⚠️ 以下所有内容总结都来自于 大语言模型的能力,如有错误,仅供参考,谨慎使用
🔴 请注意:千万不要用于严肃的学术场景,只能用于论文阅读前的初筛!
💗 如果您觉得我们的项目对您有帮助 ChatPaperFree ,还请您给我们一些鼓励!⭐️ HuggingFace免费体验
2025-03-28 更新
Scale-Equivariant Imaging: Self-Supervised Learning for Image Super-Resolution and Deblurring
Authors:Jérémy Scanvic, Mike Davies, Patrice Abry, Julián Tachella
Self-supervised methods have recently proved to be nearly as effective as supervised ones in various imaging inverse problems, paving the way for learning-based approaches in scientific and medical imaging applications where ground truth data is hard or expensive to obtain. These methods critically rely on invariance to translations and/or rotations of the image distribution to learn from incomplete measurement data alone. However, existing approaches fail to obtain competitive performances in the problems of image super-resolution and deblurring, which play a key role in most imaging systems. In this work, we show that invariance to roto-translations is insufficient to learn from measurements that only contain low-frequency information. Instead, we propose scale-equivariant imaging, a new self-supervised approach that leverages the fact that many image distributions are approximately scale-invariant, enabling the recovery of high-frequency information lost in the measurement process. We demonstrate throughout a series of experiments on real datasets that the proposed method outperforms other self-supervised approaches, and obtains performances on par with fully supervised learning.
