⚠️ 以下所有内容总结都来自于 大语言模型的能力,如有错误,仅供参考,谨慎使用
🔴 请注意:千万不要用于严肃的学术场景,只能用于论文阅读前的初筛!
💗 如果您觉得我们的项目对您有帮助 ChatPaperFree ,还请您给我们一些鼓励!⭐️ HuggingFace免费体验
2025-04-03 更新
Style transfer between Microscopy and Magnetic Resonance Imaging via Generative Adversarial Network in small sample size settings
Authors:Monika Pytlarz, Adrian Onicas, Alessandro Crimi
Cross-modal augmentation of Magnetic Resonance Imaging (MRI) and microscopic imaging based on the same tissue samples is promising because it can allow histopathological analysis in the absence of an underlying invasive biopsy procedure. Here, we tested a method for generating microscopic histological images from MRI scans of the corpus callosum using conditional generative adversarial network (cGAN) architecture. To our knowledge, this is the first multimodal translation of the brain MRI to histological volumetric representation of the same sample. The technique was assessed by training paired image translation models taking sets of images from MRI scans and microscopy. The use of cGAN for this purpose is challenging because microscopy images are large in size and typically have low sample availability. The current work demonstrates that the framework reliably synthesizes histology images from MRI scans of corpus callosum, emphasizing the network’s ability to train on high resolution histologies paired with relatively lower-resolution MRI scans. With the ultimate goal of avoiding biopsies, the proposed tool can be used for educational purposes.
将核磁共振成像(MRI)与基于同一组织样本的显微镜成像进行跨模态增强是有前景的,因为它可以在没有基础侵入性活检程序的情况下进行组织病理学分析。在这里,我们测试了一种利用条件生成对抗网络(cGAN)架构,从胼胝体MRI扫描生成显微镜组织学图像的方法。据我们所知,这是将大脑MRI转化为同一样本组织学体积表示的多模式翻译首次尝试。该技术通过训练配对图像翻译模型来评估,该模型从MRI扫描和显微镜成像中获取图像集。使用cGAN进行此操作具有挑战性,因为显微镜图像尺寸较大且样本通常稀缺。目前的工作证明该框架能够可靠地从胼胝体的MRI扫描中合成组织学图像,突出了网络在相对较低的分辨率MRI扫描与配对的高分辨率组织学上进行训练的能力。以最终避免活检为目标,所提出工具可用于教学目的。
