⚠️ 以下所有内容总结都来自于 大语言模型的能力,如有错误,仅供参考,谨慎使用
🔴 请注意:千万不要用于严肃的学术场景,只能用于论文阅读前的初筛!
💗 如果您觉得我们的项目对您有帮助 ChatPaperFree ,还请您给我们一些鼓励!⭐️ HuggingFace免费体验
2025-03-28 更新
QualiSpeech: A Speech Quality Assessment Dataset with Natural Language Reasoning and Descriptions
Authors:Siyin Wang, Wenyi Yu, Xianzhao Chen, Xiaohai Tian, Jun Zhang, Yu Tsao, Junichi Yamagishi, Yuxuan Wang, Chao Zhang
This paper explores a novel perspective to speech quality assessment by leveraging natural language descriptions, offering richer, more nuanced insights than traditional numerical scoring methods. Natural language feedback provides instructive recommendations and detailed evaluations, yet existing datasets lack the comprehensive annotations needed for this approach. To bridge this gap, we introduce QualiSpeech, a comprehensive low-level speech quality assessment dataset encompassing 11 key aspects and detailed natural language comments that include reasoning and contextual insights. Additionally, we propose the QualiSpeech Benchmark to evaluate the low-level speech understanding capabilities of auditory large language models (LLMs). Experimental results demonstrate that finetuned auditory LLMs can reliably generate