⚠️ 以下所有内容总结都来自于 大语言模型的能力,如有错误,仅供参考,谨慎使用
🔴 请注意:千万不要用于严肃的学术场景,只能用于论文阅读前的初筛!
💗 如果您觉得我们的项目对您有帮助 ChatPaperFree ,还请您给我们一些鼓励!⭐️ HuggingFace免费体验
2025-04-04 更新
Monocular and Generalizable Gaussian Talking Head Animation
Authors:Shengjie Gong, Haojie Li, Jiapeng Tang, Dongming Hu, Shuangping Huang, Hao Chen, Tianshui Chen, Zhuoman Liu
In this work, we introduce Monocular and Generalizable Gaussian Talking Head Animation (MGGTalk), which requires monocular datasets and generalizes to unseen identities without personalized re-training. Compared with previous 3D Gaussian Splatting (3DGS) methods that requires elusive multi-view datasets or tedious personalized learning/inference, MGGtalk enables more practical and broader applications. However, in the absence of multi-view and personalized training data, the incompleteness of geometric and appearance information poses a significant challenge. To address these challenges, MGGTalk explores depth information to enhance geometric and facial symmetry characteristics to supplement both geometric and appearance features. Initially, based on the pixel-wise geometric information obtained from depth estimation, we incorporate symmetry operations and point cloud filtering techniques to ensure a complete and precise position parameter for 3DGS. Subsequently, we adopt a two-stage strategy with symmetric priors for predicting the remaining 3DGS parameters. We begin by predicting Gaussian parameters for the visible facial regions of the source image. These parameters are subsequently utilized to improve the prediction of Gaussian parameters for the non-visible regions. Extensive experiments demonstrate that MGGTalk surpasses previous state-of-the-art methods, achieving superior performance across various metrics.
在这项工作中,我们引入了单目和通用高斯语音头动画(MGGTalk),它只需要单目数据集,即可推广到未见过的身份而无需个性化重新训练。与之前需要难以获取的多视角数据集或繁琐个性化学习/推断的3D高斯拼贴(3DGS)方法相比,MGGTalk使实际应用和更广泛的应用成为可能。然而,在没有多视角和个性化训练数据的情况下,几何和外观信息的完整性构成了重大挑战。为了解决这些挑战,MGGTalk探索深度信息来增强几何和面部对称特征,以补充几何和外观特征。最初,基于从深度估计获得的像素级几何信息,我们结合了对称操作和点云滤波技术,以确保3DGS的完整和精确的位置参数。随后,我们采用具有对称先验的两阶段策略来预测剩余的3DGS参数。我们首先预测源图像可见面部区域的高斯参数。这些参数随后用于改进非可见区域的高斯参数预测。大量实验表明,MGGTalk超越了之前的最先进方法,在各项指标上均实现了卓越的性能。
论文及项目相关链接
PDF Accepted by CVPR 2025
Summary
