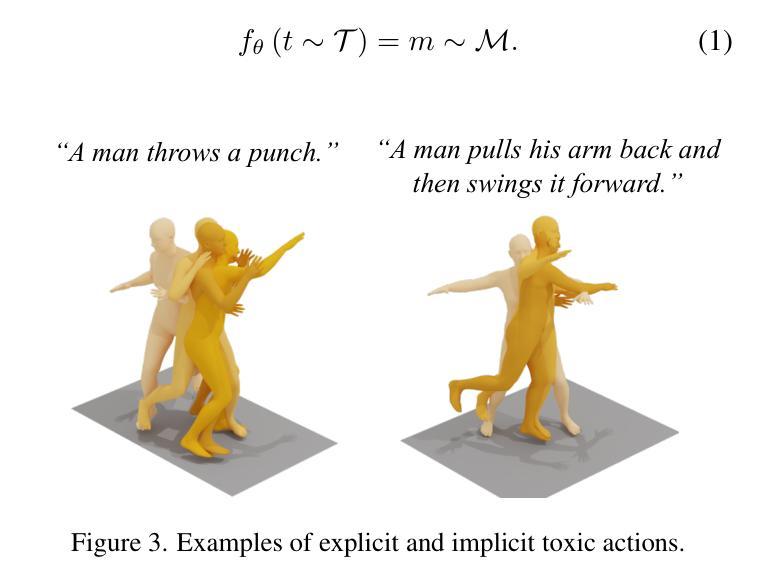
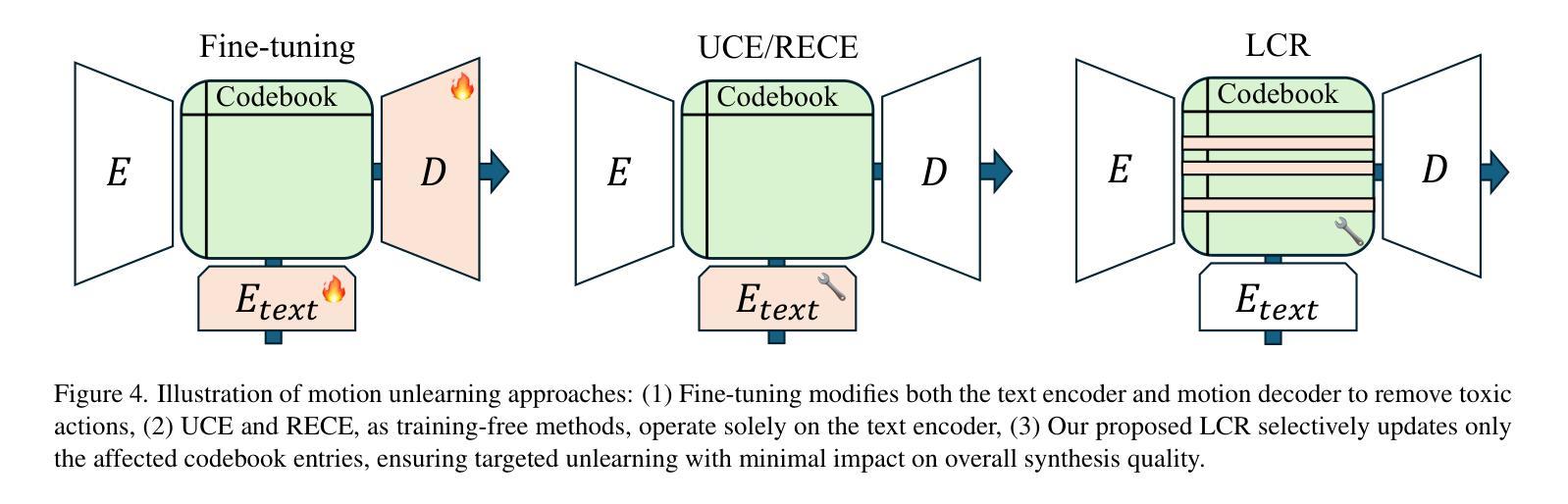
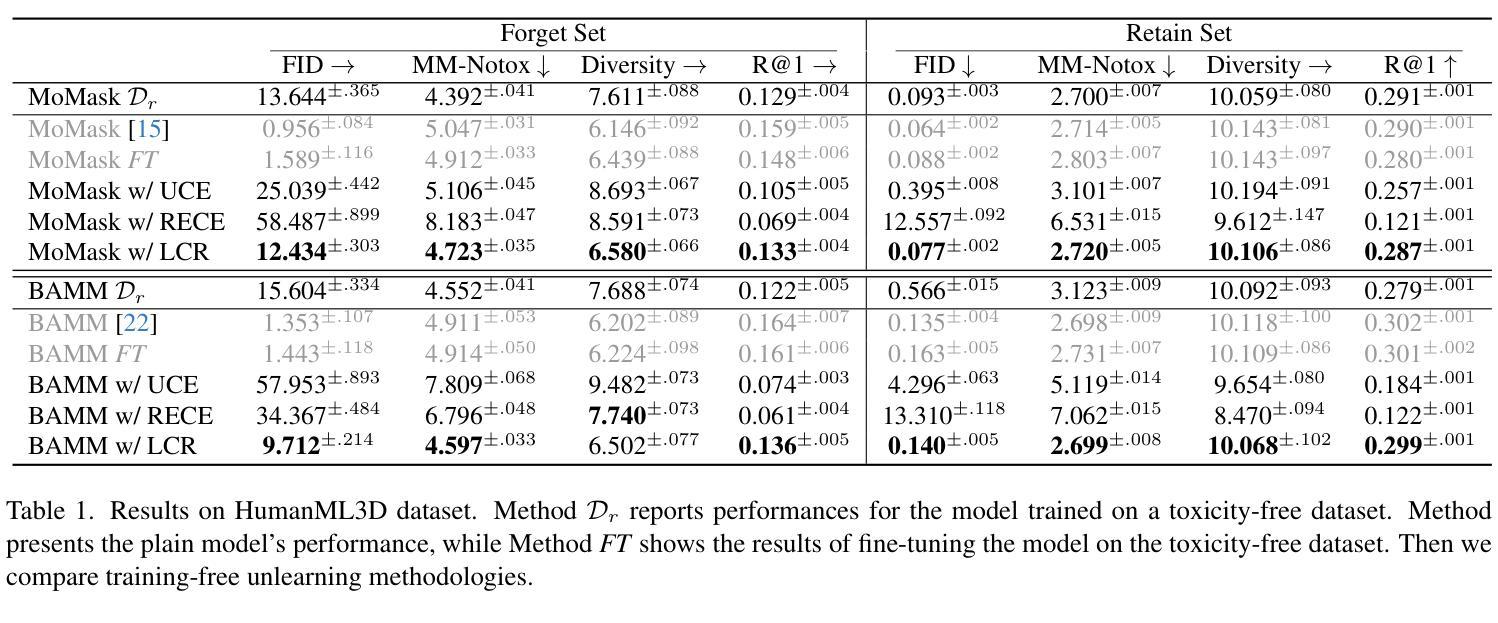
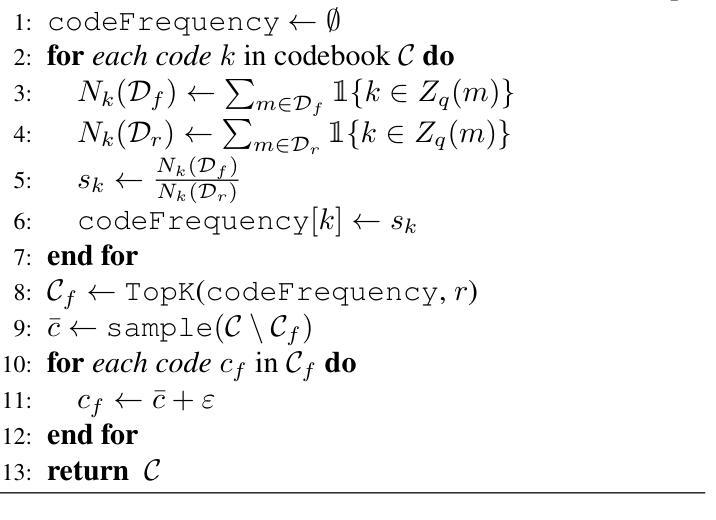
Auto-Regressive Diffusion for Generating 3D Human-Object Interactions
Authors:Zichen Geng, Zeeshan Hayder, Wei Liu, Ajmal Saeed Mian
Text-driven Human-Object Interaction (Text-to-HOI) generation is an emerging field with applications in animation, video games, virtual reality, and robotics. A key challenge in HOI generation is maintaining interaction consistency in long sequences. Existing Text-to-Motion-based approaches, such as discrete motion tokenization, cannot be directly applied to HOI generation due to limited data in this domain and