⚠️ 以下所有内容总结都来自于 大语言模型的能力,如有错误,仅供参考,谨慎使用
🔴 请注意:千万不要用于严肃的学术场景,只能用于论文阅读前的初筛!
💗 如果您觉得我们的项目对您有帮助 ChatPaperFree ,还请您给我们一些鼓励!⭐️ HuggingFace免费体验
2025-03-28 更新
ACVUBench: Audio-Centric Video Understanding Benchmark
Authors:Yudong Yang, Jimin Zhuang, Guangzhi Sun, Changli Tang, Yixuan Li, Peihan Li, Yifan Jiang, Wei Li, Zejun Ma, Chao Zhang
Audio often serves as an auxiliary modality in video understanding tasks of audio-visual large language models (LLMs), merely assisting in the comprehension of visual information. However, a thorough understanding of videos significantly depends on auditory information, as audio offers critical context, emotional cues, and semantic meaning that visual data alone often lacks. This paper proposes an audio-centric video understanding benchmark (ACVUBench) to evaluate the video comprehension capabilities of multimodal LLMs with a particular focus on auditory information. Specifically, ACVUBench incorporates 2,662 videos spanning 18 different domains with rich auditory information, together with over 13k high-quality human annotated or validated question-answer pairs. Moreover, ACVUBench introduces a suite of carefully designed audio-centric tasks, holistically testing the understanding of both audio content and audio-visual