detailed descriptions of noise and distortion, effectively identifying their types and temporal characteristics. The results further highlight the potential for incorporating reasoning to enhance the accuracy and reliability of quality assessments. The dataset will be released at https://huggingface.co/datasets/tsinghua-ee/QualiSpeech.
本文探索了一种利用自然语言描述进行语音质量评估的新视角,提供了比传统数字评分方法更丰富、更细微的见解。自然语言反馈提供了指导性的建议和详细的评价,但现有数据集缺乏这种评估方法所需的全面注释。为了弥补这一差距,我们推出了QualiSpeech数据集,这是一个全面的低级别语音质量评估数据集,涵盖了11个关键方面和包含推理和上下文洞察的自然语言详细注释。此外,我们还提出了QualiSpeech基准测试,以评估听觉大型语言模型(LLM)对低级别语音的理解能力。实验结果表明,微调后的听觉LLM能够可靠地描述噪声和失真的细节,有效地识别它们的类型和时间特征。结果还表明,通过融入推理机制可以提高质量评估的准确性和可靠性。该数据集将在https://huggingface.co/datasets/tsinghua-ee/QualiSpeech发布。
论文及项目相关链接
PDF 23 pages, 16 figures
Summary:
本文探索了利用自然语言描述进行语音质量评估的新视角,相较于传统的数字评分方法,这种方法提供更丰富、更细腻的见解。由于现有数据集缺乏全面注释,为了弥补这一差距,引入了QualiSpeech数据集和QualiSpeech Benchmark。实验结果表明,经过微调的大型听觉语言模型能够可靠地描述噪声和失真,并有效地识别其类型和时间特征。该数据集将在huggingface.co/datasets/tsinghua-ee/QualiSpeech发布。
Key Takeaways:
- 利用自然语言描述为语音质量评估提供了新的视角。
- 现有数据集缺乏全面注释,需要新的数据集来支持自然语言描述的语音质量评估方法。
- 介绍了QualiSpeech数据集和QualiSpeech Benchmark以支持语音质量评估研究。
- 实验证明了大型听觉语言模型在描述噪声和失真方面的有效性。
- 大型听觉语言模型能够识别噪声和失真的类型和时间特征。
- 自然语言反馈能够提供指导性的建议和详细的评估。
