⚠️ 以下所有内容总结都来自于 大语言模型的能力,如有错误,仅供参考,谨慎使用
🔴 请注意:千万不要用于严肃的学术场景,只能用于论文阅读前的初筛!
💗 如果您觉得我们的项目对您有帮助 ChatPaperFree ,还请您给我们一些鼓励!⭐️ HuggingFace免费体验
2025-04-04 更新
GECKO: Gigapixel Vision-Concept Contrastive Pretraining in Histopathology
Authors:Saarthak Kapse, Pushpak Pati, Srikar Yellapragada, Srijan Das, Rajarsi R. Gupta, Joel Saltz, Dimitris Samaras, Prateek Prasanna
Pretraining a Multiple Instance Learning (MIL) aggregator enables the derivation of Whole Slide Image (WSI)-level embeddings from patch-level representations without supervision. While recent multimodal MIL pretraining approaches leveraging auxiliary modalities have demonstrated performance gains over unimodal WSI pretraining, the acquisition of these additional modalities necessitates extensive clinical profiling. This requirement increases costs and limits scalability in existing WSI datasets lacking such paired modalities. To address this, we propose Gigapixel Vision-Concept Knowledge Contrastive pretraining (GECKO), which aligns WSIs with a Concept Prior derived from the available WSIs. First, we derive an inherently interpretable concept prior by computing the similarity between each WSI patch and textual descriptions of predefined pathology concepts. GECKO then employs a dual-branch MIL network: one branch aggregates patch embeddings into a WSI-level deep embedding, while the other aggregates the concept prior into a corresponding WSI-level concept embedding. Both aggregated embeddings are aligned using a contrastive objective, thereby pretraining the entire dual-branch MIL model. Moreover, when auxiliary modalities such as transcriptomics data are available, GECKO seamlessly integrates them. Across five diverse tasks, GECKO consistently outperforms prior unimodal and multimodal pretraining approaches while also delivering clinically meaningful interpretability that bridges the gap between computational models and pathology expertise. Code is made available at https://github.com/bmi-imaginelab/GECKO