interactions in videos. A thorough evaluation across a diverse range of open-source and proprietary multimodal LLMs is performed, followed by the analyses of deficiencies in audio-visual LLMs. Demos are available at https://github.com/lark-png/ACVUBench.
音频通常在视听大型语言模型(LLM)的视频理解任务中作为辅助模态,仅帮助理解视觉信息。然而,对视频的全面理解在很大程度上依赖于听觉信息,因为音频提供了关键上下文、情感线索和语义意义,而这些往往是视觉数据所缺乏的。本文针对视频理解的多模态LLM能力评估,提出了一种以音频为中心的视频理解基准测试(ACVUBench)。具体来说,ACVUBench集成了涵盖18个不同领域的2662个视频,拥有丰富的听觉信息,以及超过13k个高质量的人类标注或验证的问答对。此外,ACVUBench引入了一套精心设计的以音频为中心的任务,全面测试视频中的音频内容和视听交互的理解能力。在开源和专有多模态LLM上进行了一系列全面的评估,并对视听LLM的缺陷进行了分析。演示地址是:https://github.com/lark-png/ACVUBench。
论文及项目相关链接
Summary
本文提出一个以音频为中心的视频理解基准测试(ACVUBench),旨在评估多模态大型语言模型对视频的理解能力,特别是音频信息。ACVUBench包含18个不同领域的2662个视频和超过1.3万个高质量的人工标注或验证的问题答案对。它提供一系列精心设计以音频为中心的任务,全面测试视频中的音频内容和视听交互的理解。
Key Takeaways
- 音频在多模态大型语言模型(LLM)的视频理解任务中扮演重要角色,提供关键上下文、情感线索和语义意义。
- 提出一个以音频为中心的视频理解基准测试(ACVUBench),旨在评估LLM对视频的理解能力。
- ACVUBench包含来自不同领域的丰富视频资源,涵盖多种类型的音频信息。
- 提供一系列以音频为中心的任务,全面测试LLM对视频中的音频内容和视听交互的理解。
- 通过在多种开源和专有LLM上的全面评估,发现其在音视频理解上的不足。
- 提供演示以展示ACVUBench的功能和使用方法。
点此查看论文截图
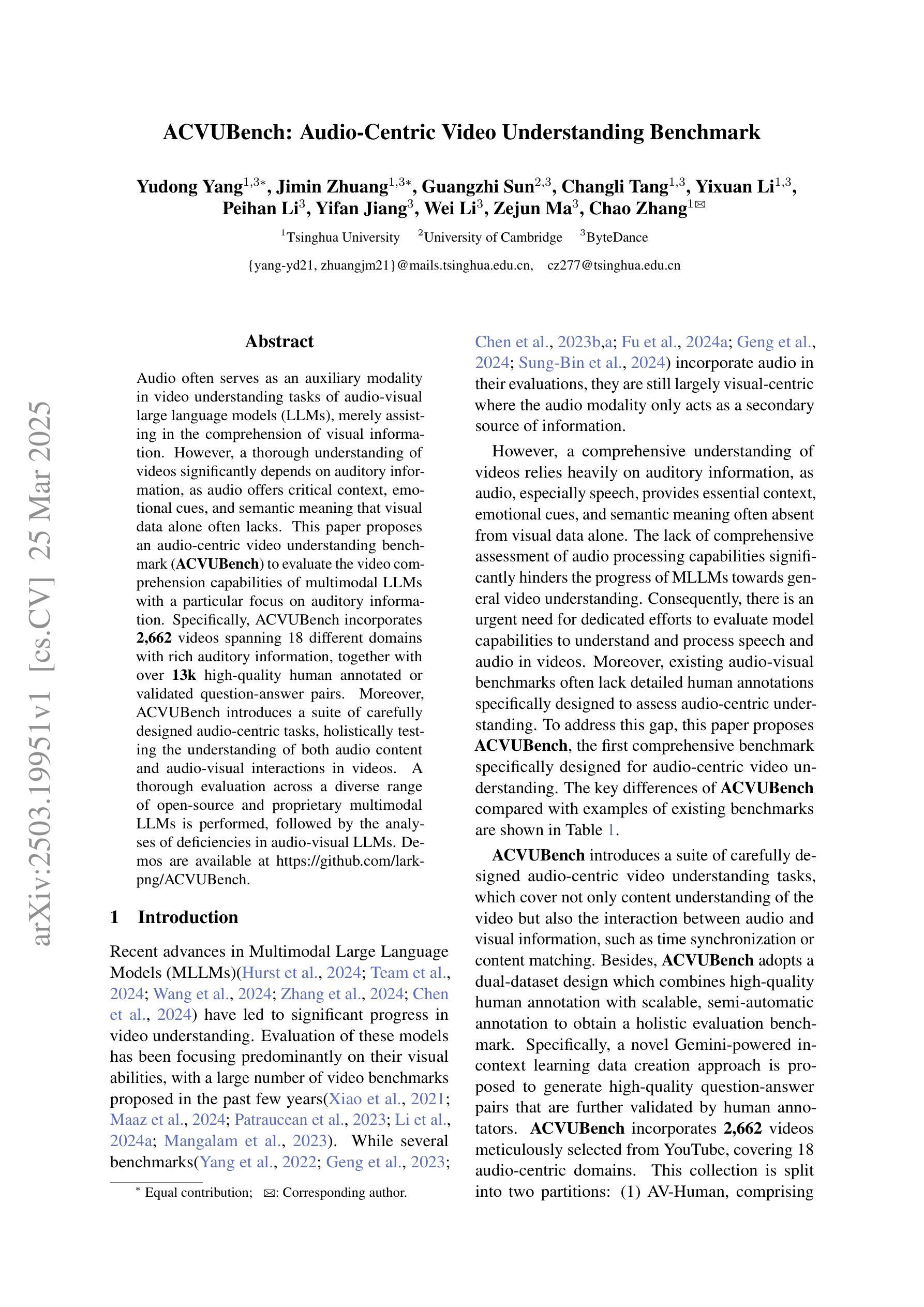
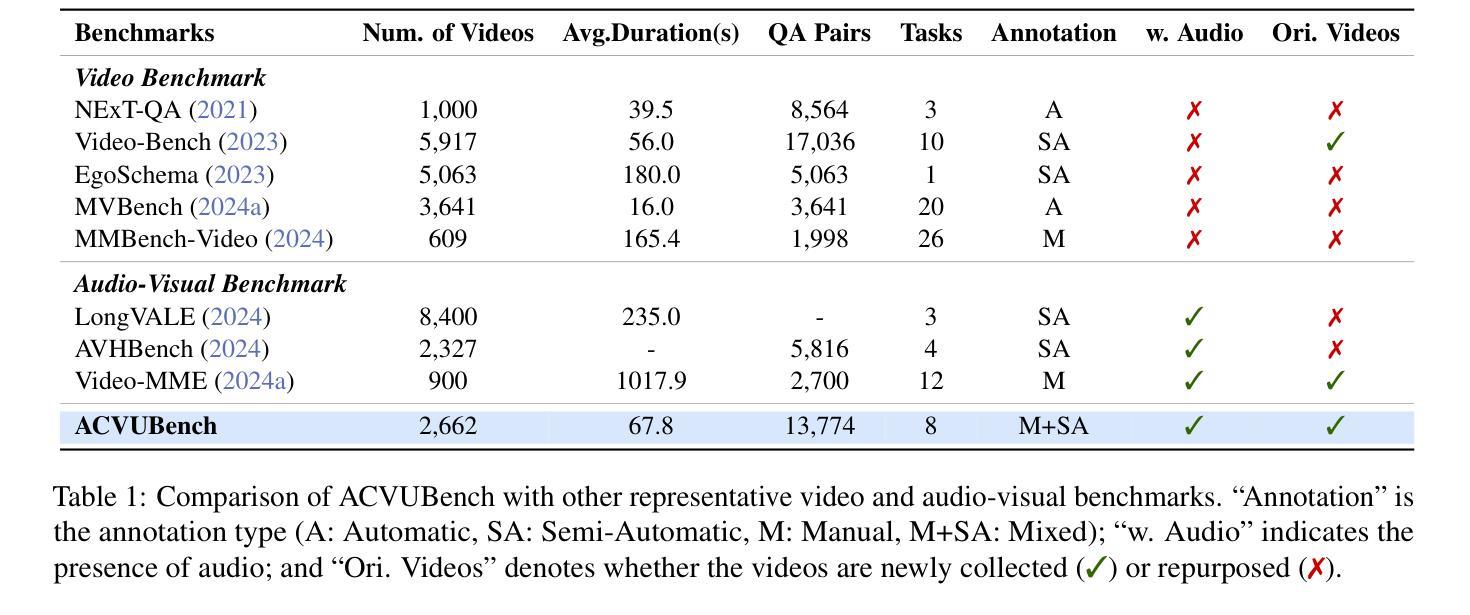
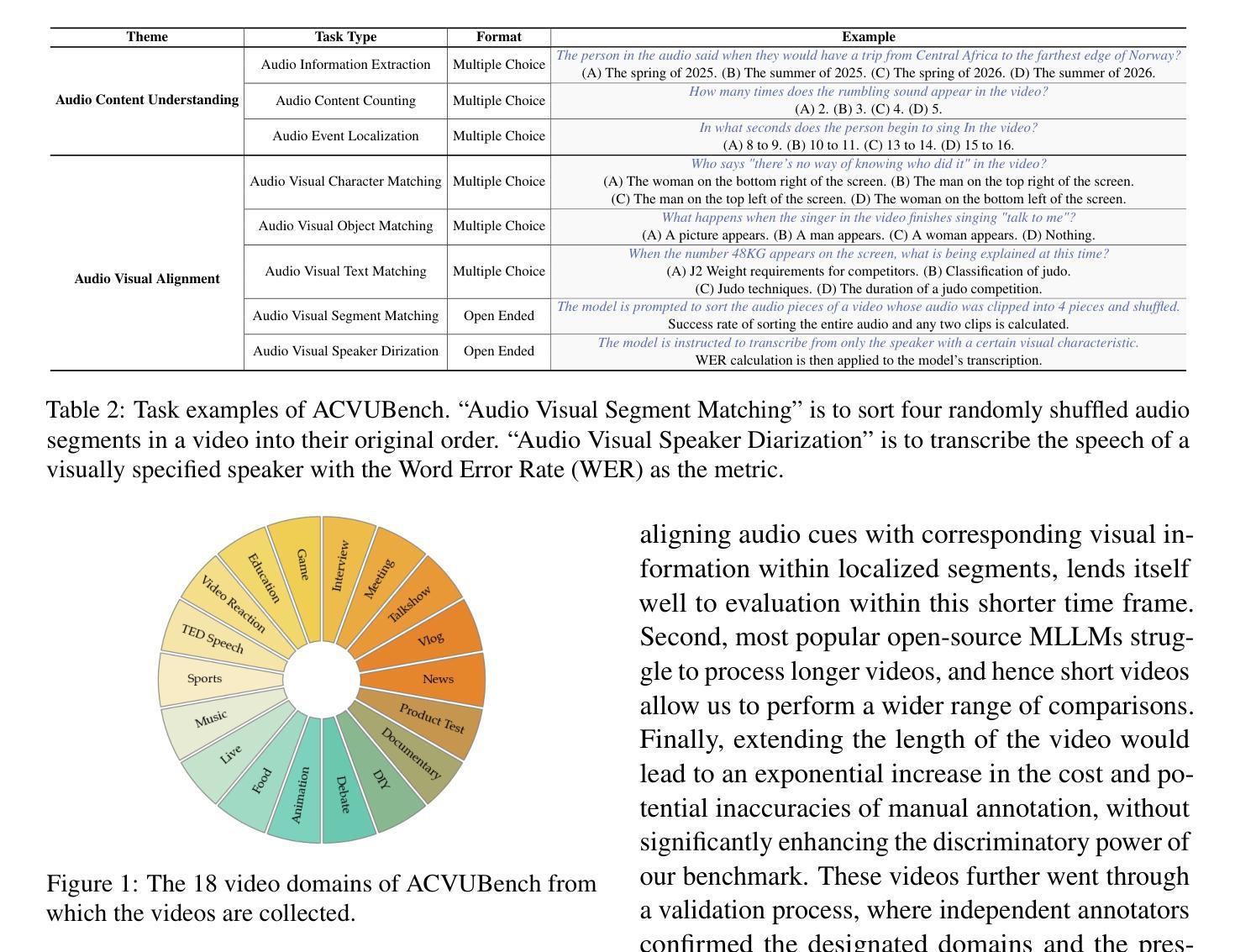
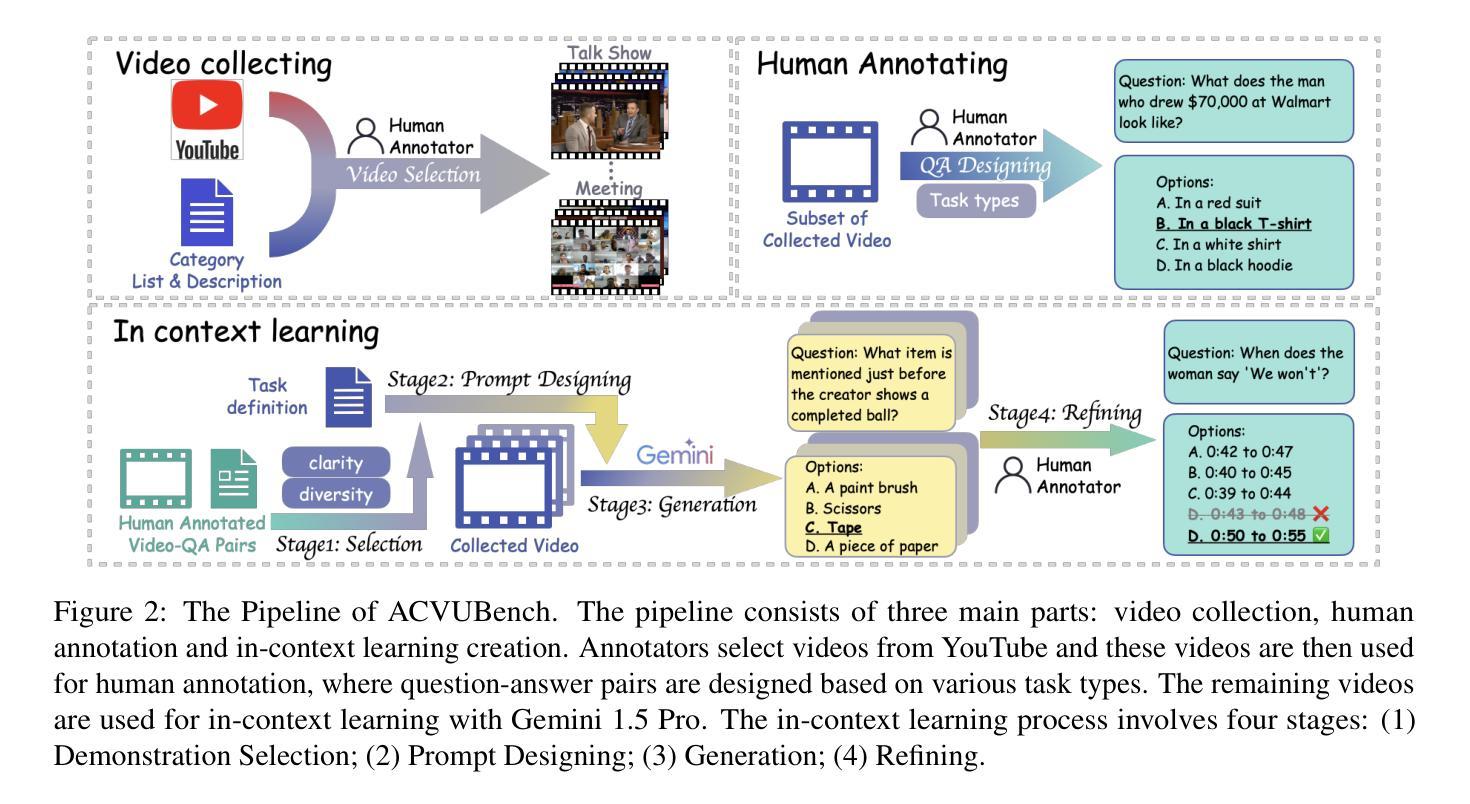
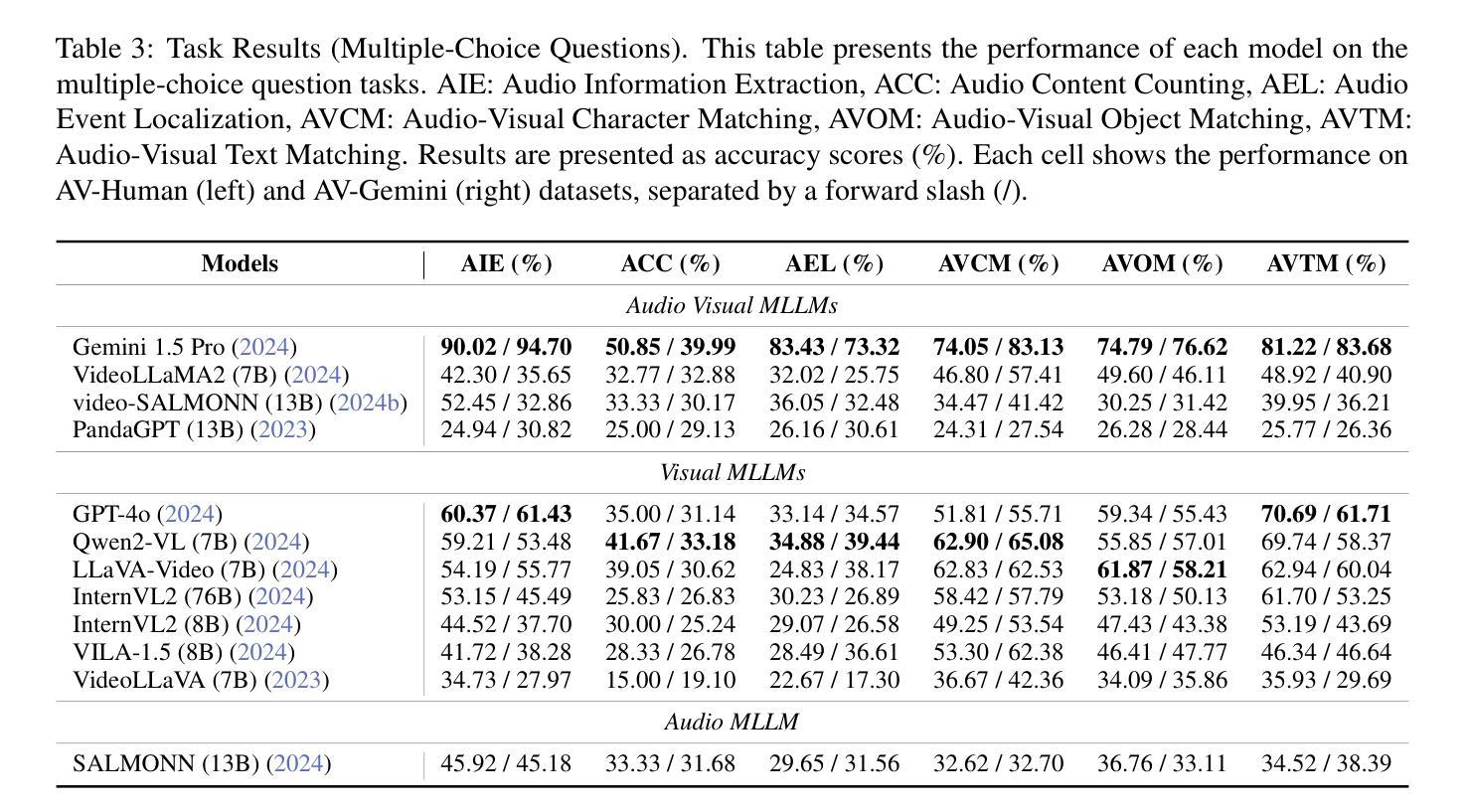