点此查看论文截图
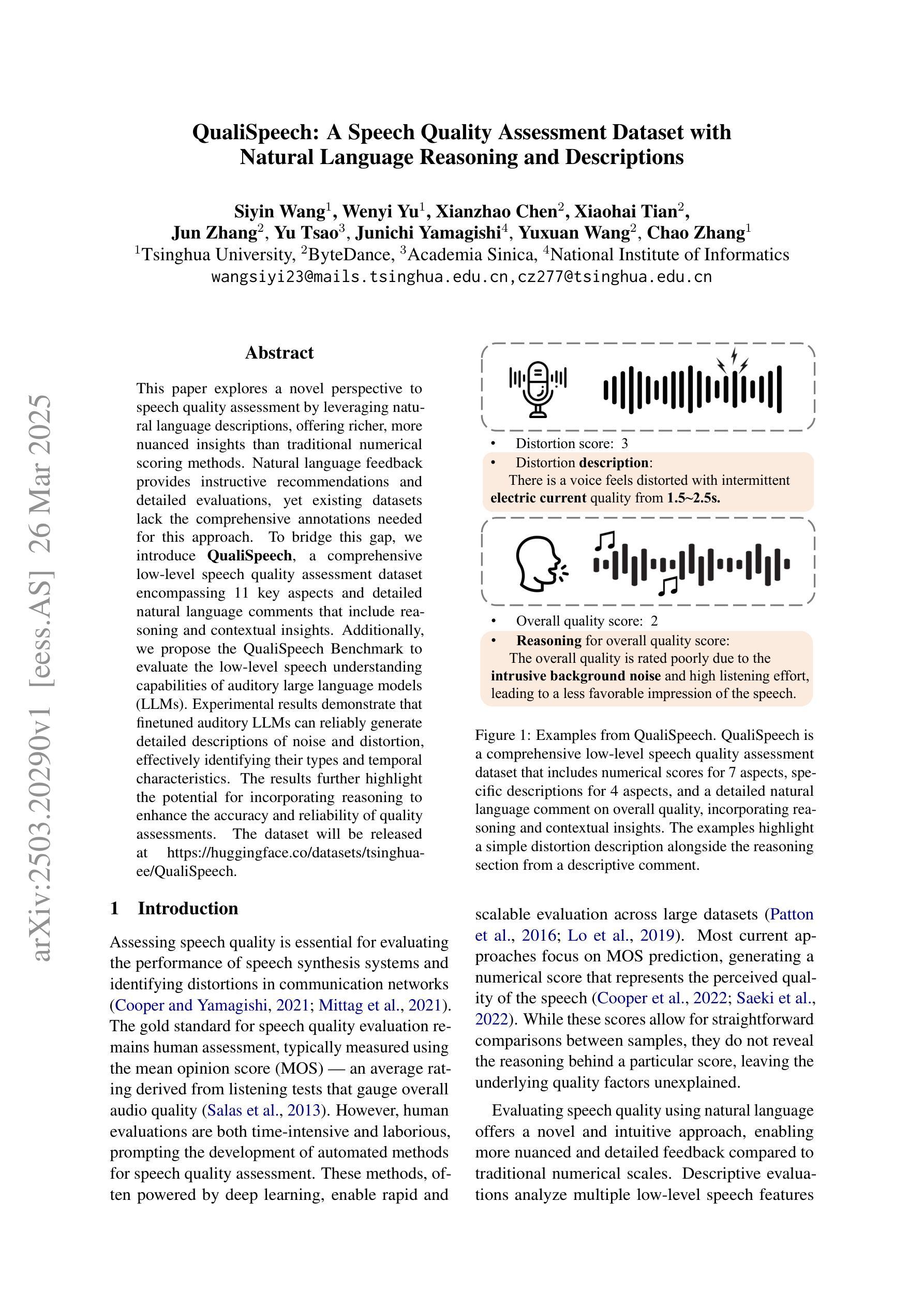
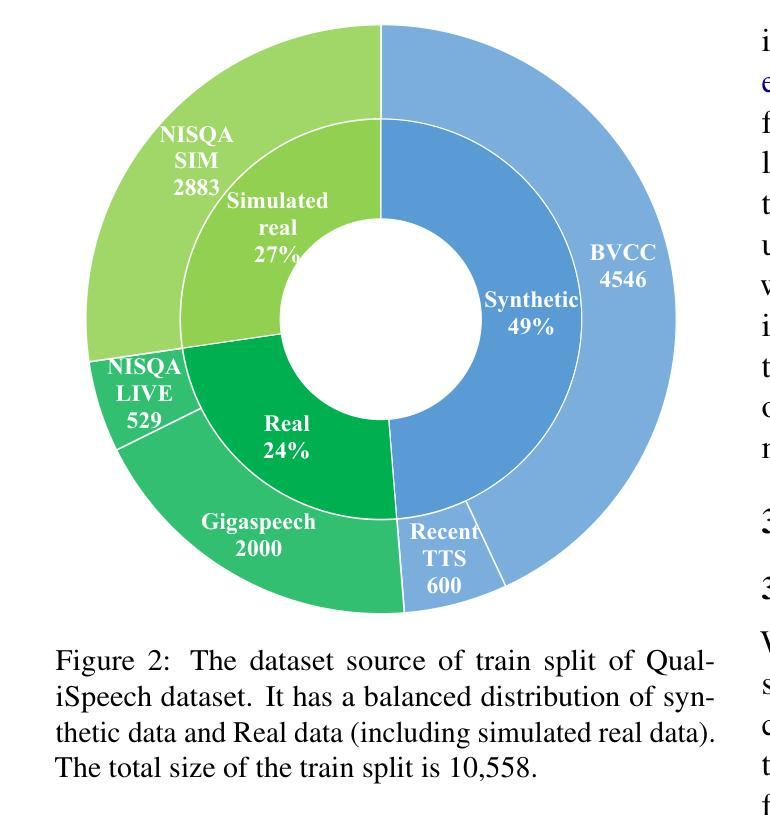
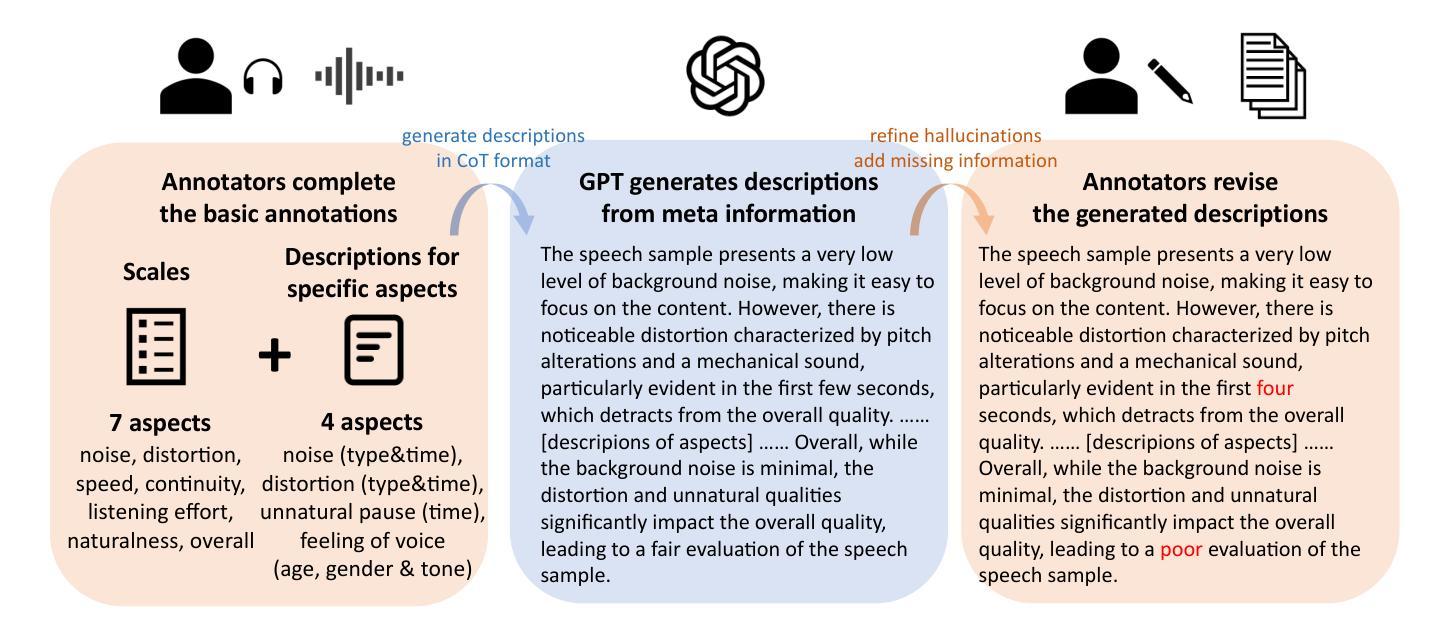
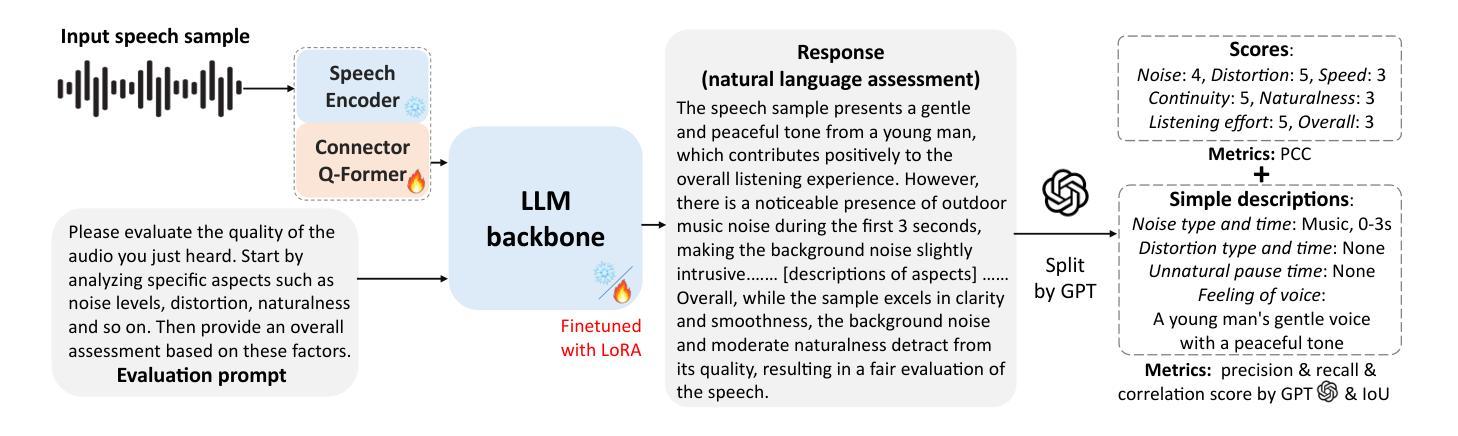
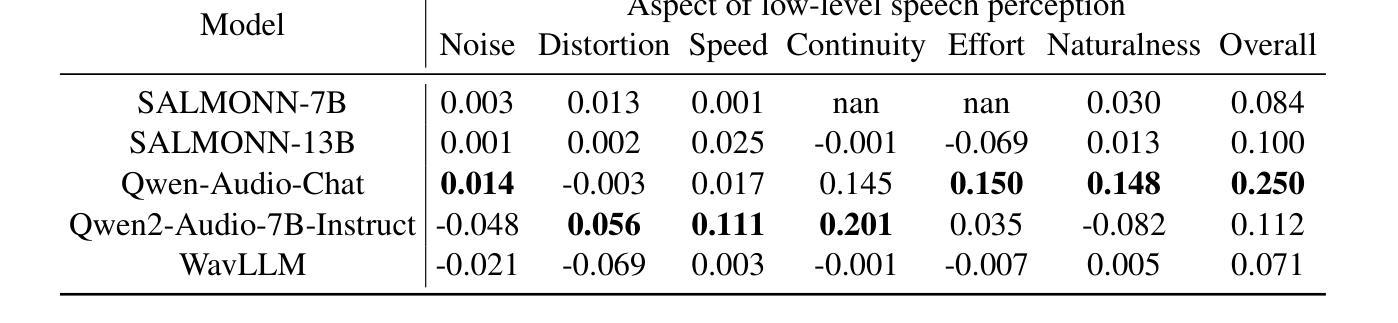
Dolphin: A Large-Scale Automatic Speech Recognition Model for Eastern Languages
Authors:Yangyang Meng, Jinpeng Li, Guodong Lin, Yu Pu, Guanbo Wang, Hu Du, Zhiming Shao, Yukai Huang, Ke Li, Wei-Qiang Zhang
This report introduces Dolphin, a large-scale multilingual automatic speech recognition (ASR) model that extends the Whisper architecture to support a wider range of languages. Our approach integrates in-house proprietary and open-source datasets to refine and optimize Dolphin’s performance. The model is specifically designed to achieve notable recognition accuracy for 40 Eastern languages across East Asia, South Asia, Southeast Asia, and the Middle East, while also supporting 22 Chinese dialects. Experimental evaluations show that Dolphin significantly outperforms current state-of-the-art open-source models across various languages. To promote reproducibility and community-driven innovation, we are making our trained models and inference source code publicly available.