自监督方法在各种成像反问题上的表现已证明几乎与有监督方法一样有效,这为科学成像和医学成像应用中的基于学习的方法铺平了道路,在这些应用中,获取真实数据既困难又昂贵。这些方法严重依赖于图像分布的平移和/或旋转不变性,以从不完全的测量数据中学习。然而,现有方法在图像超分辨率和去模糊问题上未能取得具有竞争力的表现,这在大多数成像系统中起着关键作用。在这项工作中,我们证明了对于仅包含低频信息的测量而言,对旋转和平移的鲁棒性不足以进行学习。相反,我们提出了一种新的自监督方法——尺度等变成像。该方法利用了许多图像分布近似尺度不变这一事实,能够恢复测量过程中丢失的高频信息。我们通过一系列真实数据集的实验证明,该方法优于其他自监督方法,并且性能与完全监督学习相当。
论文及项目相关链接
Summary
自监督方法已在多种成像反问题中证明了其效果接近于有监督方法。在处理难以获取或昂贵成本的现实科学与医学成像应用中,它为基于学习的方法开辟了道路。现有方法大多依赖于图像分布的平移和旋转不变性以从单一的不完整测量数据中学习。然而,在图像超分辨率和去模糊等关键问题上,这些方法表现不佳。这些问题是大多数成像系统的核心。为解决这一问题,我们提出了尺度等价成像这一新型自监督方法,它利用许多图像分布近似尺度不变这一事实来恢复测量过程中丢失的高频信息。实验证明,该方法在真实数据集上的表现优于其他自监督方法,并与全监督学习相当。