预训练多实例学习(MIL)聚合器可从无监督的补丁层面表示中提取全幻灯片图像(WSI)级别的嵌入。虽然最近利用辅助模态的多模态MIL预训练方法已经显示出比单模态WSI预训练更高的性能提升,但这些附加模态的获取需要进行大量的临床分析。这一要求增加了成本并限制了现有缺乏此类配对模态的WSI数据集的可扩展性。为解决这一问题,我们提出了Gigapixel Vision-Concept Knowledge Contrastive预训练方法(GECKO),该方法通过利用可用的WSI生成概念先验来对WSIs进行对齐。首先,我们通过计算每个WSI补丁与预定义病理学概念的文本描述之间的相似性,得出一种本质上可解释的概念先验。然后,GECKO采用双分支MIL网络:一个分支将补丁嵌入聚合成WSI级别的深度嵌入,另一个分支将概念先验聚合成相应的WSI级别概念嵌入。两个聚合嵌入通过使用对比目标进行对齐,从而预训练整个双分支MIL模型。此外,当可用辅助模态(如转录组数据)可用时,GECKO可以无缝集成它们。在五个不同的任务中,GECKO始终优于先前的单模态和多模态预训练方法,同时提供具有临床意义的可解释性,从而缩小了计算模型和病理学专业知识之间的差距。相关代码已发布在https://github.com/bmi-imaginelab/GECKO上可供使用。
论文及项目相关链接
Summary
本文介绍了一种名为GECKO的预训练方法,该方法在无监督的环境下从图像中导出全局级的表示,通过将每个病理图像补丁与预定义的病理学概念文本描述进行对比学习,利用多实例学习(MIL)聚合器进行预训练。即使在没有辅助模态数据的情况下,GECKO也能表现出出色的性能,并能无缝集成辅助模态数据以提升性能。此方法在五个不同任务上表现优越,并提供具有临床意义的解释性。
Key Takeaways
- GECKO使用无监督学习方法进行预训练,能够生成基于补丁的全片级嵌入表示。
- 通过将病理图像补丁与预先定义的病理学概念进行文本描述对比,进行训练模型的学习。
- 采用双分支多实例学习网络结构进行预训练,其中包括一个将补丁嵌入转化为全片级嵌入的分支和一个将概念嵌入转化为全片级概念嵌入的分支。
- 通过对比任务对齐这两个嵌入向量,完成整个模型的预训练。
- 当有辅助模态数据(如转录组学数据)时,GECKO可以无缝集成这些数据以提升性能。
- 在五个不同的任务上,GECKO的表现均优于先前的单模态和多模态预训练方法。
点此查看论文截图
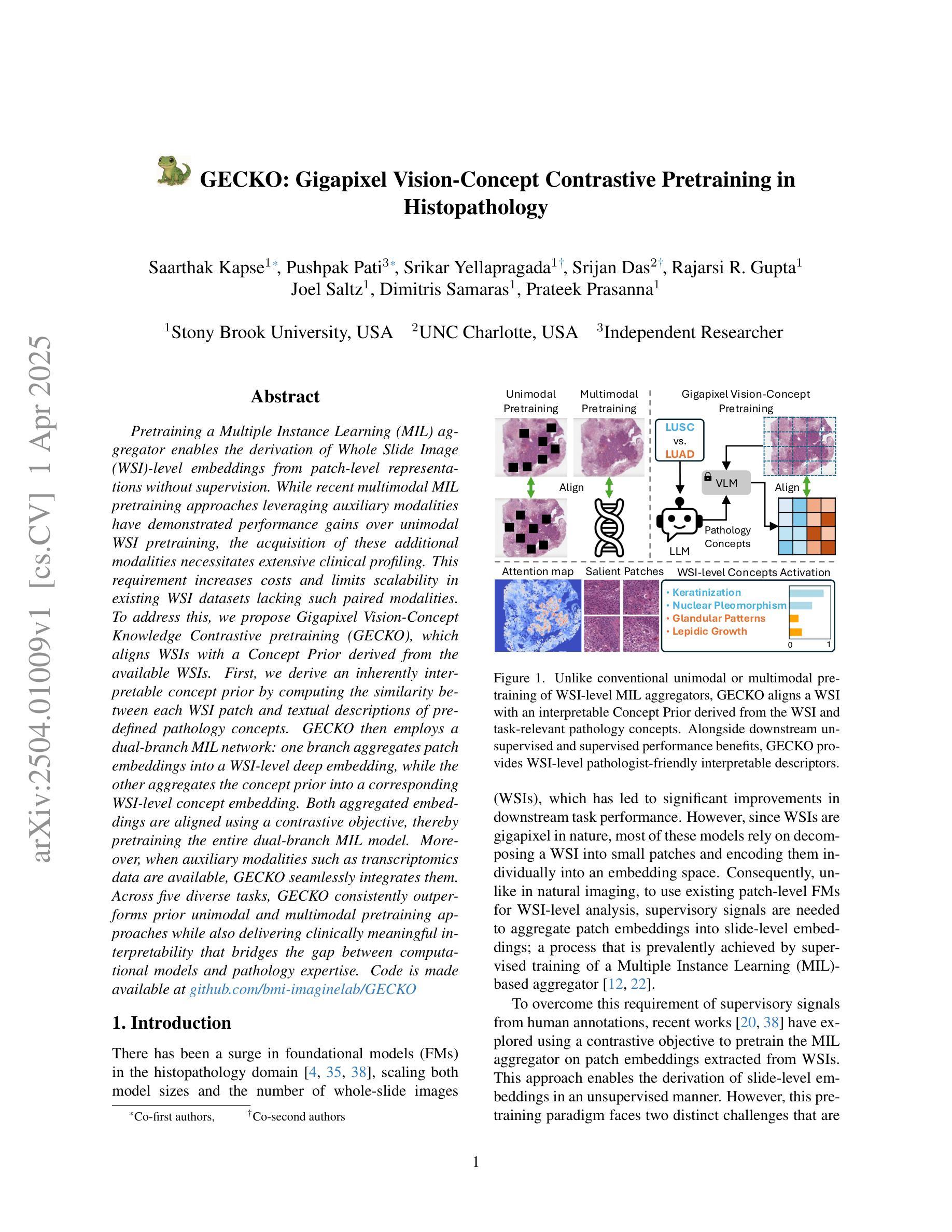
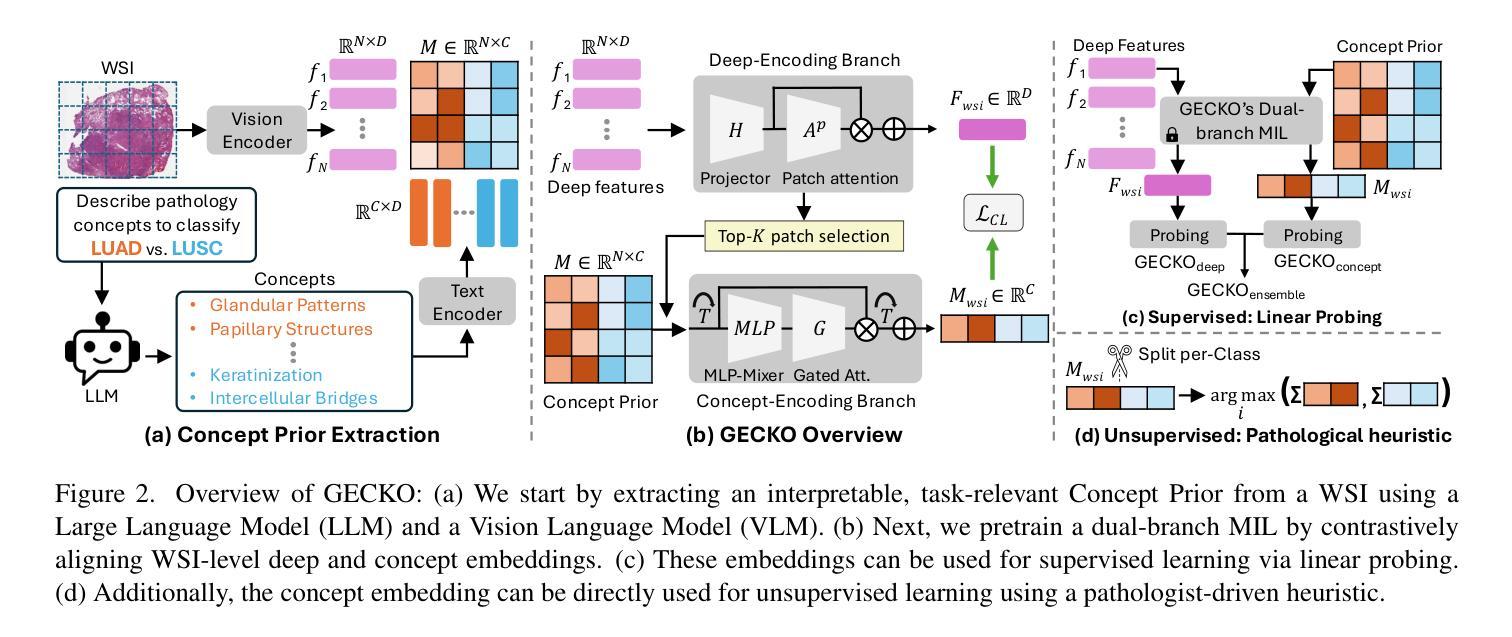
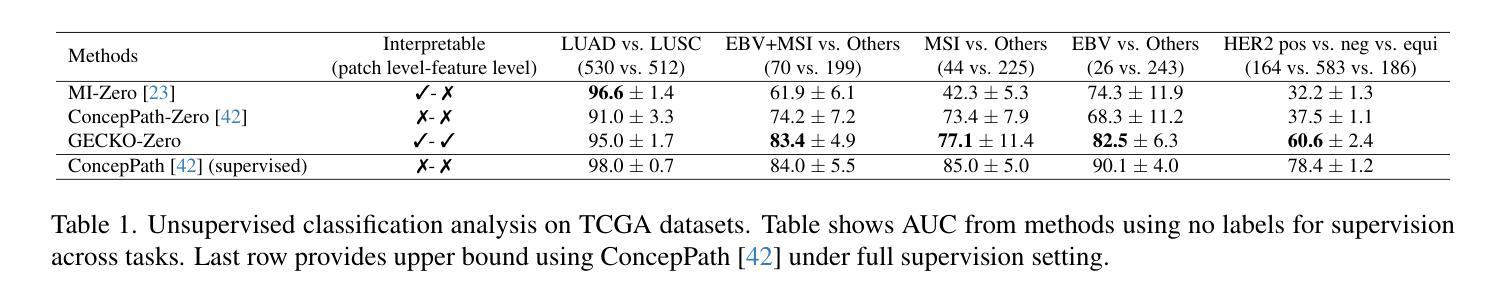
Leveraging Contrast Information for Efficient Document Shadow Removal
Authors:Yifan Liu, Jiancheng Huang, Na Liu, Mingfu Yan, Yi Huang, Shifeng Chen
Document shadows are a major obstacle in the digitization process. Due to the dense information in text and patterns covered by shadows, document shadow removal requires specialized methods. Existing document shadow removal methods, although showing some progress, still rely on additional information such as shadow masks or lack generalization and effectiveness across different shadow scenarios. This often results in incomplete shadow removal or loss of original document content and tones. Moreover, these methods tend to underutilize the information present in the original shadowed document