⚠️ 以下所有内容总结都来自于 大语言模型的能力,如有错误,仅供参考,谨慎使用
🔴 请注意:千万不要用于严肃的学术场景,只能用于论文阅读前的初筛!
💗 如果您觉得我们的项目对您有帮助 ChatPaperFree ,还请您给我们一些鼓励!⭐️ HuggingFace免费体验
2025-03-28 更新
Perceptually Accurate 3D Talking Head Generation: New Definitions, Speech-Mesh Representation, and Evaluation Metrics
Authors:Lee Chae-Yeon, Oh Hyun-Bin, Han EunGi, Kim Sung-Bin, Suekyeong Nam, Tae-Hyun Oh
Recent advancements in speech-driven 3D talking head generation have made significant progress in lip synchronization. However, existing models still struggle to capture the perceptual alignment between varying speech characteristics and corresponding lip movements. In this work, we claim that three criteria – Temporal Synchronization, Lip Readability, and Expressiveness – are crucial for achieving perceptually accurate lip movements. Motivated by our hypothesis that a desirable representation space exists to meet these three criteria, we introduce a speech-mesh synchronized representation that captures intricate correspondences between speech signals and 3D face meshes. We found that our learned representation exhibits desirable characteristics, and we plug it into existing models as a perceptual loss to better align lip movements to the given speech. In addition, we utilize this representation as a perceptual metric and introduce two other physically grounded lip synchronization metrics to assess how well the generated 3D talking heads align with these three criteria. Experiments show that training 3D talking head generation models with our perceptual loss significantly improve all three aspects of perceptually accurate lip synchronization. Codes and datasets are available at https://perceptual-3d-talking-head.github.io/.
近期语音驱动的3D动态头部生成技术的进展在唇同步方面取得了显著进步。然而,现有模型仍然难以捕捉不同语音特征与相应唇部运动之间的感知对齐。在这项工作中,我们认为三个标准——时间同步、唇可读性和表现力——对于实现感知准确的唇部运动至关重要。受存在一个符合这三个标准的理想表示空间的假设的驱动,我们引入了语音网格同步表示,该表示捕获了语音信号与3D面部网格之间的精细对应关系。我们发现我们学到的表示具有理想的特性,我们将其插入现有模型作为感知损失,以更好地将唇部运动与给定的语音对齐。此外,我们利用这种表示作为感知度量,并引入其他两个基于物理的唇同步度量标准,以评估生成的3D动态头部如何符合这三个标准。实验表明,使用我们的感知损失训练3D动态头部生成模型,在感知准确的唇同步方面三个方面都有显著提高。相关代码和数据集可通过https://perceptual-3d-talking-head.github.io/获取。