the complexity of the modality. To address the problem of interaction consistency in long sequences, we propose an autoregressive diffusion model (ARDHOI) that predicts the next continuous token. Specifically, we introduce a Contrastive Variational Autoencoder (cVAE) to learn a physically plausible space of continuous HOI tokens, thereby ensuring that generated human-object motions are realistic and natural. For generating sequences autoregressively, we develop a Mamba-based context encoder to capture and maintain consistent sequential actions. Additionally, we implement an MLP-based denoiser to generate the subsequent token conditioned on the encoded context. Our model has been evaluated on the OMOMO and BEHAVE datasets, where it outperforms existing state-of-the-art methods in terms of both performance and inference speed. This makes ARDHOI a robust and efficient solution for text-driven HOI tasks
文本驱动的人机交互(Text-to-HOI)生成是一个新兴领域,在动画、电子游戏、虚拟现实和机器人技术等领域有广泛应用。人机交互生成的关键挑战在于如何在长序列中保持交互一致性。现有的基于文本到动作(Text-to-Motion)的方法,如离散动作符号化,无法直接应用于人机交互生成,因为该领域的数据量有限且模式复杂。为了解决长序列中的交互一致性问题,我们提出了一种自回归扩散模型(ARDHOI),该模型可预测下一个连续符号。具体来说,我们引入对比变分自动编码器(cVAE)来学习连续的人机交互符号的物理可能空间,从而确保生成的人机交互动作真实自然。为了自回归地生成序列,我们开发了一种基于Mamba的上下文编码器,以捕获并维持连贯的序列动作。此外,我们实现了一种基于多层感知机的去噪器,根据编码的上下文生成后续符号。我们的模型已在OMOMO和BEHAVE数据集上进行了评估,在性能和推理速度方面均优于现有最先进的方法。这使得ARDHOI成为文本驱动的人机交互任务的稳健高效解决方案。
论文及项目相关链接
Summary
