⚠️ 以下所有内容总结都来自于 大语言模型的能力,如有错误,仅供参考,谨慎使用
🔴 请注意:千万不要用于严肃的学术场景,只能用于论文阅读前的初筛!
💗 如果您觉得我们的项目对您有帮助 ChatPaperFree ,还请您给我们一些鼓励!⭐️ HuggingFace免费体验
2025-03-28 更新
Flip Learning: Weakly Supervised Erase to Segment Nodules in Breast Ultrasound
Authors:Yuhao Huang, Ao Chang, Haoran Dou, Xing Tao, Xinrui Zhou, Yan Cao, Ruobing Huang, Alejandro F Frangi, Lingyun Bao, Xin Yang, Dong Ni
Accurate segmentation of nodules in both 2D breast ultrasound (BUS) and 3D automated breast ultrasound (ABUS) is crucial for clinical diagnosis and treatment planning. Therefore, developing an automated system for nodule segmentation can enhance user independence and expedite clinical analysis. Unlike fully-supervised learning, weakly-supervised segmentation (WSS) can streamline the laborious and intricate annotation process. However, current WSS methods face challenges in achieving precise nodule segmentation, as many of them depend on inaccurate activation maps or inefficient pseudo-mask generation algorithms. In this study, we introduce a novel multi-agent reinforcement learning-based WSS framework called Flip Learning, which relies solely on 2D/3D boxes for accurate segmentation. Specifically, multiple agents are employed to erase the target from the box to facilitate classification tag flipping, with the erased region serving as the predicted segmentation mask. The key contributions of this research are as