论文及项目相关链接
摘要
该论文介绍了在语音驱动的3D谈话头生成领域的最新进展,重点强调了唇同步的重要性。现有模型在捕捉不同语音特征与相应唇动之间的感知对齐方面仍存在困难。本文提出了三个关键标准——时间同步、唇可读性和表现力,对于实现感知准确的唇动至关重要。基于存在一个符合这三个标准的理想表示空间的假设,引入了一种语音网格同步表示,能够捕捉语音信号和3D面部网格之间的精细对应关系。实验表明,使用这种感知损失训练3D谈话头生成模型,能显著提高感知准确的唇同步的三个方面。
关键见解
- 现有模型在捕捉语音特征与唇动之间的感知对齐方面存在挑战。
- 论文提出了时间同步、唇可读性和表现力三个关键标准来衡量感知准确的唇同步。
- 基于存在的理想表示空间的假设,引入了语音网格同步表示法。
- 这种表示法被用作感知损失,以提高现有模型的唇动与语音的对齐程度。
- 还使用该表示法作为感知指标,以及其他两个基于物理的唇同步指标来评估生成的3D谈话头的质量。
- 实验结果显示,使用感知损失训练的模型在三个方面都显著提高了感知准确的唇同步。
- 相关代码和数据集已在https://perceptual-3d-talking-head.github.io/上发布。
点此查看论文截图
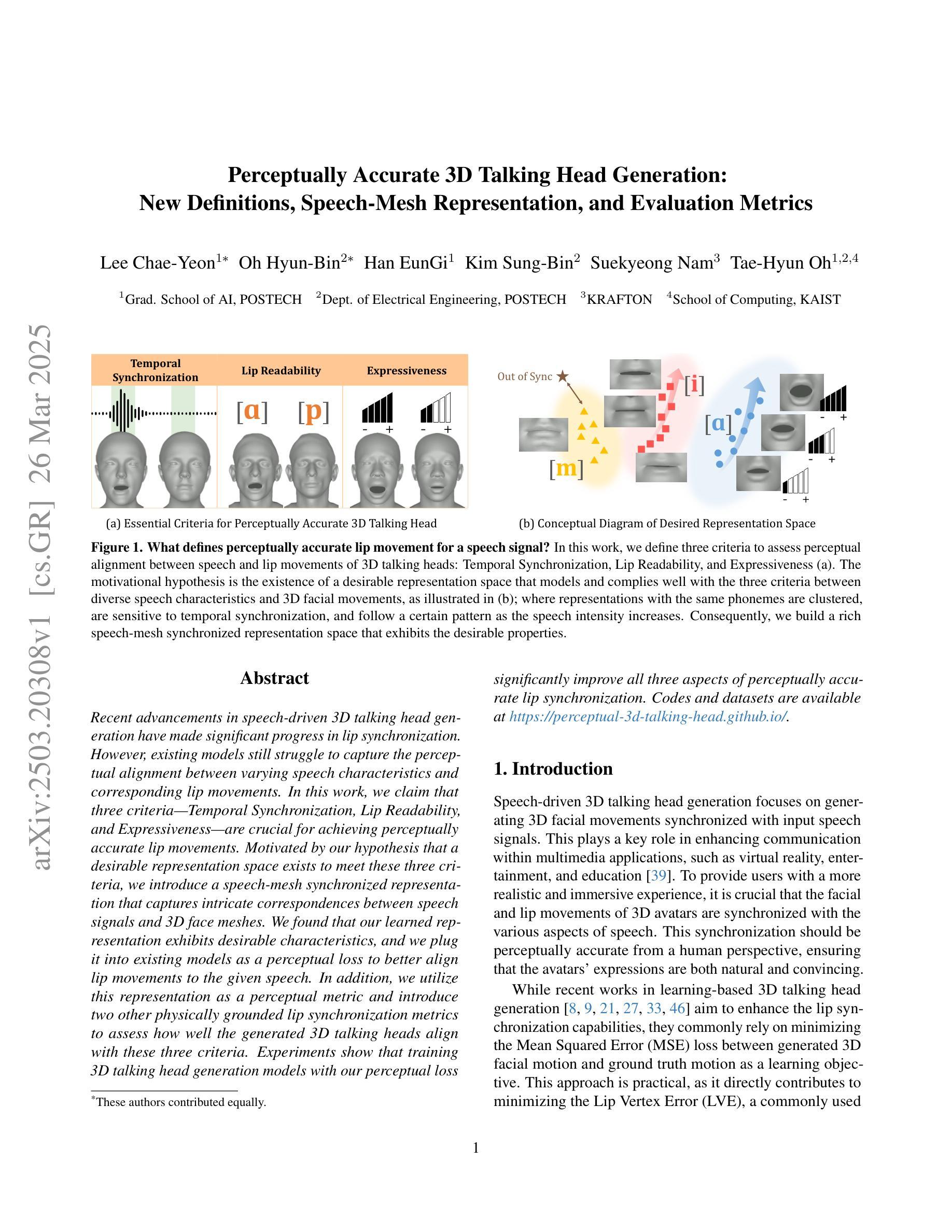
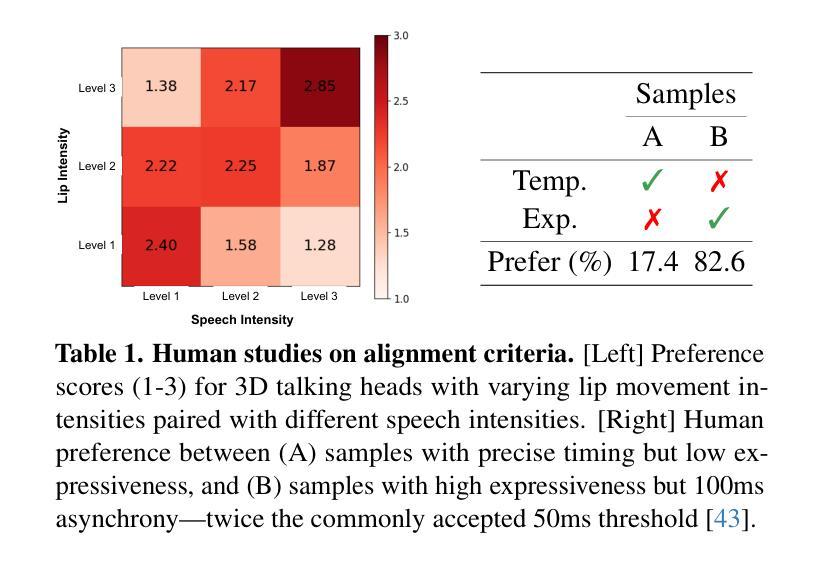
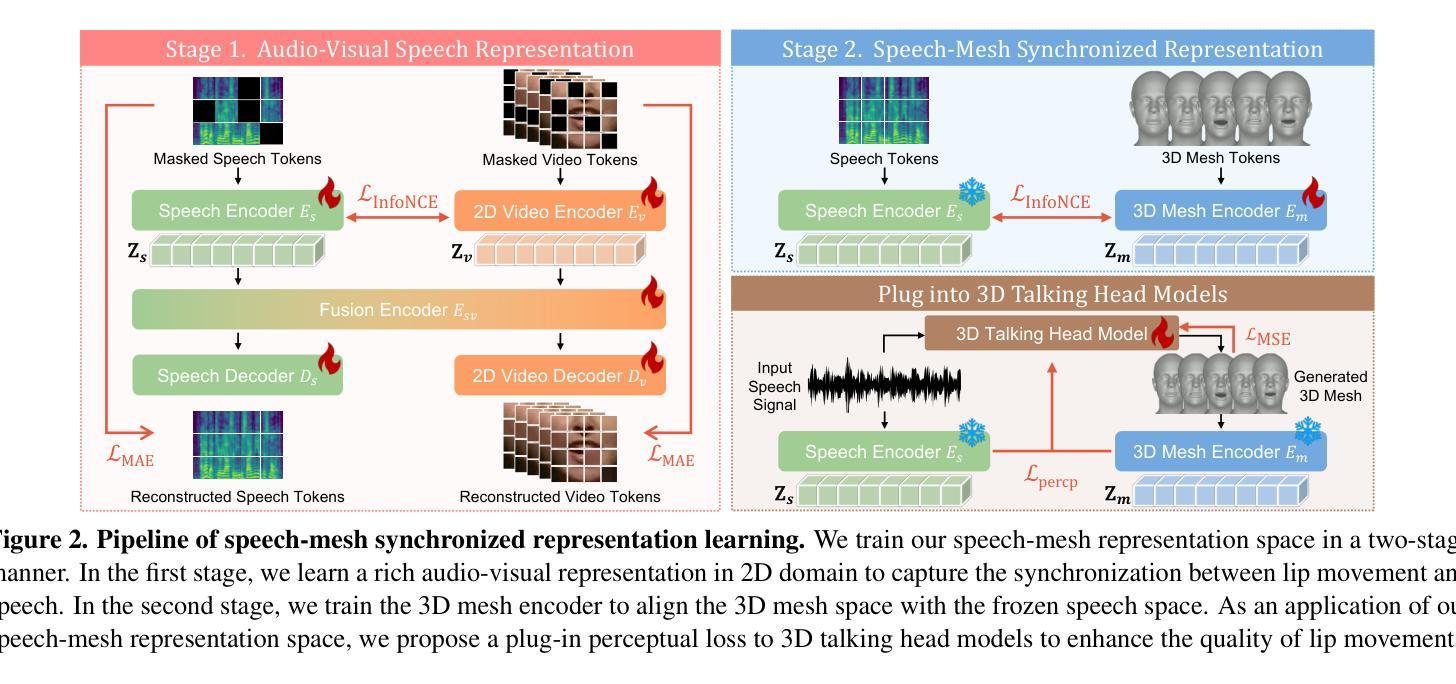
DAWN: Dynamic Frame Avatar with Non-autoregressive Diffusion Framework for Talking Head Video Generation
Authors:Hanbo Cheng, Limin Lin, Chenyu Liu, Pengcheng Xia, Pengfei Hu, Jiefeng Ma, Jun Du, Jia Pan
Talking head generation intends to produce vivid and realistic talking head videos from a single portrait and speech audio clip. Although significant progress has been made in