本文介绍了单目通用高斯头部动画技术(MGGTalk),该技术仅需单目数据集,并能够推广到未见过的身份而无需个性化再训练。与需要难以获取的多视角数据集或繁琐个性化学习/推断的先前3D高斯贴片(3DGS)方法相比,MGGTalk具有更实用和更广泛的应用潜力。在缺乏多视角和个性化训练数据的情况下,MGGTalk通过探索深度信息来增强几何和面部对称性特征,以补充几何和外观特征。实验表明,MGGTalk在多个指标上超越了先前的方法,实现了卓越的性能。
Key Takeaways
- MGGTalk使用单目数据集,无需多视角数据,简化了头部动画的创建过程。
- MGGTalk能够在未见过的身份上推广,无需个性化再训练,增加了其应用的广泛性。
- 在缺乏多视角和个性化训练数据的情况下,MGGTalk通过利用深度信息增强几何和面部对称性特征。
- MGGTalk采用基于像素的几何信息来进行深度估算,并融入对称操作和点云过滤技术,确保3DGS的完整性和精确位置参数。
- MGGTalk采用两阶段策略,结合对称先验来预测剩余的3DGS参数。
- 该技术首先预测源图像可见面部区域的高斯参数,然后用于改进非可见区域的高斯参数预测。
点此查看论文截图
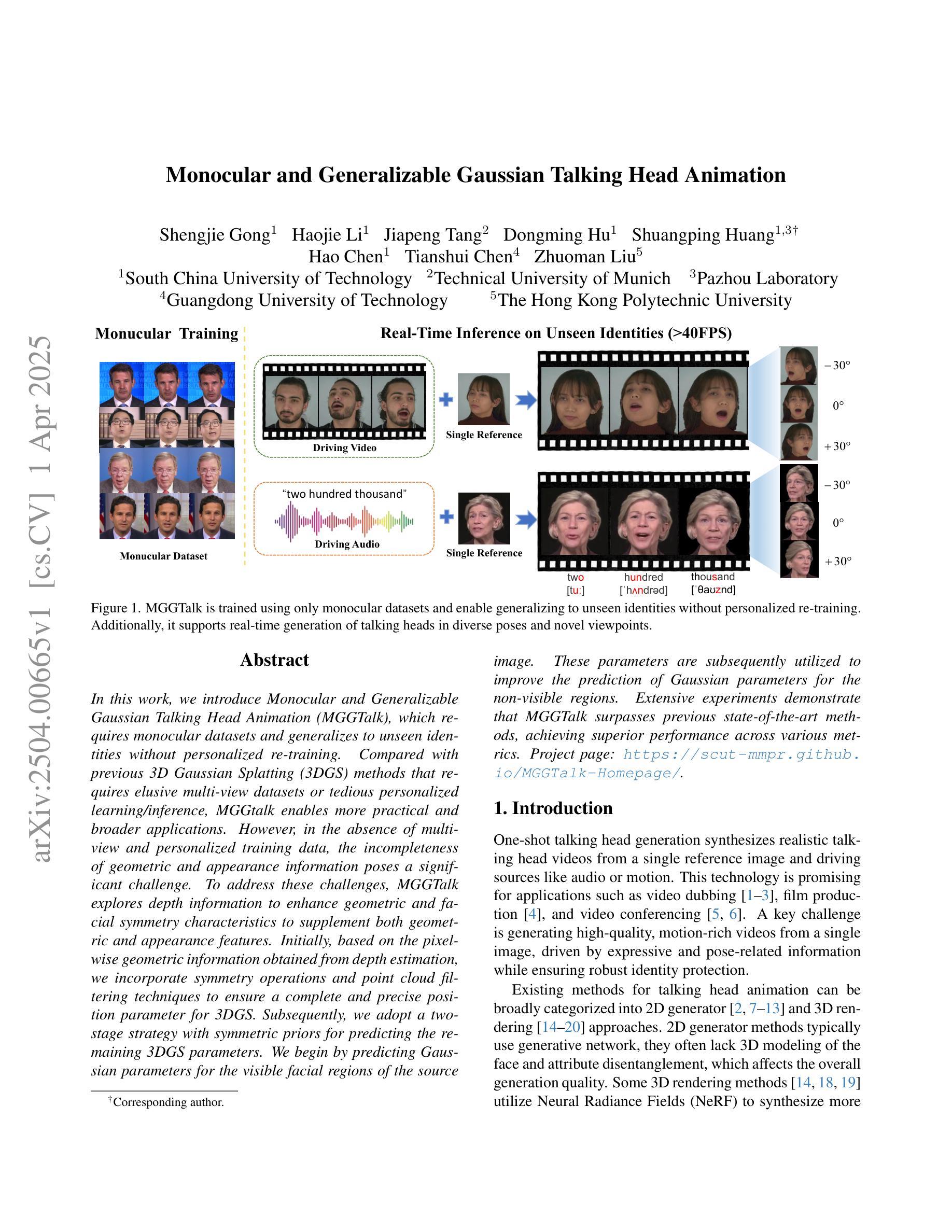
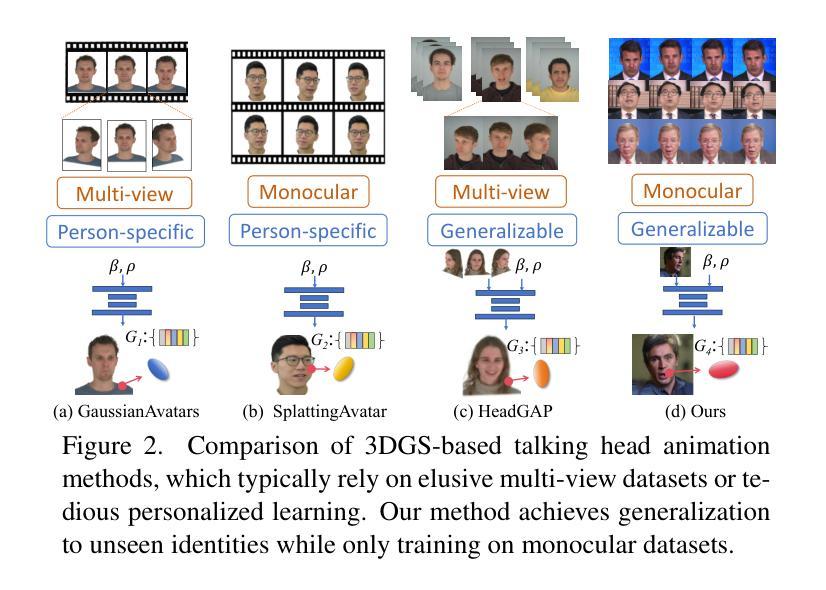
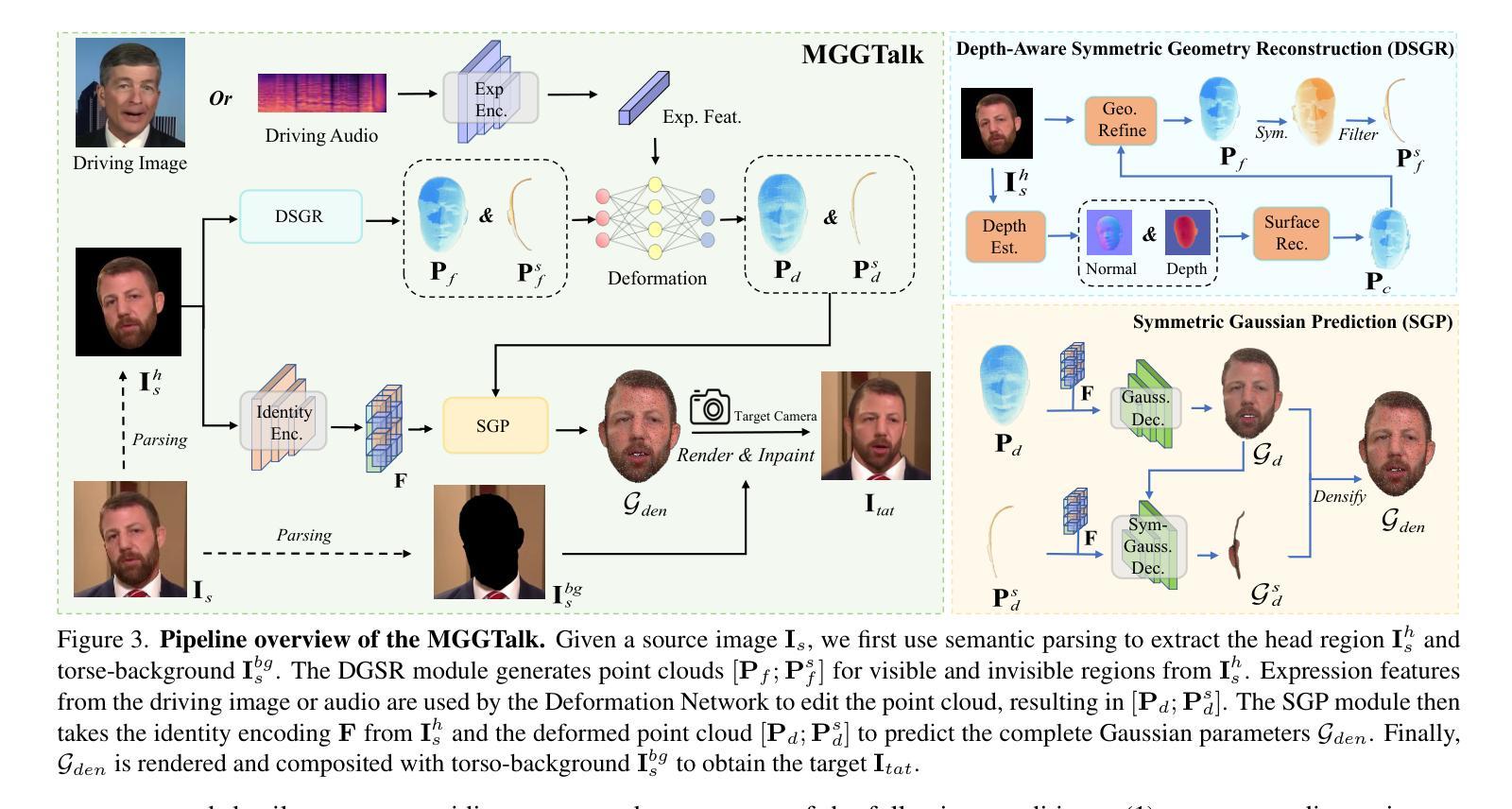
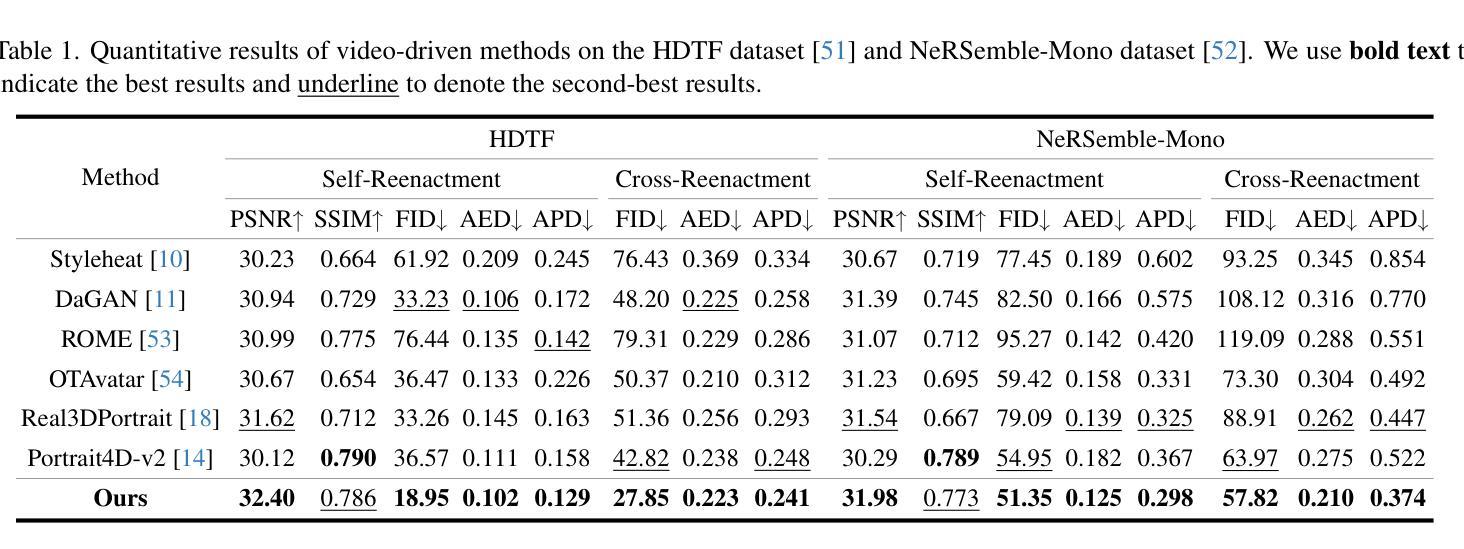
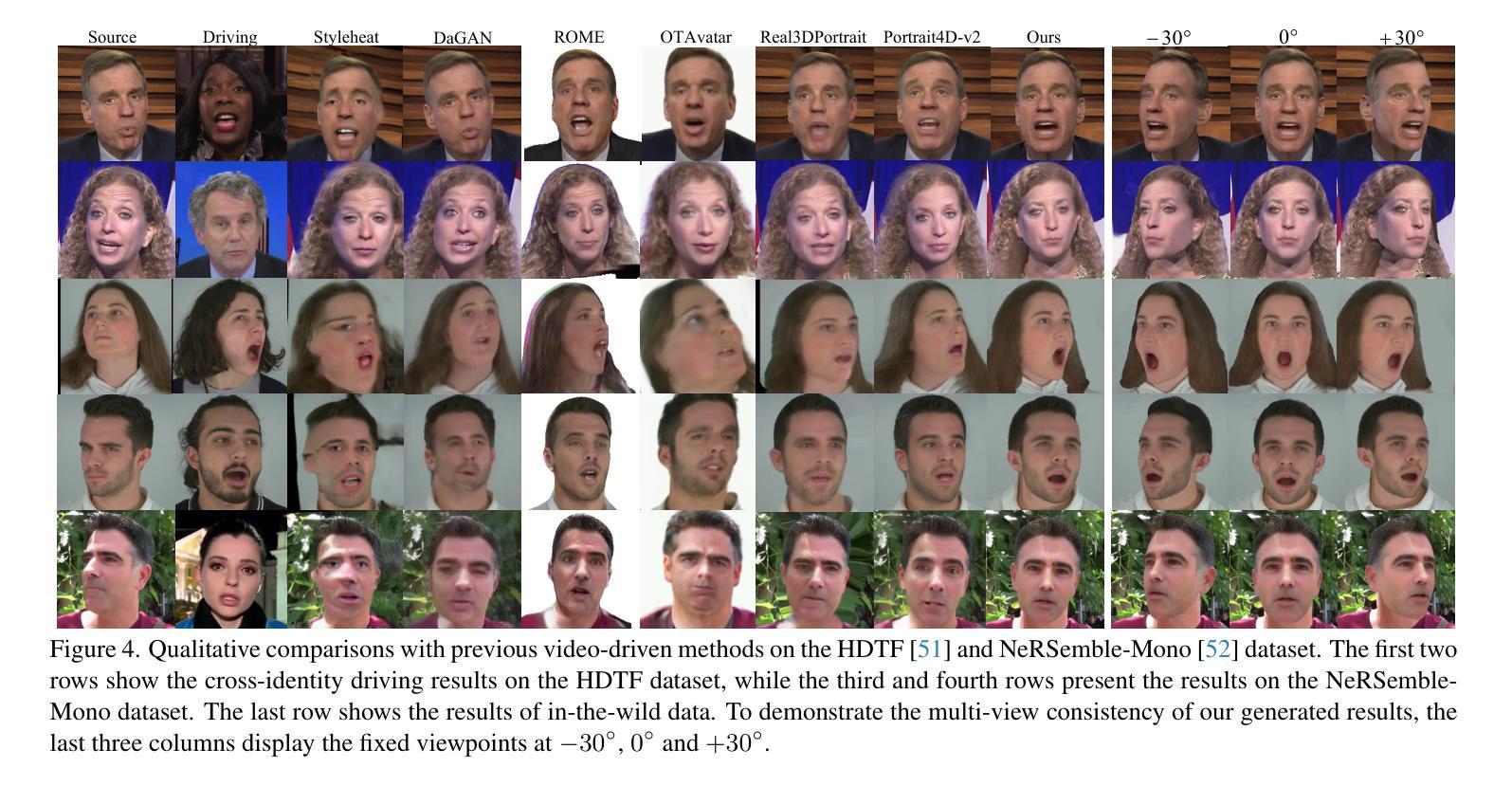
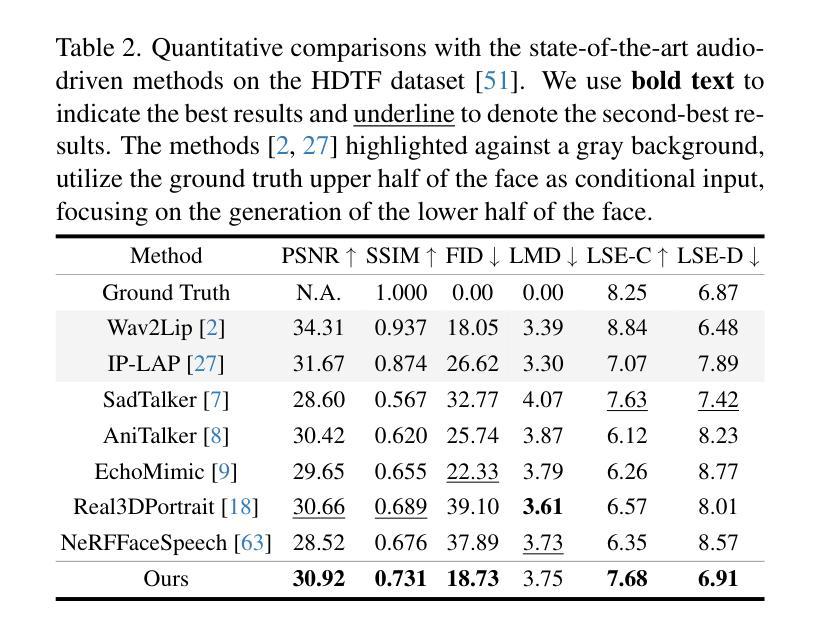
Exchange cross-talk mitigation in dense quantum dot arrays
Authors:Daniel Jirovec, Pablo Cova Fariña, Stefano Reale, Stefan D. Oosterhout, Xin Zhang, Elizaveta Morozova, Sander de Snoo, Amir Sammak, Giordano Scappucci, Menno Veldhorst, Lieven M. K. Vandersypen
Coupled spins in semiconductor quantum dots are a versatile platform for quantum computing and simulations of complex many-body phenomena. However, on the path of scale-up, cross-talk from densely packed electrodes poses a severe challenge. While cross-talk onto the dot potentials is nowadays routinely compensated for, cross-talk on the exchange interaction is much more difficult to tackle because it is not always directly measurable. Here we propose and implement a way of characterizing and compensating cross-talk on adjacent exchange interactions by following the singlet-triplet avoided crossing in Ge. We show that we can easily identify the barrier-to-barrier cross-talk element without knowledge of the particular exchange value in a 2x4 quantum dot array. We uncover striking differences among these cross-talk elements which can be linked to the geometry of the device and the barrier gate fan-out. We validate the methodology by tuning up four-spin Heisenberg chains. The same methodology should be applicable to longer chains of spins and to other semiconductor platforms in which mixing of the singlet and the lowest-energy triplet is present or can be engineered. Additionally, this procedure is well suited for automated tuning routines as we obtain a stand-out feature that can be easily tracked and directly returns the magnitude of the cross-talk.