这篇报告介绍了海豚(Dolphin)这一大规模的多语种自动语音识别(ASR)模型。海豚扩展了whisper架构,以支持更多的语言。我们的方法结合了内部专有数据集和开源数据集,以优化和改进海豚的性能。该模型被特别设计以实现东亚、南亚、东南亚和中东地区的40种东方语言的显著识别准确率,同时也支持中国的22种方言。实验评估表明,海豚在各种语言上的表现显著优于当前最先进的开源模型。为了促进可复制性和社区驱动的创新,我们将训练好的模型和推理源代码公开提供。
论文及项目相关链接
Summary:
这份报告介绍了一款名为Dolphin的大型多语种自动语音识别(ASR)模型。该模型基于Whisper架构,支持更多语种,通过整合内部专有和开源数据集来优化性能。特别为东亚、南亚、东南亚和中东地区的40种语言提供显著的识别准确性,同时支持22种中文方言。实验评估显示,Dolphin在各种语言上显著优于当前最先进的开源模型。为促进可重复性和社区驱动的创新,我们公开提供训练好的模型和推理源代码。
Key Takeaways:
- Dolphin是一个基于Whisper架构的多语种自动语音识别(ASR)模型。
- 支持广泛的40种东方语言及22种中文方言。
- 通过整合内部和开源数据集来优化性能。
- 在多种语言上的识别准确率显著高于当前最先进的开源模型。
- 模型具备高度的可扩展性和灵活性,能适应不同的语言和地区需求。
- 提供训练好的模型和推理源代码,促进可重复性和社区驱动的创新。
点此查看论文截图
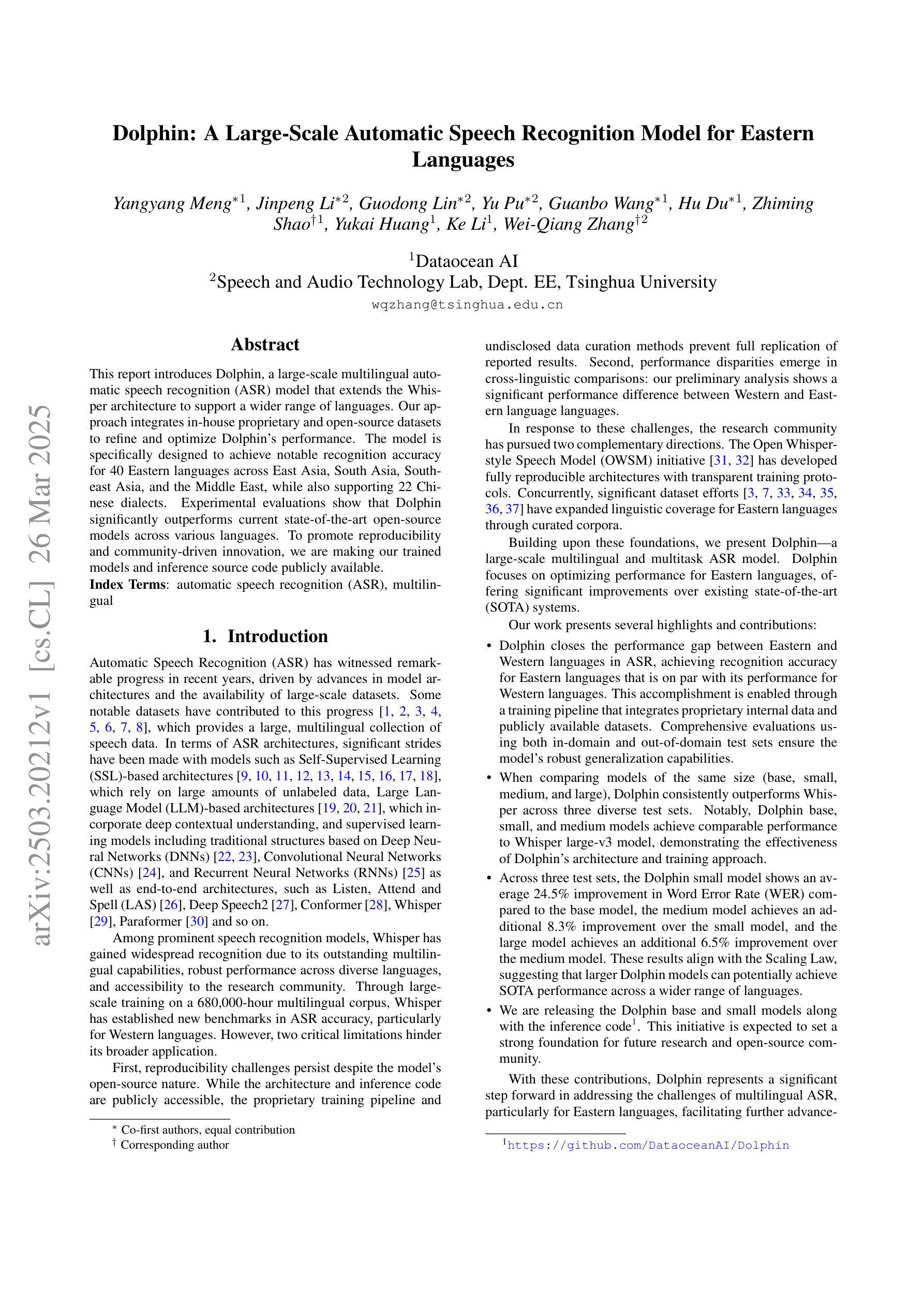
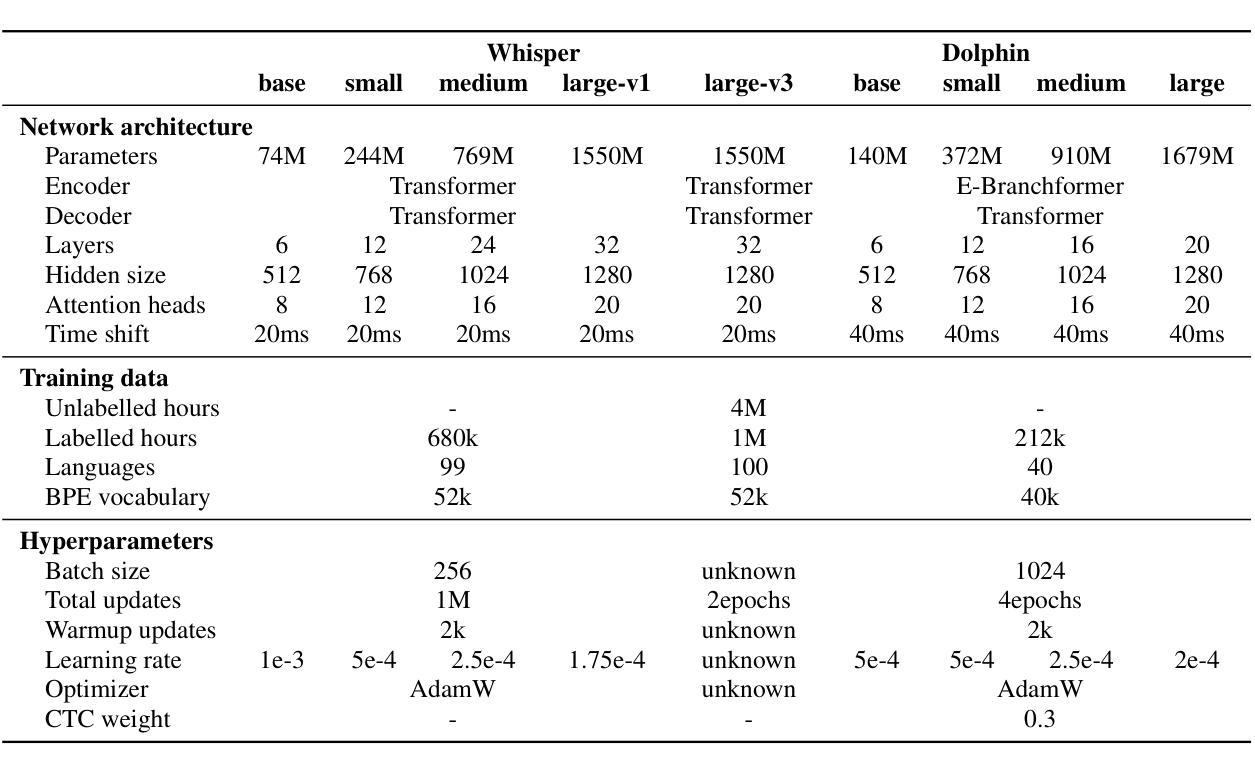
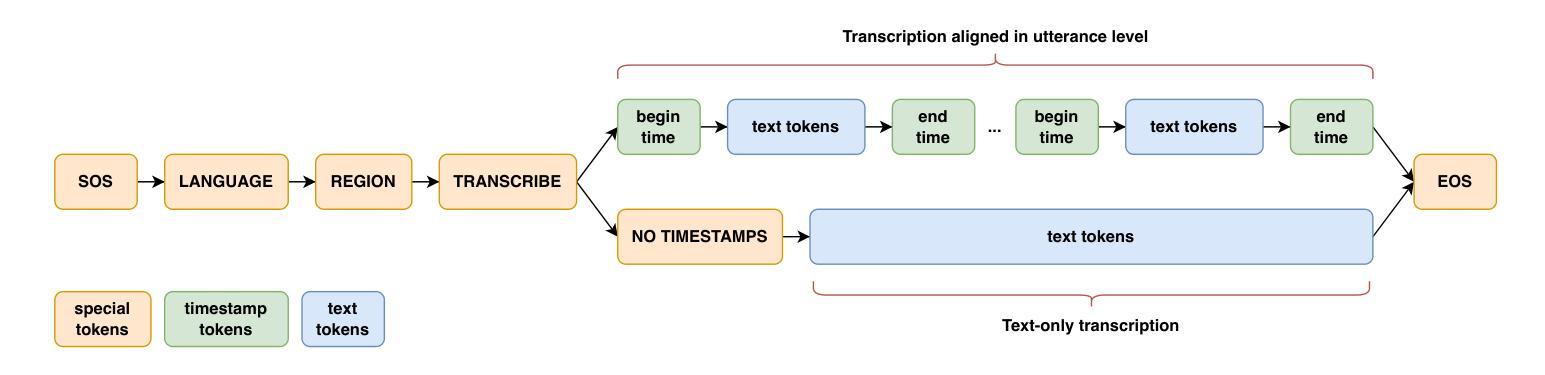
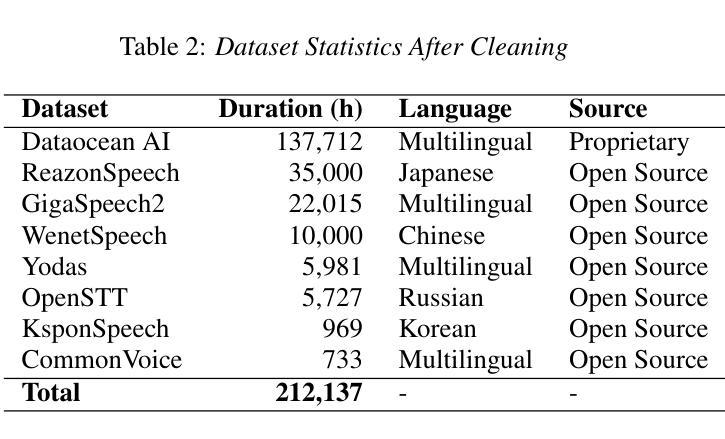
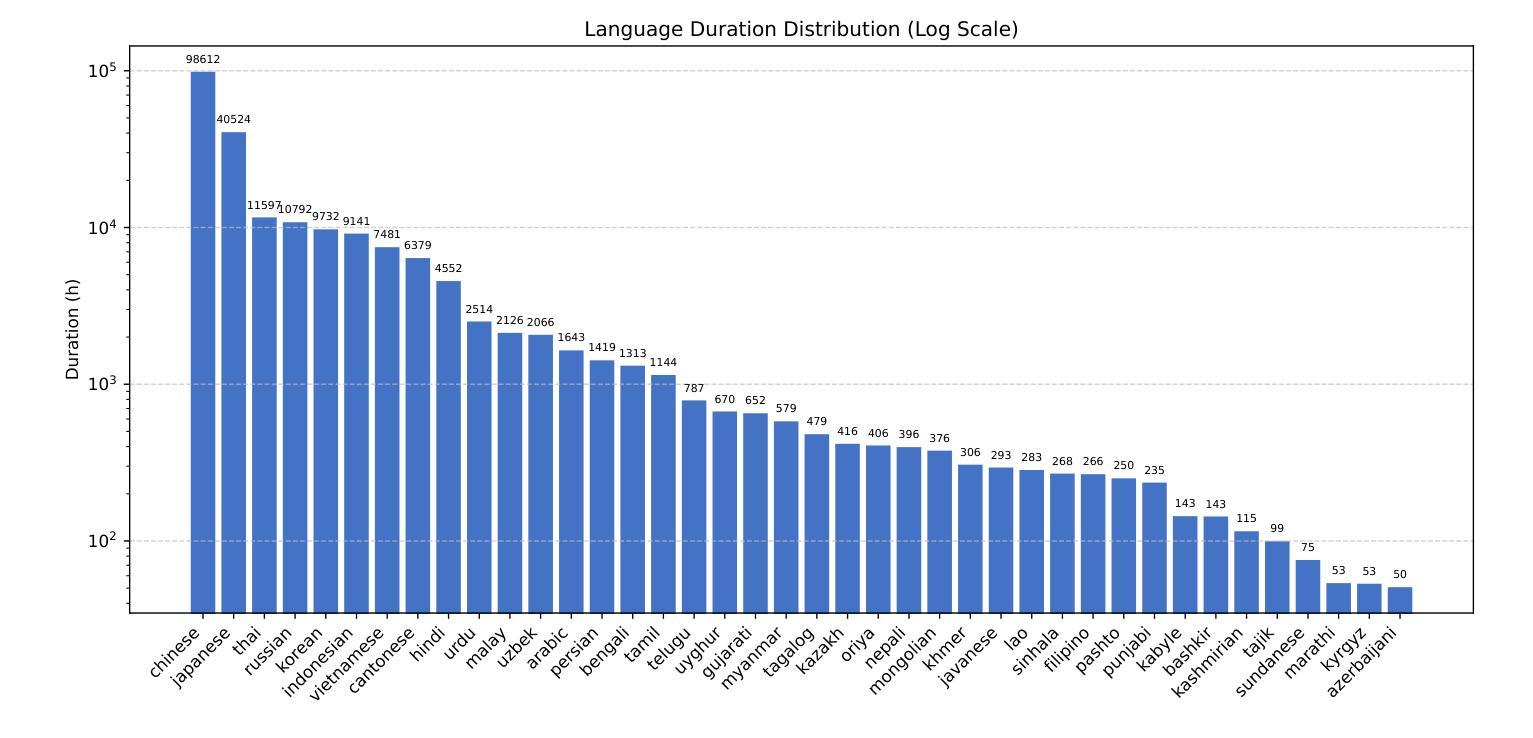
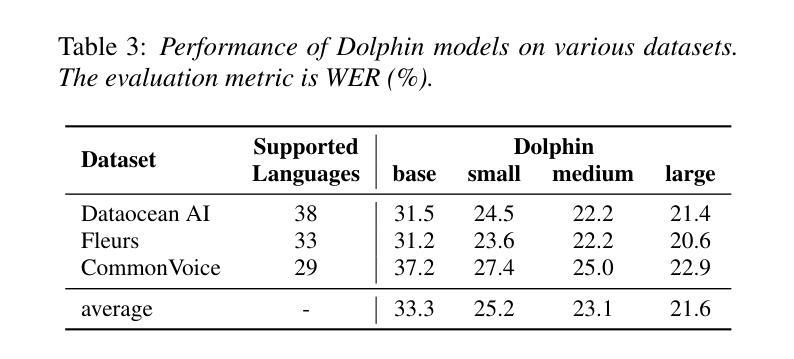
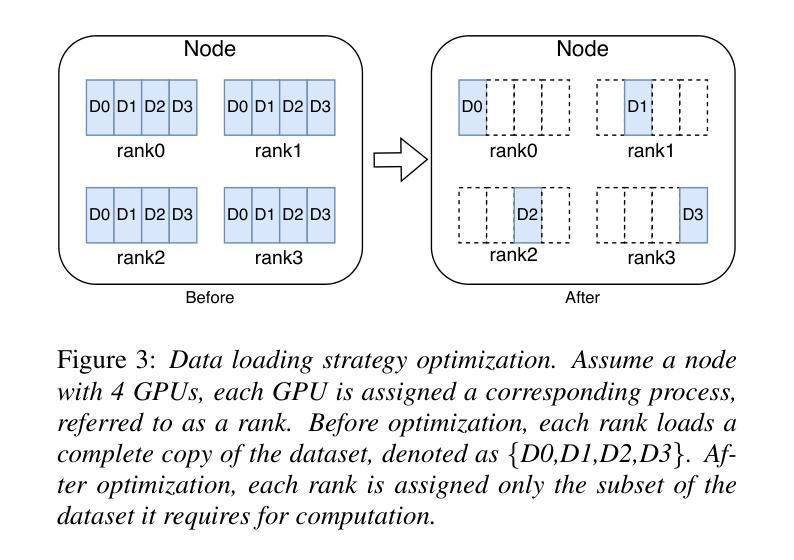
