⚠️ 以下所有内容总结都来自于 大语言模型的能力,如有错误,仅供参考,谨慎使用
🔴 请注意:千万不要用于严肃的学术场景,只能用于论文阅读前的初筛!
💗 如果您觉得我们的项目对您有帮助 ChatPaperFree ,还请您给我们一些鼓励!⭐️ HuggingFace免费体验
2025-03-28 更新
Cross-Modal Prototype Allocation: Unsupervised Slide Representation Learning via Patch-Text Contrast in Computational Pathology
Authors:Yuxuan Chen, Jiawen Li, Jiali Hu, Xitong Ling, Tian Guan, Anjia Han, Yonghong He
With the rapid advancement of pathology foundation models (FMs), the representation learning of whole slide images (WSIs) attracts increasing attention. Existing studies develop high-quality patch feature extractors and employ carefully designed aggregation schemes to derive slide-level representations. However, mainstream weakly supervised slide representation learning methods, primarily based on multiple instance learning (MIL), are tailored to specific downstream tasks, which limits their generalizability. To address this issue, some studies explore unsupervised slide representation learning. However, these approaches focus solely on the visual modality of patches, neglecting the rich semantic information embedded in textual data. In this work, we propose ProAlign, a cross-modal unsupervised slide representation learning framework. Specifically, we leverage a large language model (LLM) to generate descriptive text for the prototype types present in a WSI, introducing patch-text contrast to construct initial prototype embeddings. Furthermore, we propose a parameter-free attention aggregation strategy that utilizes the similarity between patches and these prototypes to form unsupervised slide embeddings applicable to a wide range of downstream tasks. Extensive experiments on four public datasets show that ProAlign outperforms existing unsupervised frameworks and achieves performance comparable to some weakly supervised models.
随着病理学基础模型(FMs)的快速发展,全切片图像(WSIs)的表示学习引起了越来越多的关注。现有研究开发了高质量的小块特征提取器,并采用了精心设计的聚合方案来推导切片级别的表示。然而,主流的弱监督切片表示学习方法主要基于多实例学习(MIL),这些方法针对特定的下游任务进行定制,从而限制了其通用性。为了解决这一问题,一些研究探索了无监督的切片表示学习方法。然而,这些方法仅专注于小块的视觉模式,忽略了文本数据中嵌入的丰富语义信息。在这项工作中,我们提出了ProAlign,一个跨模态无监督的切片表示学习框架。具体来说,我们利用大型语言模型(LLM)为WSI中存在的原型类型生成描述性文本,引入小块文本对比来构建初始原型嵌入。此外,我们提出了一种无需参数的无监督注意力聚合策略,该策略利用小块与这些原型之间的相似性来形成适用于各种下游任务的无监督切片嵌入。在四个公共数据集上的大量实验表明,ProAlign在现有无监督框架的基础上表现出更高的性能,并且在某些弱监督模型上取得了相当的性能。
论文及项目相关链接