论文及项目相关链接
PDF 2023 IEEE International Conference on Image Processing (ICIP)
Summary
本文探索了基于同一组织样本的核磁共振成像(MRI)与显微镜成像的跨模态增强技术。该技术能够在无需侵入性活检程序的情况下进行组织病理学分析。研究中,我们测试了一种利用条件生成对抗网络(cGAN)架构,从MRI扫描生成微观组织学图像的方法。据我们所知,这是首次将大脑MRI多模式转换为同一样本的组织学体积表示。该技术通过训练配对图像翻译模型来评估,这些模型采用来自MRI扫描和显微镜的图像集。使用cGAN进行此操作具有挑战性,因为显微镜图像尺寸较大且样本通常较少。当前工作证明,该框架能够可靠地从大脑神经纤维束(corpus callosum)的MRI扫描中合成组织学图像,突显网络在高分辨率组织学与相对较低分辨率MRI扫描配对训练的能力。以最终避免活检为目标,该工具可用于教学目的。
Key Takeaways
- 跨模态增强技术结合了MRI和显微镜成像,使组织病理学分析在无需活检的情况下成为可能。
- 利用条件生成对抗网络(cGAN)首次实现了从MRI扫描到组织学体积表示的转变。
- cGAN在此应用上具挑战性,因显微镜图像尺寸大且样本稀缺。
- 研究通过训练配对图像翻译模型评估该技术,这些模型采用MRI和显微镜图像集。
- 框架已成功合成神经纤维束(corpus callosum)的MRI扫描历史学图像。
- 网络在高分辨率组织学与较低分辨率MRI扫描配对训练时表现出能力。
点此查看论文截图
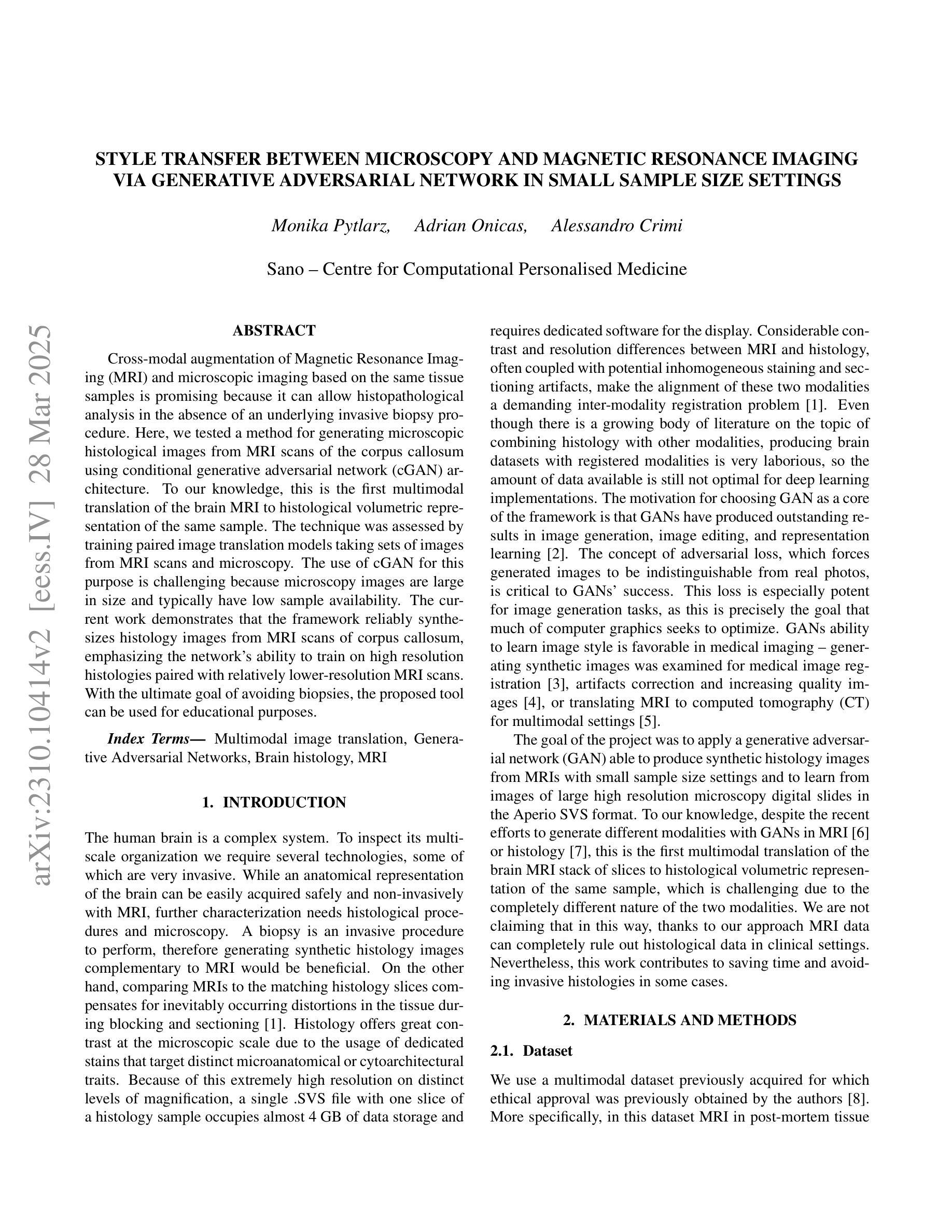
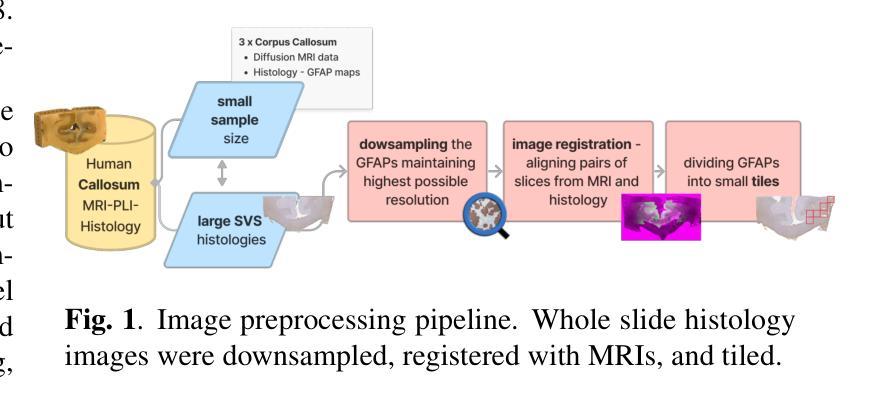
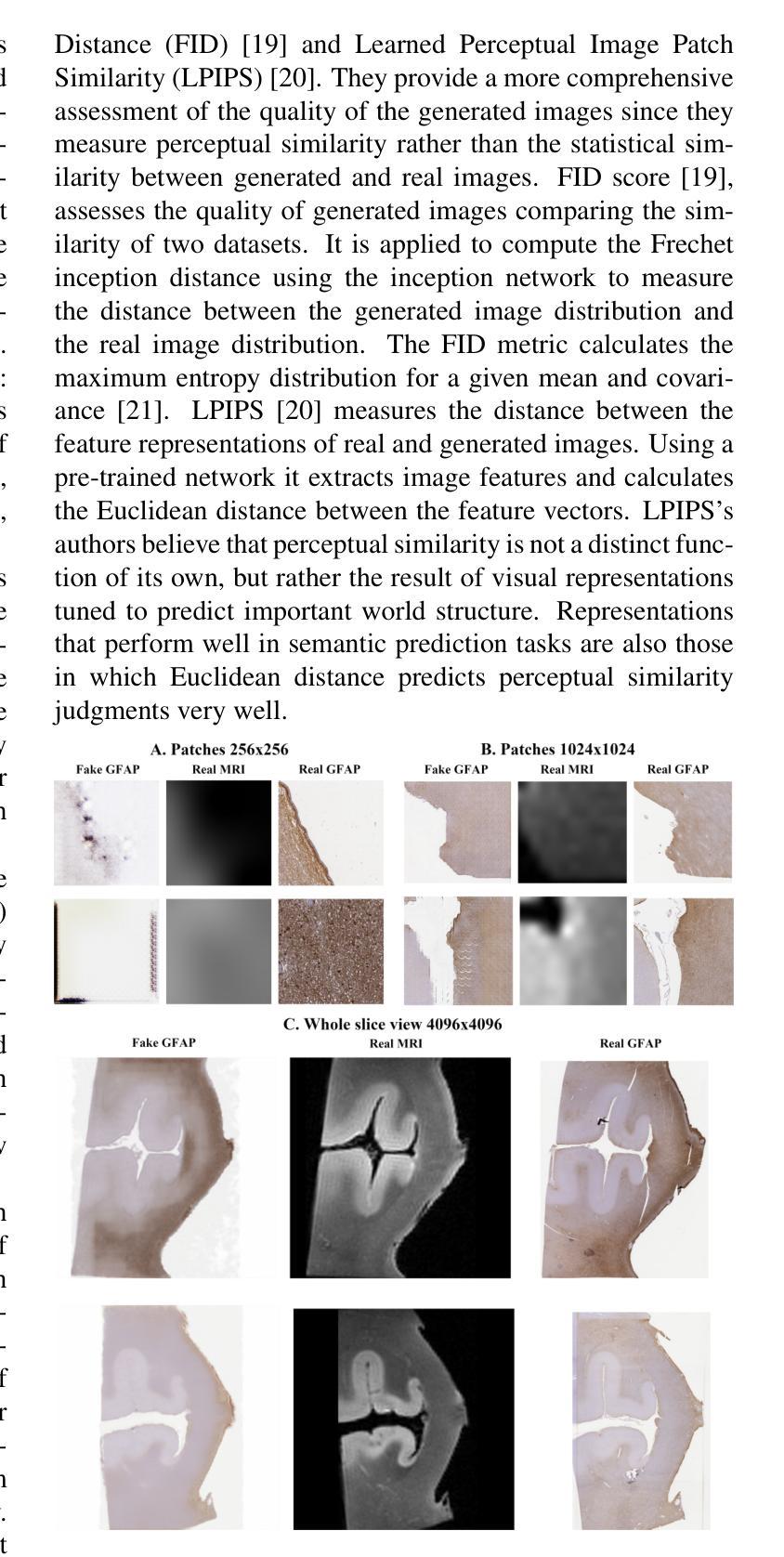
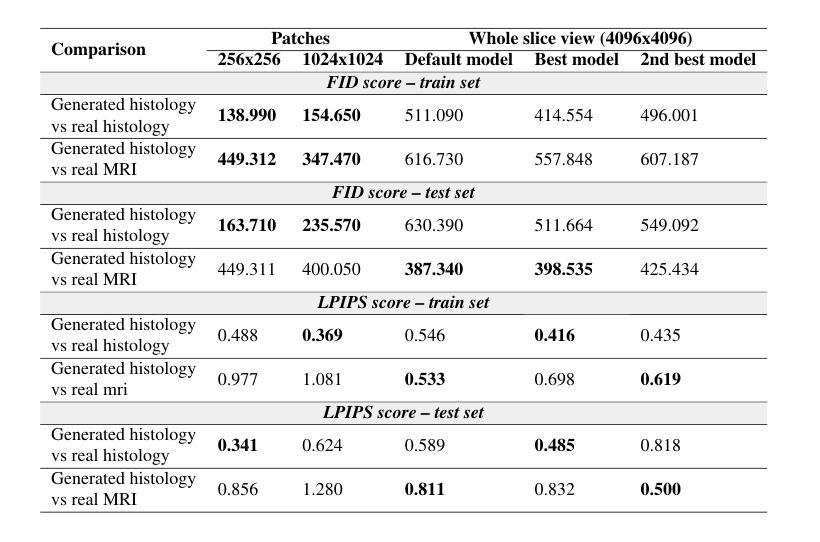