⚠️ 以下所有内容总结都来自于 大语言模型的能力,如有错误,仅供参考,谨慎使用
🔴 请注意:千万不要用于严肃的学术场景,只能用于论文阅读前的初筛!
💗 如果您觉得我们的项目对您有帮助 ChatPaperFree ,还请您给我们一些鼓励!⭐️ HuggingFace免费体验
2025-03-29 更新
Multi-View and Multi-Scale Alignment for Contrastive Language-Image Pre-training in Mammography
Authors:Yuexi Du, John Onofrey, Nicha C. Dvornek
Contrastive Language-Image Pre-training (CLIP) demonstrates strong potential in medical image analysis but requires substantial data and computational resources. Due to these restrictions, existing CLIP applications in medical imaging focus mainly on modalities like chest X-rays that have abundant image-report data available, leaving many other important modalities underexplored. Here, we propose one of the first adaptations of the full CLIP model to mammography, which presents significant challenges due to labeled data scarcity, high-resolution images with small regions of interest, and class-wise imbalance. We first develop a specialized supervision framework for mammography that leverages its multi-view nature. Furthermore, we design a symmetric local alignment module to better focus on detailed features in high-resolution images. Lastly, we incorporate a parameter-efficient fine-tuning approach for large language models pre-trained with medical knowledge to address data limitations. Our multi-view and multi-scale alignment (MaMA) method outperforms state-of-the-art baselines for three different tasks on two large real-world mammography datasets, EMBED and RSNA-Mammo, with only 52% model size compared with the largest baseline. The code is available at https://github.com/XYPB/MaMA
对比语言图像预训练(CLIP)在医学图像分析领域展现出巨大潜力,但需要大量数据和计算资源。由于这些限制,现有的CLIP在医学成像领域的应用主要集中在如胸部X射线等具有丰富图像报告数据的模态上,而许多其他重要模态尚未得到充分探索。在这里,我们首次提出将完整的CLIP模型应用于乳腺X线摄影的适应性之一,由于标记数据稀缺、高分辨率图像中小区域感兴趣以及类别不平衡等问题,这带来了很大的挑战。