本文介绍了Text-driven Human-Object Interaction(Text-to-HOI)生成领域的一个新兴挑战:在长序列中保持交互一致性。针对此挑战,提出了一种基于自动回归扩散模型(ARDHOI)的方法,通过引入对比变分自编码器(cVAE)学习连续HOI符号的物理可行空间,并使用Mamba-based上下文编码器进行序列的自动回归生成。此外,还实现了MLP-based denoiser来生成基于编码上下文的后续符号。在OMOMO和BEHAVE数据集上的实验表明,该方法在性能和推理速度方面都优于现有方法。
Key Takeaways
- Text-driven Human-Object Interaction (Text-to-HOI) 生成是一个新兴领域,应用于动画、视频游戏、虚拟现实和机器人技术。
- 保持长序列中的交互一致性是HOI生成的关键挑战。
- 现有Text-to-Motion方法如离散运动符号化不能直接应用于HOI生成。
- 提出了自动回归扩散模型(ARDHOI)来预测下一个连续符号。
- 引入对比变分自编码器(cVAE)学习物理可行的连续HOI符号空间。
- 使用Mamba-based上下文编码器和MLP-based denoiser来生成序列和细化符号。
点此查看论文截图
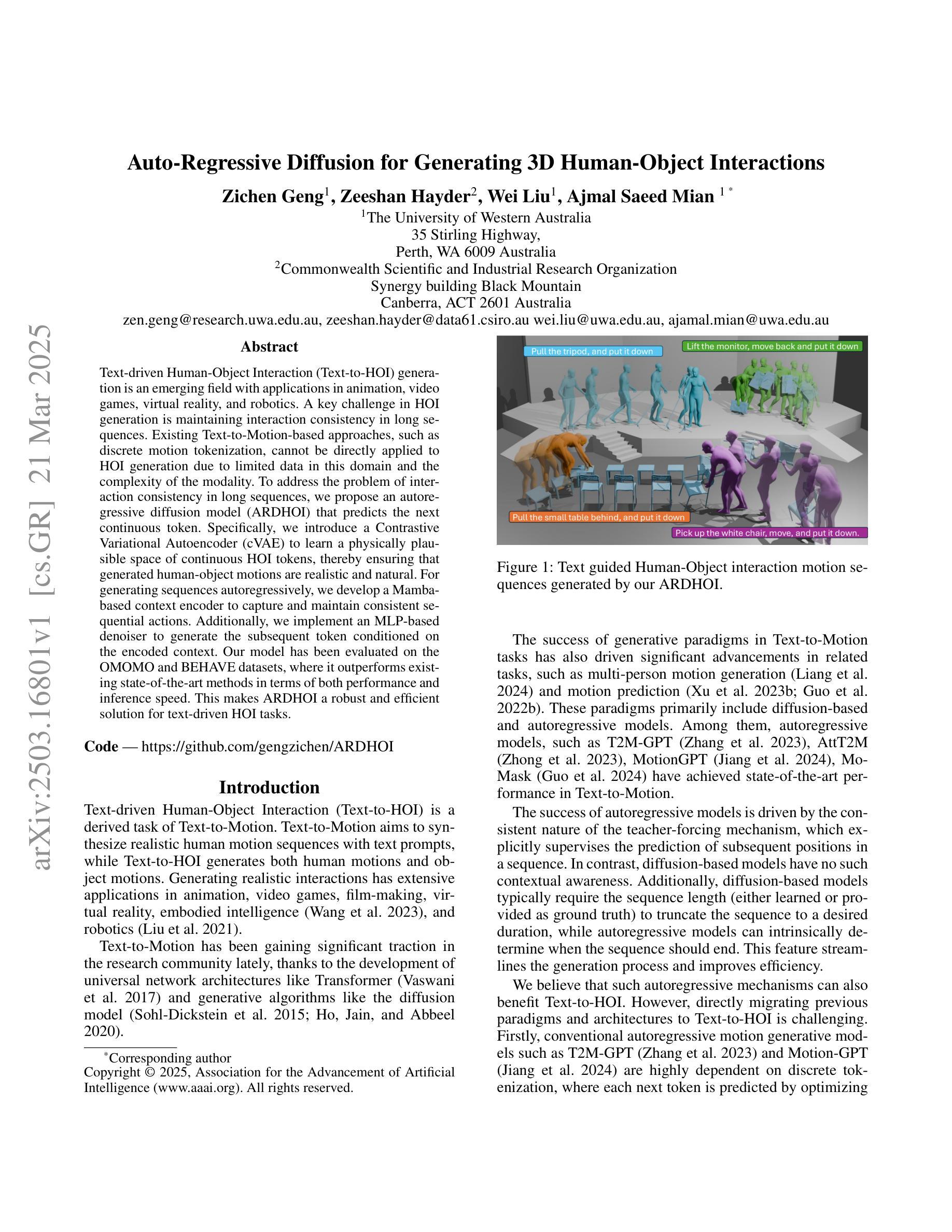
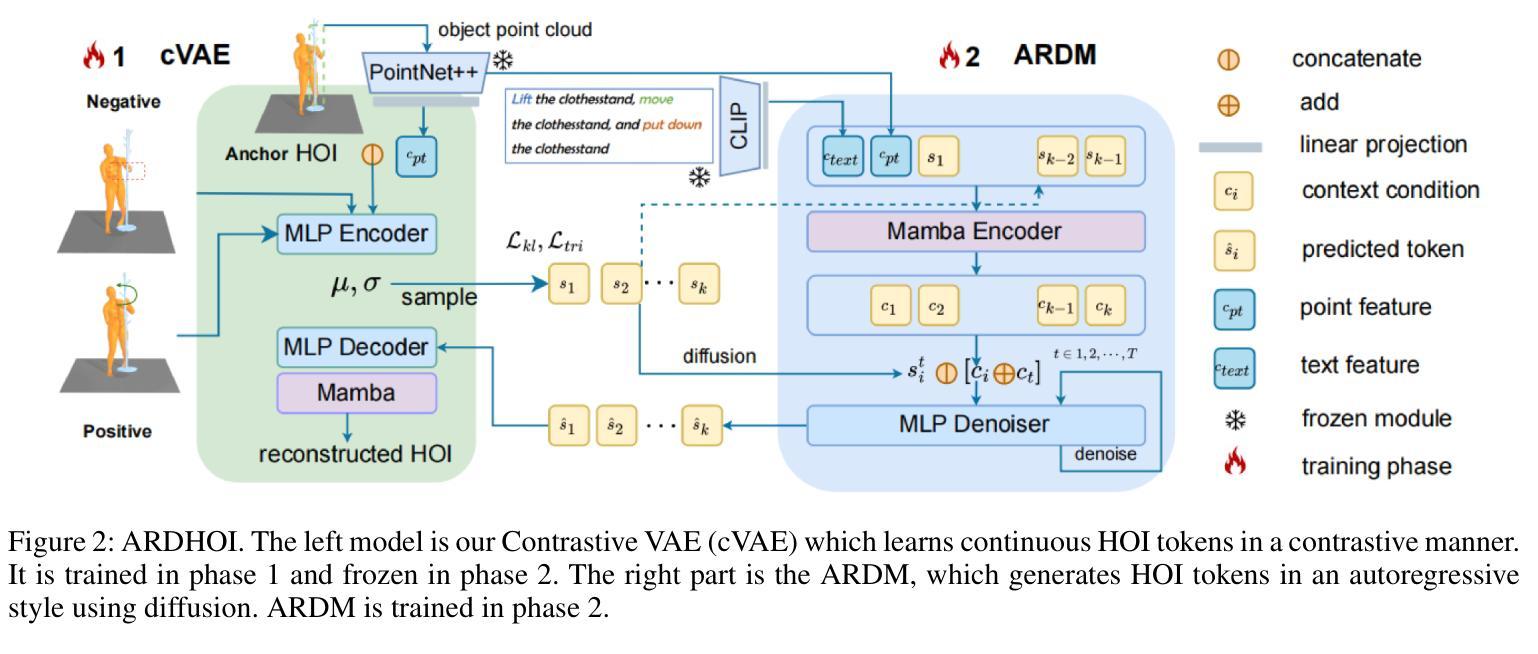
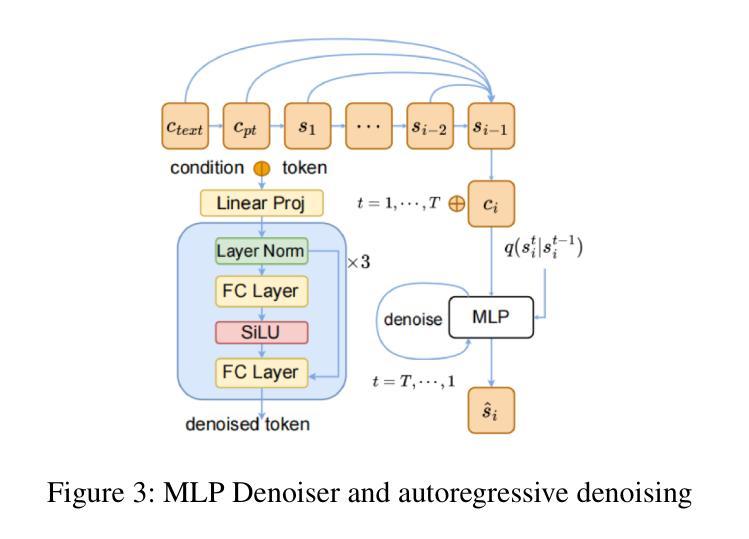
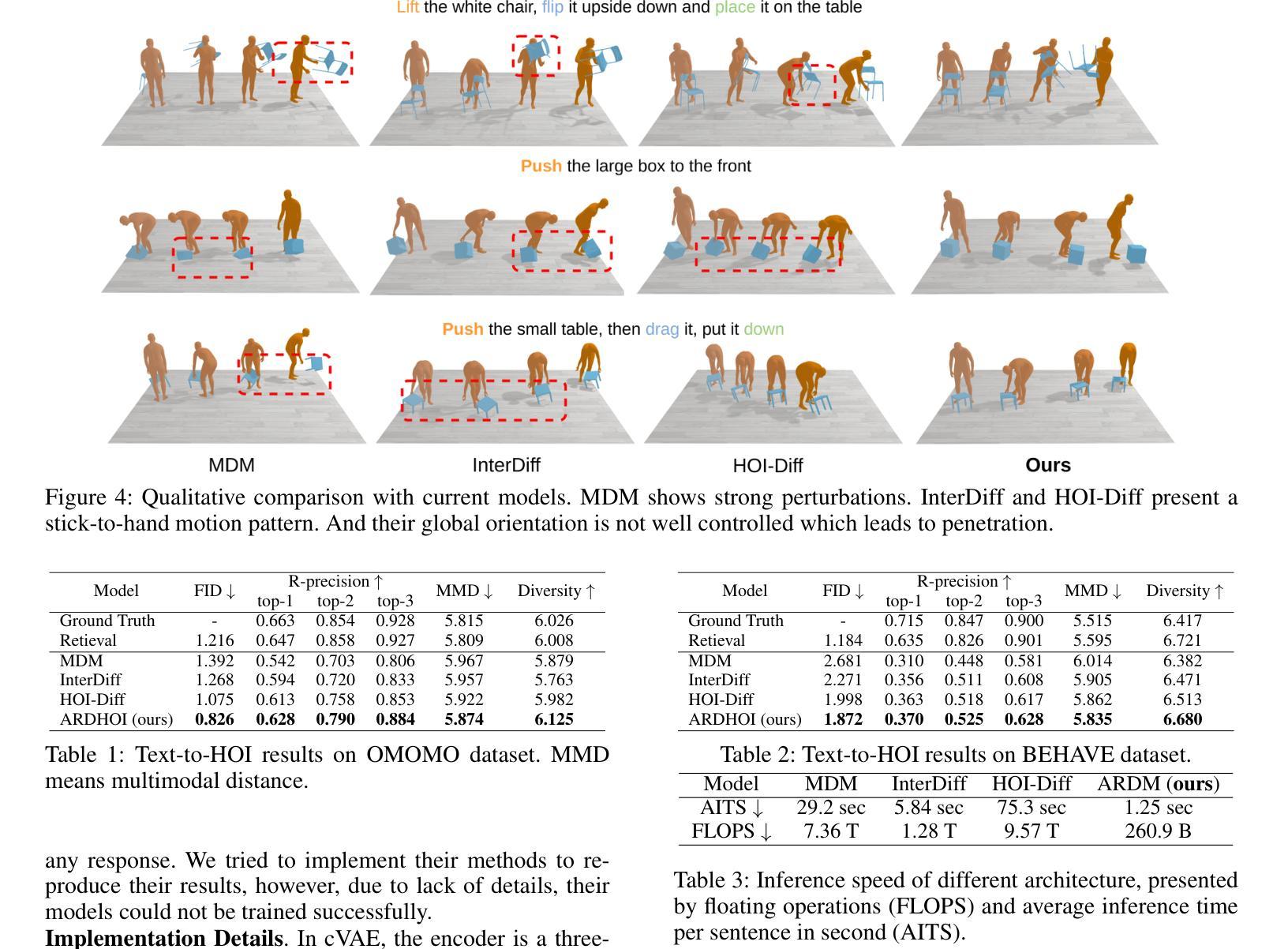
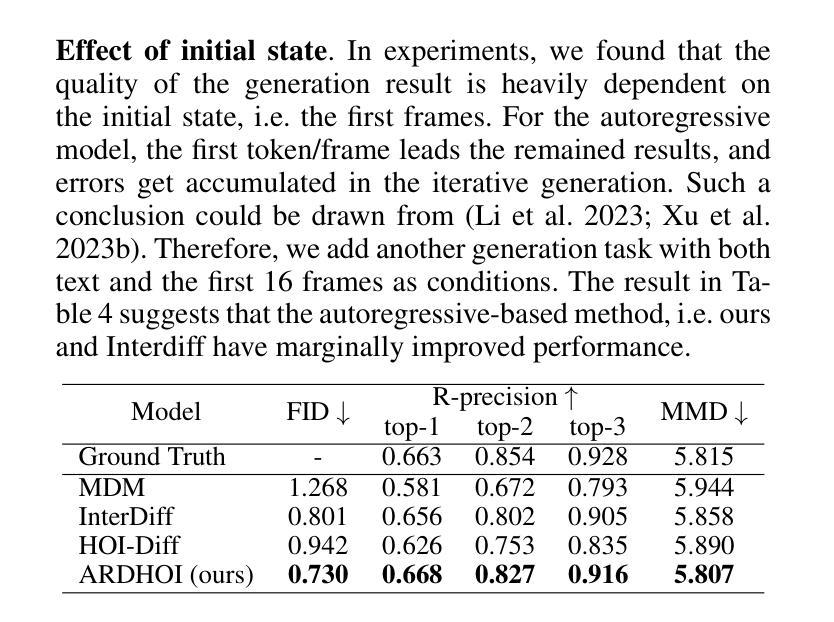