diffusion-based talking head generation, almost all methods rely on autoregressive strategies, which suffer from limited context utilization beyond the current generation step, error accumulation, and slower generation speed. To address these challenges, we present DAWN (Dynamic frame Avatar With Non-autoregressive diffusion), a framework that enables all-at-once generation of dynamic-length video sequences. Specifically, it consists of two main components: (1) audio-driven holistic facial dynamics generation in the latent motion space, and (2) audio-driven head pose and blink generation. Extensive experiments demonstrate that our method generates authentic and vivid videos with precise lip motions, and natural pose/blink movements. Additionally, with a high generation speed, DAWN possesses strong extrapolation capabilities, ensuring the stable production of high-quality long videos. These results highlight the considerable promise and potential impact of DAWN in the field of talking head video generation. Furthermore, we hope that DAWN sparks further exploration of non-autoregressive approaches in diffusion models. Our code will be publicly available at https://github.com/Hanbo-Cheng/DAWN-pytorch.
头部生成技术旨在从单一肖像和语音音频片段中生成生动逼真的头部视频。尽管基于扩散的头部生成技术已经取得了重要进展,但几乎所有方法都依赖于自回归策略,这导致了在当前生成步骤之外上下文利用有限、误差累积和生成速度较慢的问题。为了应对这些挑战,我们推出了DAWN(动态帧非自回归扩散)。这是一个框架,可以一次性生成动态长度的视频序列。具体来说,它包含两个主要组成部分:(1)在潜在运动空间中由音频驱动的面部整体动态生成;(2)由音频驱动的头部姿态和眨眼生成。大量实验表明,我们的方法能够生成真实生动的视频,具有精确的唇部动作和自然姿态/眨眼动作。此外,DAWN具有高速生成能力和强大的外推能力,确保高质量长视频的稳定生产。这些结果凸显了DAWN在头部视频生成领域的巨大潜力和前景。此外,我们希望DAWN能够激发人们对扩散模型中的非自回归方法的进一步探索。我们的代码将在https://github.com/Hanbo-Cheng/DAWN-pytorch上公开提供。
论文及项目相关链接
Summary
新一代动态头像生成技术DAWN能基于单张肖像和语音音频片段生成生动逼真的动态头像视频。该方法克服了现有生成式模型的局限性,实现了全时生成动态长度视频序列的功能。其主要技术包括音频驱动的面部动态生成和头部姿态及眨眼动作驱动。DAWN生成的视频具有逼真的唇动、自然的姿态和眨眼动作,生成速度快,并能稳定生成高质量的长视频。此外,DAWN在扩散模型中的非自回归方法具有巨大的潜力,有望推动该领域的进一步发展。代码公开于Hanbo-Cheng/DAWN-pytorch的GitHub仓库。
Key Takeaways
- DAWN是一种能生成动态头像视频的先进技术,只需单张肖像和语音音频片段。
- 克服了现有生成方法的局限,实现了全时生成动态长度视频序列的功能。
- 包括音频驱动的面部动态生成和头部姿态及眨眼动作驱动两大技术。
- 生成的视频具有逼真的唇动、自然的姿态和眨眼动作。
- 具备快速生成高质量视频的能力,包括长视频的稳定生成。
