⚠️ 以下所有内容总结都来自于 大语言模型的能力,如有错误,仅供参考,谨慎使用
🔴 请注意:千万不要用于严肃的学术场景,只能用于论文阅读前的初筛!
💗 如果您觉得我们的项目对您有帮助 ChatPaperFree ,还请您给我们一些鼓励!⭐️ HuggingFace免费体验
2025-03-29 更新
Debiasing Kernel-Based Generative Models
Authors:Tian Qin, Wei-Min Huang
We propose a novel two-stage framework of generative models named Debiasing Kernel-Based Generative Models (DKGM) with the insights from kernel density estimation (KDE) and stochastic approximation. In the first stage of DKGM, we employ KDE to bypass the obstacles in estimating the density of data without losing too much image quality. One characteristic of KDE is oversmoothing, which makes the generated image blurry. Therefore, in the second stage, we formulate the process of reducing the blurriness of images as a statistical debiasing problem and develop a novel iterative algorithm to improve image quality, which is inspired by the stochastic approximation. Extensive experiments illustrate that the image quality of DKGM on CIFAR10 is comparable to state-of-the-art models such as diffusion models and GAN models. The performance of DKGM on CelebA 128x128 and LSUN (Church) 128x128 is also competitive. We conduct extra experiments to exploit how the bandwidth in KDE affects the sample diversity and debiasing effect of DKGM. The connections between DKGM and score-based models are also discussed.