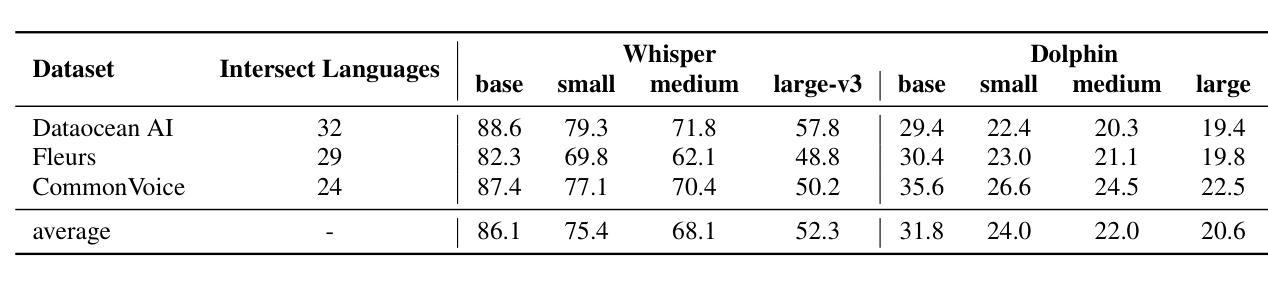
Image Generation with Supervised Selection Based on Multimodal Features for Semantic Communications
Authors:Chengyang Liang, Dong Li
Semantic communication (SemCom) has emerged as a promising technique for the next-generation communication systems, in which the generation at the receiver side is allowed with semantic features’ recovery. However, the majority of existing research predominantly utilizes a singular type of semantic information, such as text, images, or speech, to supervise and choose the generated source signals, which may not sufficiently encapsulate the comprehensive and accurate semantic information, and thus creating a performance bottleneck. In order to bridge this gap, in this paper, we propose and investigate a SemCom framework using multimodal information to supervise the generated image. To be specific, in this framework, we first extract semantic features at both the image and text levels utilizing the Convolutional Neural Network (CNN) architecture and the Contrastive Language-Image Pre-Training (CLIP) model before transmission. Then, we employ a generative diffusion model at the receiver to generate multiple images. In order to ensure the accurate extraction and facilitate high-fidelity image reconstruction, we select the “best” image with the minimum reconstruction errors by taking both the aided image and text semantic features into account. We further extend multimodal semantic communication (MMSemCom) system to the multiuser scenario for orthogonal transmission. Experimental results demonstrate that the proposed framework can not only achieve the enhanced fidelity and robustness in image transmission compared with existing communication systems but also sustain a high performance in the low signal-to-noise ratio (SNR) conditions.