image. In this paper, we refocus our approach on the document images themselves, which inherently contain rich information.We propose an end-to-end document shadow removal method guided by contrast representation, following a coarse-to-fine refinement approach. By extracting document contrast information, we can effectively and quickly locate shadow shapes and positions without the need for additional masks. This information is then integrated into the refined shadow removal process, providing better guidance for network-based removal and feature fusion. Extensive qualitative and quantitative experiments show that our method achieves state-of-the-art performance.
文档阴影是数字化过程中的一大障碍。由于文本和模式阴影覆盖的信息密集,文档阴影去除需要专门的方法。现有的文档阴影去除方法虽然有所进展,但仍需额外的信息,如阴影掩膜,且在不同阴影场景下的通用性和效果有限。这往往导致阴影去除不完全或原始文档内容和色调的损失。此外,这些方法往往没有充分利用原始带阴影的文档图像中的信息。在本文中,我们重新关注文档图像本身,它们固有地包含丰富信息。我们提出了一种由对比表示引导的端到端文档阴影去除方法,采用由粗到细的细化方法。通过提取文档对比信息,我们可以有效且快速地定位阴影的形状和位置,无需额外的掩膜。然后将这些信息融入到精细的阴影去除过程中,为基于网络的去除和特征融合提供更好的指导。大量的定性和定量实验表明,我们的方法达到了最先进的性能。
论文及项目相关链接
Summary
本文提出了一种基于对比表示引导的自端到端文档阴影去除方法。该方法采用粗到细的精细化策略,通过提取文档对比信息有效快速地定位阴影形状和位置,无需额外的遮罩。将阴影信息融入精细化阴影去除过程中,为网络去除和特征融合提供更好的指导,取得了最新的性能表现。
Key Takeaways
- 文档阴影是数字化过程中的主要障碍之一,需要专业化的方法来去除。
- 现有方法常常依赖额外的信息如阴影遮罩,但缺乏在不同阴影场景下的通用性和有效性。
- 现有方法往往未能充分利用原始带阴影文档图像中的信息。
- 本文提出了一种基于对比表示引导的自端到端文档阴影去除方法。
- 该方法采用粗到细的精细化策略,能有效快速地定位阴影形状和位置。
- 提取的阴影信息融入精细化阴影去除过程中,为网络去除和特征融合提供更好的指导。
点此查看论文截图
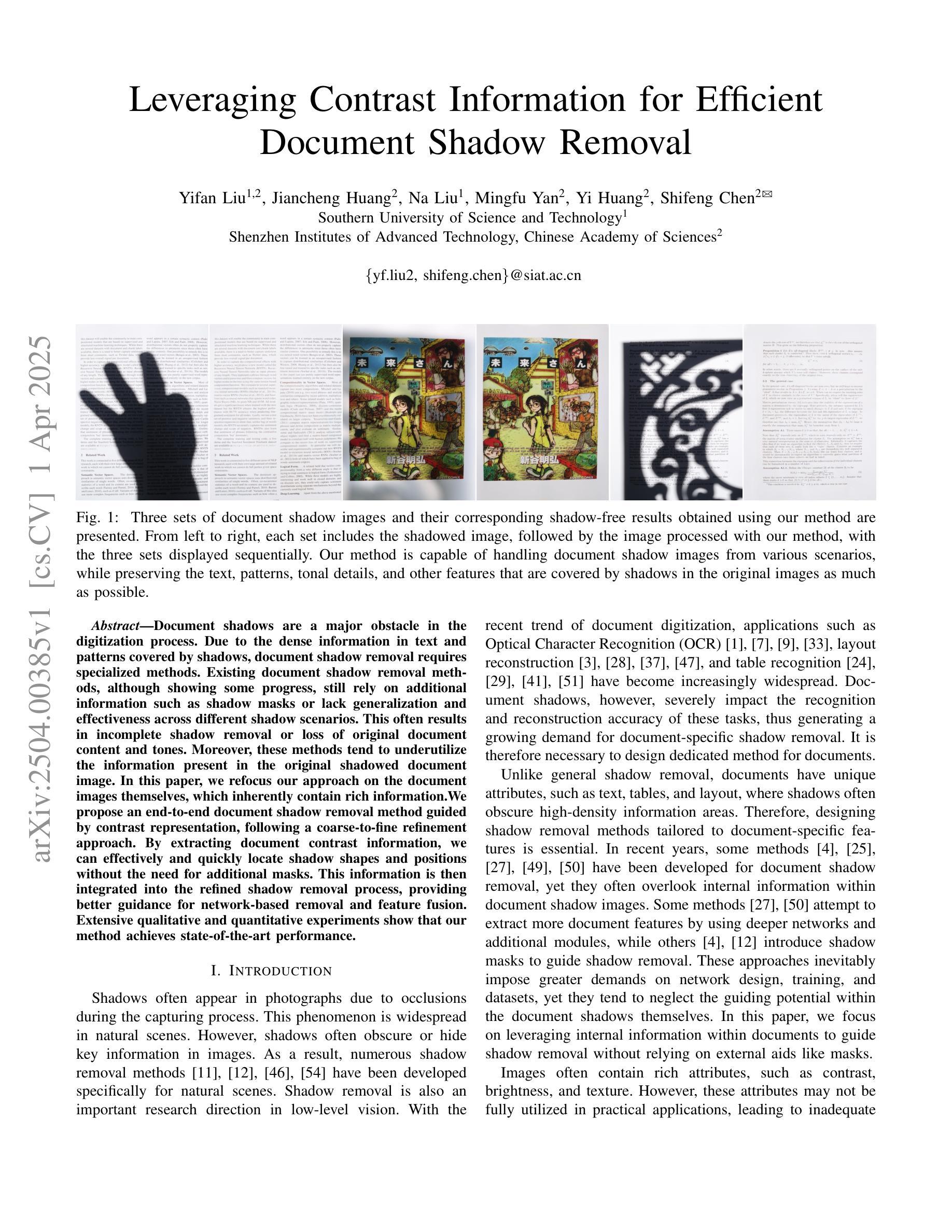
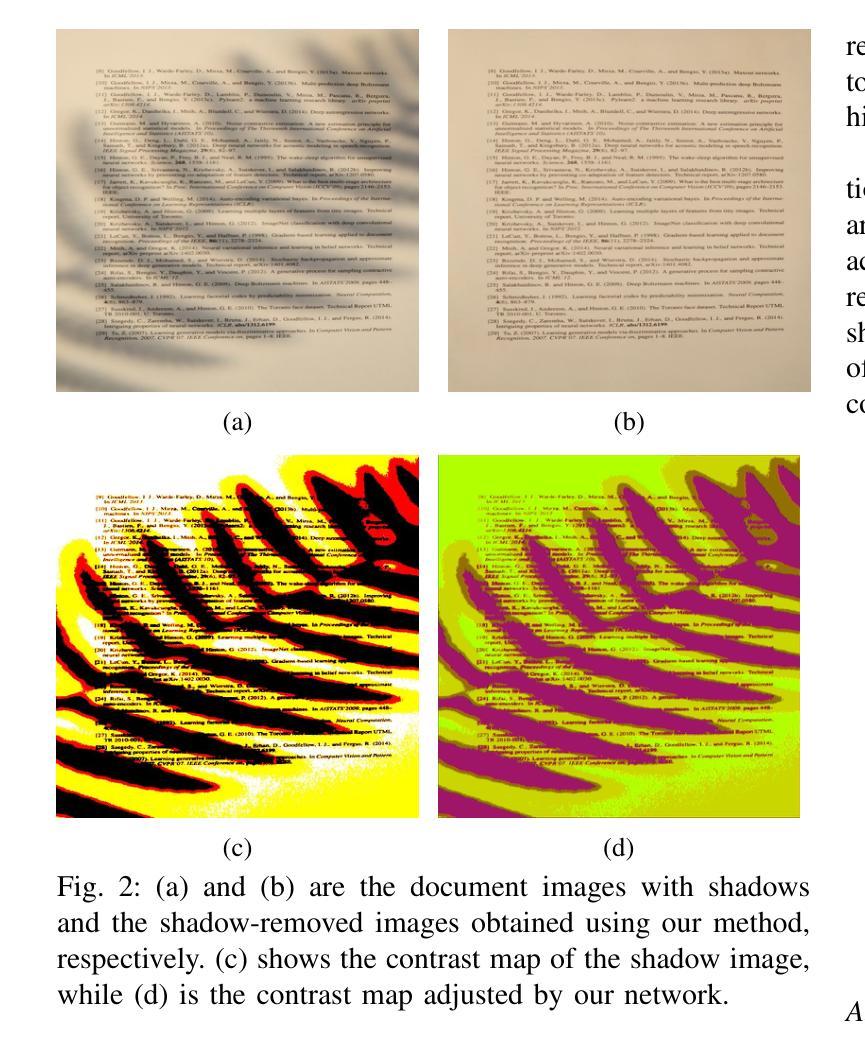
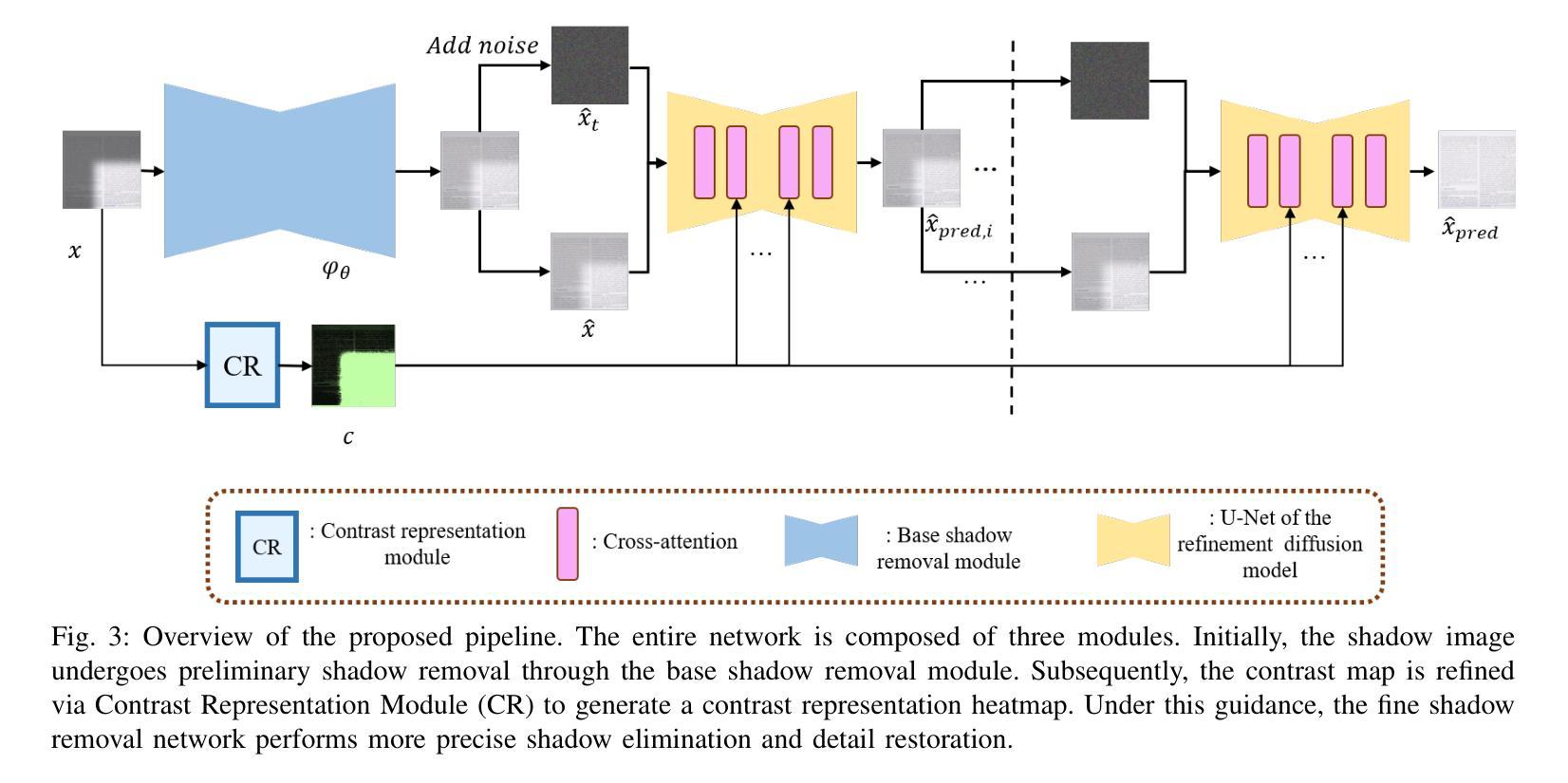
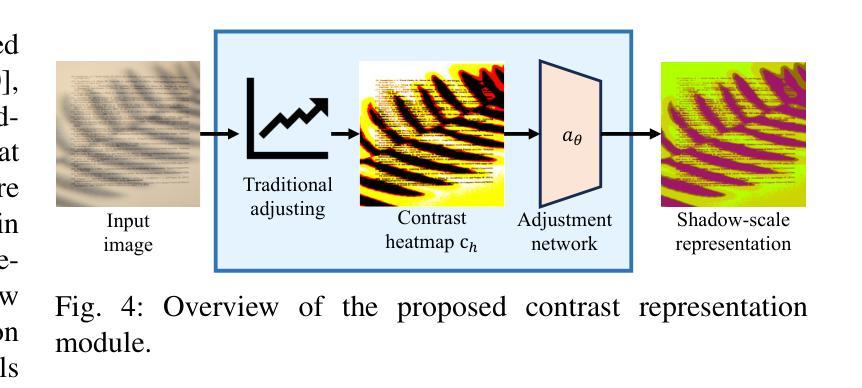
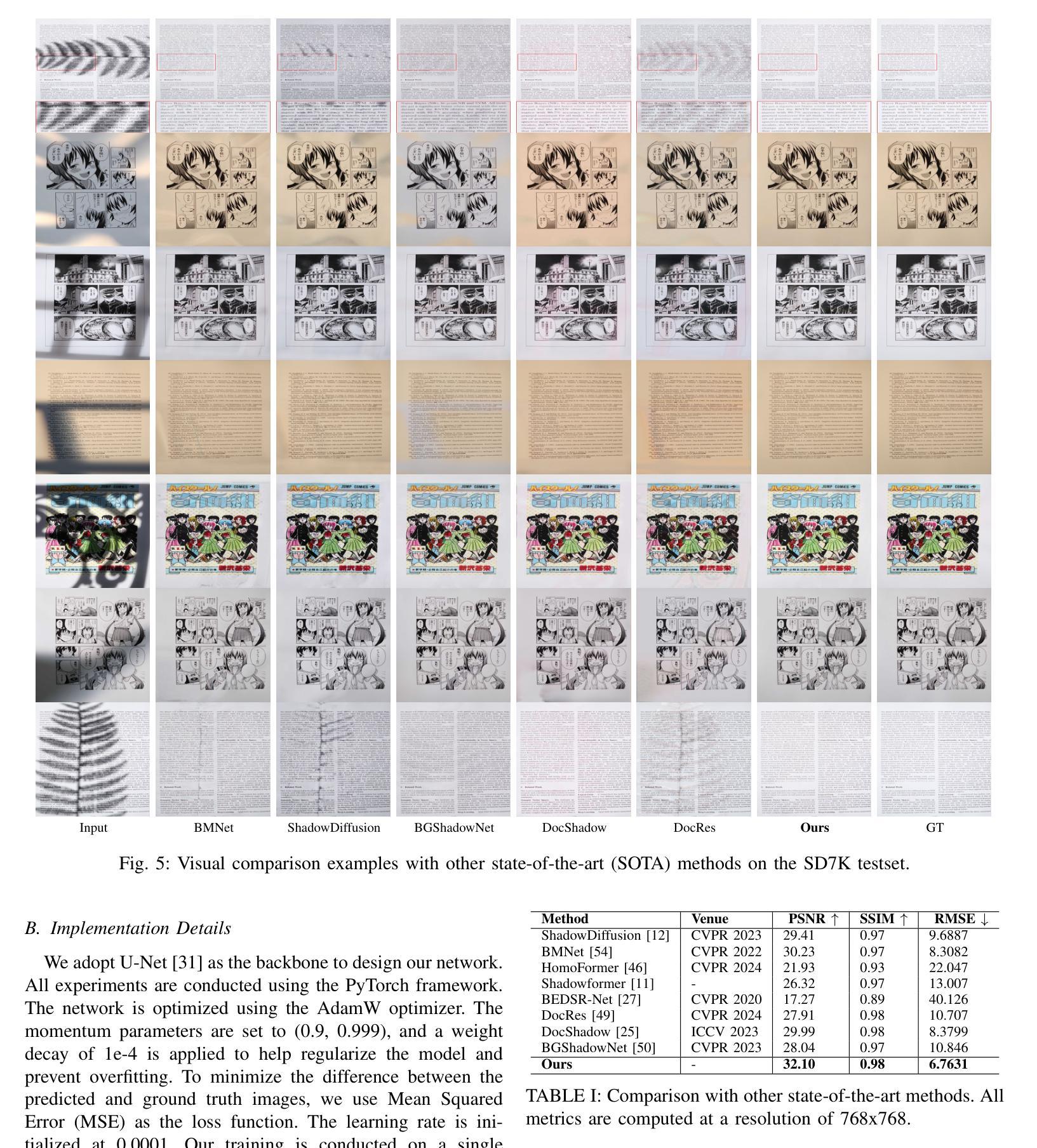
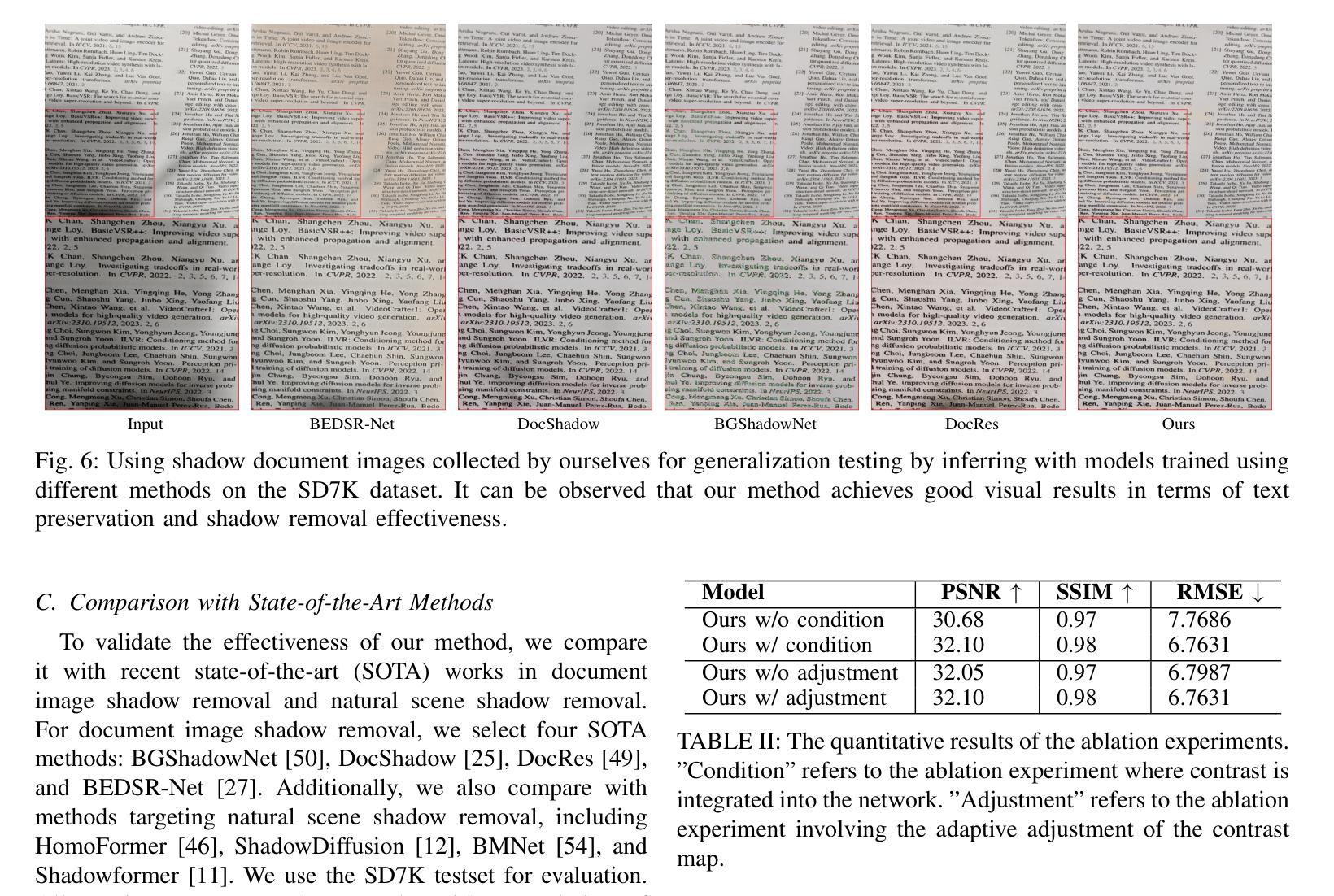
Cyclic Contrastive Knowledge Transfer for Open-Vocabulary Object Detection
Authors:Chuhan Zhang, Chaoyang Zhu, Pingcheng Dong, Long Chen, Dong Zhang