- DAWN在扩散模型中的非自回归方法具有巨大的潜力,有望推动该领域的进一步发展。
点此查看论文截图
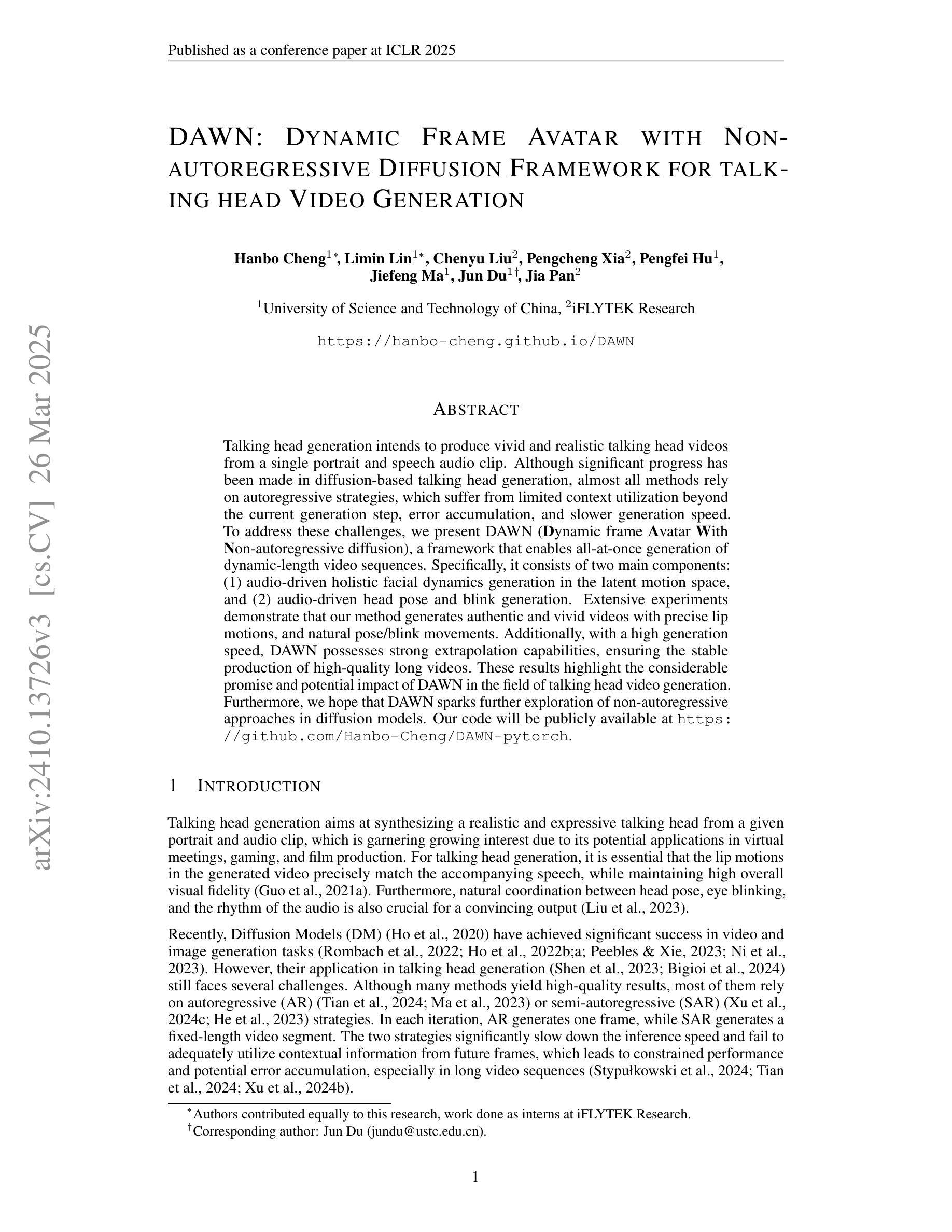
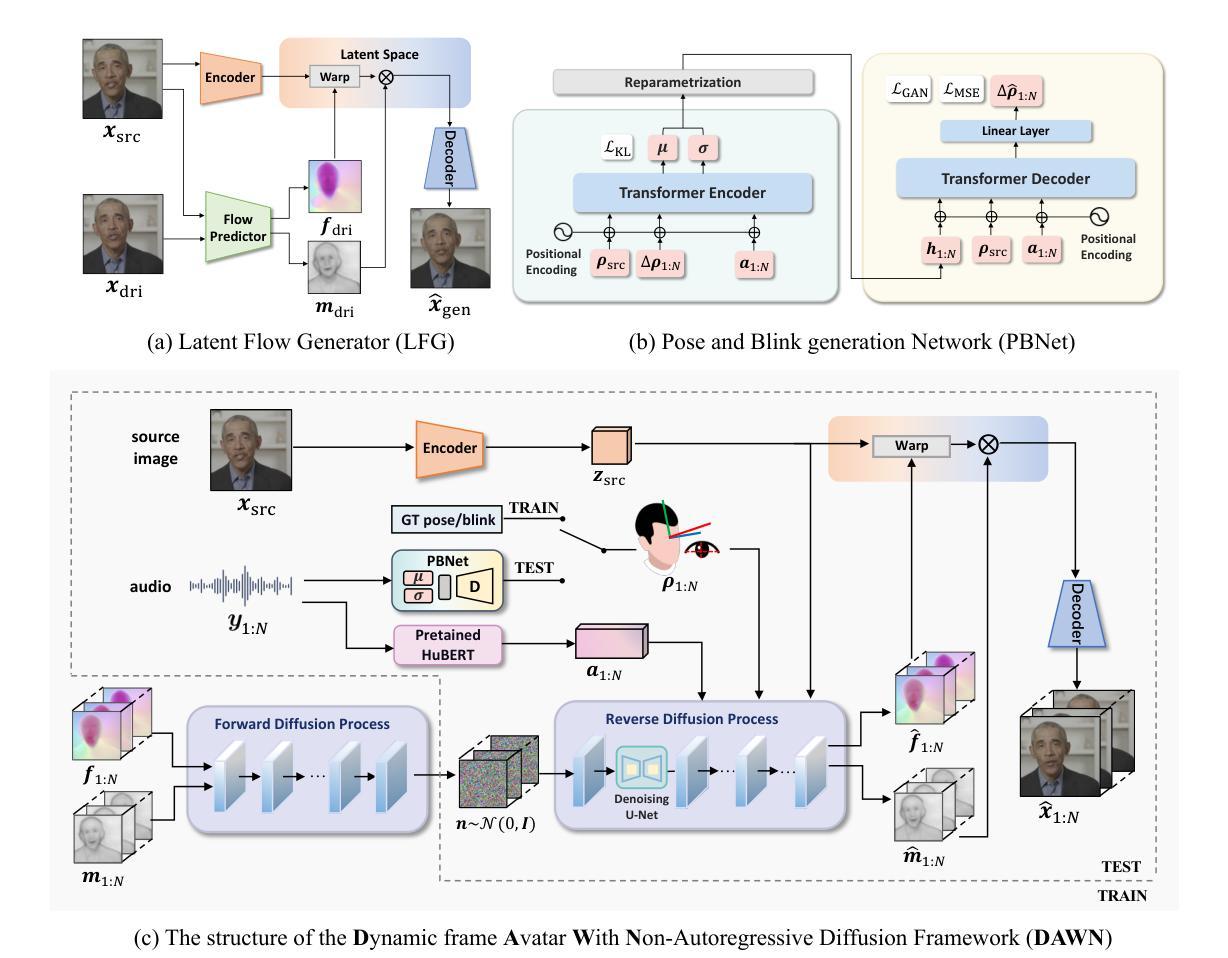
MuseTalk: Real-Time High-Fidelity Video Dubbing via Spatio-Temporal Sampling
Authors:Yue Zhang, Zhizhou Zhong, Minhao Liu, Zhaokang Chen, Bin Wu, Yubin Zeng, Chao Zhan, Yingjie He, Junxin Huang, Wenjiang Zhou
Real-time video dubbing that preserves identity consistency while achieving accurate lip synchronization remains a critical challenge. Existing approaches face a trilemma: diffusion-based methods achieve high visual fidelity but suffer from prohibitive computational costs, while GAN-based solutions sacrifice lip-sync accuracy or dental details for real-time performance. We present MuseTalk, a novel two-stage training framework that resolves this trade-off through latent space optimization and spatio-temporal data sampling strategy. Our key innovations include: (1) During the Facial Abstract Pretraining stage, we propose Informative Frame Sampling to temporally align reference-source pose pairs, eliminating redundant