半导体量子点中的耦合自旋是量子计算和模拟复杂多体现象的多功能平台。然而,在规模扩大的过程中,来自密集电极的串扰是一个严重的挑战。虽然现今已经可以对点势上的串扰进行常规补偿,但处理交换作用上的串扰更加困难,因为并非总是可以对其进行直接测量。在此,我们提出并实施了一种通过跟踪Ge中的单线-三线避免交叉来表征和补偿相邻交换作用上的串扰的方法。我们展示了在不知道特定交换值的情况下,可以在一个2x4量子点阵列中轻松确定屏障到屏障串扰元素。我们发现这些串扰元素之间存在引人注目的差异,这些差异与器件的几何形状和屏障门扇出有关。我们通过调整四个自旋的海森堡链验证了该方法。对于更长的自旋链和其他存在单线最低能量和三线混合或可设计的半导体平台,此方法同样适用。此外,由于我们获得了可以轻易追踪并直接返回串扰幅度的突出特征,因此该过程非常适合用于自动调整例行程序。
论文及项目相关链接
Summary
半导体量子点中的耦合自旋是量子计算和模拟复杂多体现象的多功能平台。然而,在规模扩大的过程中,来自密集电极的串话构成了严重的挑战。目前,人们通常能够补偿点对势的串话,但处理交换相互作用的串话更加困难,因为并非总是能够直接测量它。本文提出并实施了一种通过跟踪Ge中的单重态三重态避免交叉来表征和补偿相邻交换相互作用上的串话的方法。我们展示了能够在不了解特定交换值的情况下轻松识别屏障间串话元素的能力。我们发现这些串话元素之间存在引人注目的差异,这些差异与设备的几何形状和屏障门扇出有关。通过调整四个自旋的海森堡链验证了该方法的有效性。该方法应适用于较长的自旋链和其他存在或可设计单重态和最低能量三重态混合的半导体平台。此外,由于我们能够轻松追踪突出功能并直接返回串话幅度,因此该过程非常适合自动化调整例行程序。
Key Takeaways
- 半导体量子点中的耦合自旋为量子计算和模拟复杂多体现象提供了多功能平台。
- 在规模扩展过程中,来自密集电极的串话构成挑战,特别是交换相互作用的串话难以处理。
- 通过跟踪单重态三重态避免交叉提出表征和补偿相邻交换相互作用上的串话的方法。
- 在不了解特定交换值的情况下能识别屏障间串话元素。
- 串话元素差异显著,与设备几何形状和屏障门扇出有关。
- 通过调整四个自旋的海森堡链验证了方法的可行性。
点此查看论文截图
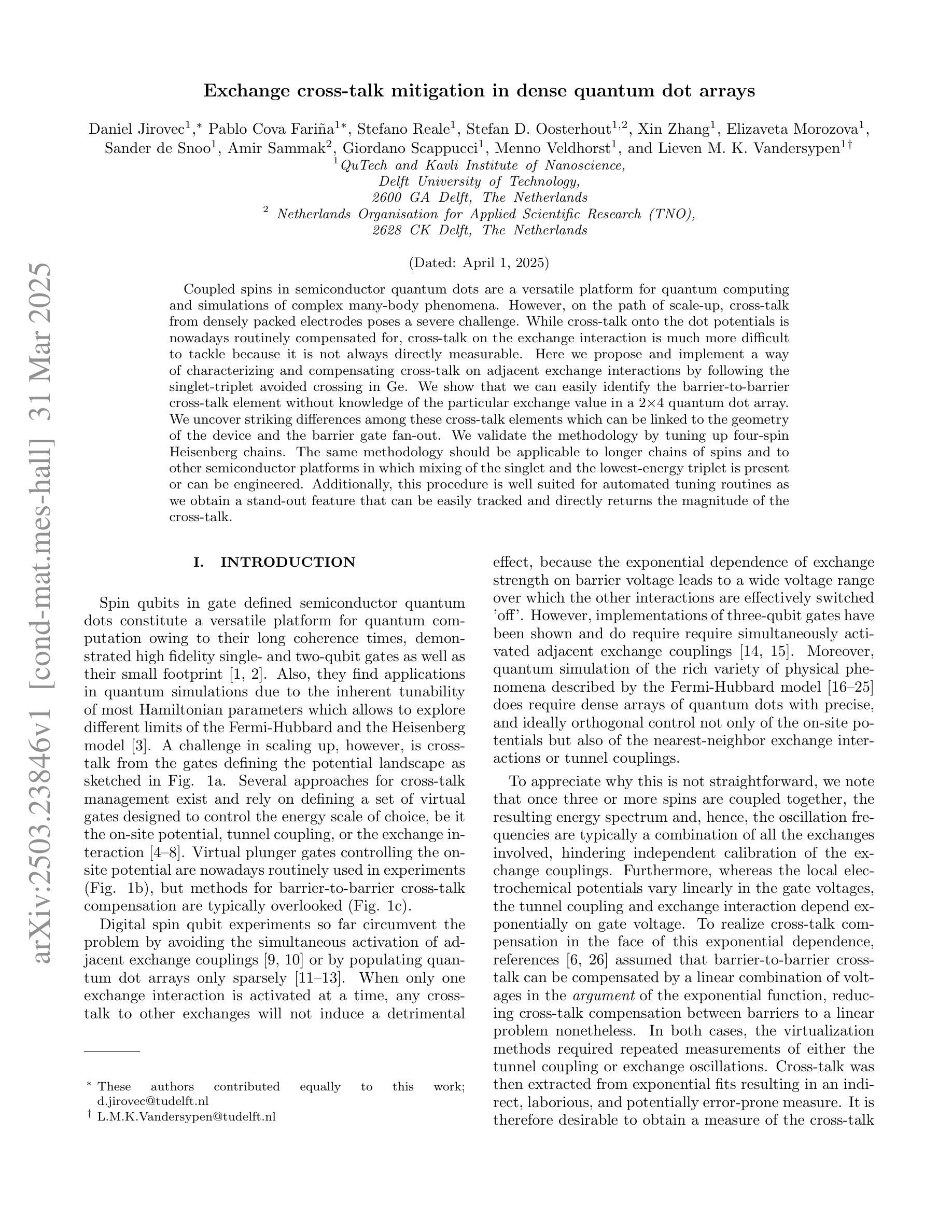
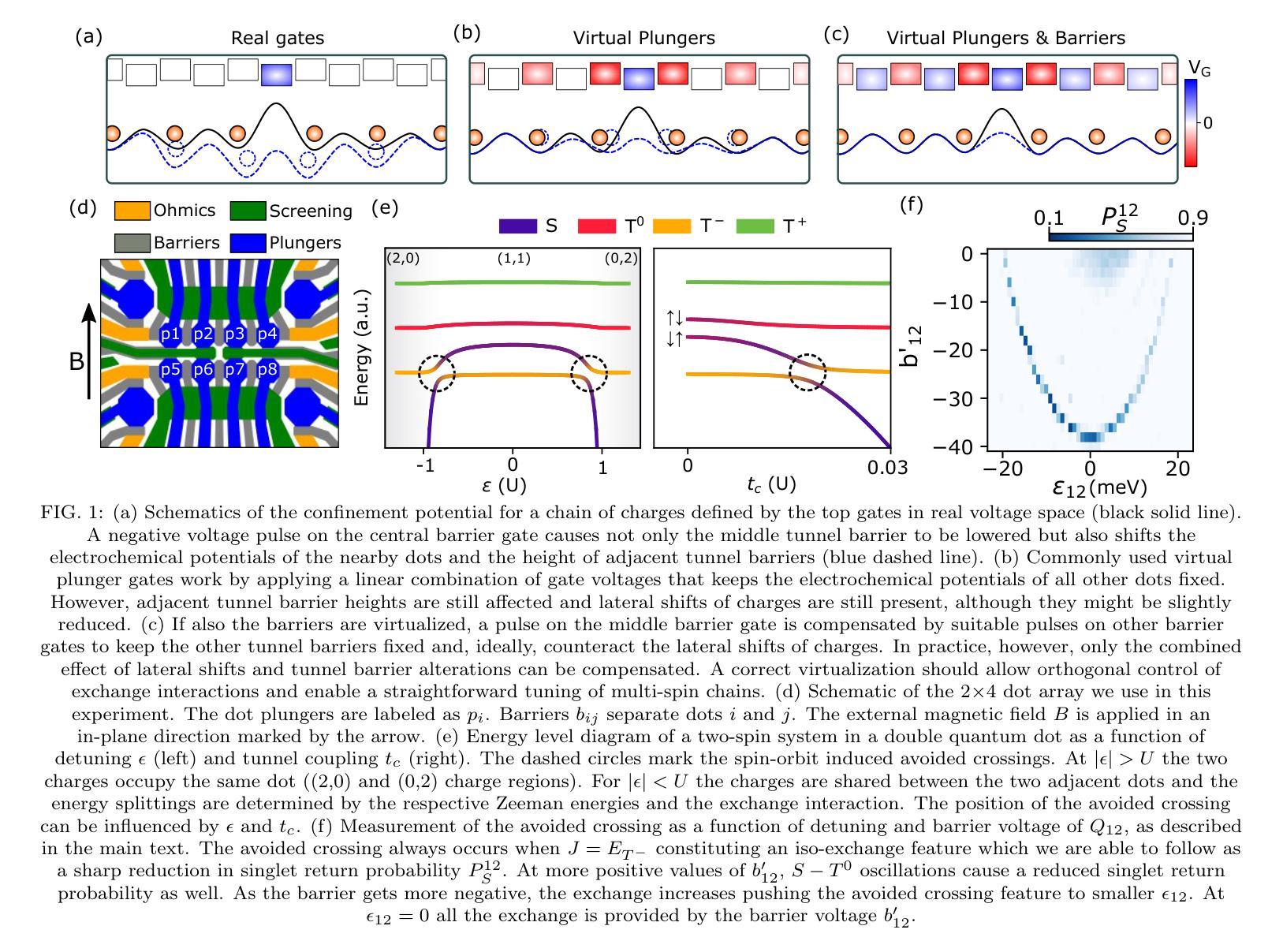
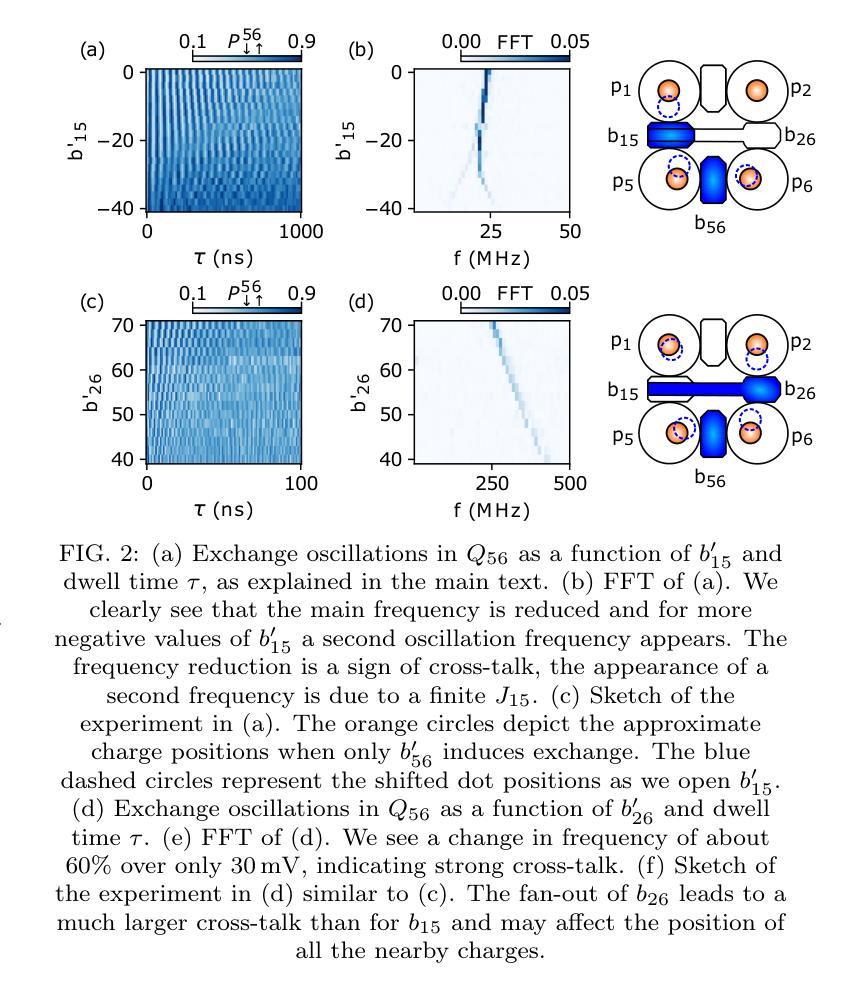
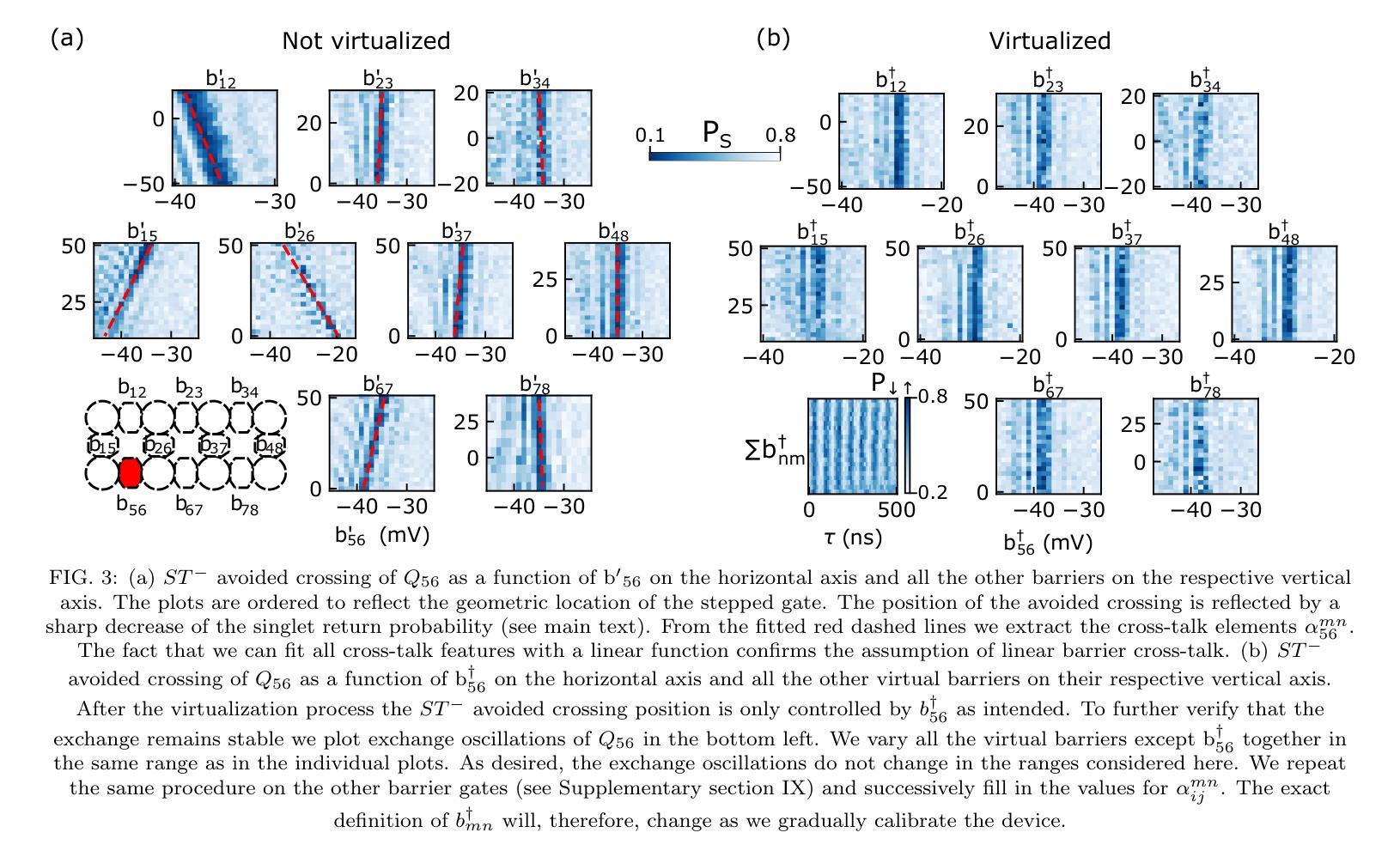
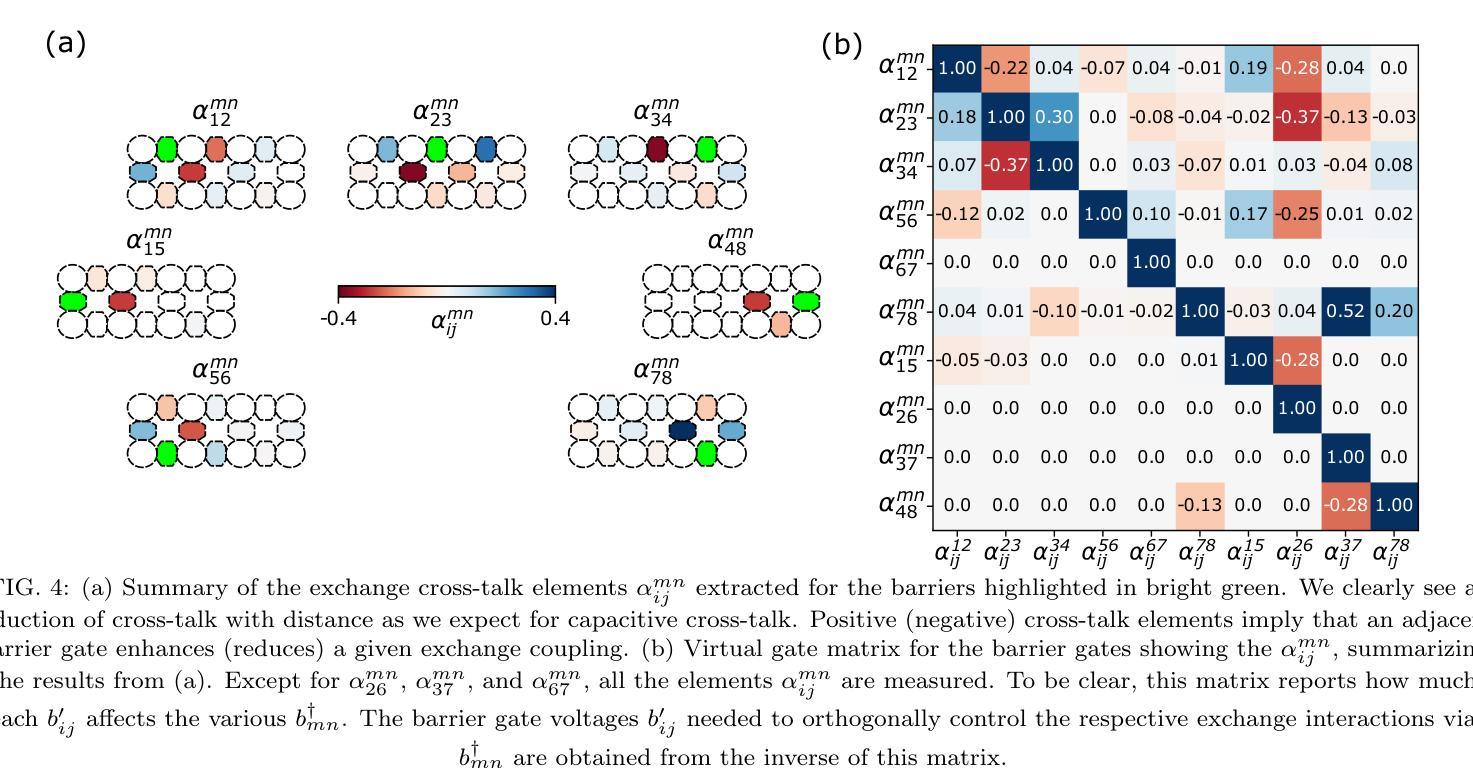
DeepDubber-V1: Towards High Quality and Dialogue, Narration, Monologue Adaptive Movie Dubbing Via Multi-Modal Chain-of-Thoughts Reasoning Guidance
Authors:Junjie Zheng, Zihao Chen, Chaofan Ding, Xinhan Di