PDF 11pages,3 figures
Summary
该研究关注于病理模型中的无监督表示学习问题。为了提升对全切片图像(WSI)的表示能力,现有研究集中在高质量的补丁特征提取和精心设计的数据聚合方案上。尽管现有的基于多实例学习(MIL)的弱监督方法在某些任务上表现良好,但其针对特定任务的特性限制了其泛化能力。针对此问题,有些研究探索无监督的方法但只聚焦于补丁的视觉模态而忽略了文本数据中的丰富语义信息。本文提出一个跨模态的无监督幻灯片表示学习框架ProAlign,利用大型语言模型(LLM)生成WSI中原型类型的描述性文本,引入补丁文本对比来构建初始原型嵌入。此外,本研究还提出了一种无参数注意力聚合策略,利用补丁与这些原型的相似性形成适用于多种下游任务的无监督幻灯片嵌入。在四个公共数据集上的实验表明,ProAlign优于现有的无监督框架,并实现了与某些弱监督模型相当的性能。
Key Takeaways
- 现有研究在病理模型中的全切片图像表示学习方面取得了进展,但仍面临泛化能力的问题。
- 基于多实例学习(MIL)的弱监督方法具有任务特定性,限制了其泛化能力。
- 研究提出了跨模态的无监督幻灯片表示学习框架ProAlign。
- ProAlign使用大型语言模型(LLM)生成描述性文本以增强补丁特征的学习。
- ProAlign引入补丁文本对比来构建初始原型嵌入,并通过无参数注意力聚合策略形成适用于多种下游任务的幻灯片嵌入。
- ProAlign在多个公共数据集上的性能优于现有无监督框架,并与某些弱监督模型相当。
点此查看论文截图
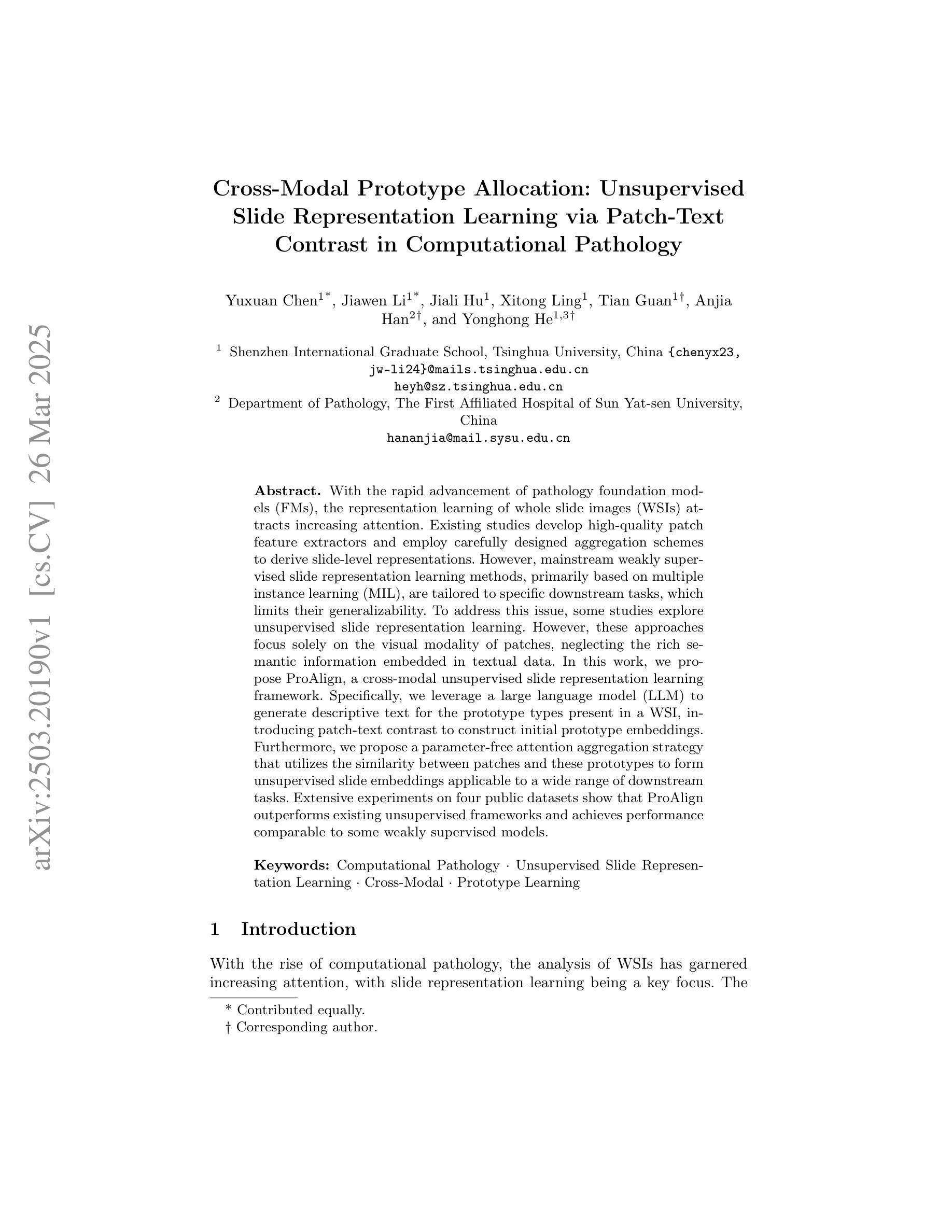
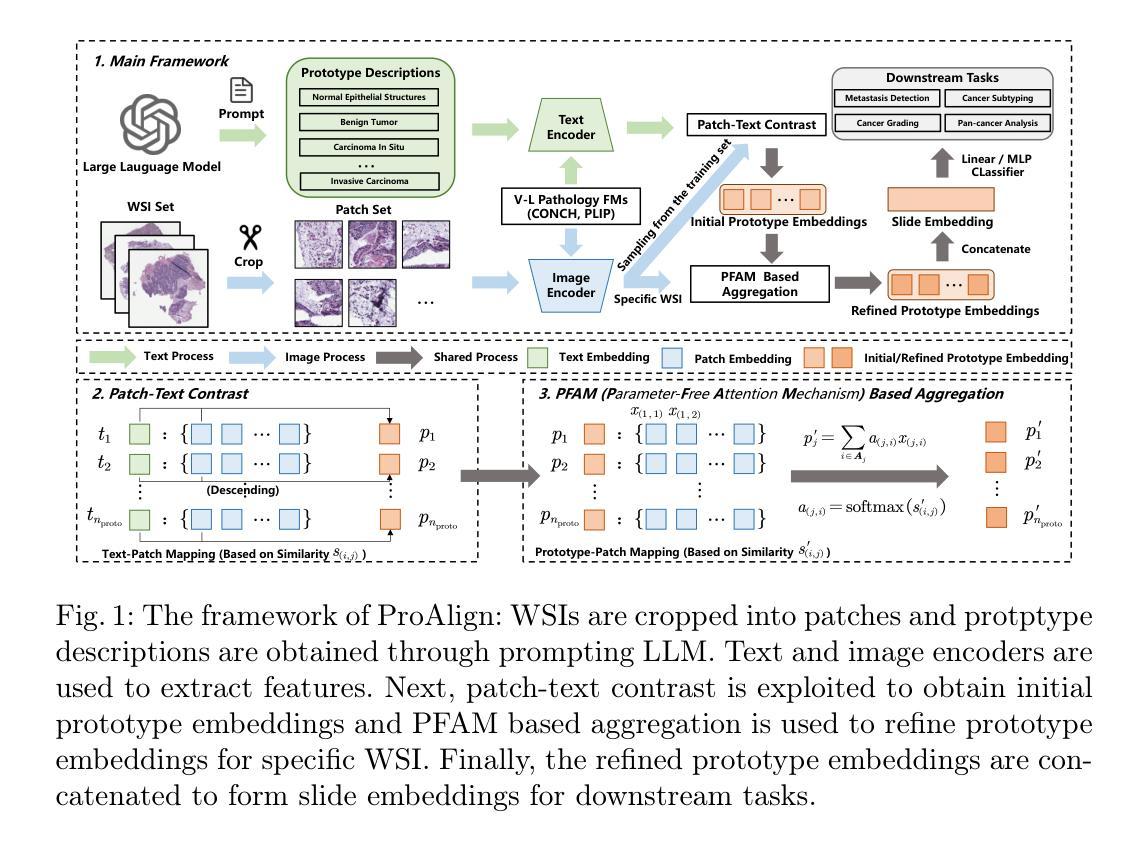
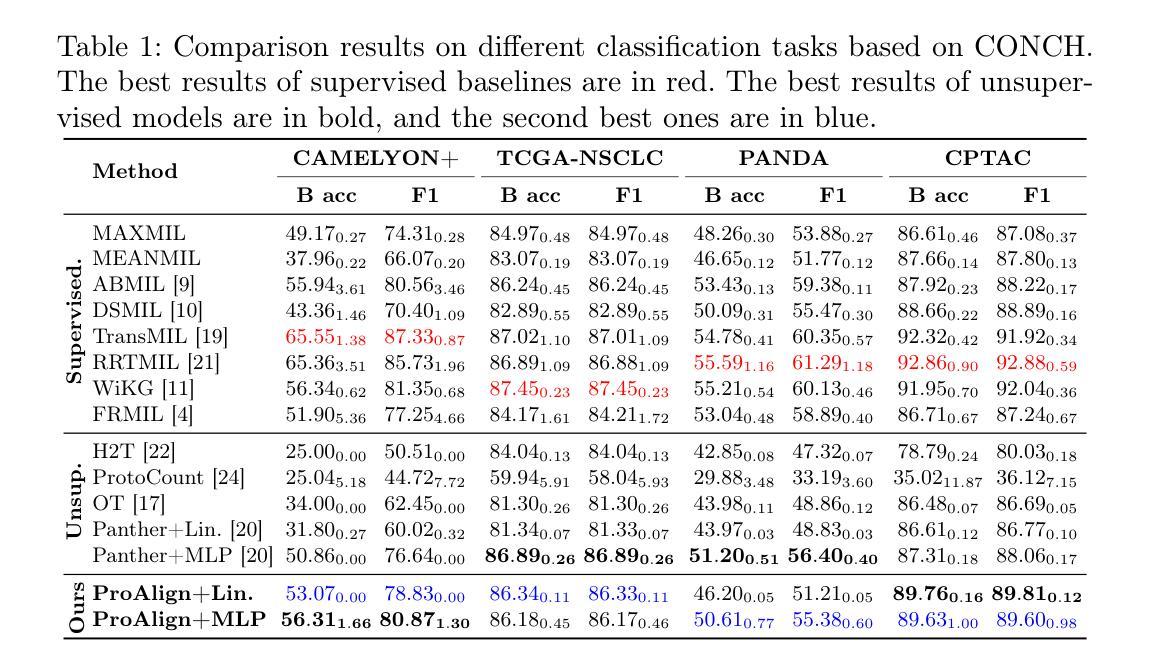
Similarity-Dissimilarity Loss for Multi-label Supervised Contrastive Learning
Authors:Guangming Huang, Yunfei Long, Cunjin Luo
Supervised contrastive learning has achieved remarkable success by leveraging label information; however, determining positive samples in multi-label scenarios remains a critical challenge. In multi-label supervised contrastive learning (MSCL), relations among multi-label samples are not yet fully defined, leading to ambiguity in identifying positive samples and formulating contrastive loss functions to construct the representation space. To address these challenges, we: (i) first define five distinct multi-label relations in MSCL to systematically identify positive samples, (ii) introduce a novel Similarity-Dissimilarity Loss that dynamically re-weights samples through computing the similarity and dissimilarity factors between positive samples and given anchors based on multi-label relations, and (iii) further provide theoretical grounded proof for our method through rigorous mathematical analysis that supports the formulation and effectiveness of the proposed loss function. We conduct the experiments across both image and text modalities, and extend the evaluation to medical domain. The results demonstrate that our method consistently outperforms baselines in a comprehensive evaluation, confirming its effectiveness and robustness. Code is available at: https://github.com/guangminghuang/similarity-dissimilarity-loss.