PersonaBooth: Personalized Text-to-Motion Generation
Authors:Boeun Kim, Hea In Jeong, JungHoon Sung, Yihua Cheng, Jeongmin Lee, Ju Yong Chang, Sang-Il Choi, Younggeun Choi, Saim Shin, Jungho Kim, Hyung Jin Chang
This paper introduces Motion Personalization, a new task that generates personalized motions aligned with text descriptions using several basic motions containing Persona. To support this novel task, we introduce a new large-scale motion dataset called PerMo (PersonaMotion), which captures the unique personas of multiple actors. We also propose a multi-modal finetuning method of a pretrained motion diffusion model called PersonaBooth. PersonaBooth addresses two main challenges: i) A significant distribution gap between the persona-focused PerMo dataset and the pretraining datasets, which lack persona-specific data, and ii) the difficulty of capturing a consistent persona from the motions vary in content (action type). To tackle the dataset distribution gap, we introduce a persona token to accept new persona features and perform multi-modal adaptation for both text and visuals during finetuning. To capture a consistent persona, we incorporate a contrastive learning technique to enhance intra-cohesion among samples with the same persona. Furthermore, we introduce a context-aware fusion mechanism to maximize the integration of persona cues from multiple input motions. PersonaBooth outperforms state-of-the-art motion style transfer methods, establishing a new benchmark for motion personalization.