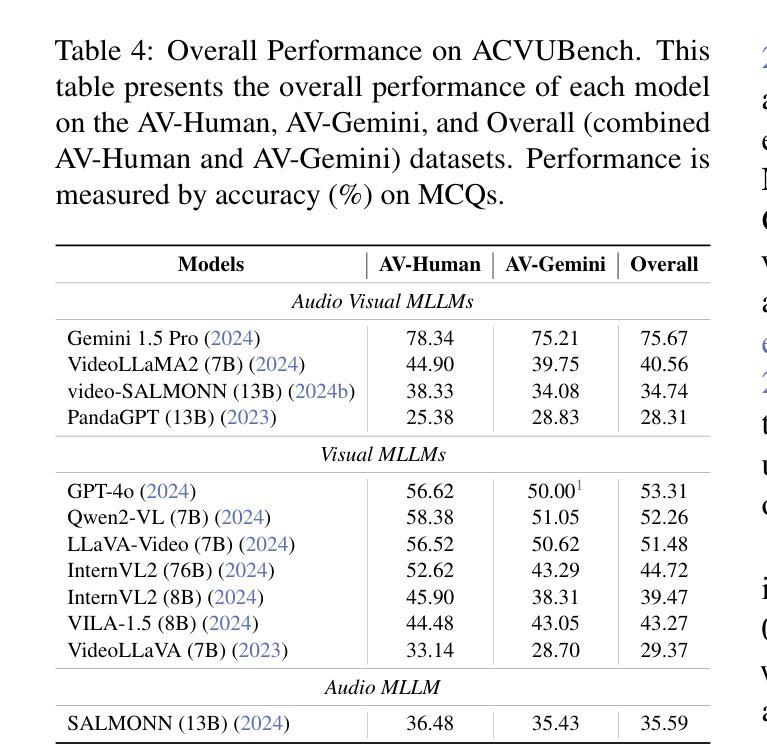
HLV-1K: A Large-scale Hour-Long Video Benchmark for Time-Specific Long Video Understanding
Authors:Heqing Zou, Tianze Luo, Guiyang Xie, Victor, Zhang, Fengmao Lv, Guangcong Wang, Junyang Chen, Zhuochen Wang, Hansheng Zhang, Huaijian Zhang
Multimodal large language models have become a popular topic in deep visual understanding due to many promising real-world applications. However, hour-long video understanding, spanning over one hour and containing tens of thousands of visual frames, remains under-explored because of 1) challenging long-term video analyses, 