In pursuit of detecting unstinted objects that extend beyond predefined categories, prior arts of open-vocabulary object detection (OVD) typically resort to pretrained vision-language models (VLMs) for base-to-novel category generalization. However, to mitigate the misalignment between upstream image-text pretraining and downstream region-level perception, additional supervisions are indispensable, eg, image-text pairs or pseudo annotations generated via self-training strategies. In this work, we propose CCKT-Det trained without any extra supervision. The proposed framework constructs a cyclic and dynamic knowledge transfer from language queries and visual region features extracted from VLMs, which forces the detector to closely align with the visual-semantic space of VLMs. Specifically, 1) we prefilter and inject semantic priors to guide the learning of queries, and 2) introduce a regional contrastive loss to improve the awareness of queries on novel objects. CCKT-Det can consistently improve performance as the scale of VLMs increases, all while requiring the detector at a moderate level of computation overhead. Comprehensive experimental results demonstrate that our method achieves performance gain of +2.9% and +10.2% AP50 over previous state-of-the-arts on the challenging COCO benchmark, both without and with a stronger teacher model.
在追求检测超出预定义类别范围的无尽对象时,开放词汇表对象检测(OVD)的现有技术通常会借助预训练的视觉语言模型(VLM)来实现从基础到新型类别的泛化。然而,为了减轻上游图像文本预训练和下游区域级别感知之间的不匹配,额外的监督是必不可少的,例如通过自训练策略生成的图像文本对或伪注释。在本研究中,我们提出了无需任何额外监督的CCKT-Det。所提出的框架构建了从语言查询和从VLM中提取的视觉区域特征之间的循环和动态知识转移,这迫使检测器与VLM的视觉语义空间紧密对齐。具体来说,1)我们对查询进行预筛选并注入语义先验来指导查询的学习;2)引入区域对比损失来提高查询对新型对象的感知能力。随着VLM规模的增加,CCKT-Det能够持续提高性能,同时只需要适度的计算开销来运行检测器。综合实验结果表明,我们的方法在具有挑战性的COCO基准测试上,相较于之前的最优方法,提高了+2.9%和+10.2%的AP50性能,分别是在没有和具有更强教师模型的情况下取得的。
论文及项目相关链接
PDF 10 pages, 5 figures, Published as a conference paper at ICLR 2025
Summary:
本文提出一种无需额外监督的CCKT-Det框架,用于开放词汇表对象检测。该框架通过构建循环和动态知识转移,从语言查询到视觉区域特征进行训练,促进检测器与VLMs的视觉语义空间对齐。通过预筛选和注入语义先验来指导查询学习,并引入区域对比损失来提高查询对新型对象的感知能力。实验结果显示,该方法在COCO基准测试上较之前的方法有显著提升。
Key Takeaways:
1.CCKT-Det利用循环和动态知识转移,实现在无需额外监督下提高开放词汇表对象检测性能。