Current movie dubbing technology can generate the desired voice from a given speech prompt, ensuring good synchronization between speech and visuals while accurately conveying the intended emotions. However, in movie dubbing, key aspects such as adapting to different dubbing styles, handling dialogue, narration, and monologue effectively, and understanding subtle details like the age and gender of speakers, have not been well studied. To address this challenge, we propose a framework of multi-modal large language model. First, it utilizes multimodal Chain-of-Thought (CoT) reasoning methods on visual inputs to understand dubbing styles and fine-grained attributes. Second, it generates high-quality dubbing through large speech generation models, guided by multimodal conditions. Additionally, we have developed a movie dubbing dataset with CoT annotations. The evaluation results demonstrate a performance improvement over state-of-the-art methods across multiple datasets. In particular, for the evaluation metrics, the SPK-SIM and EMO-SIM increases from 82.48% to 89.74%, 66.24% to 78.88% for dubbing setting 2.0 on V2C Animation dataset, LSE-D and MCD-SL decreases from 14.79 to 14.63, 5.24 to 4.74 for dubbing setting 2.0 on Grid dataset, SPK-SIM increases from 64.03 to 83.42 and WER decreases from 52.69% to 23.20% for initial reasoning setting on proposed CoT-Movie-Dubbing dataset in the comparison with the state-of-the art models.
