⚠️ 以下所有内容总结都来自于 大语言模型的能力,如有错误,仅供参考,谨慎使用
🔴 请注意:千万不要用于严肃的学术场景,只能用于论文阅读前的初筛!
💗 如果您觉得我们的项目对您有帮助 ChatPaperFree ,还请您给我们一些鼓励!⭐️ HuggingFace免费体验
2025-03-28 更新
ARFlow: Human Action-Reaction Flow Matching with Physical Guidance
Authors:Wentao Jiang, Jingya Wang, Haotao Lu, Kaiyang Ji, Baoxiong Jia, Siyuan Huang, Ye Shi
Human action-reaction synthesis, a fundamental challenge in modeling causal human interactions, plays a critical role in applications ranging from virtual reality to social robotics. While diffusion-based models have demonstrated promising performance, they exhibit two key limitations for interaction synthesis: reliance on complex noise-to-reaction generators with intricate conditional mechanisms, and frequent physical violations in generated motions. To address these issues, we propose Action-Reaction Flow Matching (ARFlow), a novel framework that establishes direct action-to-reaction mappings, eliminating the need for complex conditional mechanisms. Our approach introduces two key innovations: an x1-prediction method that directly outputs human motions instead of velocity fields, enabling explicit constraint enforcement; and a training-free, gradient-based physical guidance mechanism that effectively prevents body penetration artifacts during sampling. Extensive experiments on NTU120 and Chi3D datasets demonstrate that ARFlow not only outperforms existing