2.该框架利用预训练的视觉语言模型(VLMs)构建知识转移,促进检测器与视觉语言模型的视觉语义空间对齐。
3.通过预筛选和注入语义先验来指导查询学习,提高检测器对未知对象的识别能力。
4.引入区域对比损失,增强查询对新型对象的感知能力。随着视觉语言模型规模的增加,CCKT-Det的性能不断提升。
点此查看论文截图
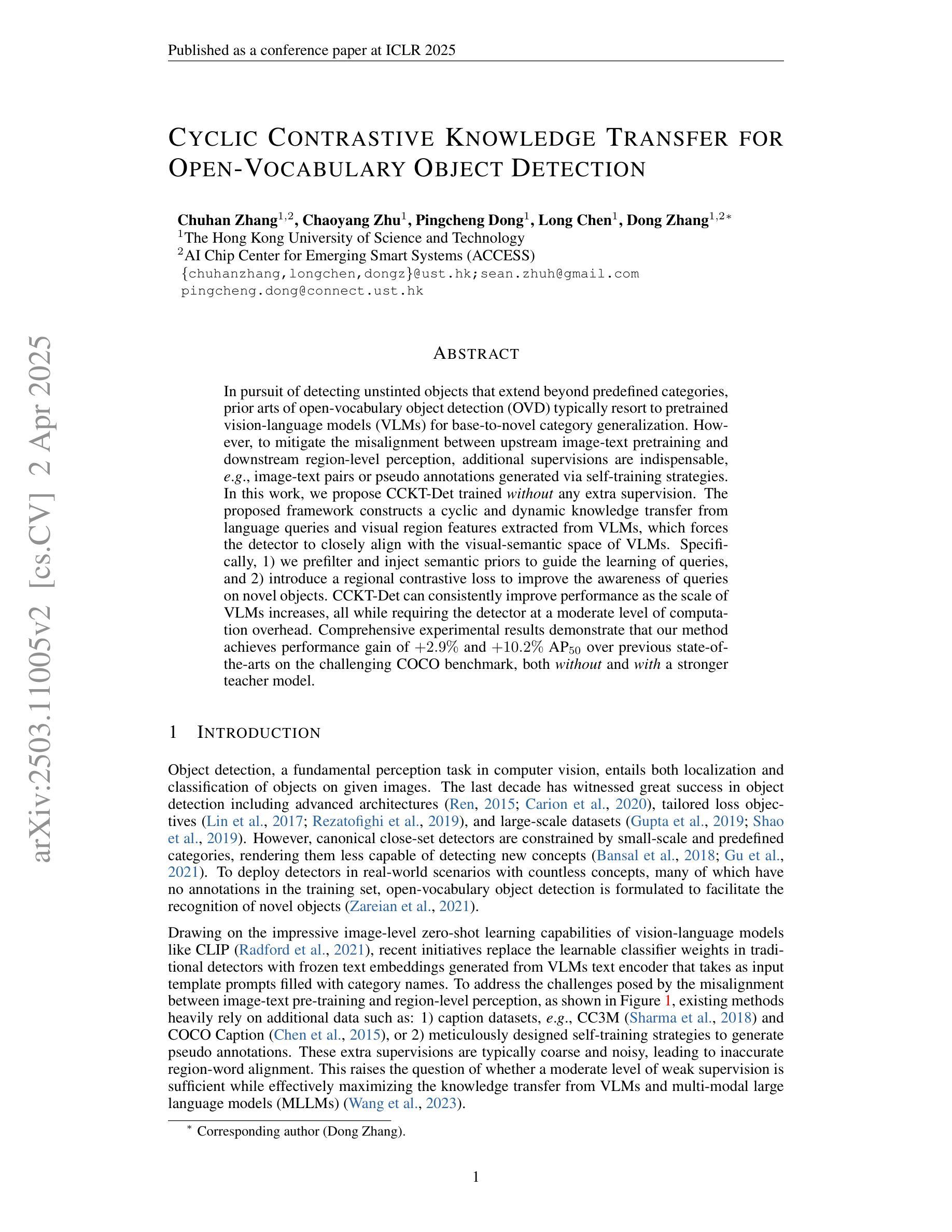
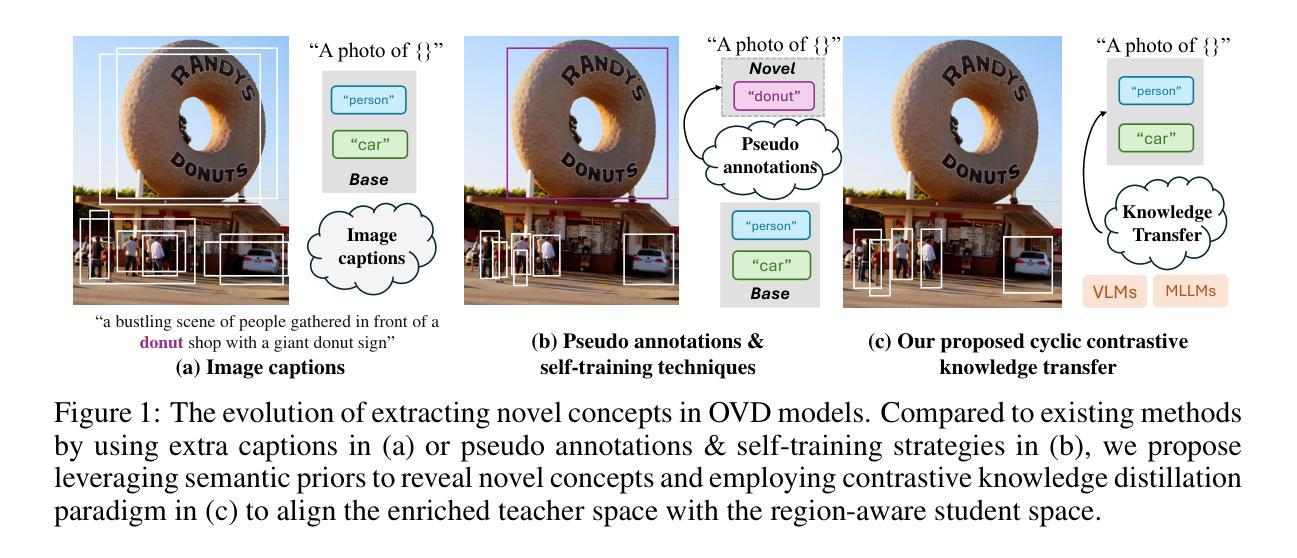
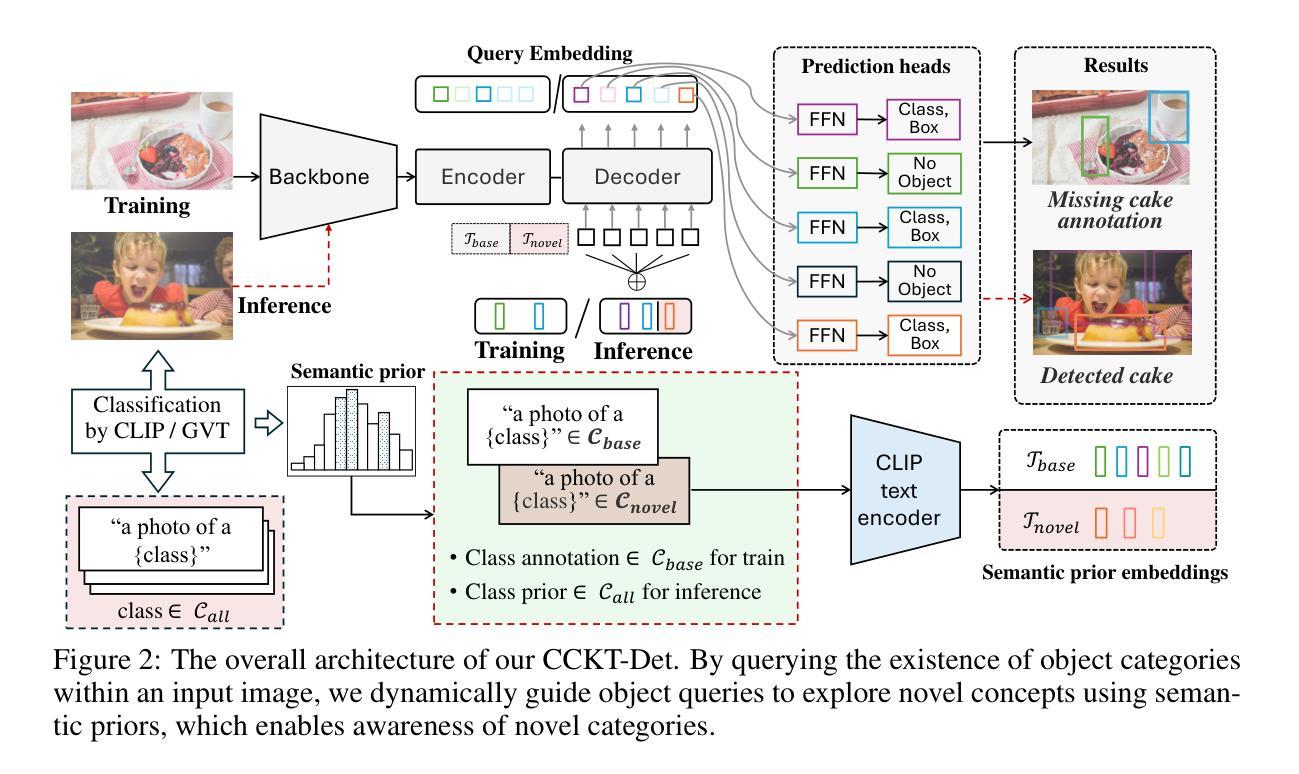
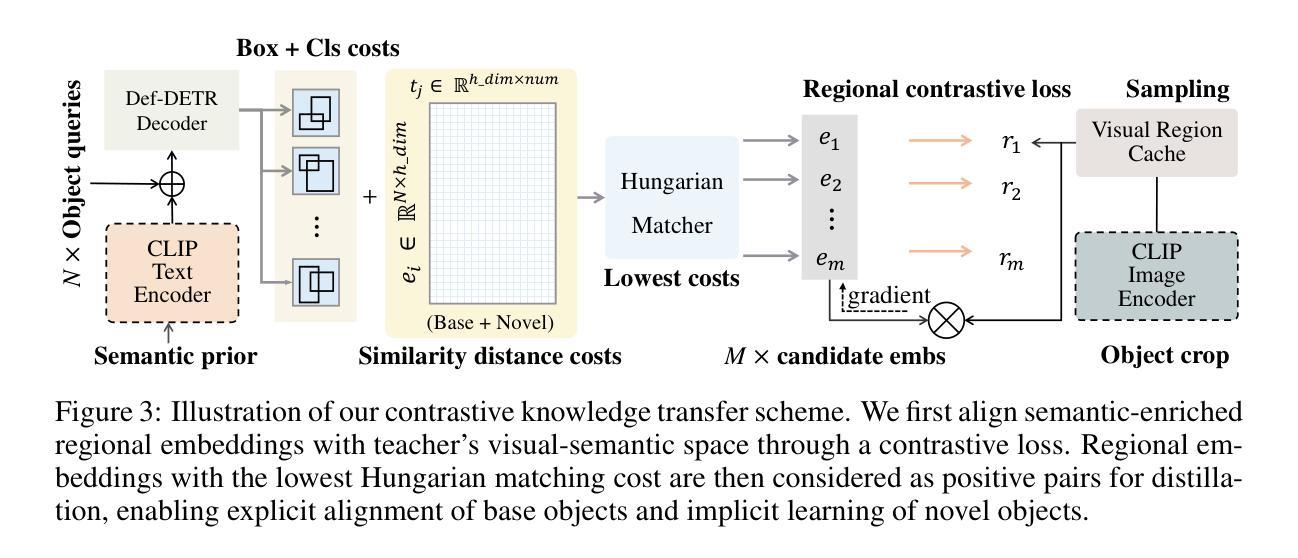
Bayesian Learning-driven Prototypical Contrastive Loss for Class-Incremental Learning
Authors:Nisha L. Raichur, Lucas Heublein, Tobias Feigl, Alexander Rügamer, Christopher Mutschler, Felix Ott
The primary objective of methods in continual learning is to learn tasks in a sequential manner over time (sometimes from a stream of data), while mitigating the detrimental phenomenon of catastrophic forgetting. This paper proposes a method to learn an effective representation between previous and newly encountered class prototypes. We propose a prototypical network with a Bayesian learning-driven contrastive loss (BLCL), tailored specifically for class-incremental learning scenarios. We introduce a contrastive loss that incorporates novel classes into the latent representation by reducing intra-class and increasing inter-class distance. Our approach dynamically adapts the balance between the cross-entropy and contrastive loss functions with a Bayesian learning technique. Experimental results conducted on the CIFAR-10, CIFAR-100, and ImageNet100 datasets for image classification and images of a GNSS-based dataset for interference classification validate the efficacy of our method, showcasing its superiority over existing state-of-the-art approaches. Git: https://gitlab.cc-asp.fraunhofer.de/darcy_gnss/gnss_class_incremental_learning
持续学习方法的主要目标是以顺序方式随时间学习任务(有时从数据流中),同时减轻灾难性遗忘的不利现象。本文提出了一种在先前遇到和新遇到的类别原型之间学习有效表示的方法。我们提出了一个以贝叶斯学习驱动的对比损失(BLCL)为特色的原型网络,专门用于类别增量学习场景。我们引入了一种对比损失,通过减少类内距离并增加类间距离,将新类别纳入潜在表示。我们的方法使用贝叶斯学习技术动态调整交叉熵和对比损失函数之间的平衡。在CIFAR-10、CIFAR-100和ImageNet100数据集上进行图像分类的实验,以及在基于GNSS的数据集上进行干扰分类的实验,验证了我们的方法的有效性,展示了其优于现有最先进的方法。Git链接:https://gitlab.cc-asp.fraunhofer.de/darcy_gnss/gnss_class_incremental_learning