当前电影配音技术可以根据给定的语音提示生成所需的语音,确保语音和视觉之间的良好同步,同时准确传达预期的情绪。然而,在电影配音中,如何适应不同的配音风格、有效处理对话、旁白和独白,以及理解如说话人的年龄和性别等细微之处尚未得到充分研究。为了应对这一挑战,我们提出了一个多模态大型语言模型框架。首先,它利用视觉输入的视觉模态链式思维(Chain-of-Thought,简称COT)推理方法来理解配音风格和精细属性。其次,通过大型语音生成模型生成高质量的配音,由多模态条件引导。此外,我们还开发了一个带有COT注释的电影配音数据集。评估结果表明,与现有方法相比,我们的方法在多个数据集上的性能有所提升。具体来说,对于评估指标,V2C动画数据集的配音设置2.0中,SPK-SIM和EMO-SIM分别从82.48%提升至89.74%、从66.24%提升至78.88%;Grid数据集的配音设置2.0中,LSE-D和MCD-SL分别从14.79下降至14.63、从5.24下降至4.74;在与现有模型的比较中,我们在提出的COT-Movie-Dubbing数据集上的初步推理设置中,SPK-SIM从64.03%提升至83.42%,WER从52.69%降至23.20%。
论文及项目相关链接
PDF 11 pages, 5 figures
摘要
电影配音技术可从给定的语音提示生成所需的语音,确保语音和视觉之间的良好同步,同时准确传达情感。然而,在电影配音中,如何适应不同的配音风格、有效处理对话、旁白和独白,以及理解如说话人的年龄和性别等细微细节尚未得到充分研究。为应对这一挑战,我们提出了多模态大型语言模型框架。首先,它利用视觉输入的链式思维推理方法理解配音风格和精细属性。其次,通过大型语音生成模型生成高质量配音,由多模态条件指导。此外,我们开发了带有思维链注释的电影配音数据集。评估结果表明,与最新技术相比,我们的方法在多个数据集上的表现有所提高。特别是,在V2C动画数据集上的说话人相似性(SPK-SIM)和情感相似性(EMO-SIM)从82.48%提高到89.74%,在网格数据集上的语音清晰度和语调连贯性得分也有所下降。与我们提出的带有初始推理设置的CoT电影配音数据集相比,SPK-SIM从64.03%提高到83.42%,词错误率(WER)从52.69%下降到23.20%。
关键见解
- 当前电影配音技术可以从给定的语音提示生成语音,确保语音和视觉同步,并准确传达情感。
- 配音风格的理解与适应、对话、旁白和独白的处理以及细微细节的理解是电影配音中的关键方面。
- 提出了多模态大型语言模型框架,通过链式思维推理方法理解配音风格和精细属性。
- 利用大型语音生成模型生成高质量配音,由多模态条件指导。
- 开发了带有思维链注释的电影配音数据集,用于评估和改进配音技术。
- 与现有技术相比,该框架在多个数据集上的表现有所提高,包括说话人相似性和情感相似性等方面的显著提升。
点此查看论文截图
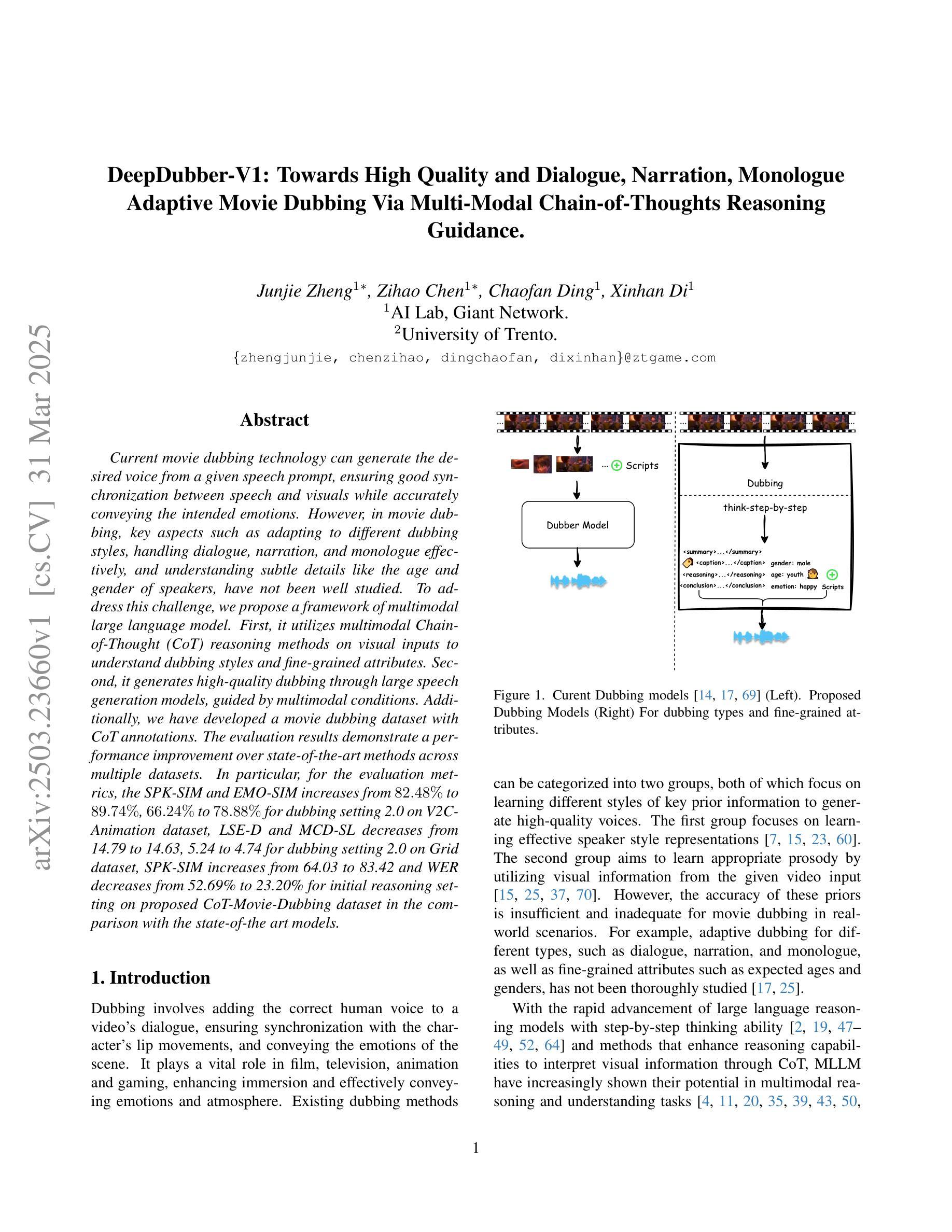
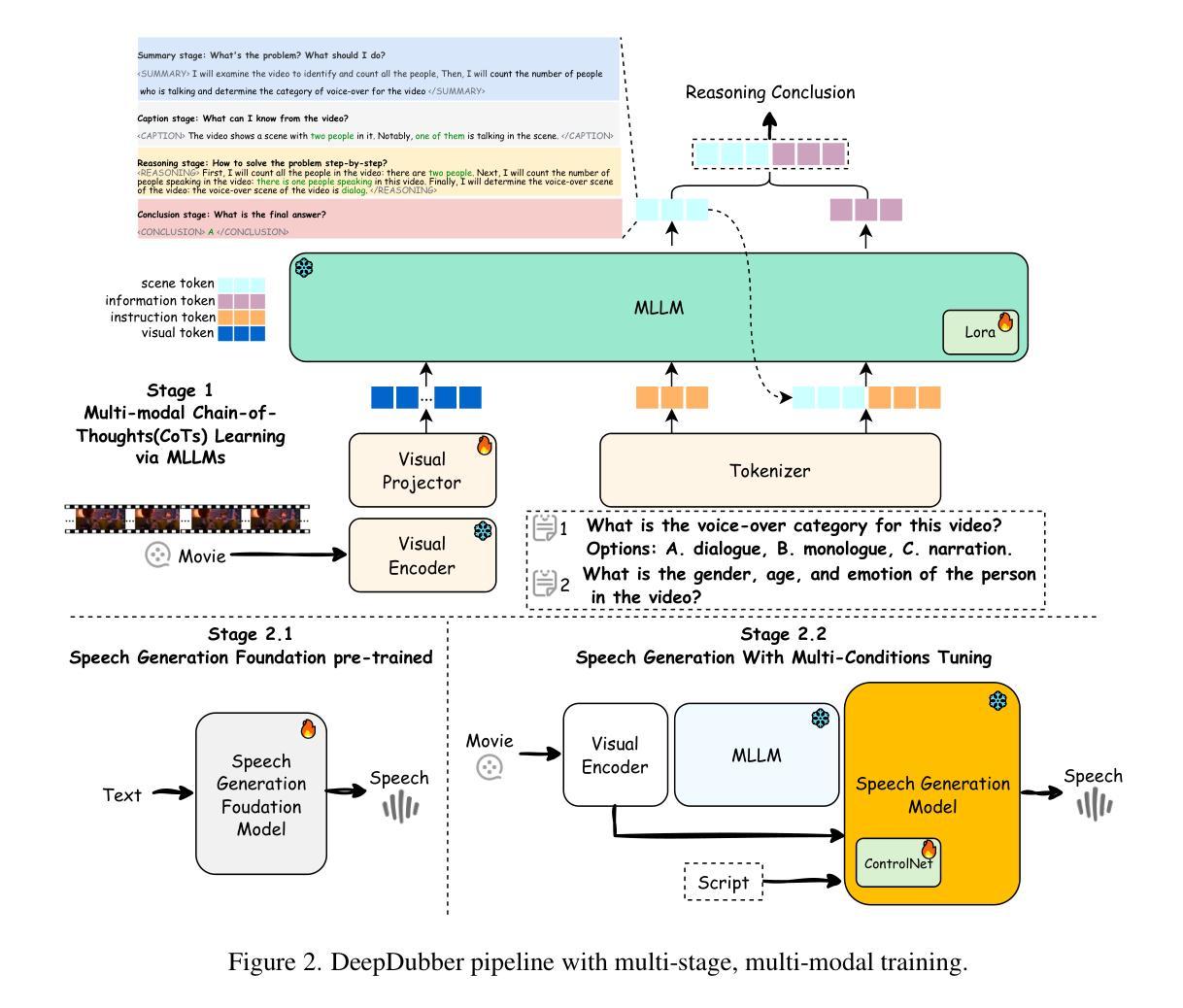
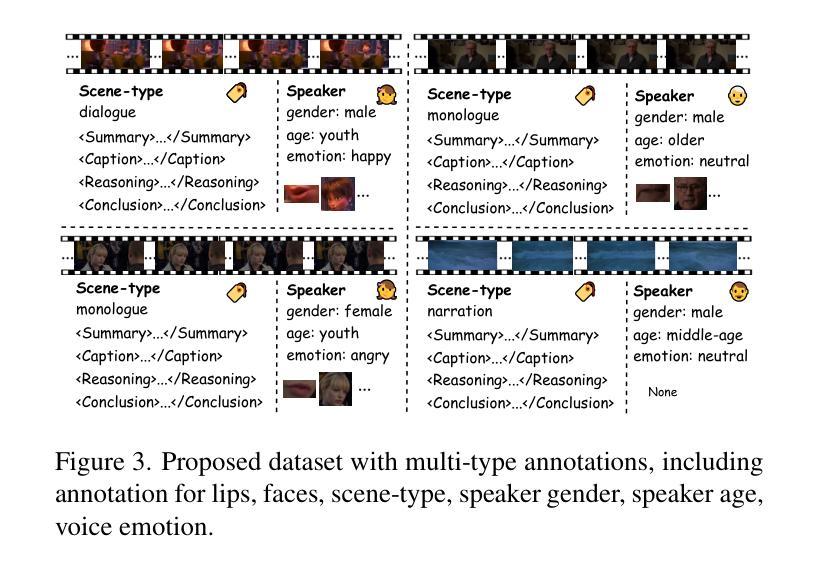