follows: (1) Adoption of a superpixel/supervoxel-based approach to encode the standardized environment, capturing boundary priors and expediting the learning process. (2) Introduction of three meticulously designed rewards, comprising a classification score reward and two intensity distribution rewards, to steer the agents’ erasing process precisely, thereby avoiding both under- and over-segmentation. (3) Implementation of a progressive curriculum learning strategy to enable agents to interact with the environment in a progressively challenging manner, thereby enhancing learning efficiency. Extensively validated on the large in-house BUS and ABUS datasets, our Flip Learning method outperforms state-of-the-art WSS methods and foundation models, and achieves comparable performance as fully-supervised learning algorithms.
二维乳腺超声(BUS)和三维自动乳腺超声(ABUS)中的结节精确分割对于临床诊断和治疗计划至关重要。因此,开发用于结节分割的自动化系统可以提高用户独立性并加速临床分析。与完全监督学习不同,弱监督分割(WSS)可以简化繁琐且复杂的注释过程。然而,当前的WSS方法在实现精确结节分割方面面临挑战,因为它们中的许多方法依赖于不准确的激活图或低效的伪掩膜生成算法。在本研究中,我们引入了一种基于多智能体强化学习的新型WSS框架,称为Flip Learning,它仅依赖于2D/3D框进行精确分割。具体来说,使用多个智能体从框中擦除目标,以促进分类标签翻转,擦除区域作为预测的分割掩膜。本研究的关键贡献如下:(1)采用基于超像素/监督体素的方法对标准化环境进行编码,捕获边界先验信息并加速学习过程。(2)引入了三种精心设计的奖励,包括分类得分奖励和两个强度分布奖励,以精确引导智能体的擦除过程,从而避免欠分割和过分割。(3)实现了一种渐进的课程学习策略,使智能体能够以渐进挑战的方式与环境进行交互,从而提高学习效率。在大型内部BUS和ABUS数据集上进行了广泛验证,我们的Flip Learning方法优于最新的WSS方法和基础模型,并且其性能与完全监督学习算法相当。
论文及项目相关链接