本文介绍了动作个性化这一新任务,该任务通过使用包含个性特征的基本动作来生成与文本描述相对应的个人化动作。为了支持这一新任务,我们引入了一个名为PerMo(PersonaMotion)的大规模动作数据集,它能够捕捉多个角色的独特个性。我们还提出了一种预训练运动扩散模型的多模态微调方法,该方法被称为PersonaBooth。PersonaBooth解决了两个主要挑战:一是以个性为中心的PerMo数据集与缺乏个性特定数据的预训练数据集之间的显著分布差异;二是从内容多变的动作中捕捉一致个性的难度。为了解决数据集分布差异问题,我们引入了一个个性令牌来接受新的个性特征,并在微调过程中执行文本和视觉的多模态适应。为了捕捉一致的个性,我们采用对比学习技术来增强同一角色样本之间的内部凝聚力。此外,我们还引入了一种上下文感知融合机制,以最大限度地整合来自多个输入动作的角色线索。PersonaBooth在动作风格转换方法上表现出卓越性能,为动作个性化树立了新的基准。
论文及项目相关链接
Summary
该论文提出了Motion Personalization任务,即通过文本描述生成个性化的动作。为此,论文引入了一个名为PerMo的大型动作数据集,用于捕捉多个角色的独特个性。同时,论文还提出了一种对预训练运动扩散模型PersonaBooth的多模态微调方法。