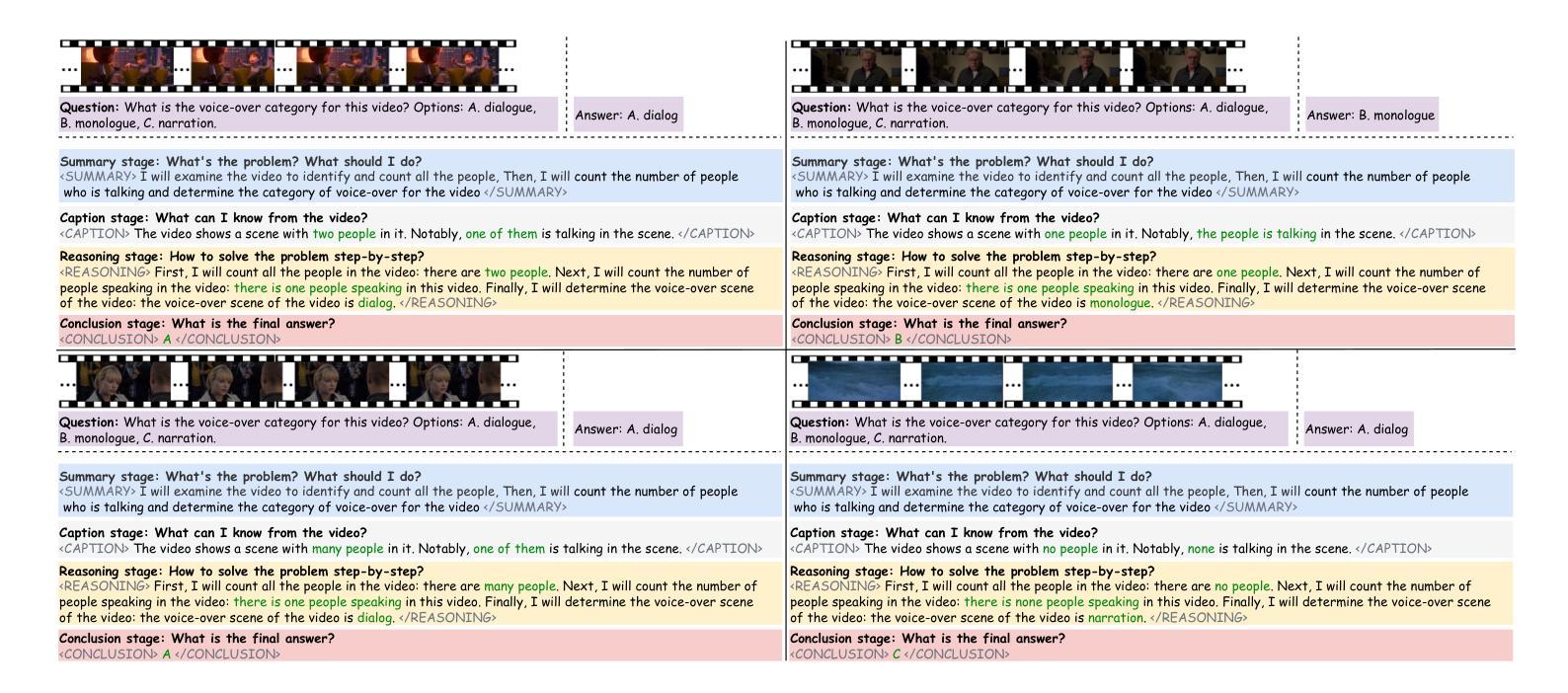
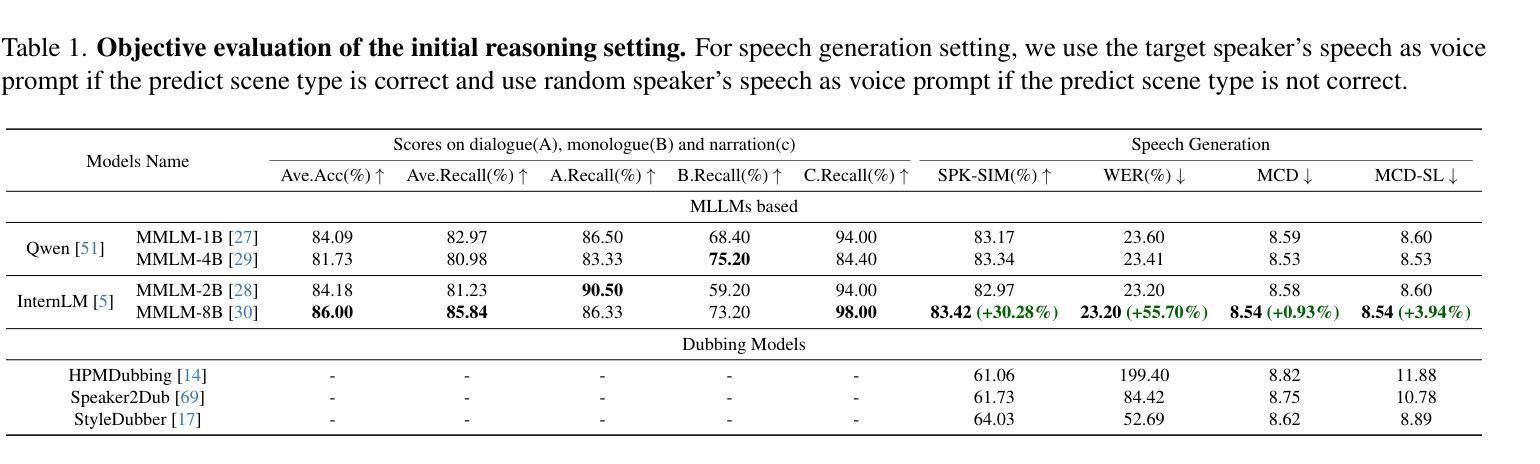
STSA: Spatial-Temporal Semantic Alignment for Visual Dubbing
Authors:Zijun Ding, Mingdie Xiong, Congcong Zhu, Jingrun Chen
Existing audio-driven visual dubbing methods have achieved great success. Despite this, we observe that the semantic ambiguity between spatial and temporal domains significantly degrades the synthesis stability for the dynamic faces. We argue that aligning the semantic features from spatial and temporal domains is a promising approach to stabilizing facial motion. To achieve this, we propose a Spatial-Temporal Semantic Alignment (STSA) method, which introduces a dual-path alignment mechanism and a differentiable semantic representation. The former leverages a Consistent Information Learning (CIL) module to maximize the mutual information at multiple scales, thereby reducing the manifold differences between spatial and temporal domains. The latter utilizes probabilistic heatmap as ambiguity-tolerant guidance to avoid the abnormal dynamics of the synthesized faces caused by slight semantic jittering. Extensive experimental results demonstrate the superiority of the proposed STSA, especially in terms of image quality and synthesis stability. Pre-trained weights and inference code are available at https://github.com/SCAILab-USTC/STSA.