feature interference while preserving identity cues. (2) In the Lip-Sync Adversarial Finetuning stage, we employ Dynamic Margin Sampling to spatially select the most suitable lip-movement-promoting regions, balancing audio-visual synchronization and dental clarity. (3) MuseTalk establishes an effective audio-visual feature fusion framework in the latent space, delivering 30 FPS output at 256*256 resolution on an NVIDIA V100 GPU. Extensive experiments demonstrate that MuseTalk outperforms state-of-the-art methods in visual fidelity while achieving comparable lip-sync accuracy. %The codes and models will be made publicly available upon acceptance. The code is made available at \href{https://github.com/TMElyralab/MuseTalk}{https://github.com/TMElyralab/MuseTalk}
实时视频配音在保持身份一致性同时实现准确的唇部同步仍然是一个关键挑战。现有方法面临一个三元困境:基于扩散的方法虽然视觉保真度高,但计算成本高昂,而基于GAN的解决方案则为了实时性能牺牲了唇同步准确性或牙齿细节。我们提出了MuseTalk,这是一种新型的两阶段训练框架,通过潜在空间优化和时空数据采样策略来解决这一权衡问题。我们的关键创新包括:(1)在面部抽象预训练阶段,我们提出了信息帧采样,以时间上的对齐参考源姿势对,消除冗余特征干扰,同时保留身份线索。(2)在唇同步对抗微调阶段,我们采用动态边距采样,以空间上选择最适合促进唇部运动的区域,平衡音频视频同步和牙齿清晰度。