methods in terms of Fr'echet Inception Distance and motion diversity but also significantly reduces body collisions, as measured by our new Intersection Volume and Intersection Frequency metrics.
人类行为反应合成是模拟因果人类交互的一个基本挑战,在虚拟现实到社交机器人等应用中发挥着关键作用。虽然基于扩散的模型已经表现出了有前景的性能,但它们在交互合成方面表现出两个关键局限性:依赖复杂的噪声到反应生成器,具有复杂的条件机制,以及生成动作中的频繁物理违规。为了解决这些问题,我们提出了Action-Reaction Flow Matching(ARFlow)这一新型框架,建立了直接的动作到反应映射,消除了对复杂条件机制的需求。我们的方法引入了两个关键创新点:x1预测方法直接输出人类动作而不是速度场,实现了明确的约束执行;以及基于梯度的无训练物理引导机制,有效防止采样过程中的身体穿透伪影。在NTU120和Chi3D数据集上的大量实验表明,ARFlow不仅在Fréchet Inception距离和运动多样性方面优于现有方法,而且通过我们新的交集体积和交集频率指标测量,显著减少了身体碰撞。
论文及项目相关链接
PDF Project Page: https://arflow2025.github.io/
Summary
动作反应合成是人类交互建模的核心挑战,对虚拟现实、社交机器人等领域的应用至关重要。针对扩散模型在交互合成中的两个关键局限——依赖复杂的噪声到反应生成器以及频繁的物理违规动作,我们提出了行动反应流匹配(ARFlow)框架。该框架建立直接的行动到反应映射,无需复杂的条件机制。通过引入x1预测方法和无训练、基于梯度的物理引导机制,ARFlow在NTU120和Chi3D数据集上的实验表明,不仅在Fréchet Inception Distance和动作多样性方面优于现有方法,还能显著降低身体碰撞。
Key Takeaways
- 动作反应合成是建模因果人类交互的核心挑战,对虚拟现实和社交机器人等领域应用至关重要。
- 扩散模型在交互合成中面临两大局限:依赖复杂噪声到反应生成器及物理违规动作的频繁出现。
- ARFlow框架通过建立直接的行动到反应映射来解决这些问题,无需复杂的条件机制。
- ARFlow引入的x1预测方法可直接输出人类动作,而非速度场,从而能明确执行约束。
- ARFlow采用无训练、基于梯度的物理引导机制,有效防止采样过程中的身体穿透伪影。
- 在NTU120和Chi3D数据集上的实验表明,ARFlow在Fréchet Inception Distance和动作多样性方面优于现有方法。
点此查看论文截图
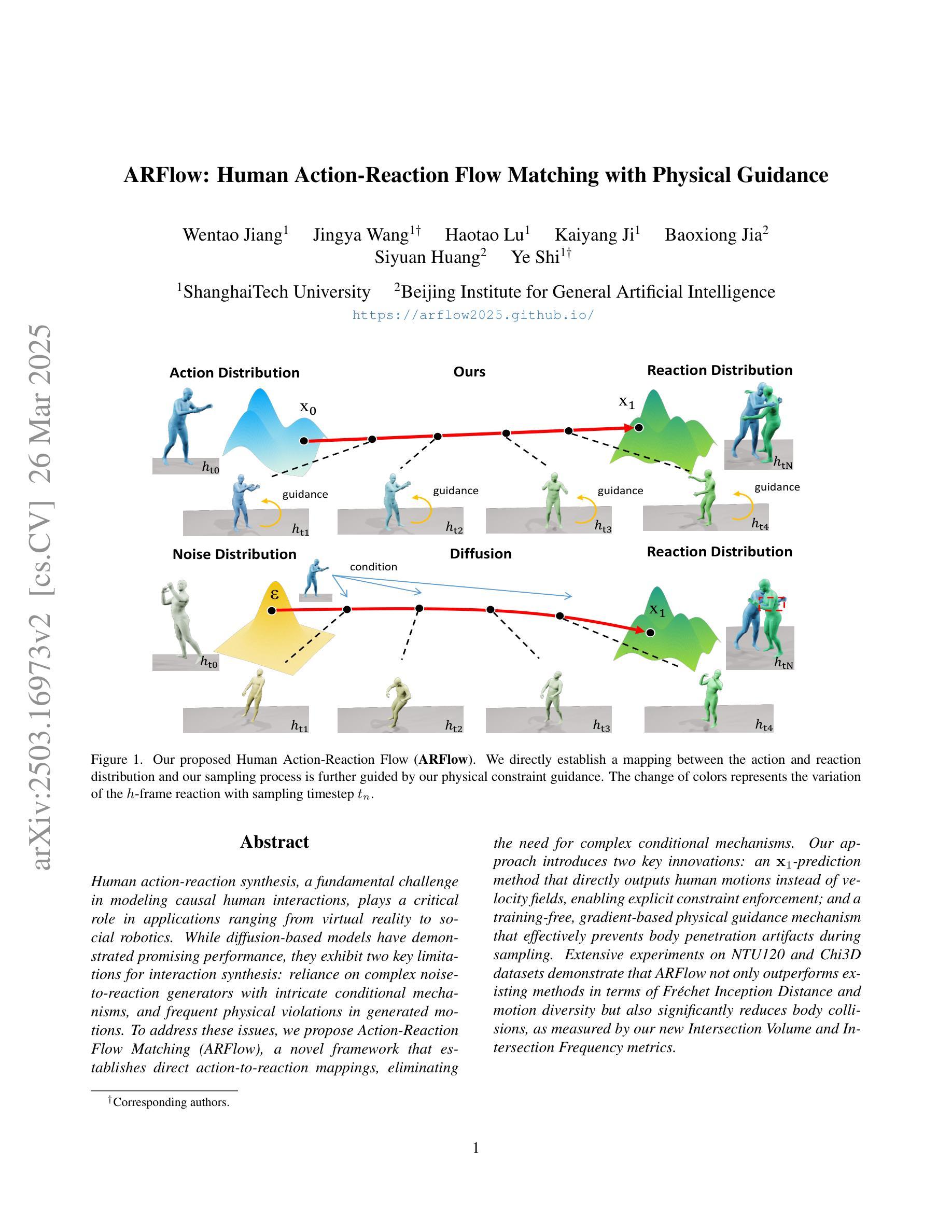
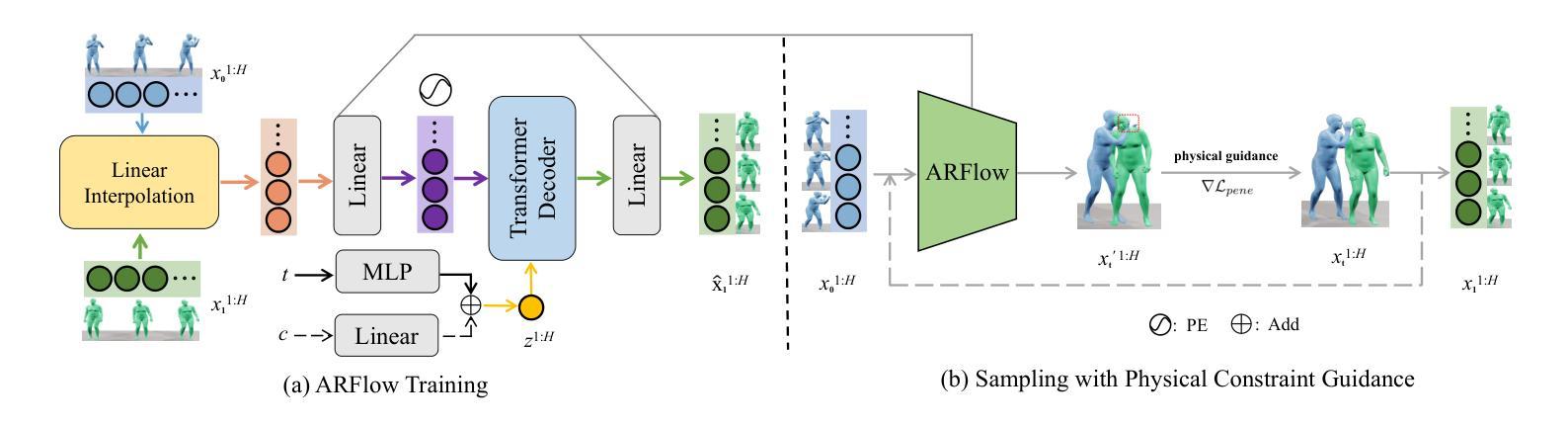
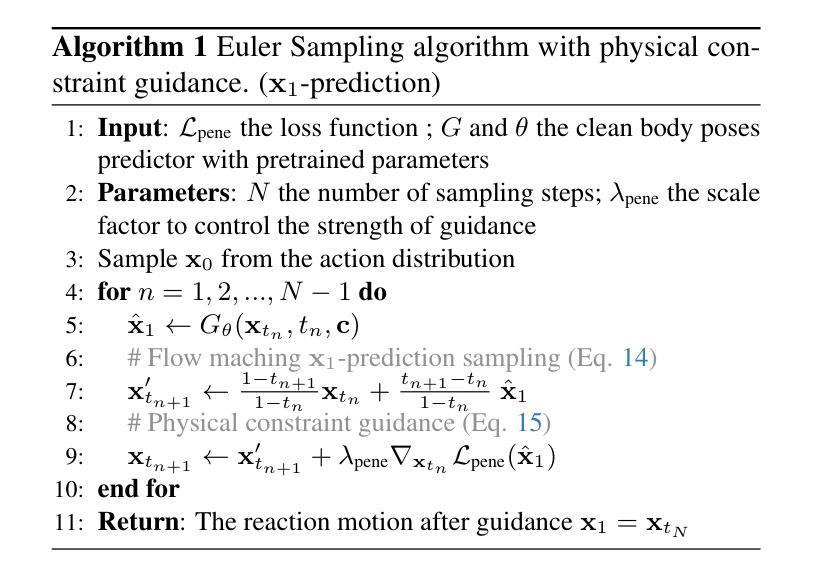
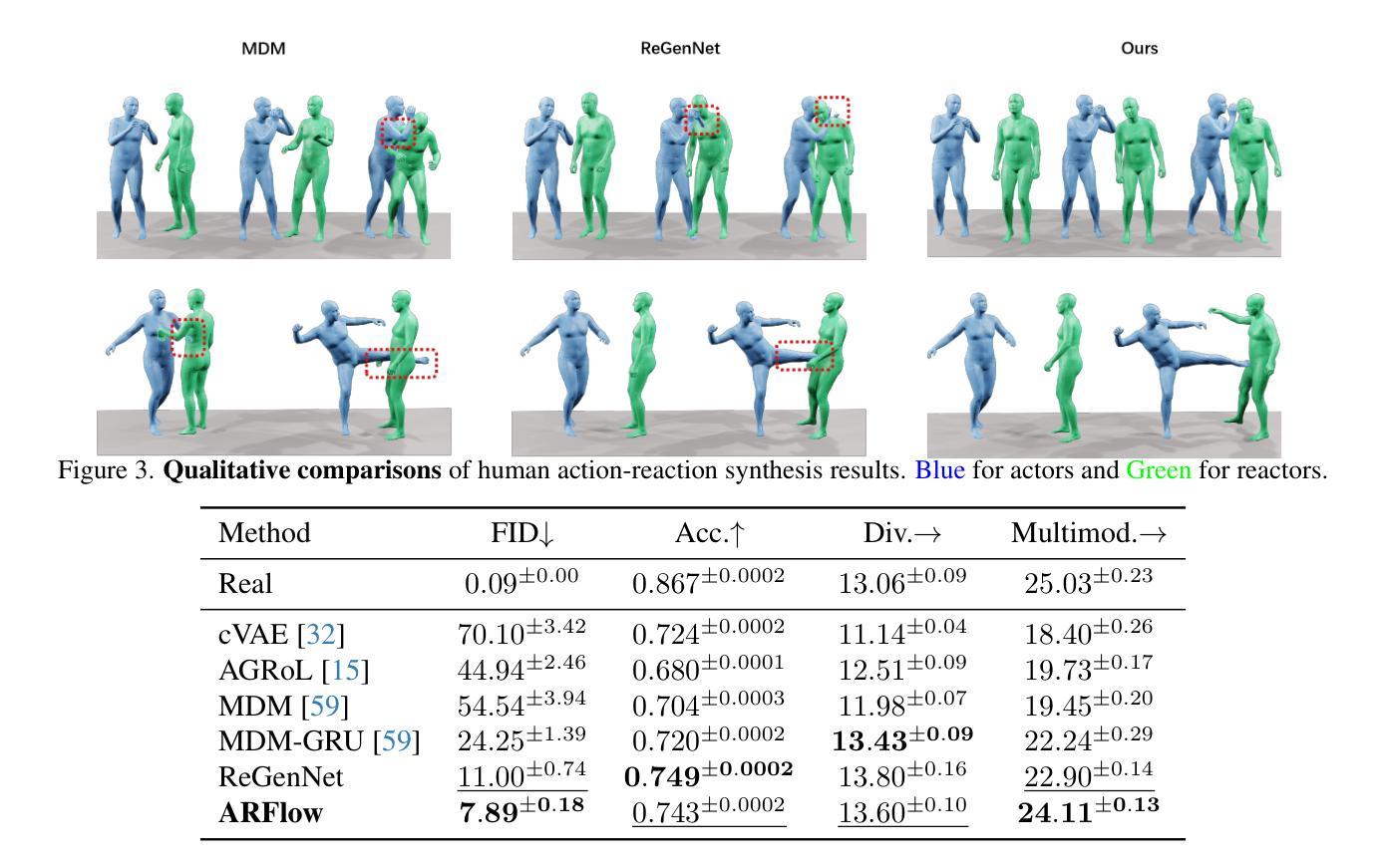