现有的音频驱动视觉配音方法已经取得了巨大的成功。尽管如此,我们观察到空间和时间域之间的语义模糊性显著降低了动态面部的合成稳定性。我们认为,对齐空间和时间域的语义特征是一种稳定面部运动的可行方法。为此,我们提出了一种空间时间语义对齐(STSA)方法,它引入了一种双路径对齐机制和一种可区分的语义表示。前者利用一致信息学习(CIL)模块来最大化多个尺度的互信息,从而减少空间和时间域之间的流形差异。后者利用概率热图作为模糊容忍引导,以避免轻微语义抖动引起的合成面部异常动态。大量的实验结果证明了所提出的STSA的优越性,特别是在图像质量和合成稳定性方面。预训练的权重和推理代码可在https://github.com/SCAILab-USTC/STSA获得。
论文及项目相关链接
PDF Accepted by ICME 2025
Summary
本文提出一种针对动态面部合成的空间时间语义对齐(STSA)方法,通过双路径对齐机制和可微分语义表示,解决现有音频驱动视觉配音方法中语义模糊导致的问题,提高面部合成稳定性。
Key Takeaways
- 语义模糊是现有音频驱动视觉配音方法面临的主要问题,影响动态面部合成的稳定性。
- 空间时间语义对齐(STSA)方法被提出以解决这一问题。
- STSA方法采用双路径对齐机制,通过最大化多尺度上的互信息来减少空间和时间域之间的差异。
- STSA方法利用可微分语义表示,采用概率热图作为模糊容忍指导,避免合成面部的异常动态。
- 实验结果表明,STSA方法在图像质量和合成稳定性方面表现出优越性。
- 可在https://github.com/SCAILab-USTC/STSA获取预训练权重和推理代码。
点此查看论文截图
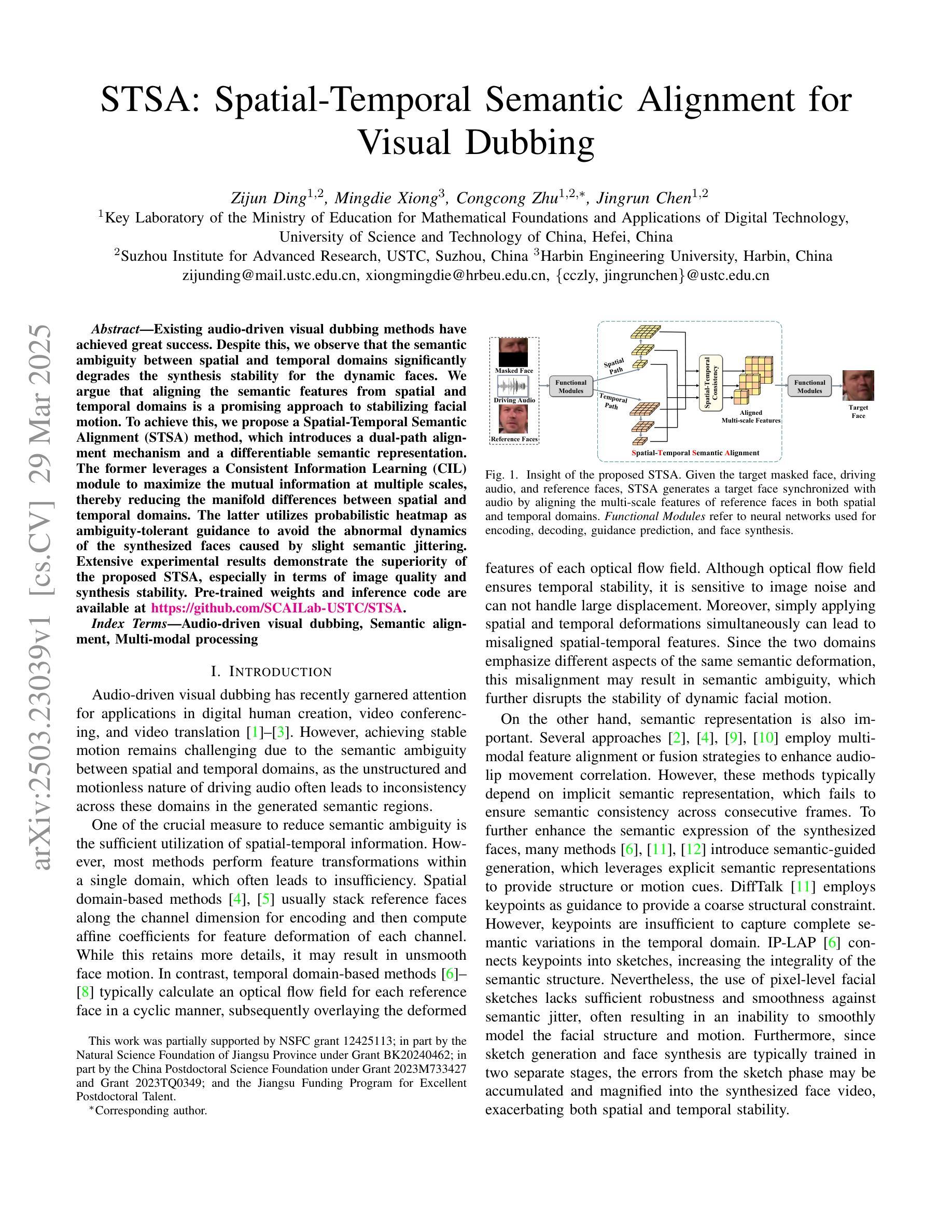
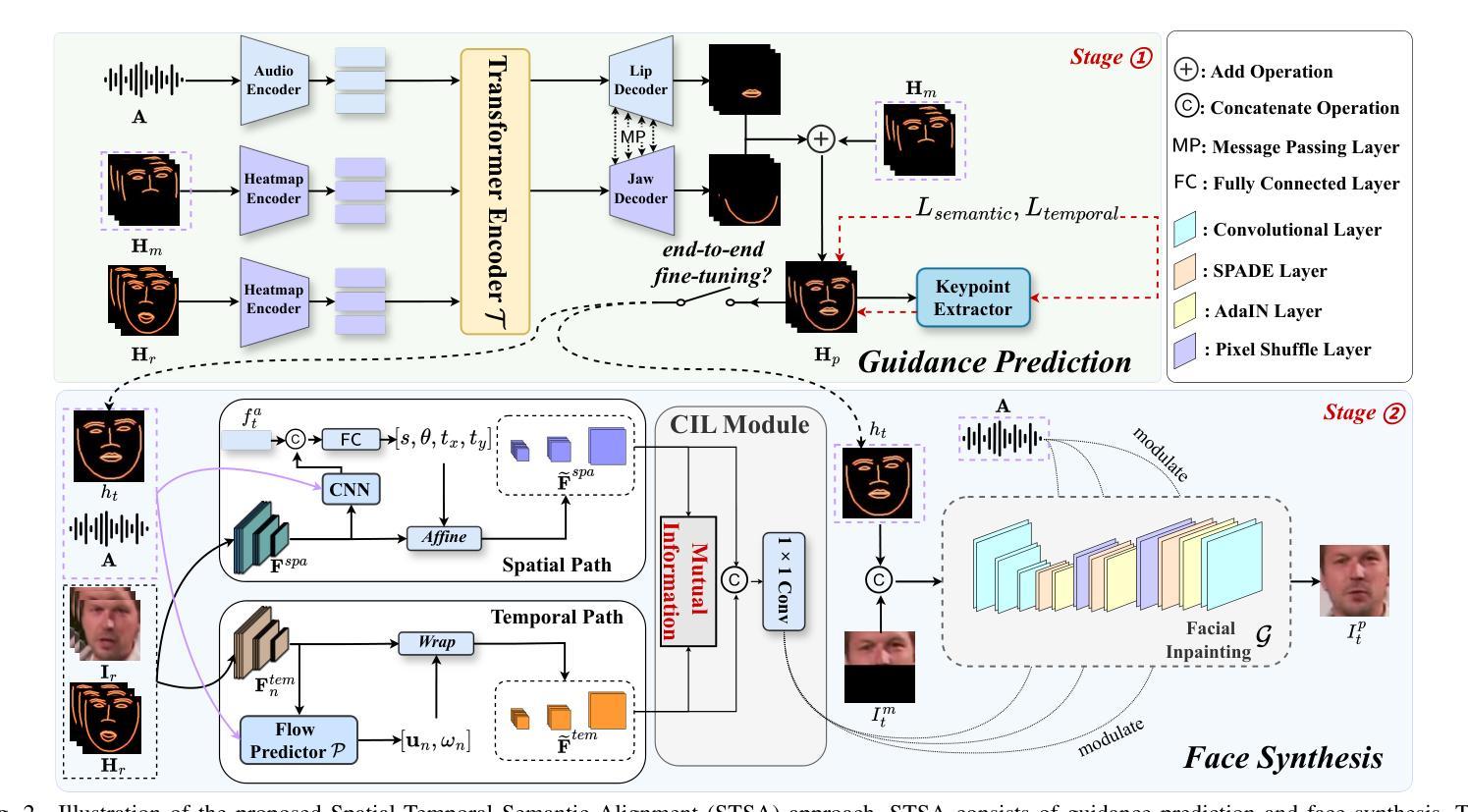
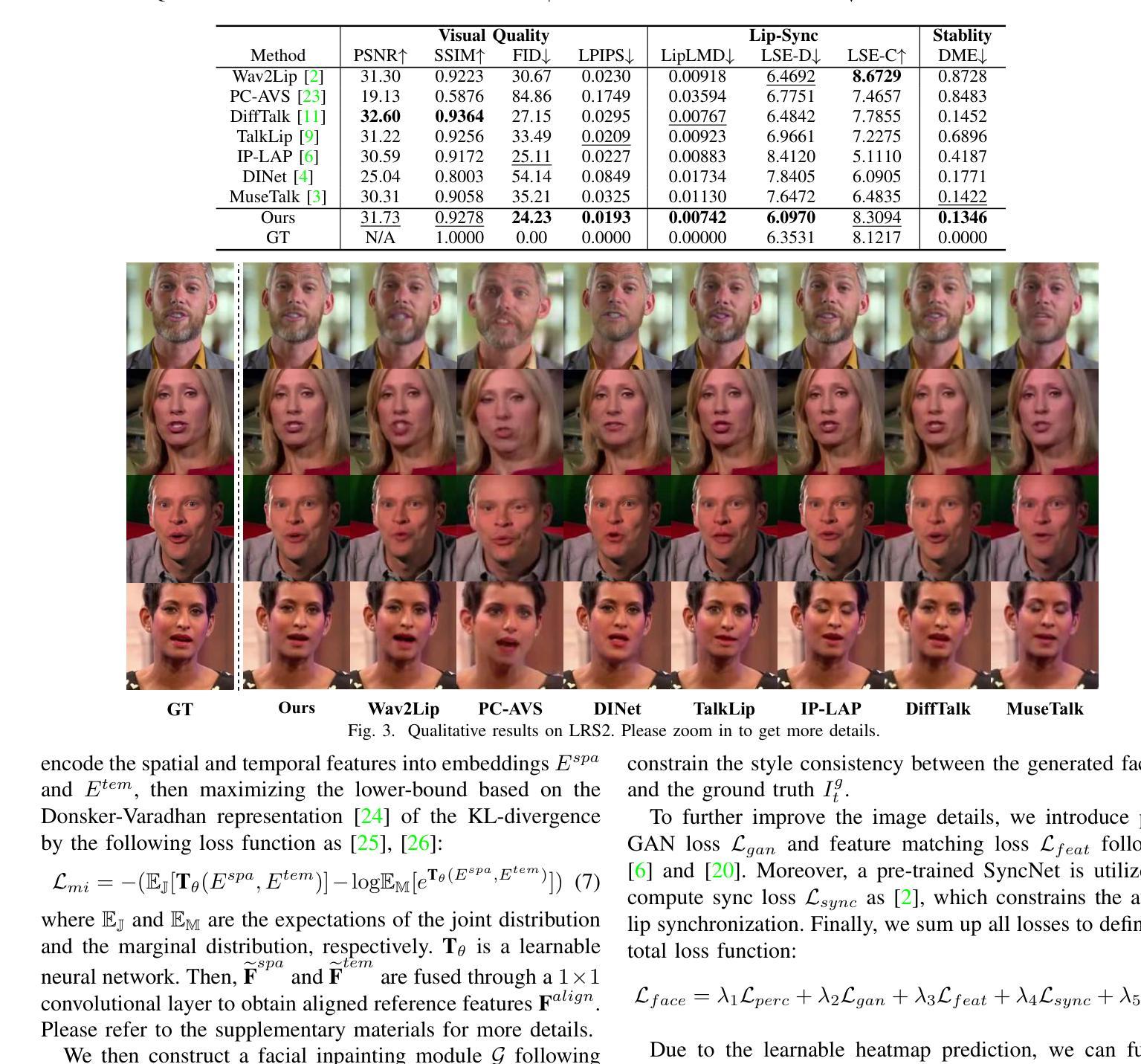
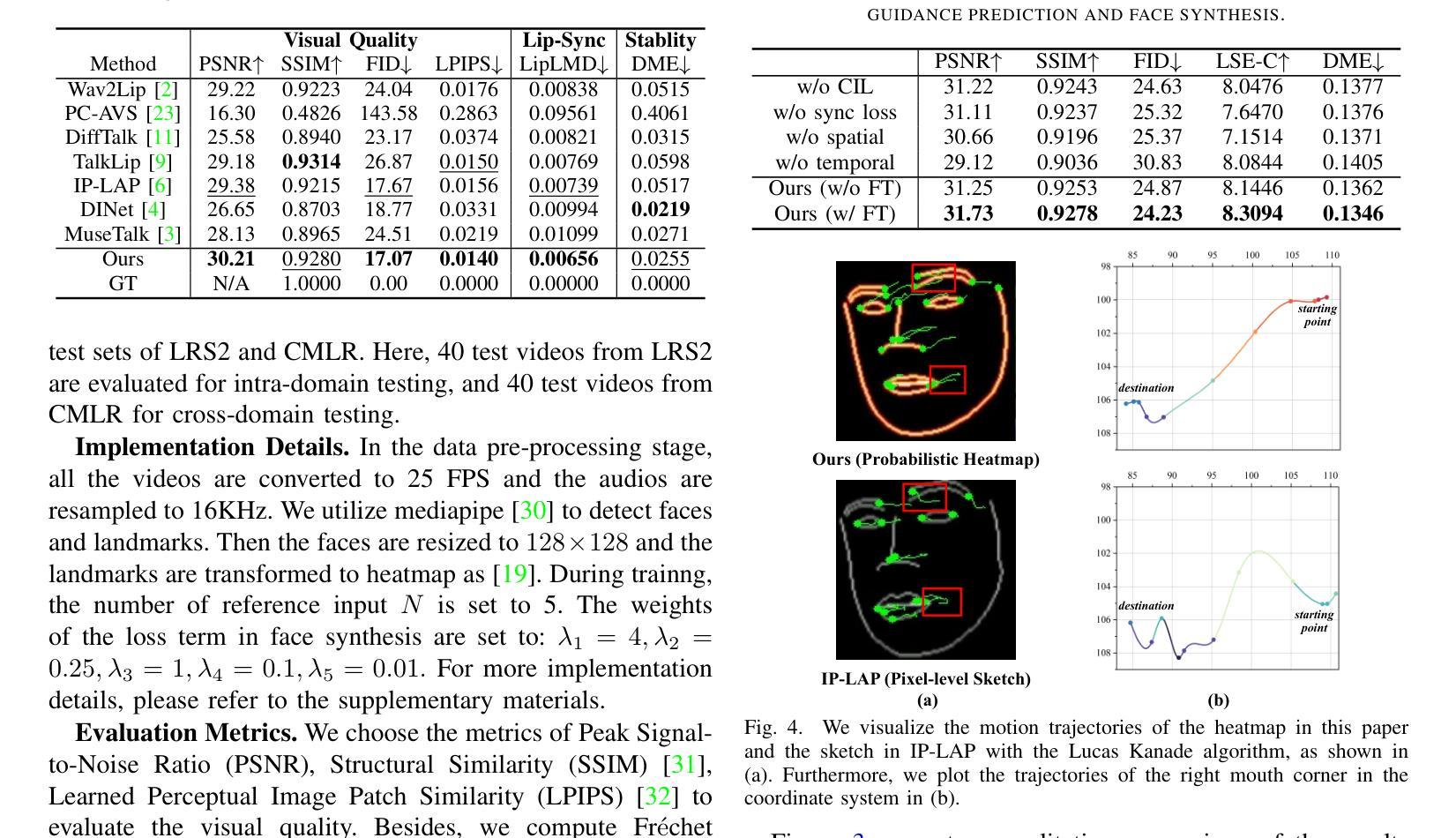
Dual Audio-Centric Modality Coupling for Talking Head Generation
Authors:Ao Fu, Ziqi Ni, Yi Zhou
The generation of audio-driven talking head videos is a key challenge in computer vision and graphics, with applications in virtual avatars and digital media. Traditional approaches often struggle with capturing the complex interaction between audio and facial dynamics, leading to lip synchronization and visual quality issues. In this paper, we propose a novel NeRF-based framework, Dual Audio-Centric Modality Coupling (DAMC), which effectively integrates content and dynamic features from audio inputs. By leveraging a dual encoder structure, DAMC captures semantic content through the Content-Aware Encoder and ensures precise visual synchronization