语义通信(SemCom)作为一种新兴技术,已成为下一代通信系统的有前途的技术。在语义通信中,接收端的生成允许恢复语义特征。然而,现有的大多数研究主要利用单一的语义信息类型(如文本、图像或语音)来监督和选择生成的源信号,这可能无法充分包含全面和准确的语义信息,从而限制了性能。为了弥补这一差距,本文提出了一种利用多模态信息监督生成图像的SemCom框架。具体来说,在此框架中,我们首先在传输之前,利用卷积神经网络(CNN)架构和对比语言-图像预训练(CLIP)模型,在图像和文本层面提取语义特征。然后,我们在接收端采用生成扩散模型生成多个图像。为了确保准确的提取和高质量图像重建,我们综合考虑辅助图像和文本语义特征,选择重建错误最小的“最佳”图像。我们将多模态语义通信(MMSemCom)系统进一步扩展到多用户场景,以实现正交传输。实验结果表明,与传统的通信系统相比,该框架在图像传输中不仅提高了保真度和稳健性,而且在低信噪比(SNR)条件下也保持了高性能。
论文及项目相关链接
Summary
在语义通信(SemCom)中,现有研究主要使用单一类型的语义信息(如文本、图像或语音)来监督和选择生成的源信号,这可能会限制语义信息的全面性和准确性,造成性能瓶颈。为了弥补这一缺陷,本文提出了一个使用多模态信息监督生成图像的SemCom框架。