论文及项目相关链接
PDF 27 pages, 22 figures
Summary
本文提出了一个针对类增量学习场景的原型网络与贝叶斯学习驱动的对比损失(BLCL)方法。该方法通过减少类内距离和增加类间距离来构建有效的类表示,并动态平衡交叉熵和对比损失函数。实验结果表明,该方法在图像分类和干扰分类数据集上均优于现有方法。
Key Takeaways
- 论文主要目标是解决持续学习中的灾难性遗忘问题,学习一系列任务时保留之前学习的知识。
- 提出了一种原型网络与贝叶斯学习驱动的对比损失方法(BLCL),专为类增量学习设计。
- 对比损失旨在通过减少类内距离和增加类间距离来构建有效的类表示。
- 使用贝叶斯学习技术动态平衡交叉熵和对比损失函数之间的关系。
- 在CIFAR-10、CIFAR-100、ImageNet100数据集上的图像分类实验验证了该方法的有效性。
- 还展示了该方法在基于GNSS的数据集上的干扰分类任务上的优势。
点此查看论文截图
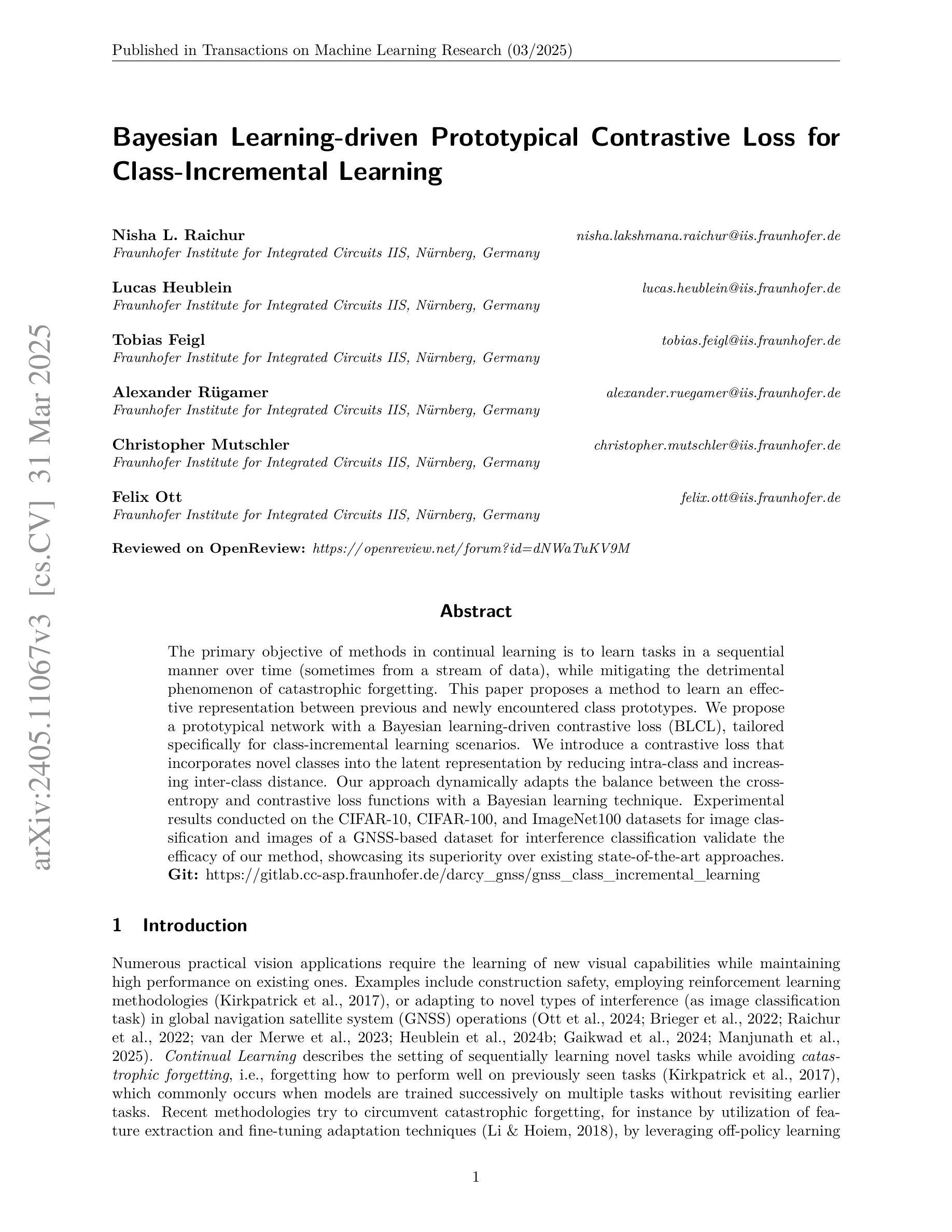
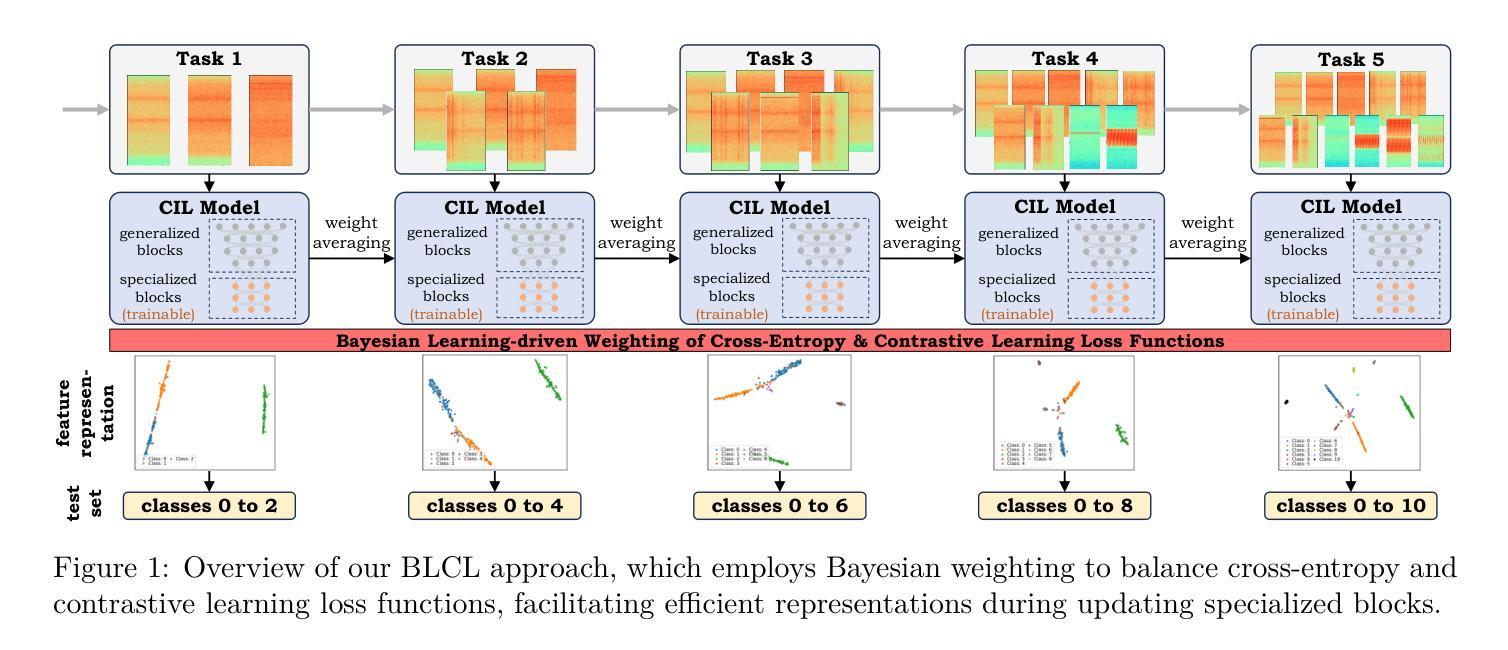
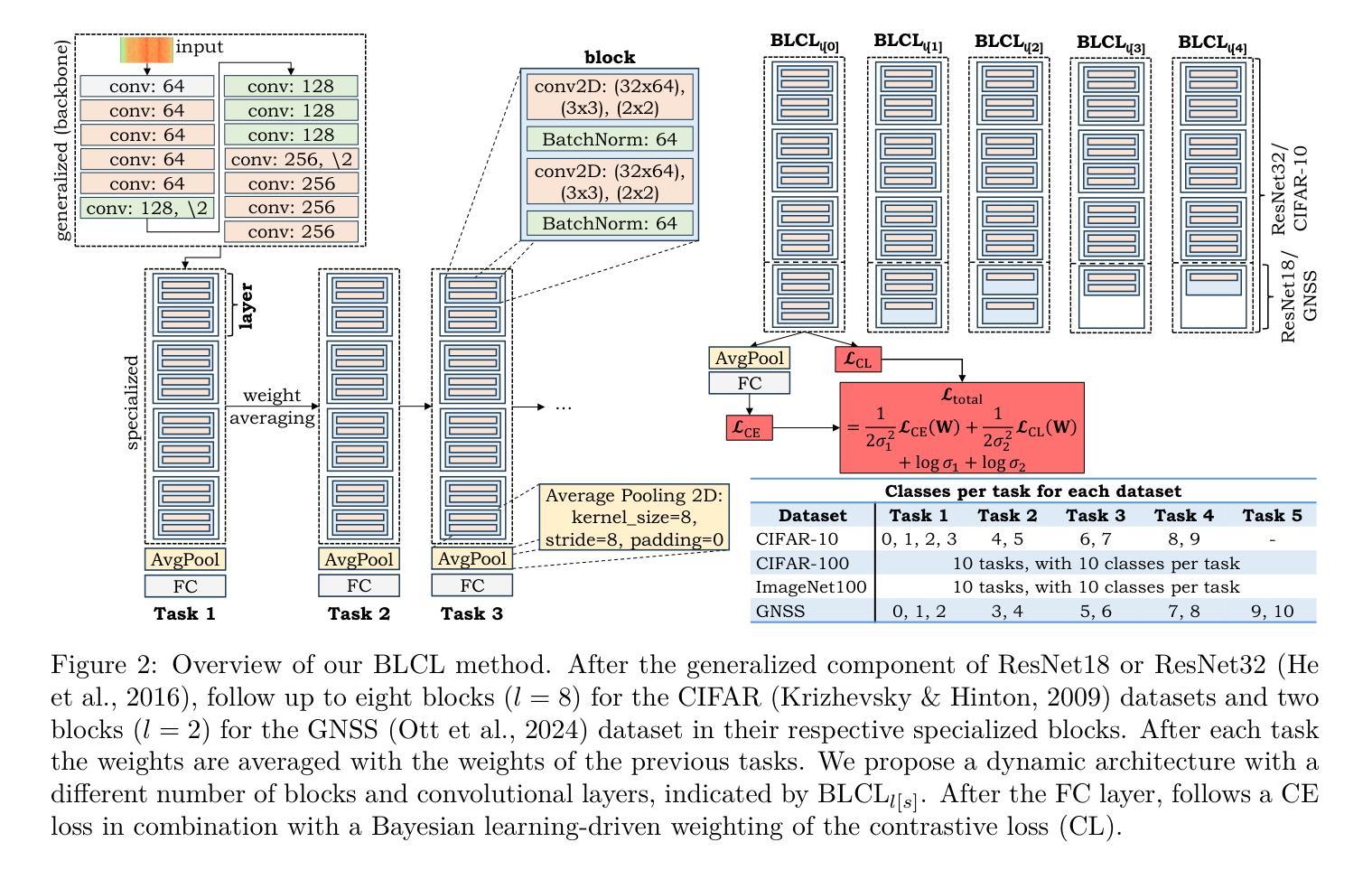
Modeling Caption Diversity in Contrastive Vision-Language Pretraining
Authors:Samuel Lavoie, Polina Kirichenko, Mark Ibrahim, Mahmoud Assran, Andrew Gordon Wilson, Aaron Courville, Nicolas Ballas
There are a thousand ways to caption an image. Contrastive Language Pretraining (CLIP) on the other hand, works by mapping an image and its caption to a single vector – limiting how well CLIP-like models can represent the diverse ways to describe an image. In this work, we introduce Llip, Latent Language Image Pretraining, which models the diversity of captions that could match an image. Llip’s vision encoder outputs a set of visual features that are mixed into a final representation by conditioning on information derived from the text. We show that Llip outperforms non-contextualized baselines like CLIP and SigLIP on a variety of tasks even with large-scale encoders. Llip improves zero-shot classification by an average of 2.9% zero-shot classification benchmarks with a ViT-G/14 encoder. Specifically, Llip attains a zero-shot top-1 accuracy of 83.5% on ImageNet outperforming a similarly sized CLIP by 1.4%. We also demonstrate improvement on zero-shot retrieval on MS-COCO by 6.0%. We provide a comprehensive analysis of the components introduced by the method and demonstrate that Llip leads to richer visual representations.