该方法解决了两个主要挑战:一是PerMo数据集与缺乏个性化数据的预训练数据集之间的分布差距;二是从内容多变的动作中捕捉一致个性的难度。为缩小数据集分布差距,引入了个性化令牌以接受新的个性化特征,并在微调过程中进行文本和视觉的多模态适应。为捕捉一致个性,采用对比学习技术增强同一角色样本之间的内部凝聚力。此外,论文还引入了上下文感知融合机制,以最大化从多个输入动作中整合个性线索。PersonaBooth在动作风格转换方法中表现出色,为运动个性化树立了新标杆。
Key Takeaways
- 引入了Motion Personalization任务,即根据文本描述生成个性化动作。
- 提出了PerMo数据集,用于捕捉多个角色的独特个性。
- 介绍了对预训练运动扩散模型PersonaBooth的多模态微调方法。
- 解决两个主要挑战:数据集分布差距和从内容多变的动作中捕捉一致个性的难度。
- 通过引入个性化令牌和新机制缩小数据集分布差距。
- 采用对比学习技术增强同一角色样本之间的内部凝聚力。
点此查看论文截图
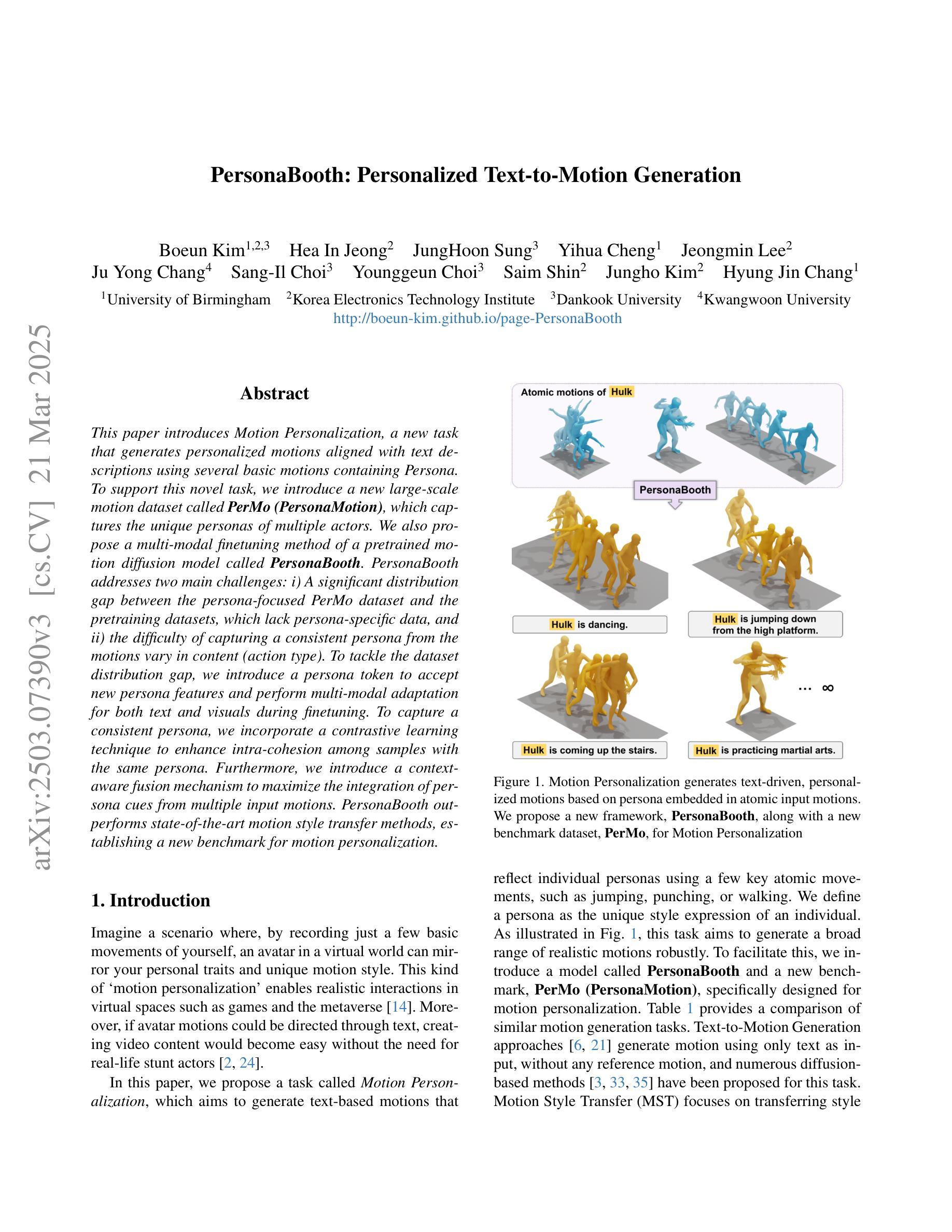
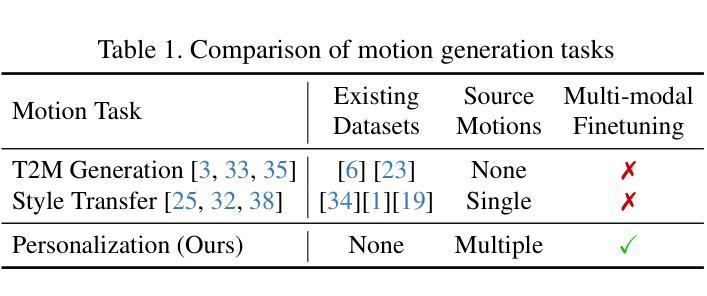
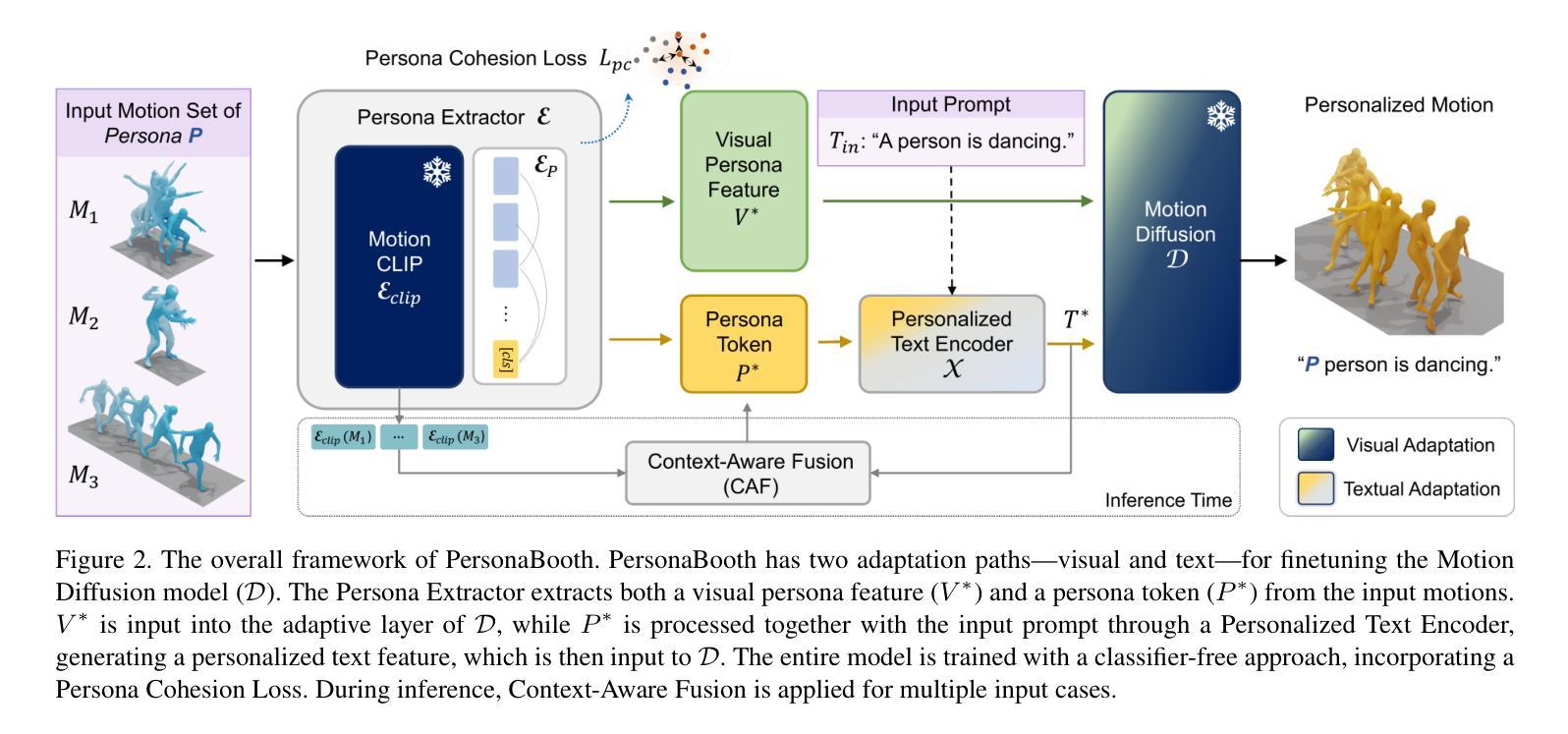
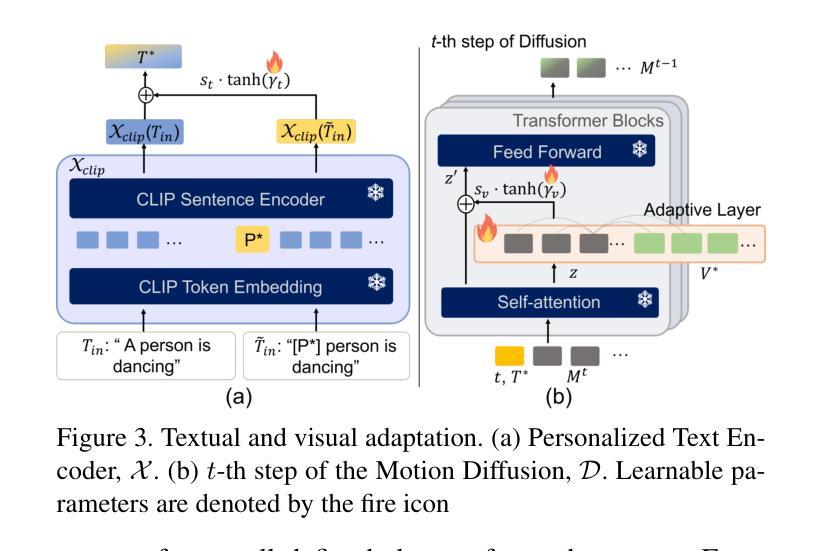
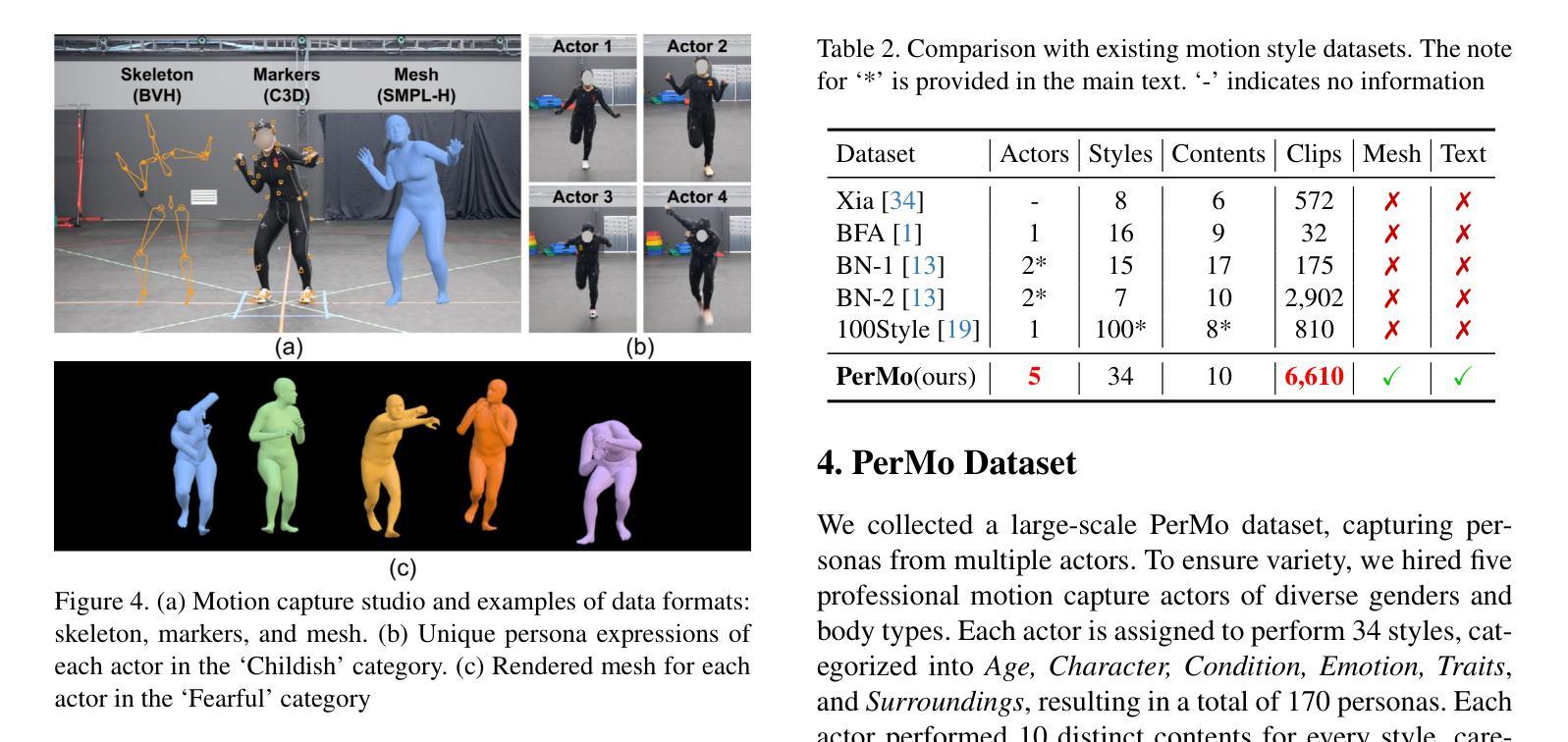
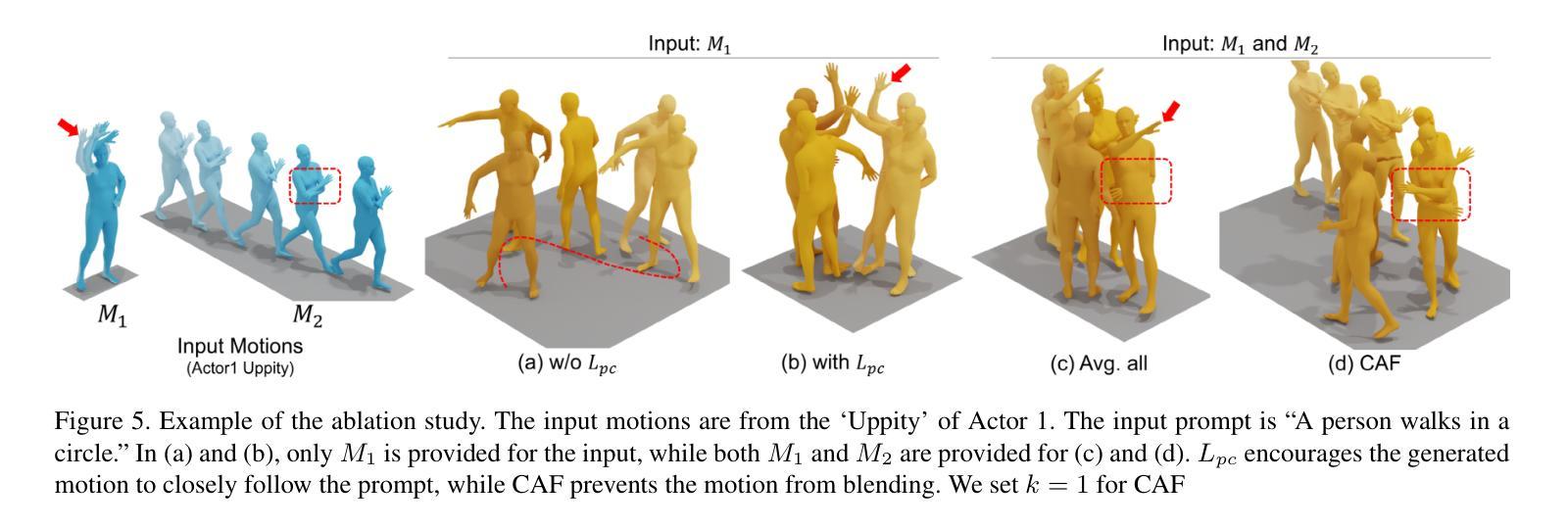
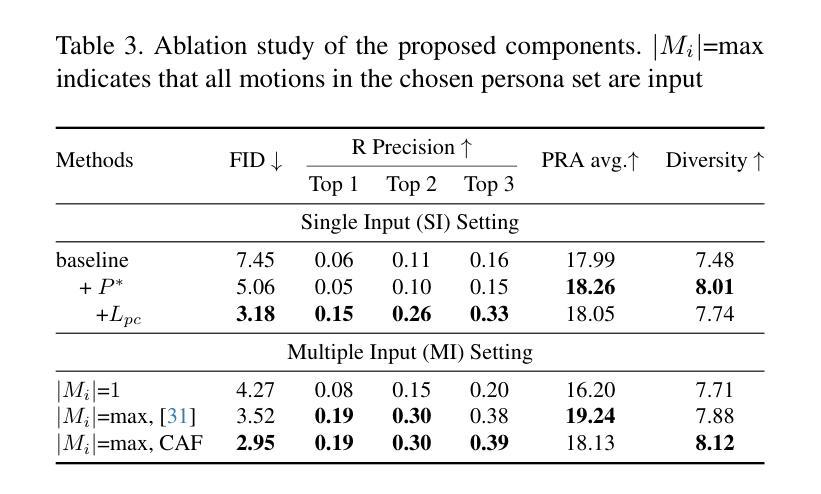