Key Takeaways
- 自监督方法在多种成像反问题中效果显著,尤其适用于缺乏真实数据的情况。
- 现有方法主要依赖平移和旋转不变性从单一的不完整测量数据中学习。
- 在图像超分辨率和去模糊问题上,现有方法表现不足。
- 尺度等价成像是一种新型自监督方法,利用图像分布的尺度不变性来恢复高频信息。
- 该方法在真实数据集上的实验表现优于其他自监督方法。
- 尺度等价成像方法的性能与全监督学习相当。
点此查看论文截图
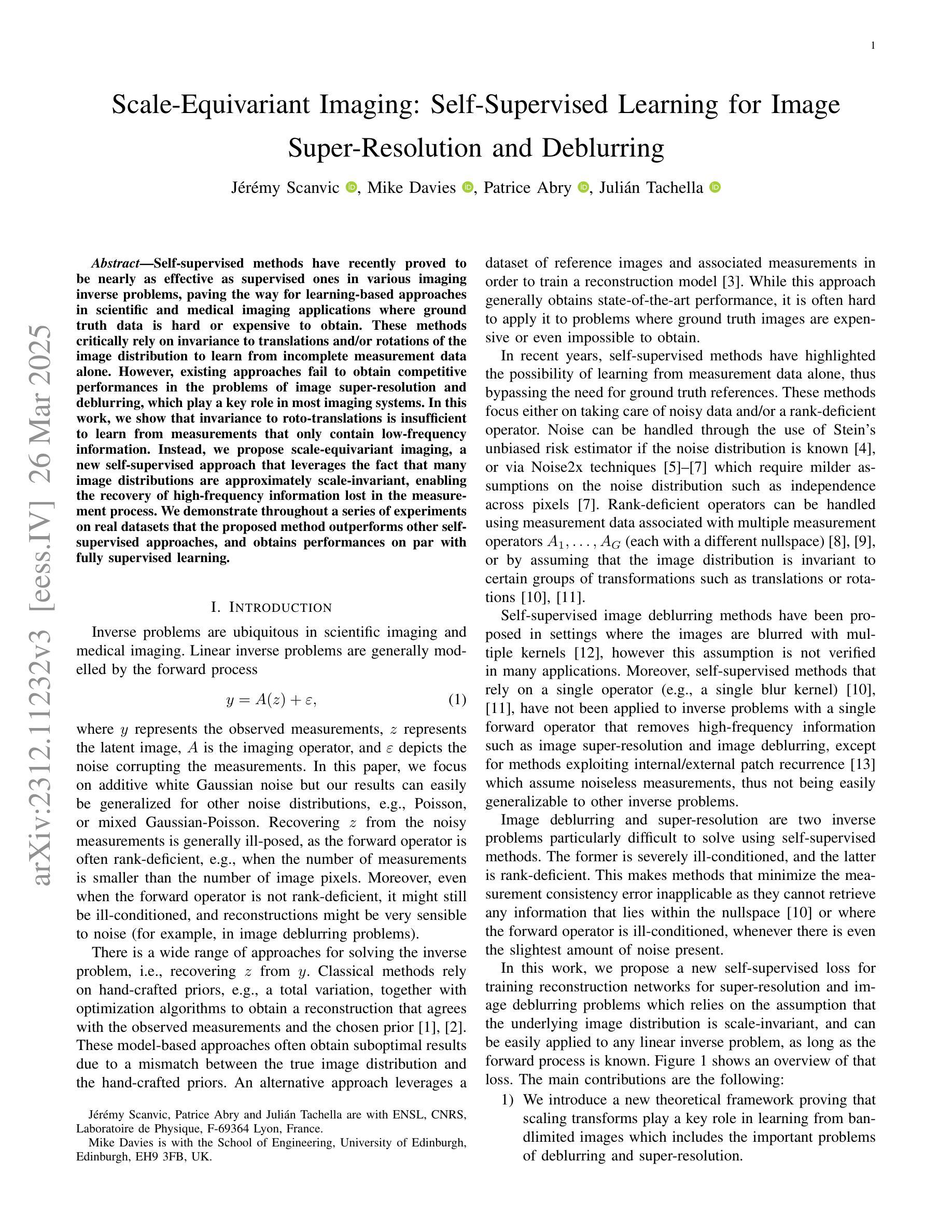
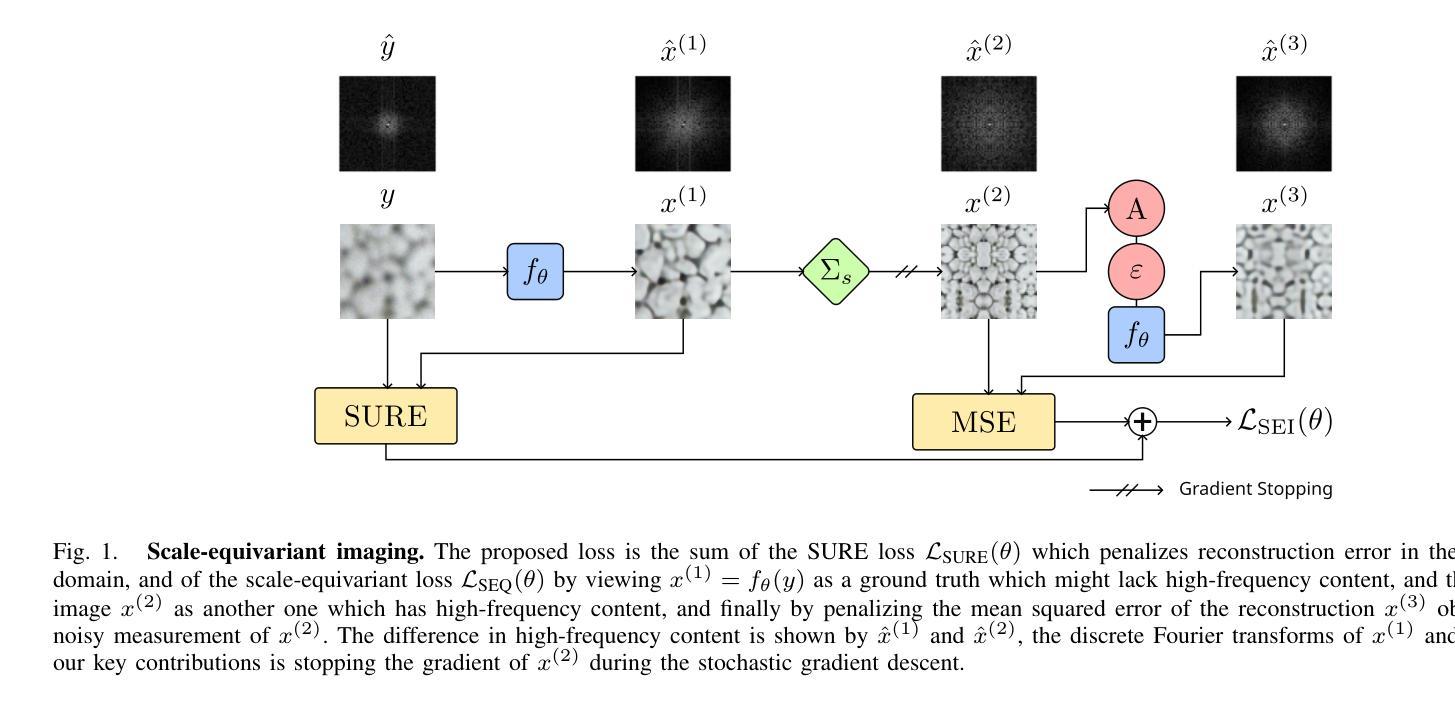
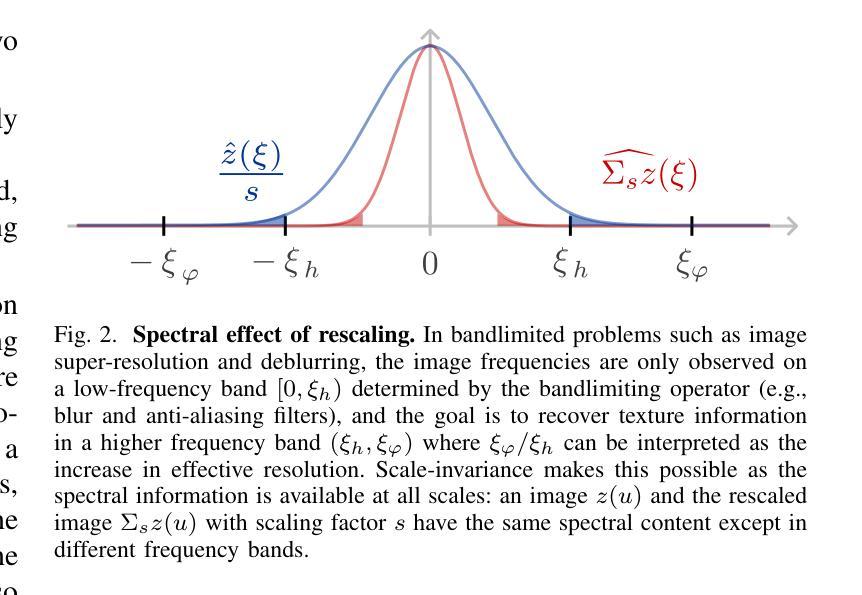
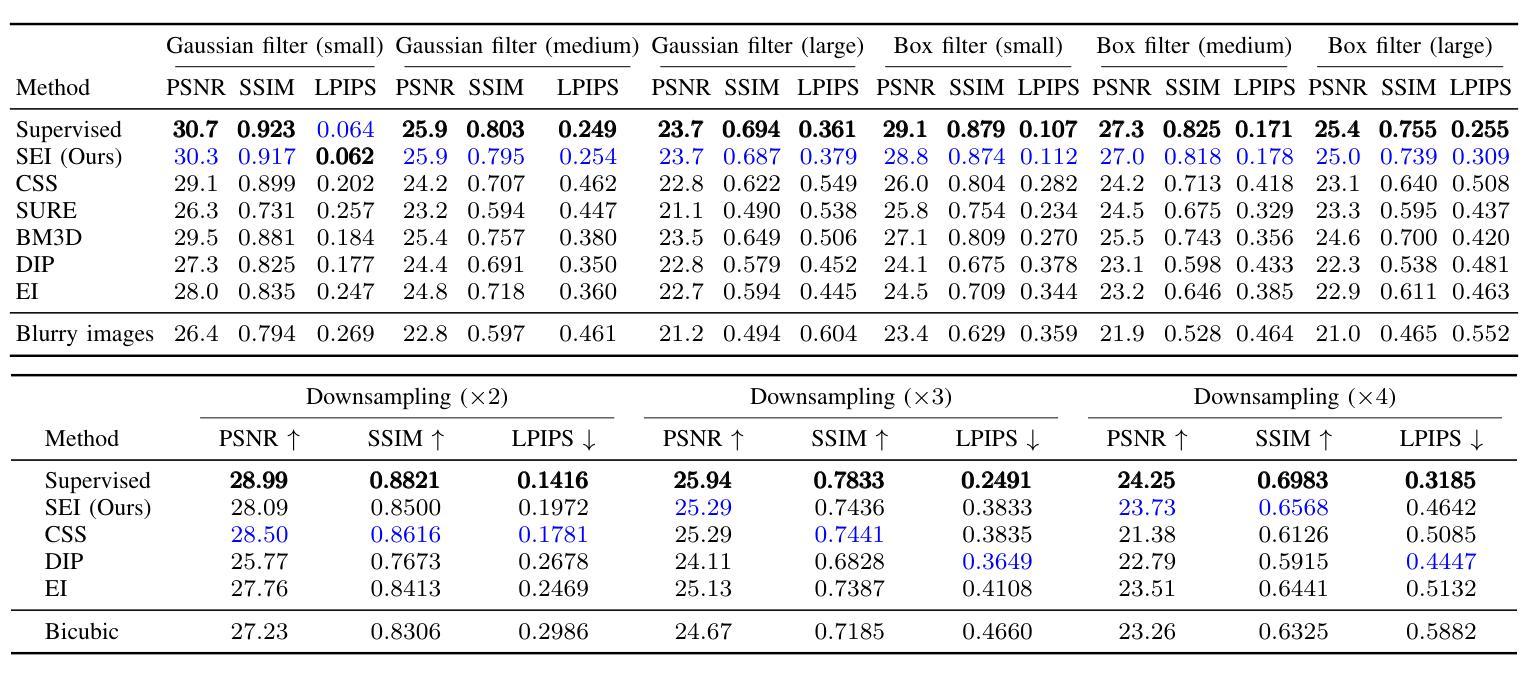
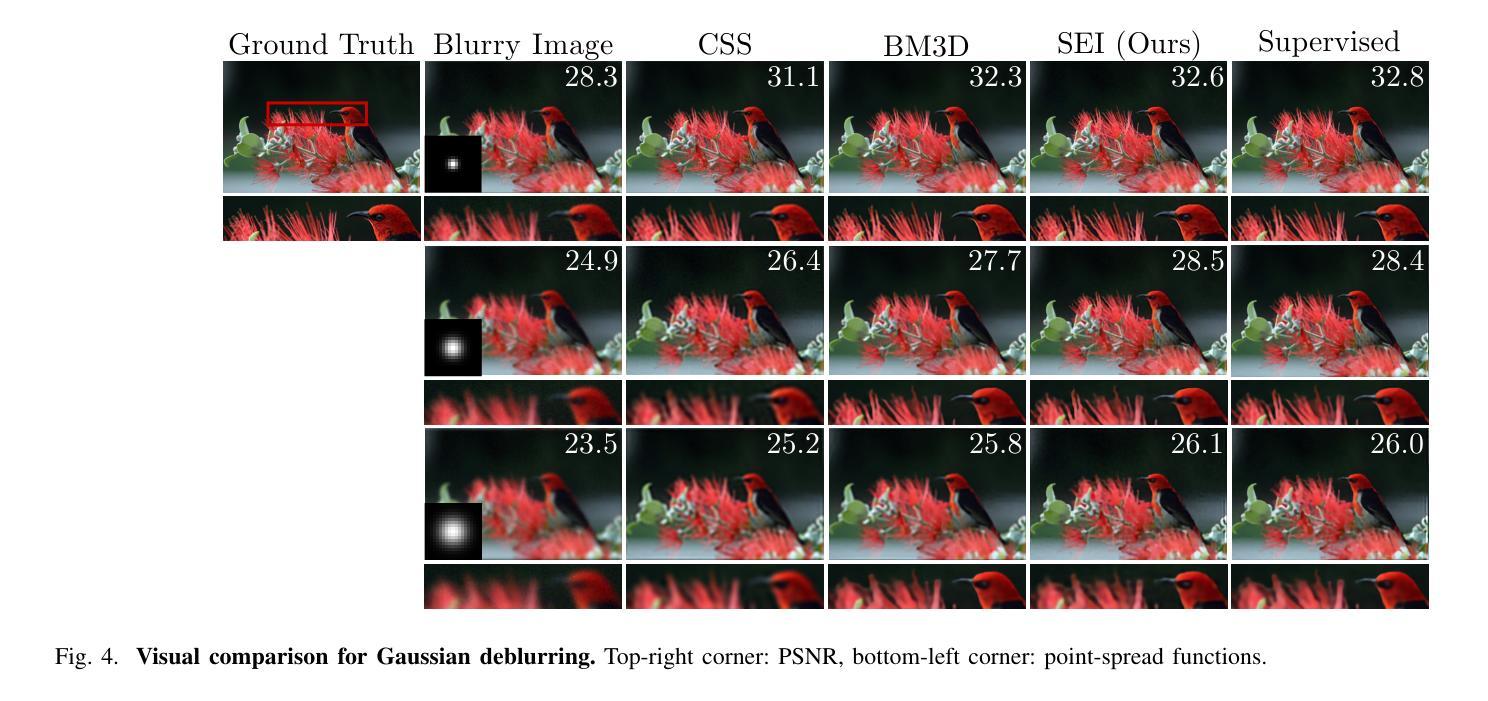
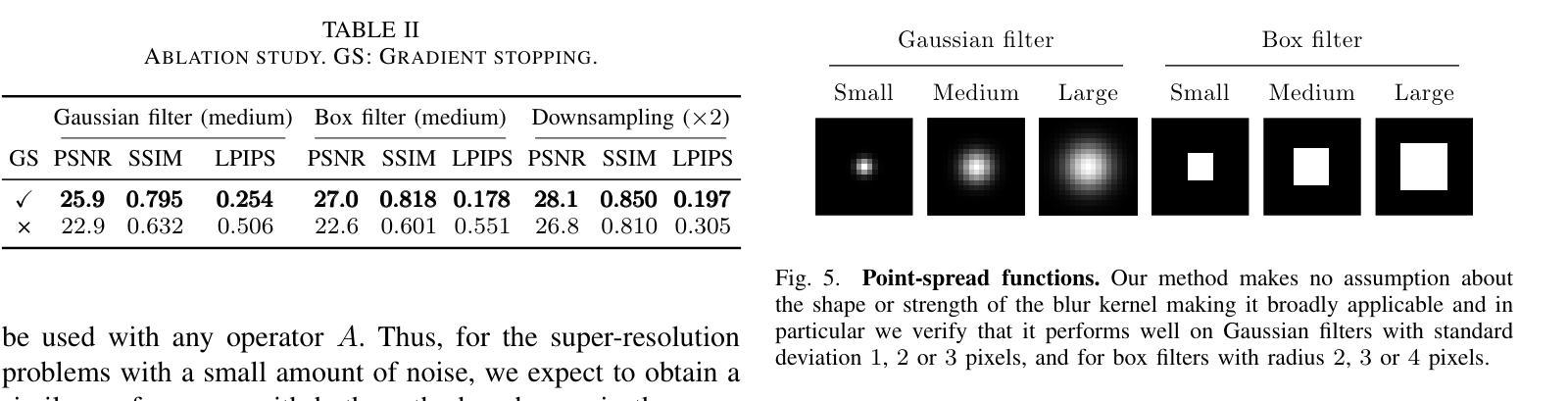