我们首先开发了一个针对乳腺X线摄影的专项监督框架,该框架利用乳腺X线摄影的多视图特性。此外,我们设计了一个对称局部对齐模块,以更好地关注高分辨率图像中的详细特征。最后,为了解决数据局限性问题,我们采用了一种针对已用医学知识训练的大型语言模型的参数高效微调方法。我们的多视图和多尺度对齐(MaMA)方法在两个大型真实世界乳腺X线摄影数据集EMBED和RSNA-Mammo的三个不同任务上均优于最新基线技术,并且我们的模型大小只有最大基线技术的52%。代码可通过https://github.com/XYPB/MaMA获取。
论文及项目相关链接
PDF This paper is accepted by IPMI 2025 for Oral Presentation
Summary
本文探索了Contrastive Language-Image Pre-training(CLIP)在医学图像分析领域的应用,特别是在针对乳腺X光图像分析中的挑战。文章提出一种针对乳腺X光图像的CLIP模型适应方法,通过开发专门监督框架、设计对称局部对齐模块以及融入参数高效的微调策略,实现了在两大真实乳腺X光数据集上的优越性能。
Key Takeaways
- CLIP在医学图像分析领域展现潜力,尤其在针对特定模态如乳腺X光图像的应用上。
- 乳腺X光图像分析面临数据稀缺、高分辨率图像中小区域识别及类别不平衡等挑战。
- 提出针对乳腺X光图像的专门监督框架,利用多视角特性。
- 设计对称局部对齐模块,以更好地关注高分辨率图像中的细节特征。
- 融入参数高效的微调策略,以适应大型预训练语言模型在医学知识方面的需求。
- 提出的多视角和多尺度对齐(MaMA)方法在两个大型真实乳腺X光数据集上实现优越性能。
点此查看论文截图
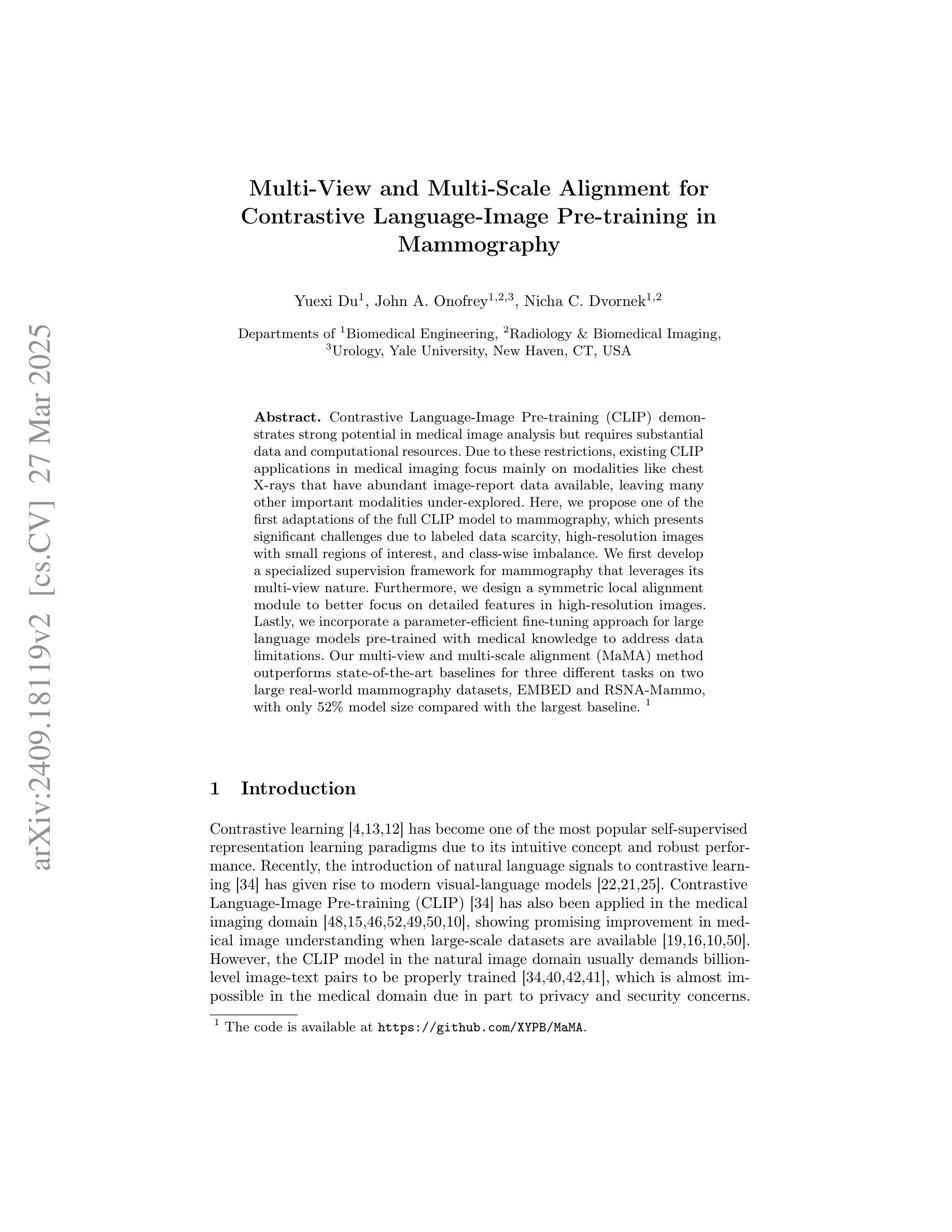
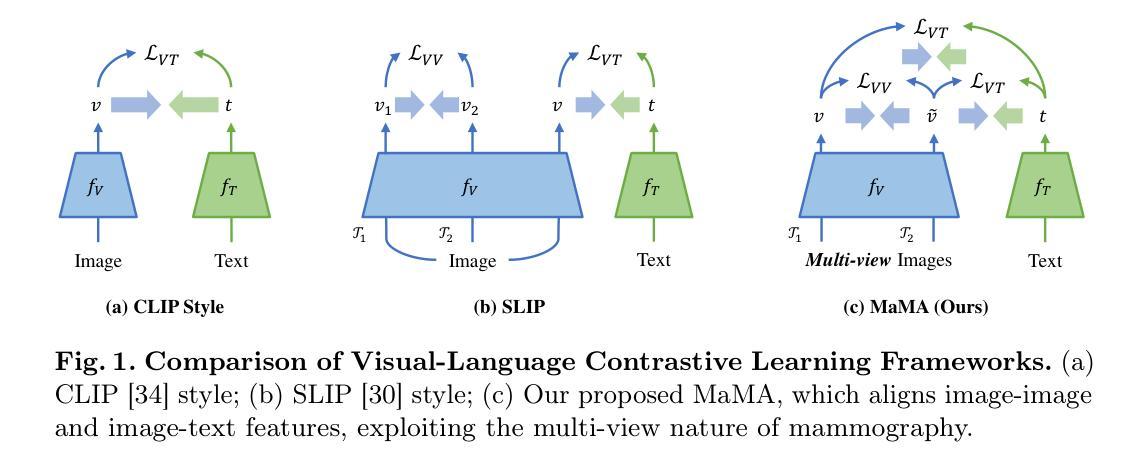
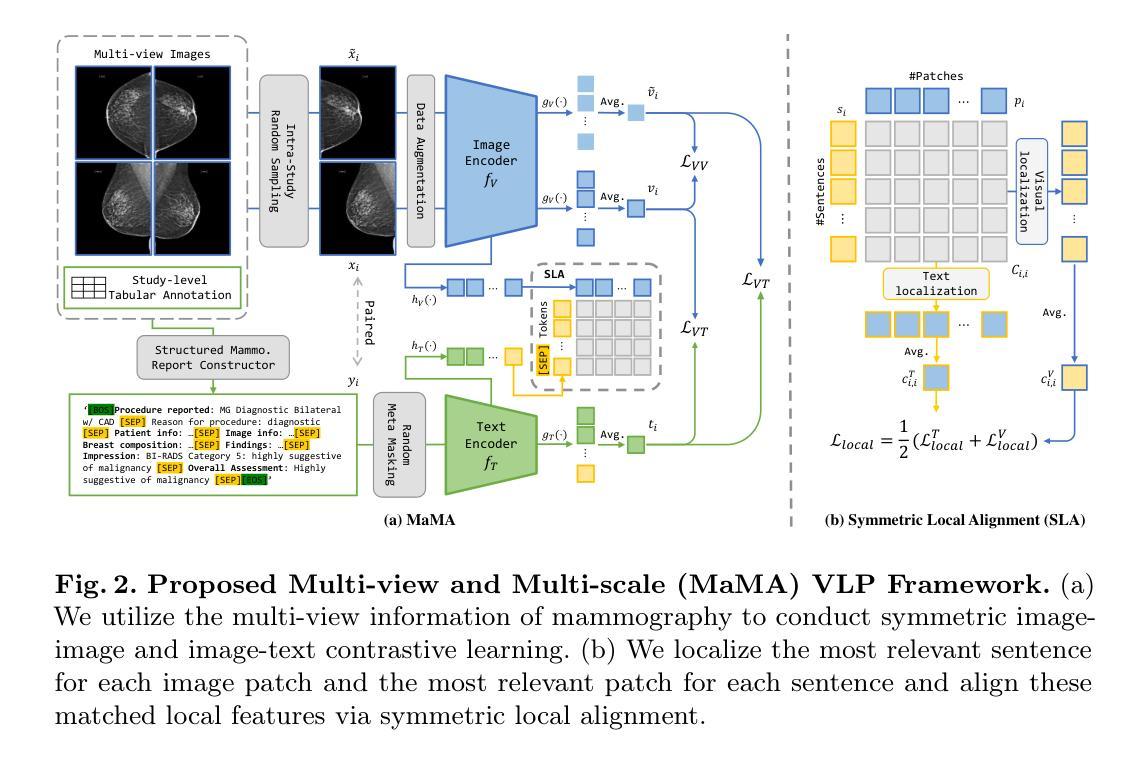