2) inefficient large-model approaches, and 3) lack of large-scale benchmark datasets. Among them, in this paper, we focus on building a large-scale hour-long long video benchmark, HLV-1K, designed to evaluate long video understanding models. HLV-1K comprises 1009 hour-long videos with 14,847 high-quality question answering (QA) and multi-choice question asnwering (MCQA) pairs with time-aware query and diverse annotations, covering frame-level, within-event-level, cross-event-level, and long-term reasoning tasks. We evaluate our benchmark using existing state-of-the-art methods and demonstrate its value for testing deep long video understanding capabilities at different levels and for various tasks. This includes promoting future long video understanding tasks at a granular level, such as deep understanding of long live videos, meeting recordings, and movies.
多模态大型语言模型由于许多有前途的现实世界应用,已成为深度视觉理解中的热门话题。然而,长达一小时的视频理解,涉及超过一小时的内容,包含数万个视频帧,仍然未被充分探索,原因在于1)具有挑战性的长期视频分析,2)低效的大型模型方法,以及3)缺乏大规模基准数据集。本文中,我们专注于构建大规模一小时长视频基准HLV-1K,旨在评估长视频理解模型。HLV-1K包含1009个长达一小时的视频,有14847个高质量的问答(QA)和多项选择问答(MCQA)对,包括时间感知查询和多样注释,涵盖帧级、事件内级、跨事件级和长期推理任务。我们使用现有的最先进方法评估我们的基准测试,并展示了其在测试不同层次和不同任务深度长视频理解能力方面的价值。这包括促进未来对长视频理解任务的细分层面,如长直播视频、会议记录和电影等的深度理解。
论文及项目相关链接
PDF Accepted to ICME 2025