对于图像的描述存在千差万别的方式。另一方面,对比语言预训练(CLIP)通过将图像及其描述映射到单个向量上,限制了CLIP类似模型描述图像多样性的方式。在这项工作中,我们引入了潜文本图像预训练(Llip),该模型可以模拟与图像匹配的描述的多样性。Llip的视觉编码器输出一系列视觉特征,这些特征通过基于文本派生信息的条件作用混合成最终的表示。我们表明,即使在大型编码器的情况下,Llip在各种任务上的表现也超过了CLIP和SigLIP等非上下文基准线。在使用ViT-G/1B编码器进行的零样本分类基准测试中,Llip将零样本分类的平均准确率提高了2.9%。具体来说,Llip在ImageNet上的零样本top-1准确率达到了83.5%,超过了类似大小的CLIP模型1.4%。我们还证明了在MS-COCO上的零样本检索提高了6.0%。我们对该方法引入的组件进行了综合分析,并证明Llip导致了更丰富的视觉表示。
论文及项目相关链接
PDF 14 pages, 8 figures, 7 tables, to be published at ICML2024
Summary
本文介绍了Llip(Latent Language Image Pretraining)模型,它解决了Contrastive Language Pretraining(CLIP)在图像描述方面存在的单一性缺陷。Llip模型能够模拟与图像匹配的多种描述方式,通过视觉编码器输出一系列视觉特征,并结合文本信息生成最终的表示。