我们提出了一种基于内核密度估计(KDE)和随机逼近洞察的新型两阶段生成模型框架,名为去偏内核基生成模型(DKGM)。在DKGM的第一阶段,我们使用KDE来绕过估计数据密度时的障碍,同时不会过多损失图像质量。KDE的一个特点是过度平滑,这会使生成的图像模糊。因此,在第二阶段,我们将减少图像的模糊度过程表述为一个统计去偏问题,并开发了一种新型迭代算法来提高图像质量,该算法受到随机逼近的启发。大量实验表明,DKGM在CIFAR10上的图像质量与最先进的扩散模型和GAN模型相当。DKGM在CelebA 128x128和LSUN(教堂)128x128上的性能也具有竞争力。我们进行了额外的实验,以探讨KDE中的带宽如何影响DKGM的样本多样性和去偏效果。还讨论了DKGM与基于分数的模型之间的联系。
论文及项目相关链接
Summary
提出名为Debiasing Kernel-Based Generative Models(DKGM)的新型两阶段生成模型框架,结合核密度估计(KDE)和随机逼近的见解。第一阶段使用KDE绕过估计数据密度的障碍,同时尽量不损失图像质量。KDE的过度平滑特性会导致生成的图像模糊,因此在第二阶段,将减少图像模糊的过程制定为统计偏差问题,并开发了一种受随机逼近启发的新型迭代算法以提高图像质量。实验表明,DKGM在CIFAR10上的图像质量与最先进的扩散模型和GAN模型相当,在CelebA 128x128和LSUN(Church)128x128上的性能也具有竞争力。
Key Takeaways
- 提出了名为DKGM的新型两阶段生成模型框架,结合了核密度估计和随机逼近的方法。
- 第一阶段使用KDE绕过数据密度估计的障碍,同时保持较高的图像质量。
- KDE的过度平滑问题导致生成的图像模糊,第二阶段通过统计偏差问题的解决方法来减少图像模糊。
- 开发了一种新型迭代算法,受随机逼近启发,以提高图像质量。
- 实验表明DKGM在CIFAR10上的图像质量与最先进模型相当,并且在其他数据集上也有竞争力。
- 进行了关于KDE带宽影响样本多样性和DKGM去偏效果的额外实验。
点此查看论文截图
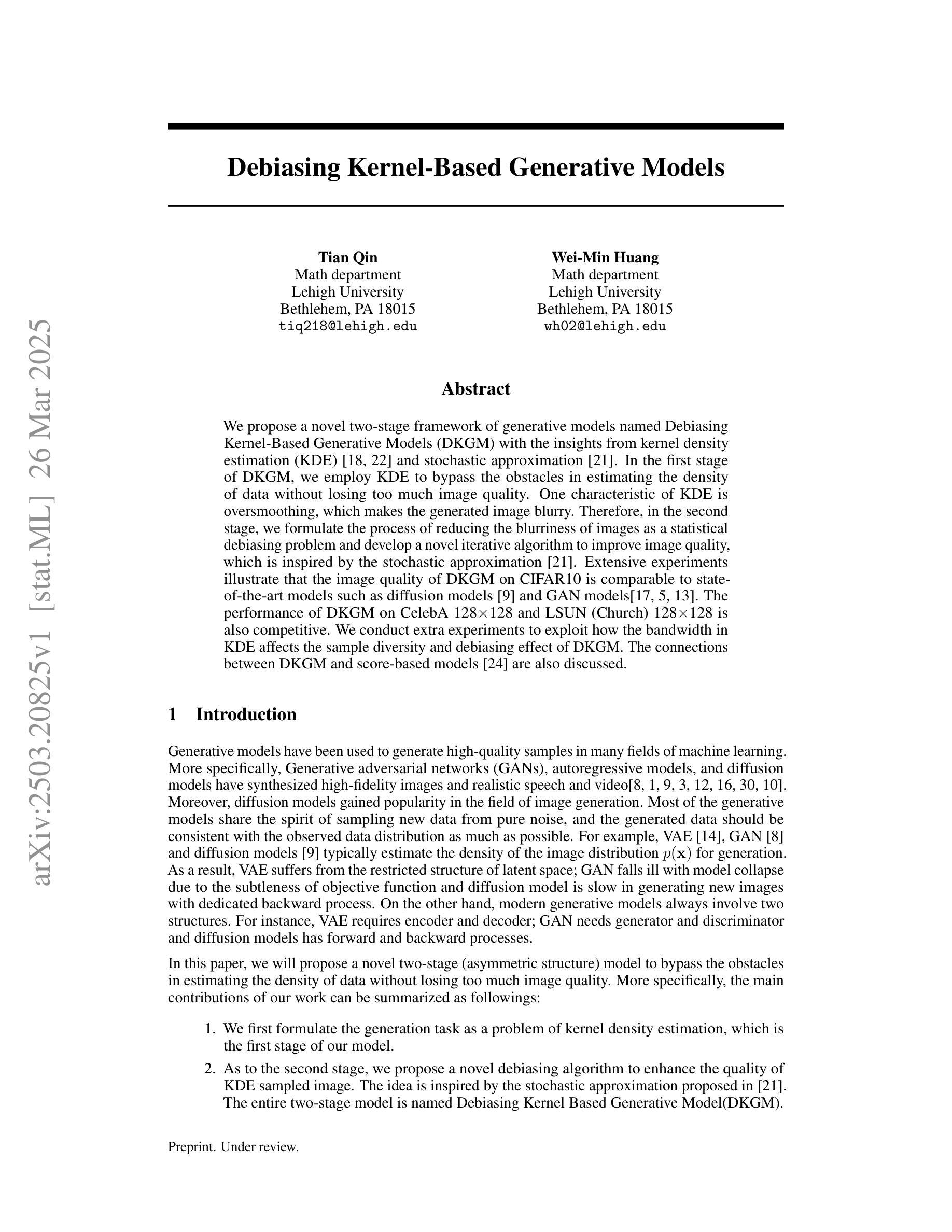
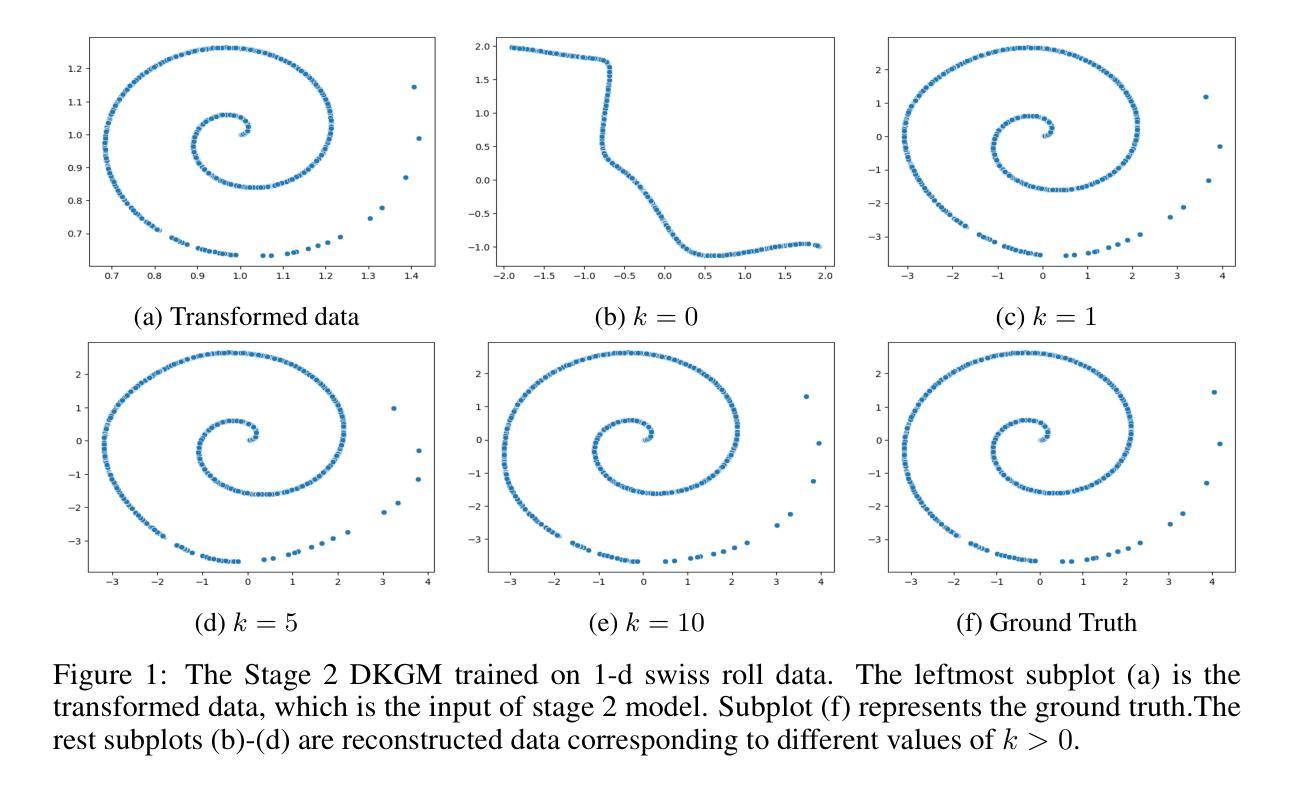