Summary
针对长时间视频理解任务,本文构建了一个大规模小时级长视频基准测试集HLV-1K,旨在评估长时间视频理解模型性能。该基准测试集包含一千多小时长的视频和对应高质量问答对数据。同时,本文使用现有先进方法对基准测试集进行评估,证明了其在不同层次和不同任务上测试深度长时间视频理解能力的价值。
Key Takeaways
- 多模态大型语言模型在深度视觉理解中受到广泛关注,但长时间视频理解仍然是一个未被充分探索的领域。
- 本文构建了大规模小时级长视频基准测试集HLV-1K,包含一千多小时长的视频和对应高质量问答对数据。
- HLV-1K设计了时间感知查询和多样化注释,覆盖帧级别、事件内级别、跨事件级别和长期推理任务等多个层面。
- 本文使用现有先进方法对基准测试集进行评估,旨在评估模型性能。证明了其在测试深度长时间视频理解能力上的价值。本文将为未来的长时间视频理解任务,如直播视频、会议记录和电影等提供更深入的视角和评估方法。
点此查看论文截图
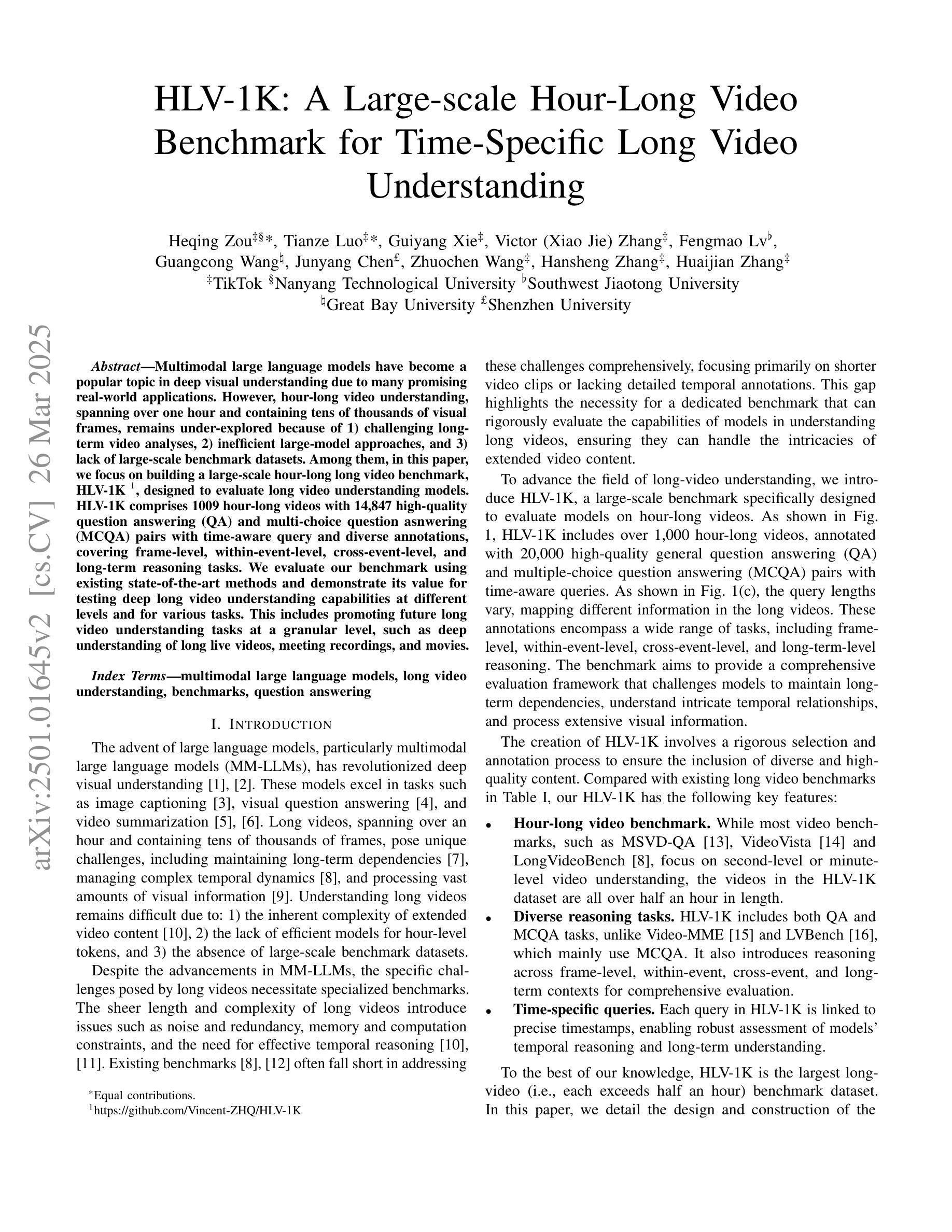
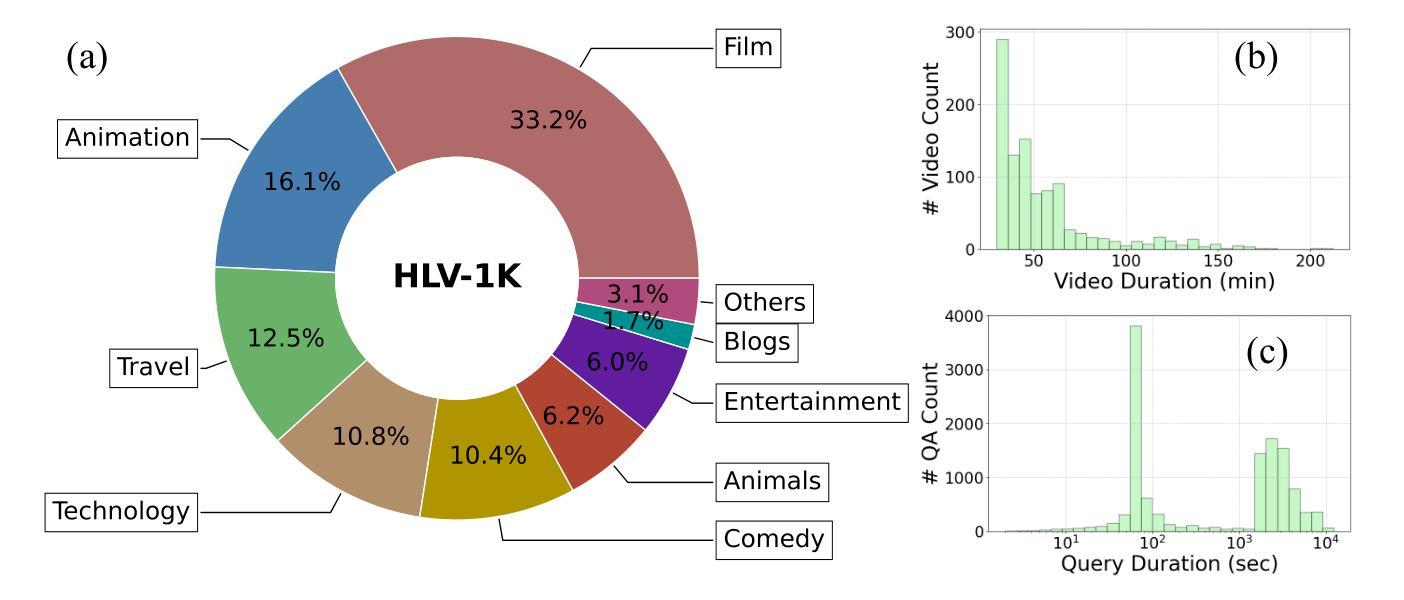
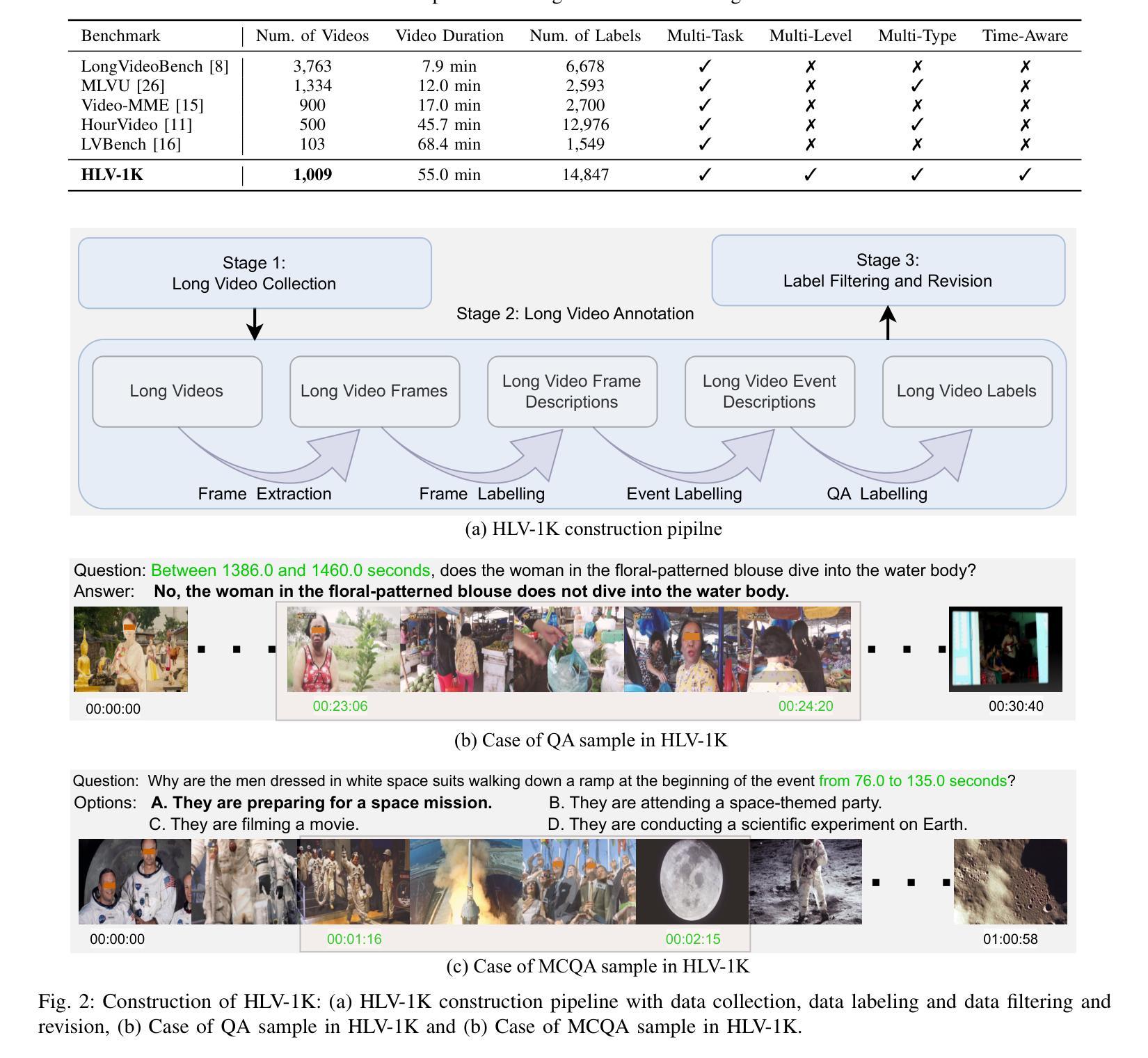
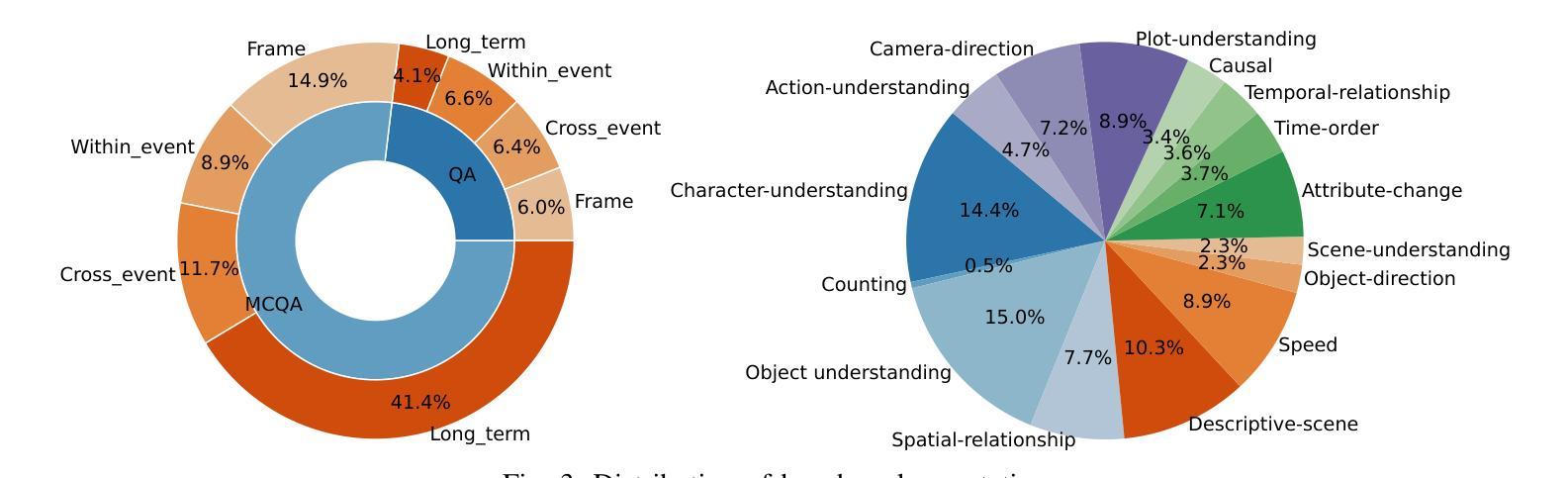
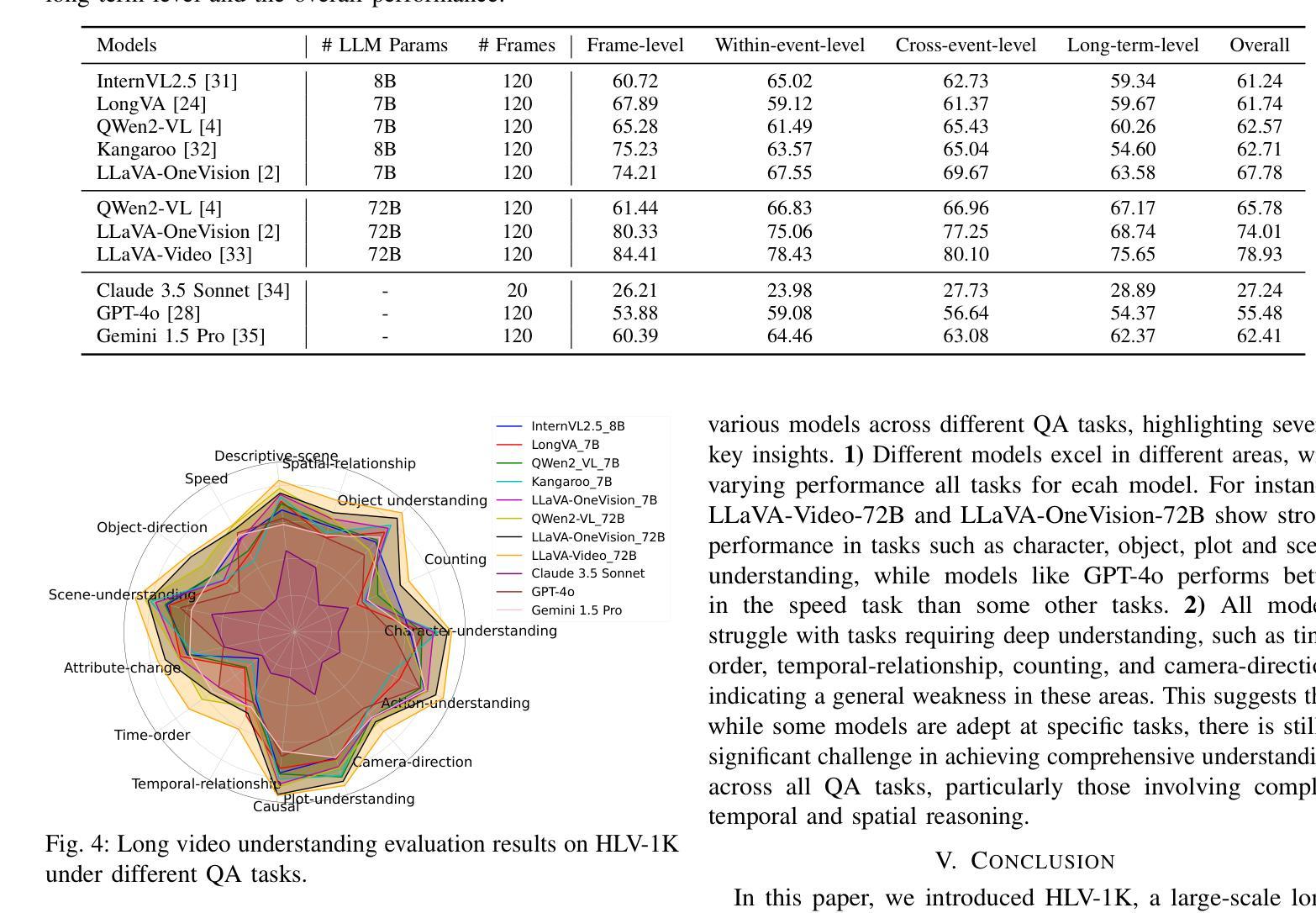