实验表明,Llip在各种任务上优于非上下文化的基线模型如CLIP和SigLIP,即使在大型编码器上也能实现更好的性能。特别是在零样本分类和零样本检索任务上,Llip取得了显著的改进。
Key Takeaways
- Llip模型解决了CLIP在图像描述方面的单一性问题,能够模拟与图像匹配的多种描述方式。
- Llip使用视觉编码器输出一系列视觉特征,并结合文本信息生成最终的表示。
- Llip在各种任务上优于非上下文化的基线模型,如CLIP和SigLIP。
- Llip在零样本分类任务上实现了平均2.9%的改进,特别是在ImageNet数据集上,使用ViT-G/14编码器达到了83.5%的零样本top-1准确率,超越了类似大小的CLIP模型。
- Llip在MS-COCO数据集上的零样本检索任务上也取得了6.0%的改进。
- 文中对Llip模型引入的组件进行了综合分析,证明了其有效性。
点此查看论文截图
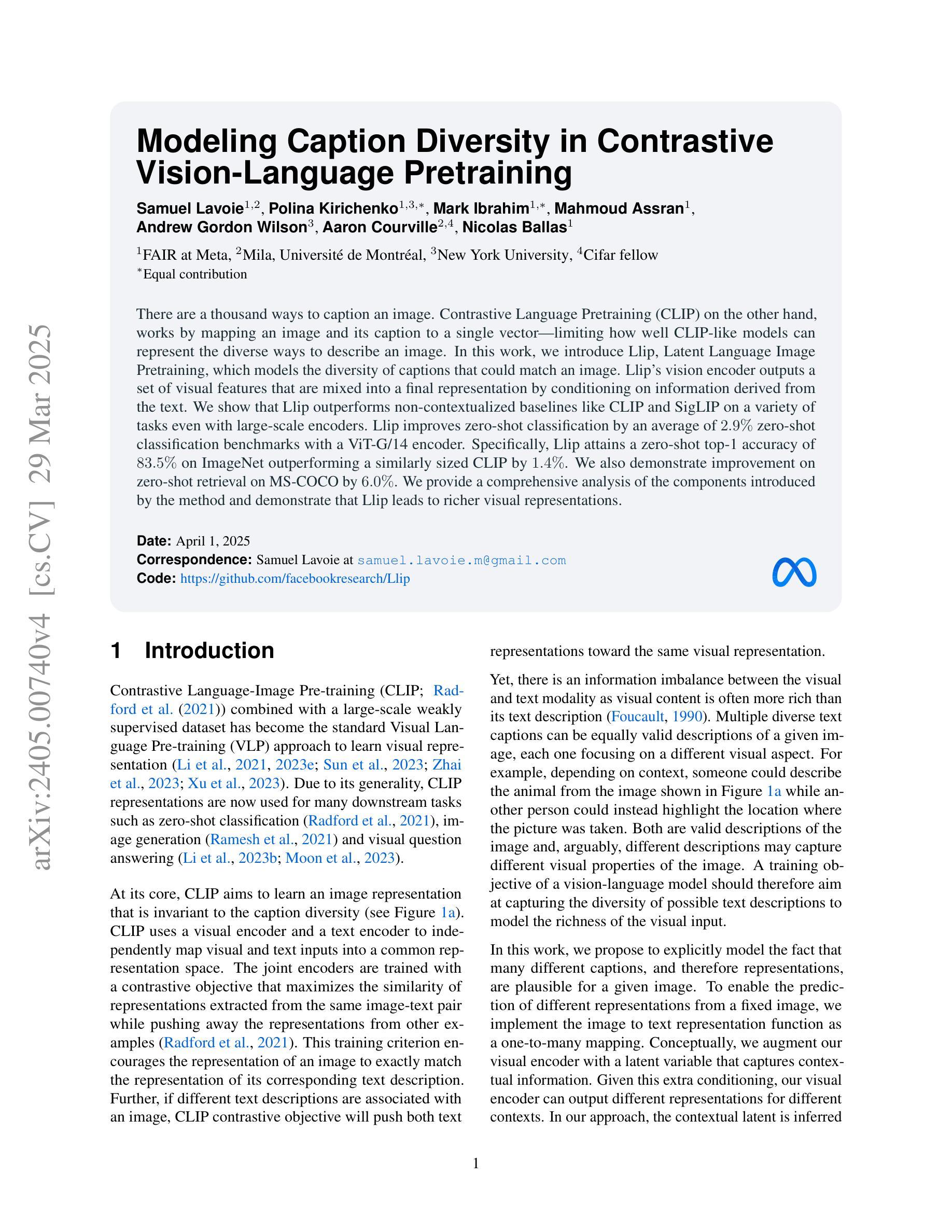
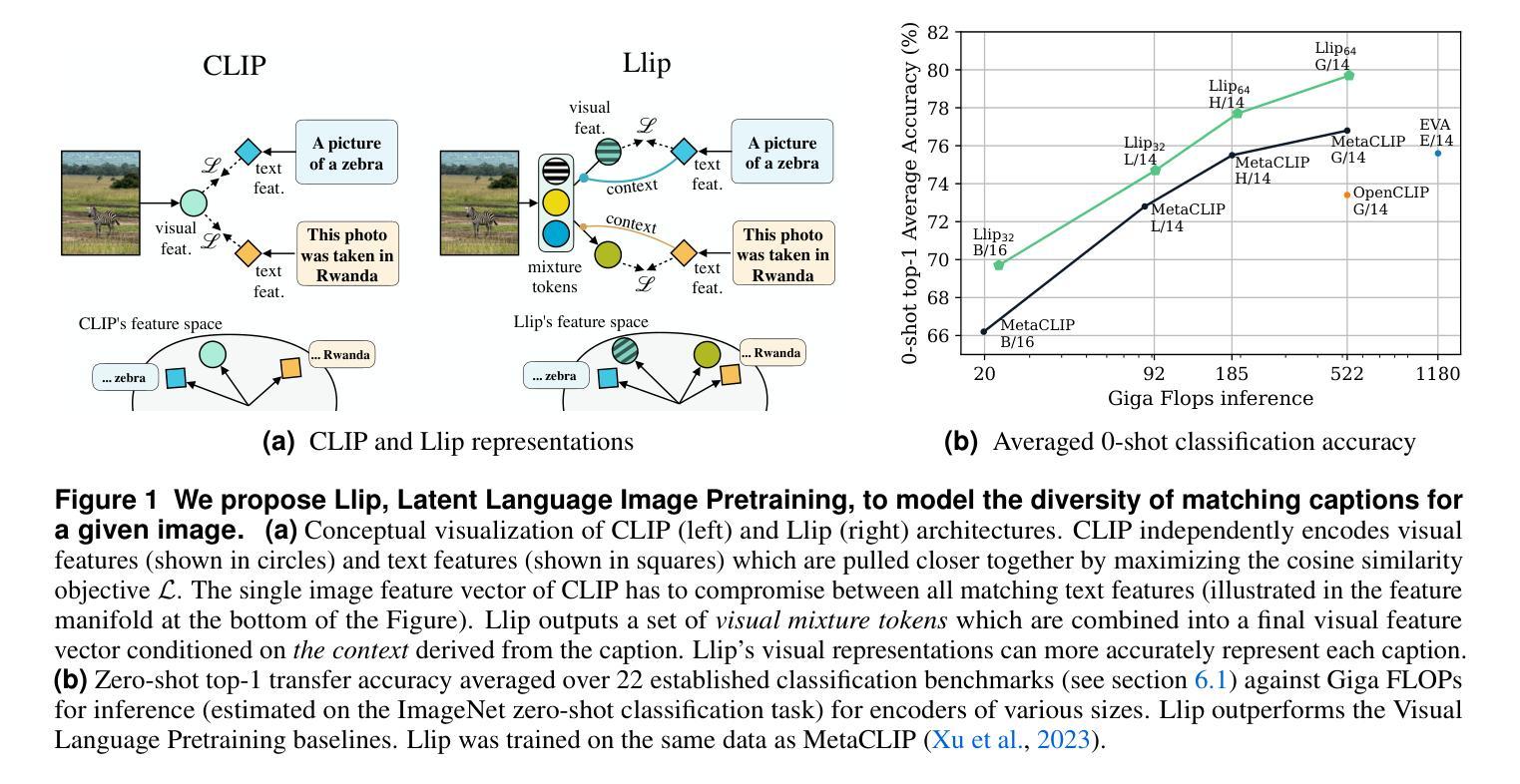
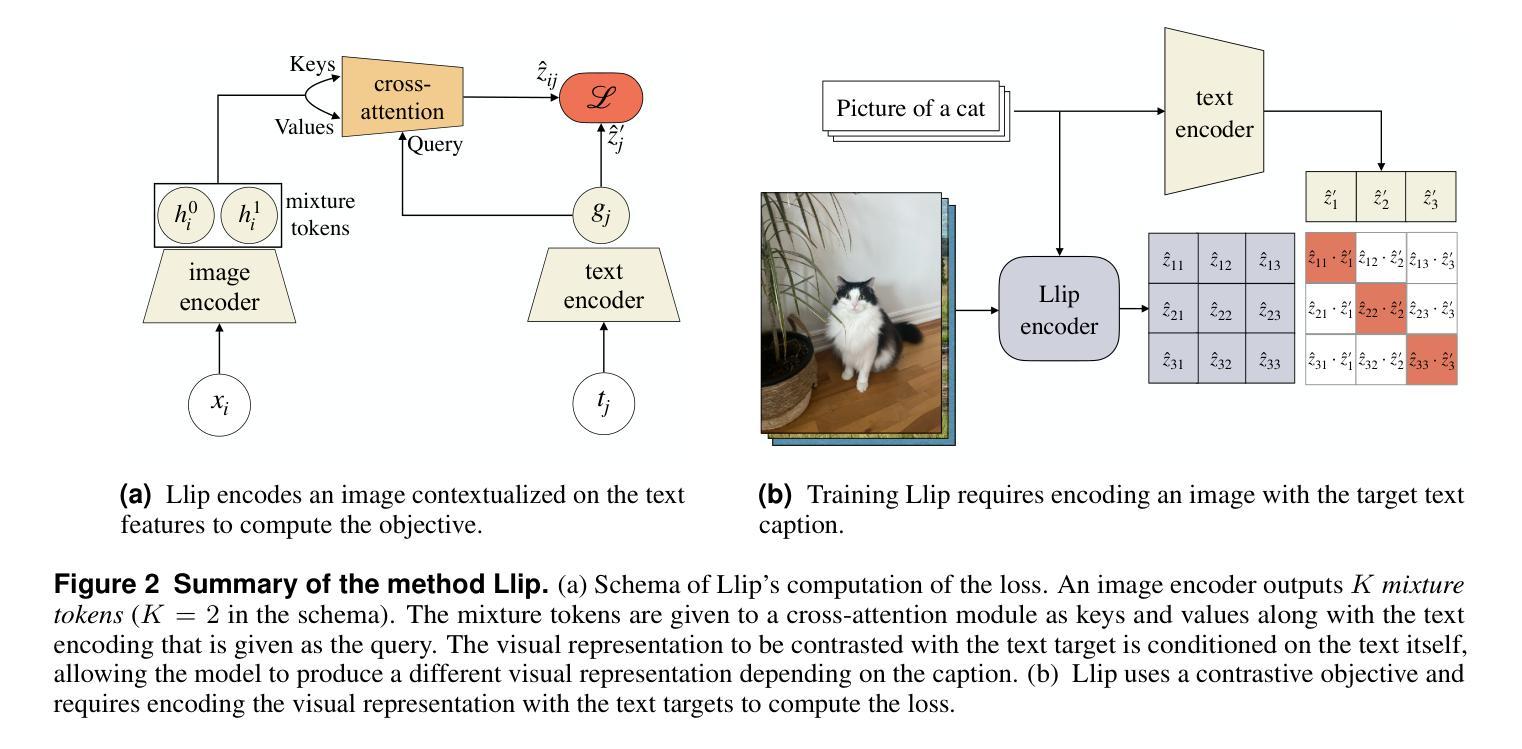
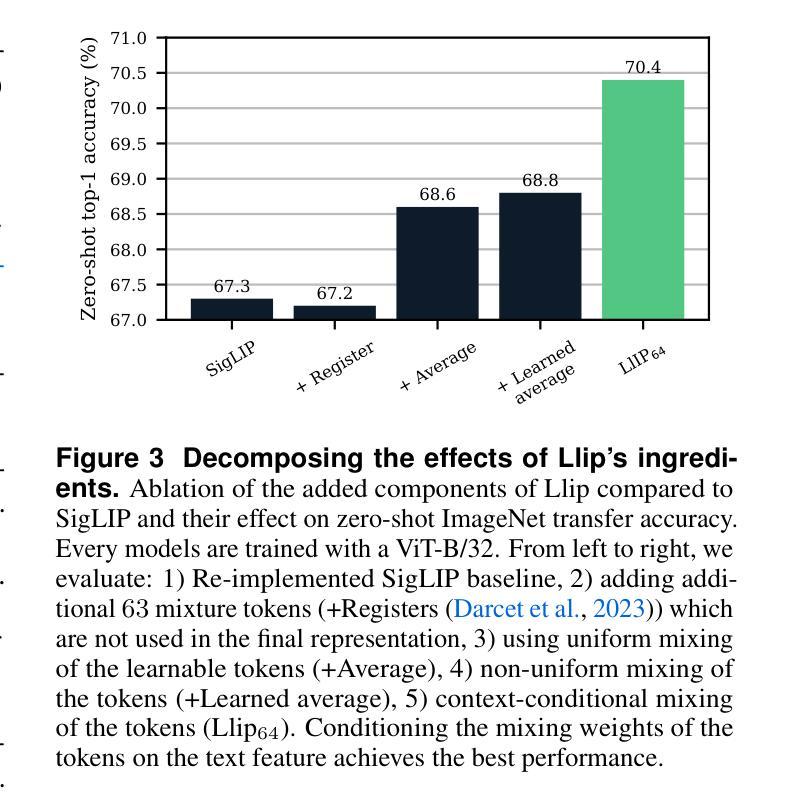
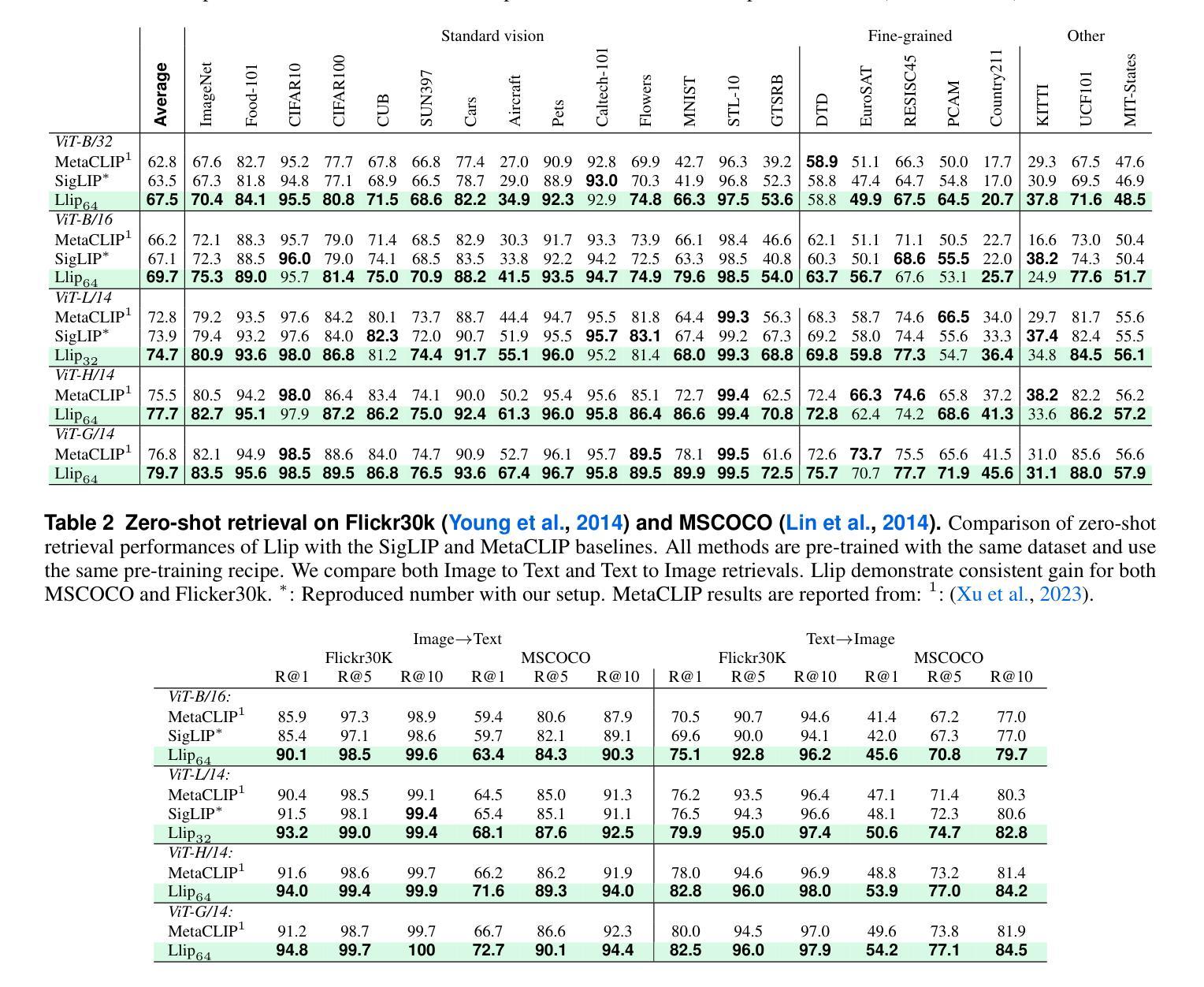