PDF Accepted by Medical Image Analysis. 24 pages, 13 figures, 18 tabels
Summary
本文介绍了一种基于多智能体强化学习的新型弱监督分割(WSS)框架——Flip Learning,用于在二维和三维超声图像中进行乳腺结节分割。Flip Learning仅利用二维或三维框进行精确分割,通过多智能体擦除目标促进分类标签翻转,并利用精心设计的奖励和渐进的课程学习策略来提高学习效果。在大量内部BUS和ABUS数据集上的验证表明,Flip Learning方法优于现有的WSS方法和基础模型,并与全监督学习算法的性能相当。
Key Takeaways
- Flip Learning是一种基于多智能体强化学习的弱监督分割(WSS)框架,用于乳腺结节的精确分割。
- Flip Learning仅使用二维或三维框进行分割,简化了繁琐的标注过程。
- 通过多智能体擦除目标并促进分类标签翻转,生成预测分割掩膜。
- 采用超像素/超体素方法编码标准化环境,提高学习效率。
- 引入三种精心设计的奖励,包括分类得分奖励和两个强度分布奖励,以精确引导智能体的擦除过程。
- 实施渐进的课程学习策略,使智能体能够以渐进的方式与环境互动,提高学习效率。
点此查看论文截图
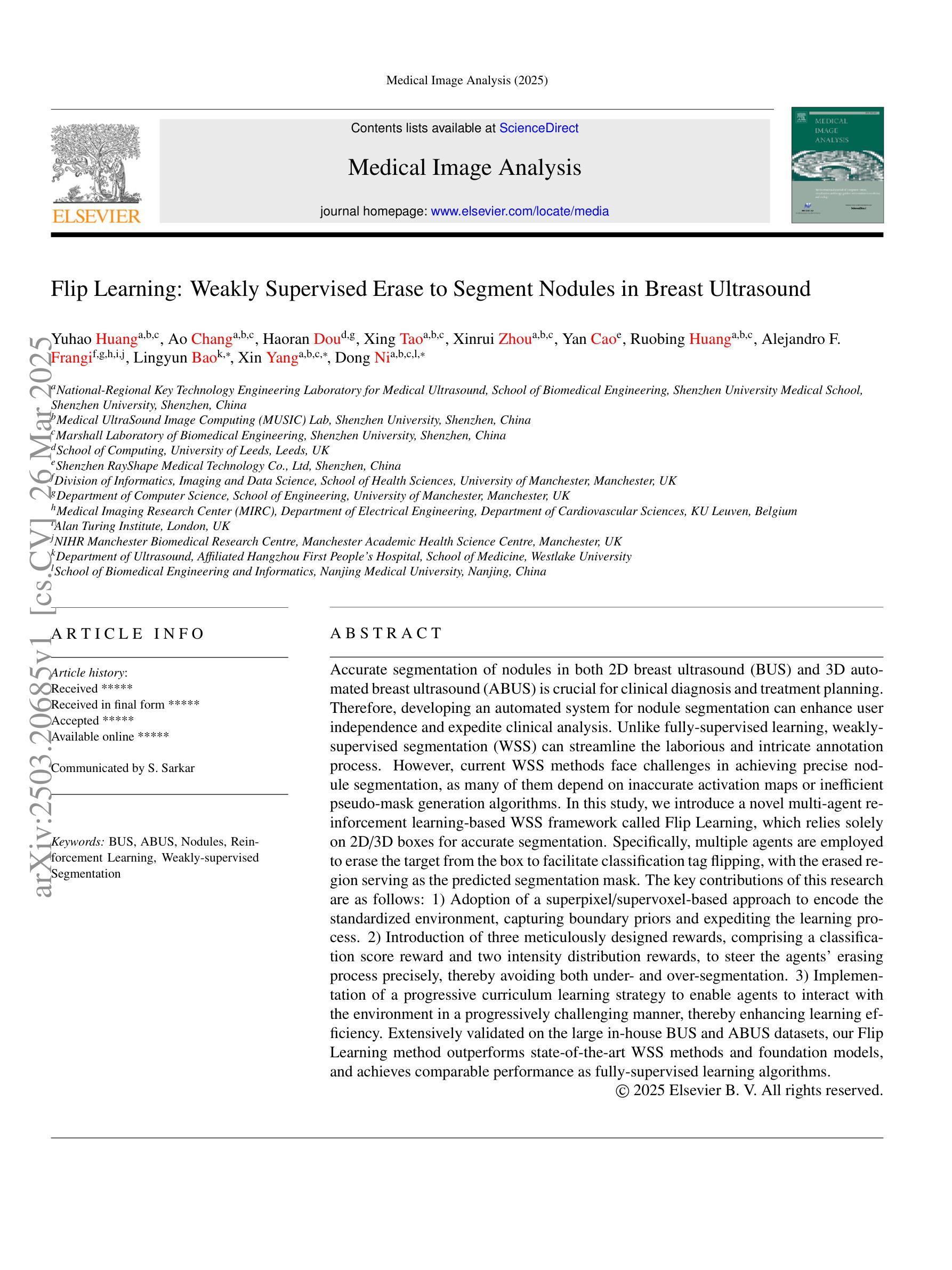
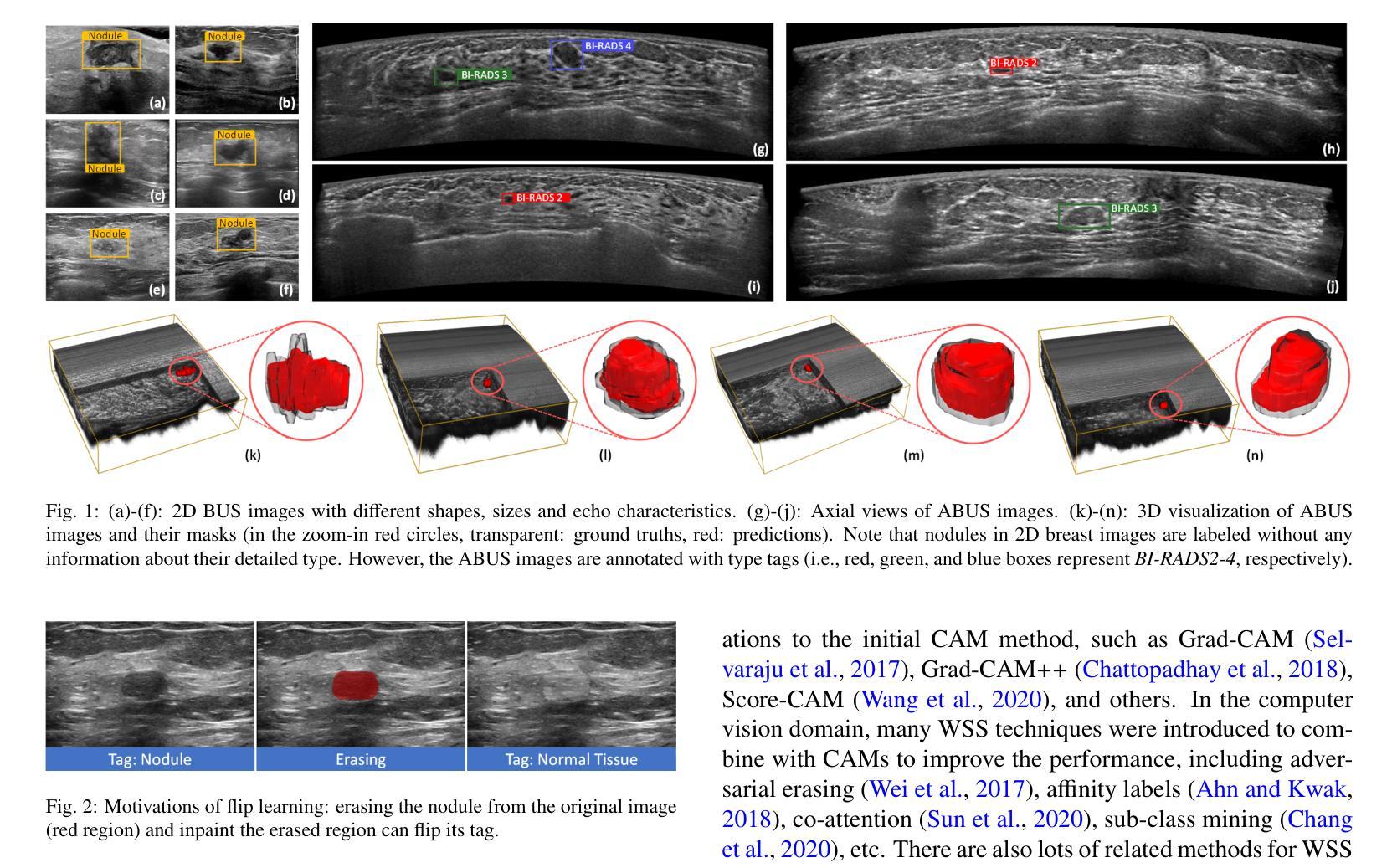
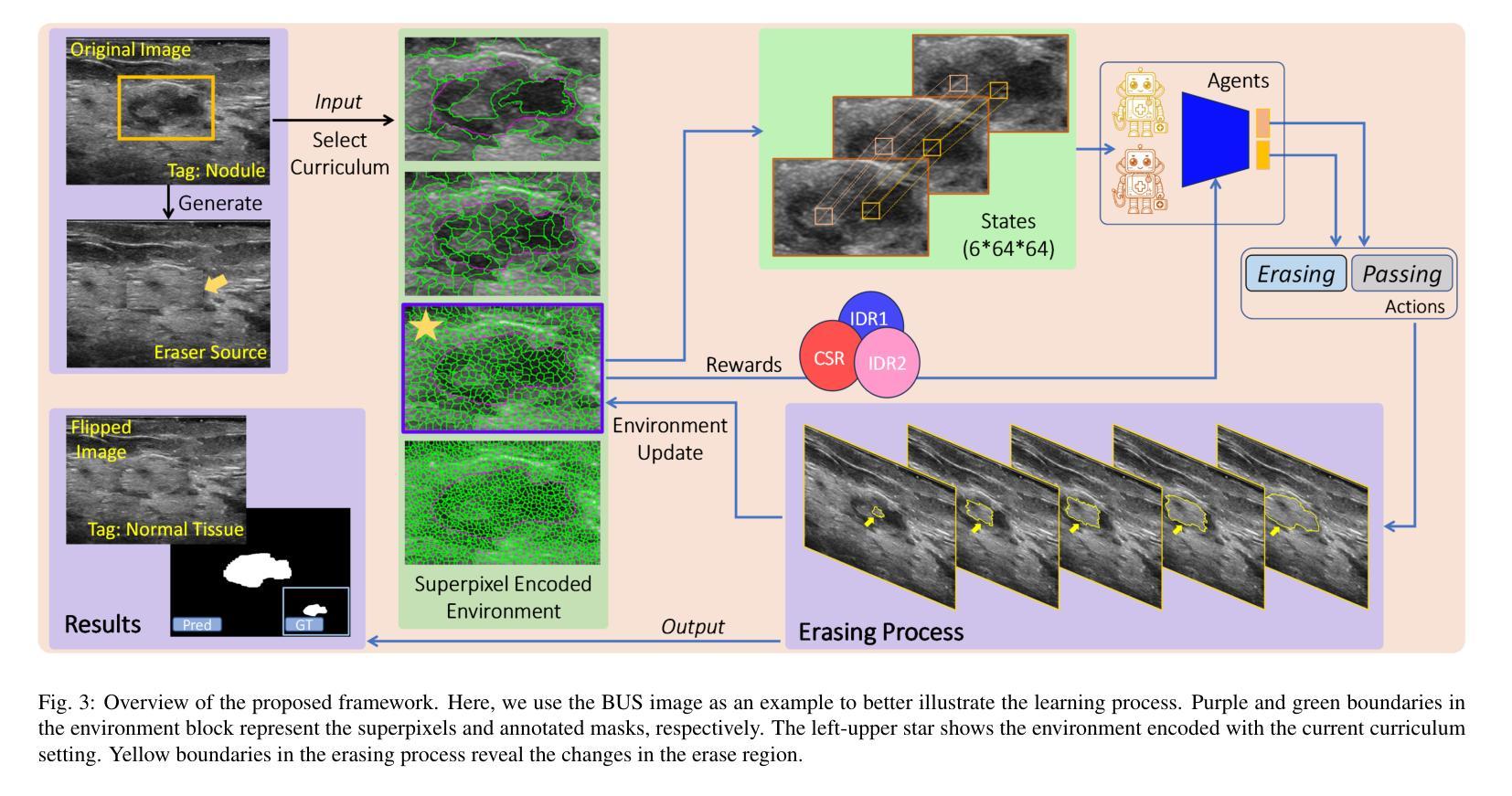
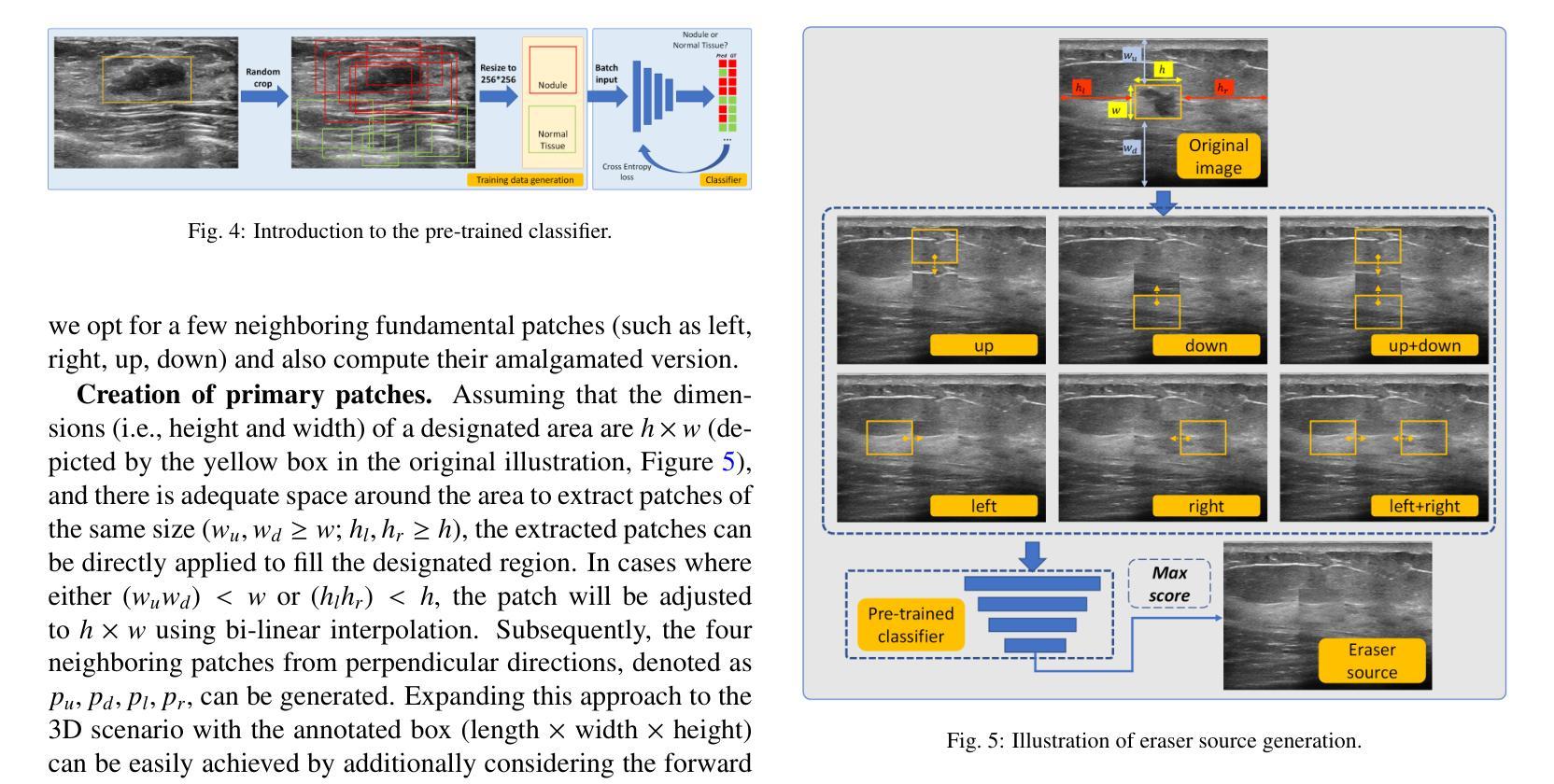