该框架在传输前,利用卷积神经网络(CNN)和对比语言图像预训练(CLIP)模型提取图像和文本级别的语义特征。接收端采用生成扩散模型生成多张图像,并结合辅助图像和文本语义特征选择“最佳”图像,以最小化重建误差。此外,本文将多模态语义通信系统(MMSemCom)扩展到多用户场景,实现正交传输。实验结果表明,该框架与现有通信系统相比,在提高图像传输的保真度和稳健性的同时,在低信噪比(SNR)条件下也能保持高性能。
Key Takeaways
- 语义通信(SemCom)作为下一代通信系统的有前途的技术,允许在接收端进行语义特征的恢复。
- 现有研究主要使用单一类型的语义信息,可能无法充分捕捉全面准确的语义信息,存在性能瓶颈。
- 本文提出了一个使用多模态信息监督生成图像的SemCom框架,该框架在传输前提取图像和文本级别的语义特征。
- 接收端采用生成扩散模型生成多张图像,并结合图像和文本语义特征选择最佳图像。
- 该框架通过最小化重建误差,确保了语义特征的准确提取和高保真图像重建。
- 框架扩展到了多用户场景,实现正交传输。
点此查看论文截图
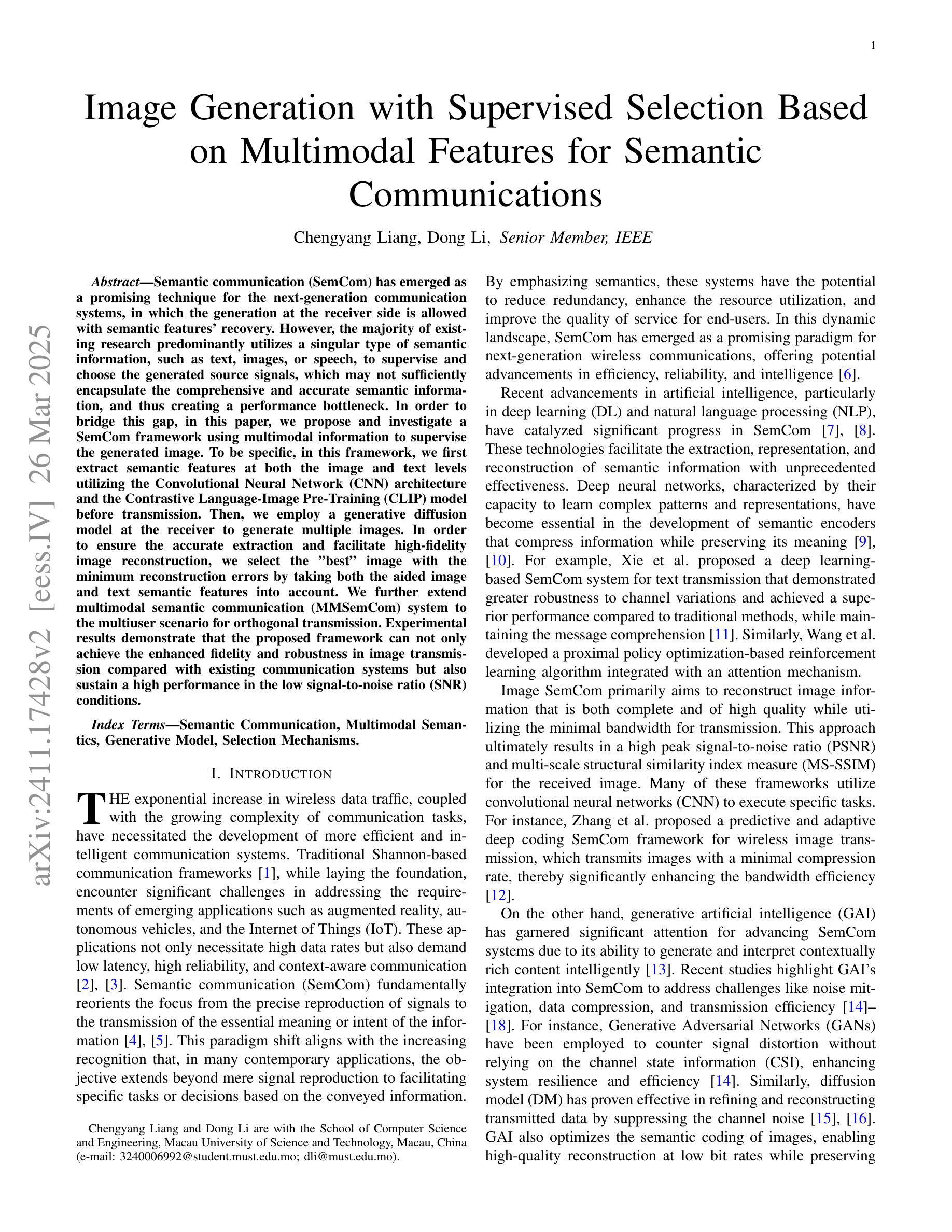

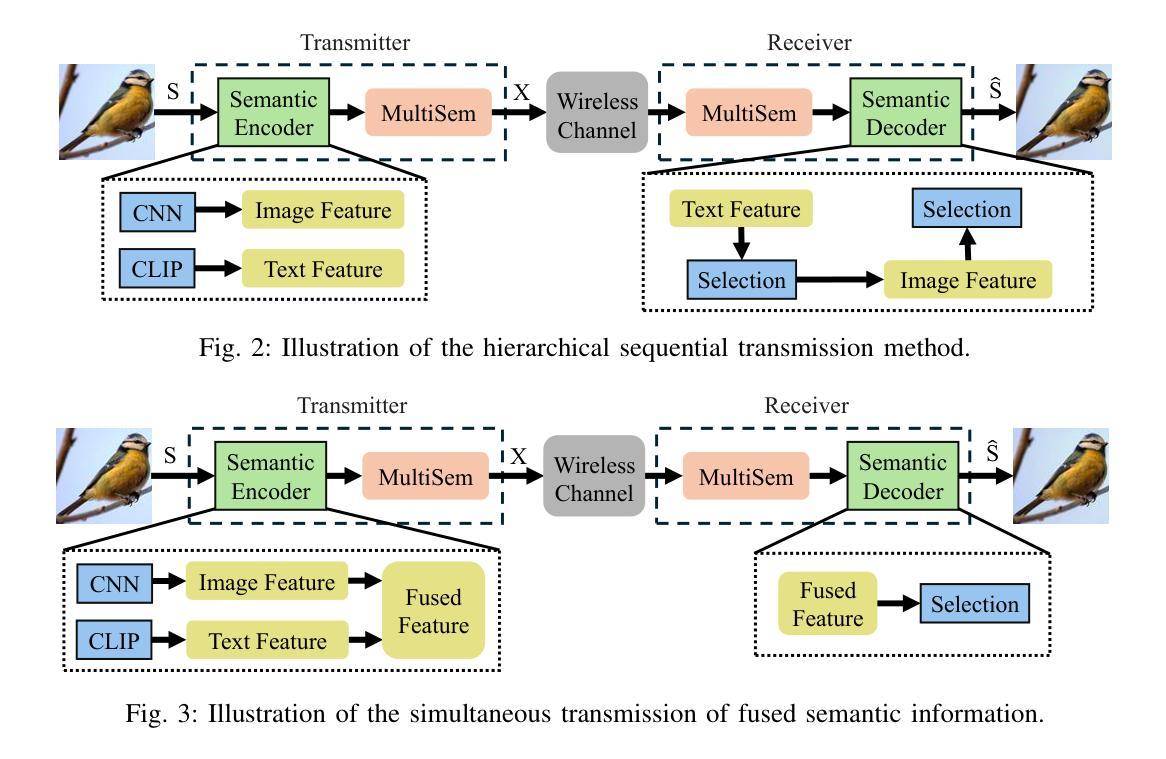
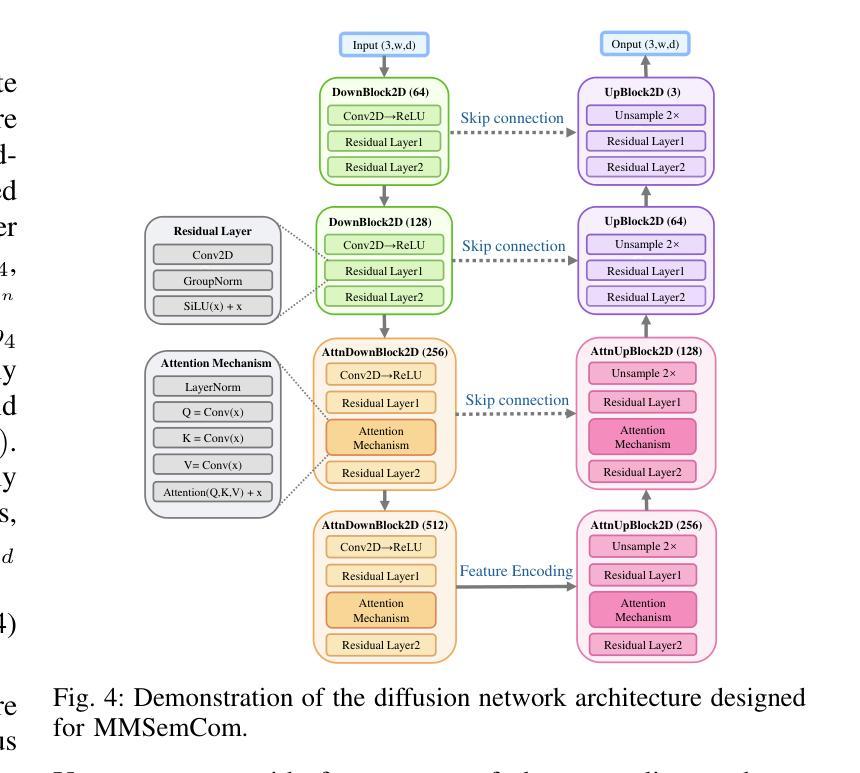