(3)MuseTalk在潜在空间中建立了有效的音频视频特征融合框架,可在NVIDIA V100 GPU上以256*256分辨率输出30 FPS。大量实验表明,MuseTalk在视觉保真度方面优于最先进的方法,同时实现相当的唇同步准确性。%代码和模型在接受后将公开发布。代码可通过以下链接获取:https://github.com/TMElyralab/MuseTalk
论文及项目相关链接
PDF 15 pages, 4 figures
Summary
本文提出了一种名为MuseTalk的新型两阶段训练框架,解决了实时视频配音中身份一致性保持与准确唇同步的关键挑战。通过潜在空间优化和时空数据采样策略,实现了高视觉保真度和实时性能之间的平衡。其核心创新包括面部抽象预训练阶段的参考源姿势对的时间对齐,以及唇同步对抗微调阶段的动态边界采样,以实现音频视频的同步和牙齿清晰度之间的平衡。MuseTalk在潜在空间建立了有效的音频视觉特征融合框架,可在NVIDIA V100 GPU上以30 FPS输出256*256分辨率的图像。实验表明,MuseTalk在视觉保真度方面优于现有技术,同时达到了相当的唇同步精度。
Key Takeaways
- MuseTalk是一个两阶段训练框架,用于解决实时视频配音中的身份一致性和准确唇同步的挑战。
- 在面部抽象预训练阶段,通过信息帧采样实现参考源姿势对的时间对齐,保留身份线索,消除冗余特征干扰。
- 在唇同步对抗微调阶段,采用动态边界采样,平衡音频视频的同步和牙齿清晰度。
- MuseTalk建立了有效的音频视觉特征融合框架,可在NVIDIA V100 GPU上实现30 FPS的高分辨率输出。
- MuseTalk在视觉保真度方面表现出卓越性能,同时达到了相当的唇同步精度。
- 该方法的代码和模型在接收后将公开发布,目前可访问链接:https://github.com/TMElyralab/MuseTalk。
点此查看论文截图
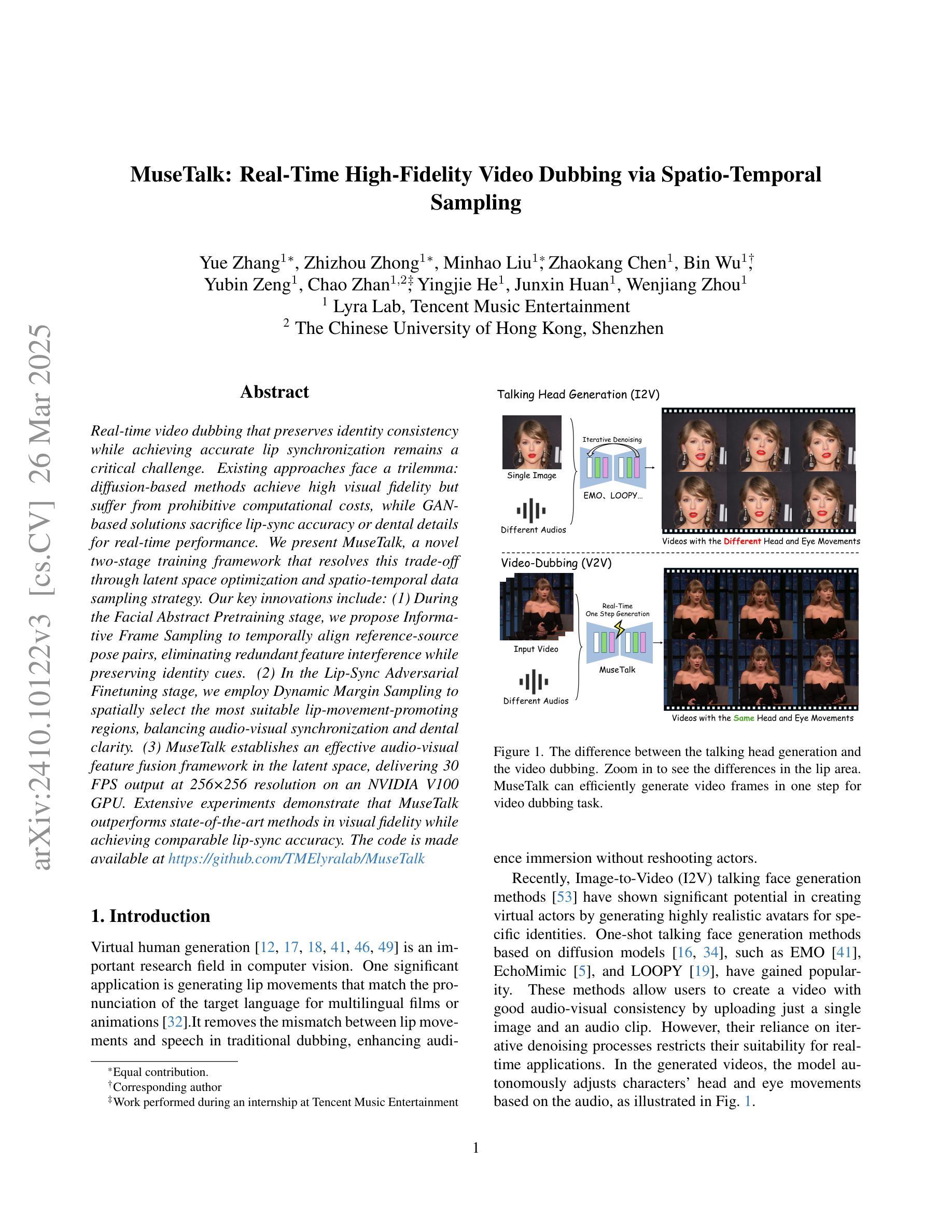
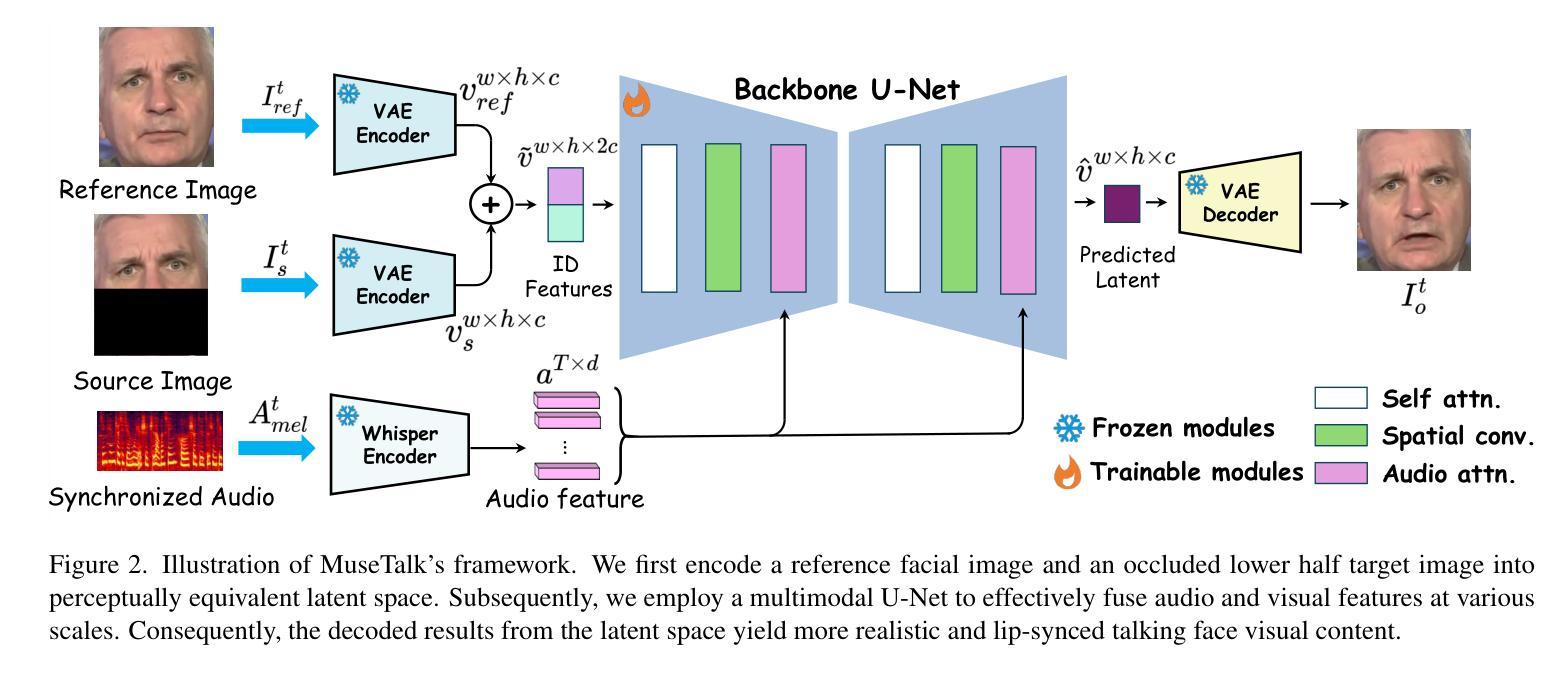
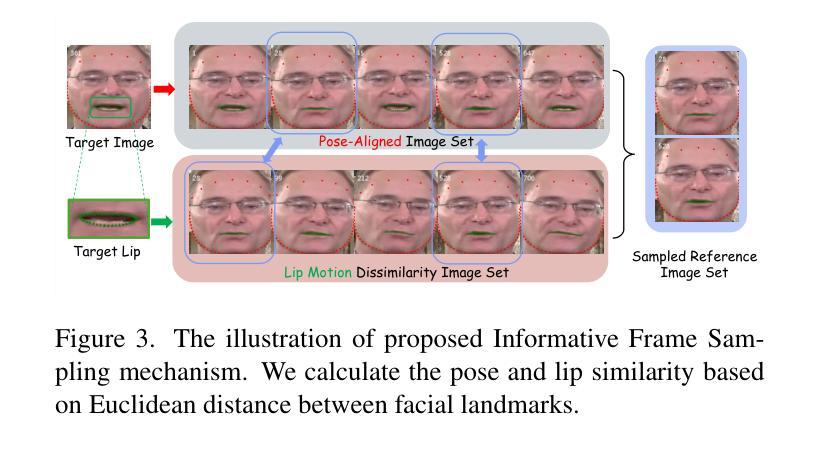
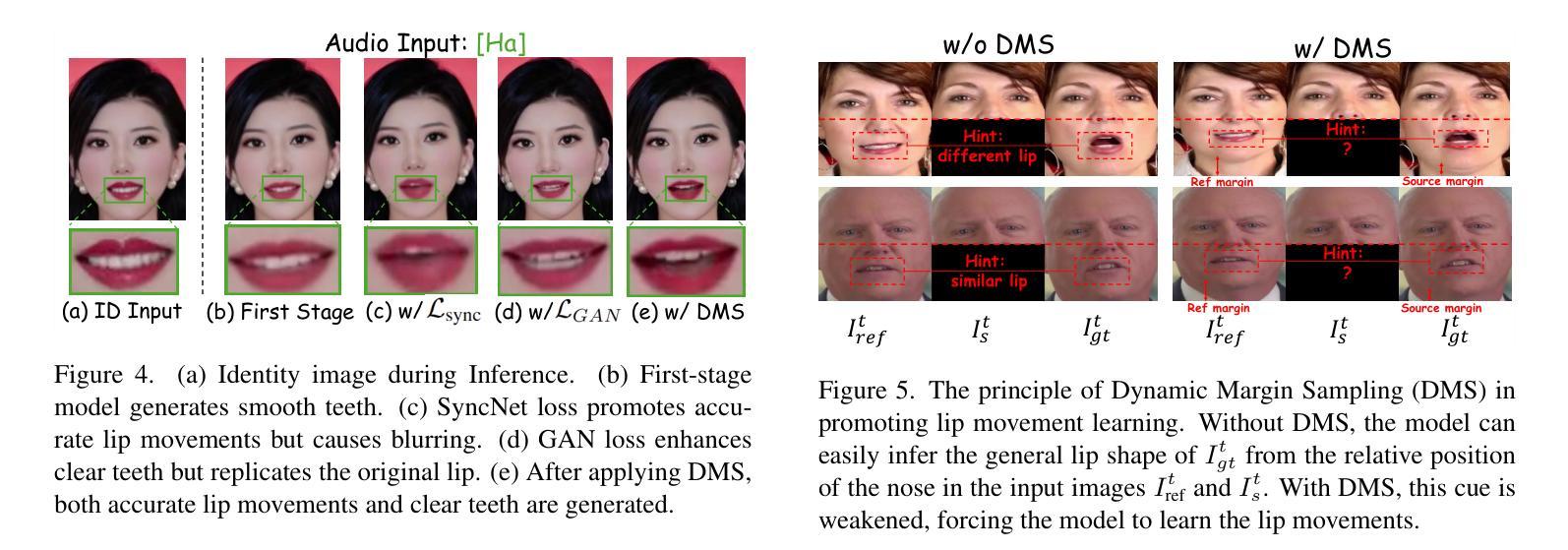
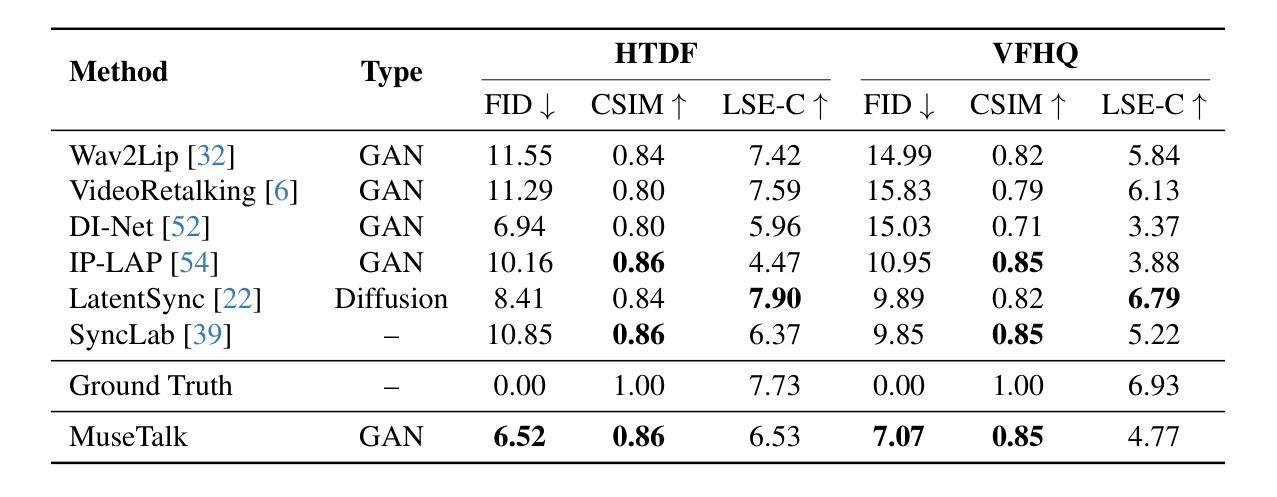