有监督对比学习通过利用标签信息取得了显著的成功,但在多标签场景中确定正样本仍然是一个关键挑战。在多标签有监督对比学习(MSCL)中,多标签样本之间的关系尚未被完全定义,这导致在识别正样本和制定对比损失函数以构建表示空间时存在模糊性。为了解决这些挑战,我们:(i)首先在MSCL中定义了五种不同的多标签关系,以系统地识别正样本,(ii)引入了一种新的相似性-差异性损失,通过计算正样本与给定锚点之间的相似性和差异性因素来动态重新加权样本,这基于多标签关系,(iii)通过严格的数学分析为我们的方法提供了理论上的证明,这支持了所提出的损失函数的形成和有效性。我们在图像和文本模态上进行了实验,并将评估扩展到了医疗领域。结果表明,我们的方法在综合评估中始终优于基线,证实了其有效性和稳健性。代码可用在:https://github.com/guangminghuang/similarity-dissimilarity-loss。
论文及项目相关链接
Summary
多标签监督对比学习(MSCL)在利用标签信息方面取得了显著成功,但确定多标签场景中的正样本仍存在挑战。本文定义了五种多标签关系,以系统地识别正样本,并引入了一种新的相似度-差异度损失,该损失通过计算正样本与给定锚点之间的相似度和差异度因子来动态重新加权样本。实验证明,该方法在图像和文本模态以及医学领域均表现优异,且较基线方法更有效和稳健。
Key Takeaways
- 多标签监督对比学习(MSCL)在利用标签信息上取得了显著成果,但仍面临确定正样本的挑战。
- 提出了五种多标签关系的定义,以更系统地识别正样本。
- 引入了一种新的相似度-差异度损失,该损失能基于多标签关系动态重新加权样本。
- 提供了理论证明,并通过严格的数学分析支持了所提损失函数的有效性。
- 实验证明该方法在图像和文本模态以及医学领域均有效。
- 与基线方法相比,该方法表现更稳健和有效。
点此查看论文截图
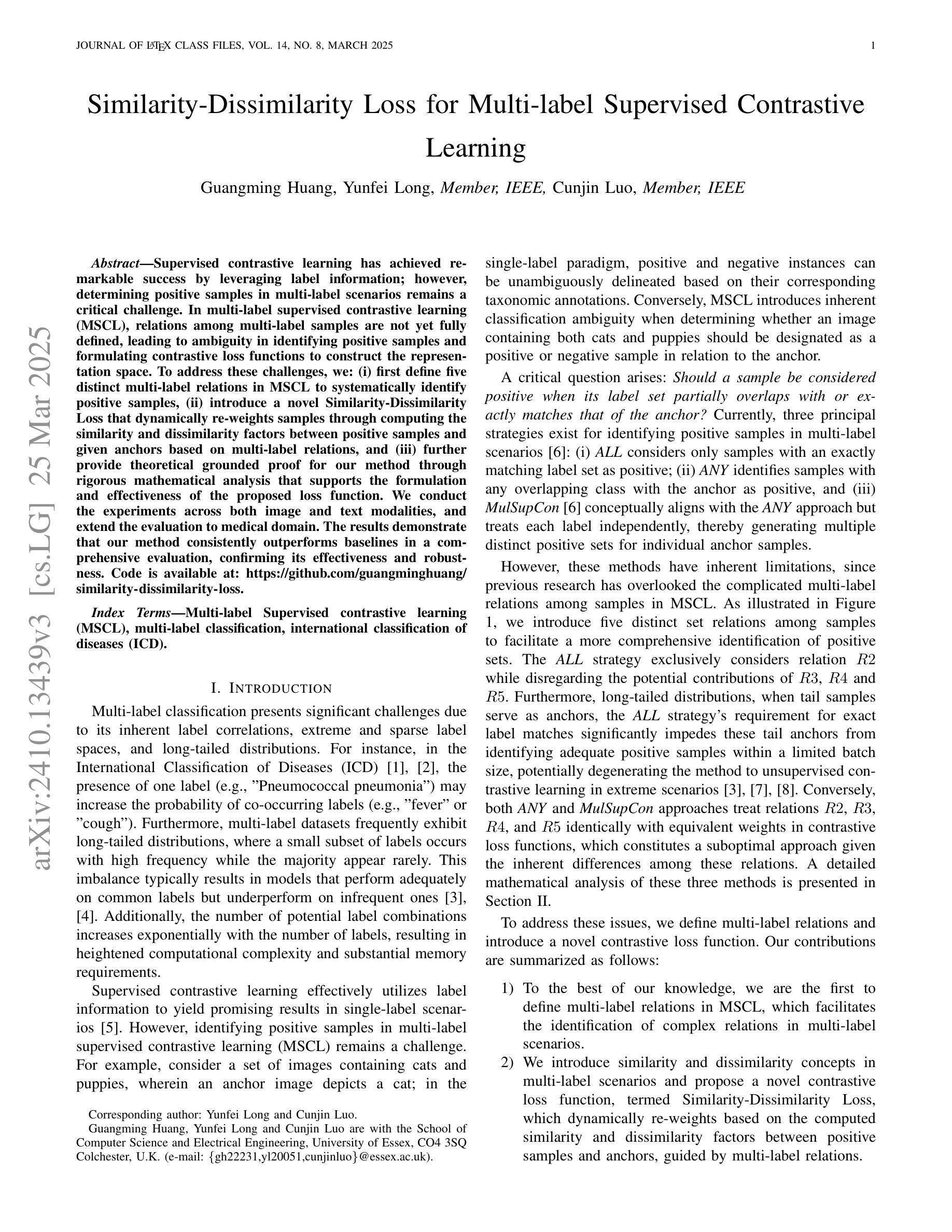
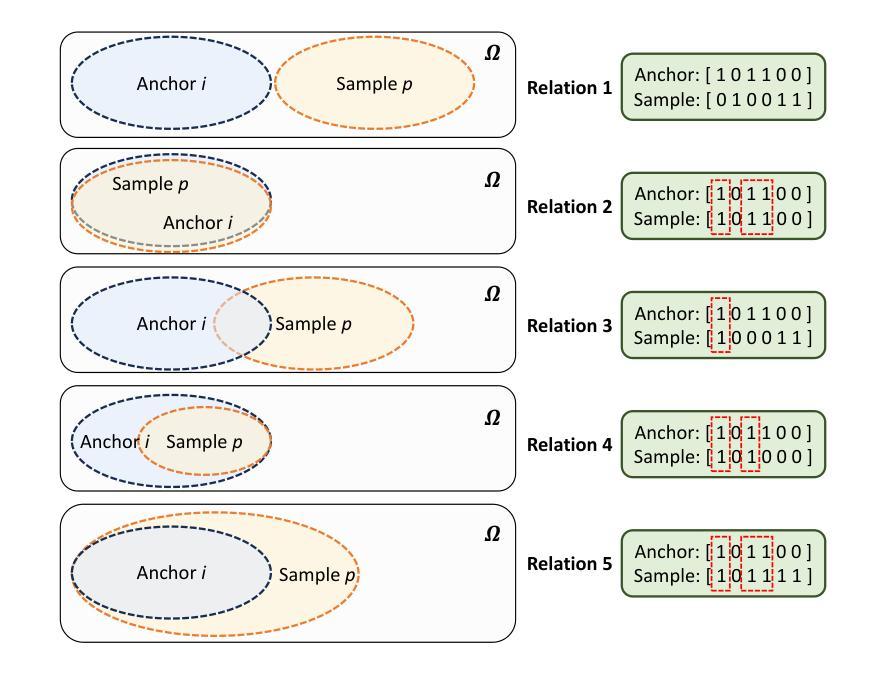
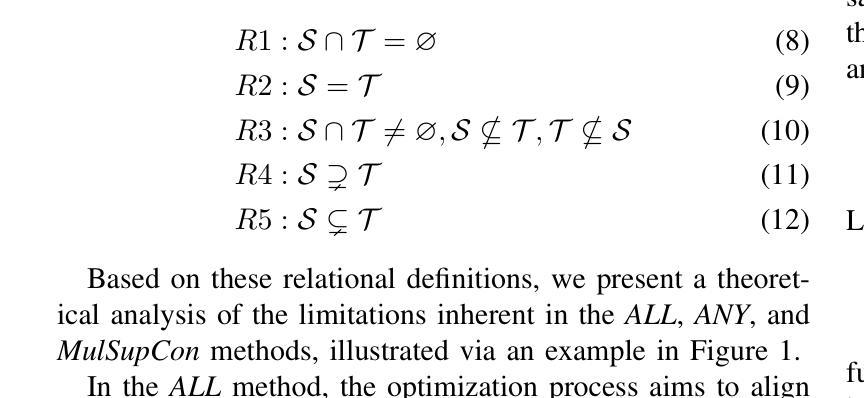