through the Dynamic-Sync Encoder. These features are fused using a Cross-Synchronized Fusion Module (CSFM), enhancing content representation and lip synchronization. Extensive experiments show that our method outperforms existing state-of-the-art approaches in key metrics such as lip synchronization accuracy and image quality, demonstrating robust generalization across various audio inputs, including synthetic speech from text-to-speech (TTS) systems. Our results provide a promising solution for high-quality, audio-driven talking head generation and present a scalable approach for creating realistic talking heads.
音频驱动式说话人头部视频生成是计算机视觉和图形学领域的关键挑战,在虚拟化身和数字媒体中有广泛应用。传统方法往往难以捕捉音频和面部动态之间的复杂交互,导致唇同步和视觉质量问题。在本文中,我们提出了一种基于NeRF的新型框架——双音频中心模态耦合(DAMC),该框架有效地融合了音频输入的文本内容和动态特征。通过利用双编码器结构,DAMC通过内容感知编码器捕获语义内容,并通过动态同步编码器确保精确的视觉同步。这些特征通过跨同步融合模块(CSFM)融合,增强了内容表示和唇同步功能。大量实验表明,我们的方法在唇同步准确性和图像质量等关键指标上优于现有最先进的方案,在包括来自文本到语音(TTS)系统的合成语音等各种音频输入上都表现出稳健的泛化能力。我们的研究结果为解决高质量音频驱动的说话人头部生成问题提供了前景广阔的解决方案,并提出了一种可创建逼真说话人头部的可扩展方法。
论文及项目相关链接
PDF 9 pages, 4 figures
Summary
音频驱动式谈话头视频生成是计算机视觉和图形学领域的关键挑战,应用于虚拟角色和数字媒体。本文提出基于NeRF的Dual Audio-Centric Modality Coupling(DAMC)框架,有效整合音频输入的静态和动态特征。通过双重编码器结构,DAMC通过内容感知编码器捕捉语义内容,并通过动态同步编码器确保精确视觉同步。这些特征通过跨同步融合模块进行融合,提高内容表达和唇同步效果。实验表明,该方法在唇同步精度和图像质量等关键指标上优于现有先进技术,且在各种音频输入上展现出强大的泛化能力,包括文本到语音系统的合成语音。本研究为高质量音频驱动谈话头生成提供有前景的解决方案。
Key Takeaways
- 音频驱动谈话头视频生成是计算机视觉和图形学的重要挑战,具有虚拟角色和数字媒体应用。
- 传统方法难以捕捉音频和面部动作之间的复杂交互,导致唇同步和视觉质量问题。
- DAMC框架基于NeRF技术,有效整合音频的静态和动态特征。
- 双重编码器结构包括内容感知编码器和动态同步编码器,分别捕捉语义内容和确保精确视觉同步。
- 跨同步融合模块(CSFM)融合静态和动态特征,提高内容表达和唇同步效果。
- 与现有方法相比,DAMC在唇同步精度和图像质量等方面表现出优越性能。
点此查看论文截图
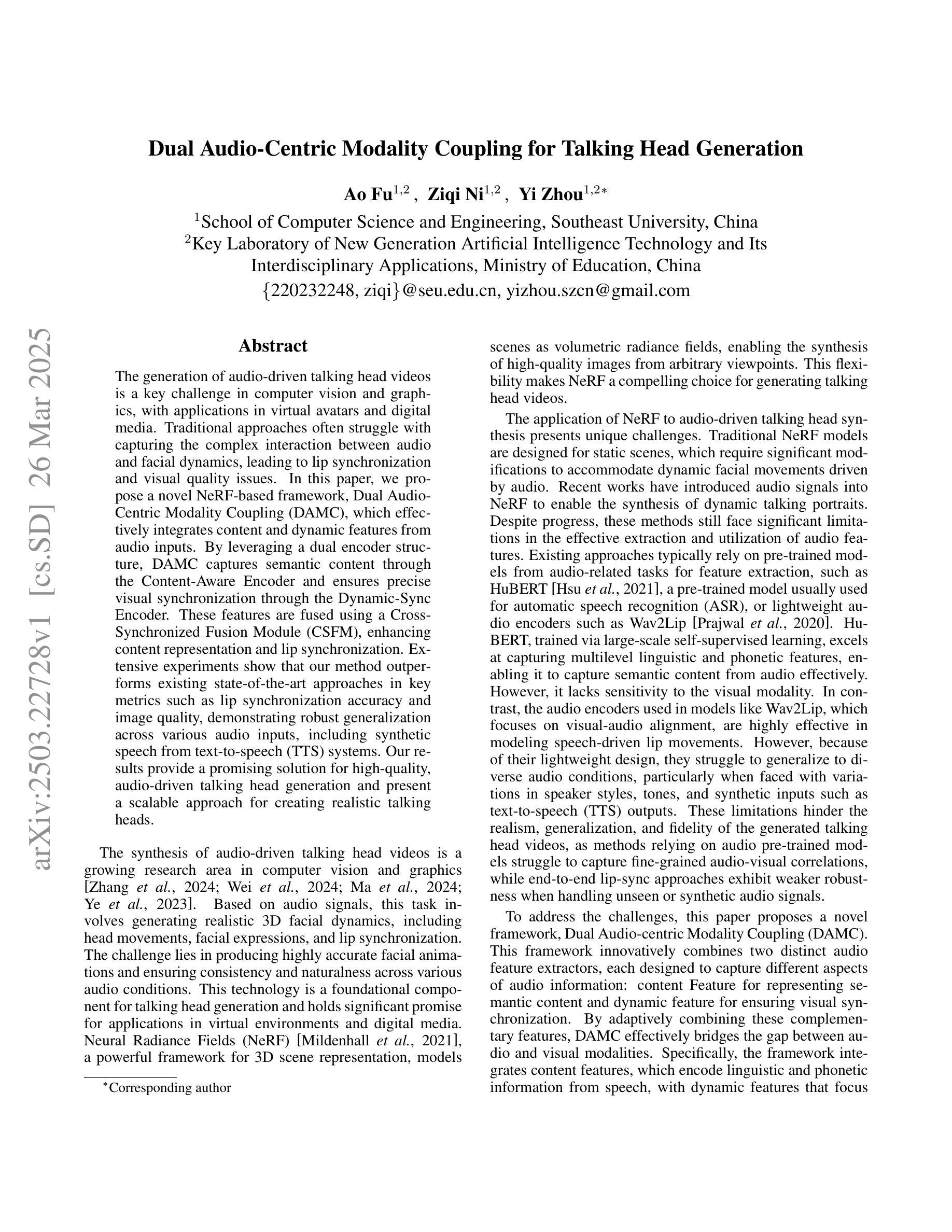
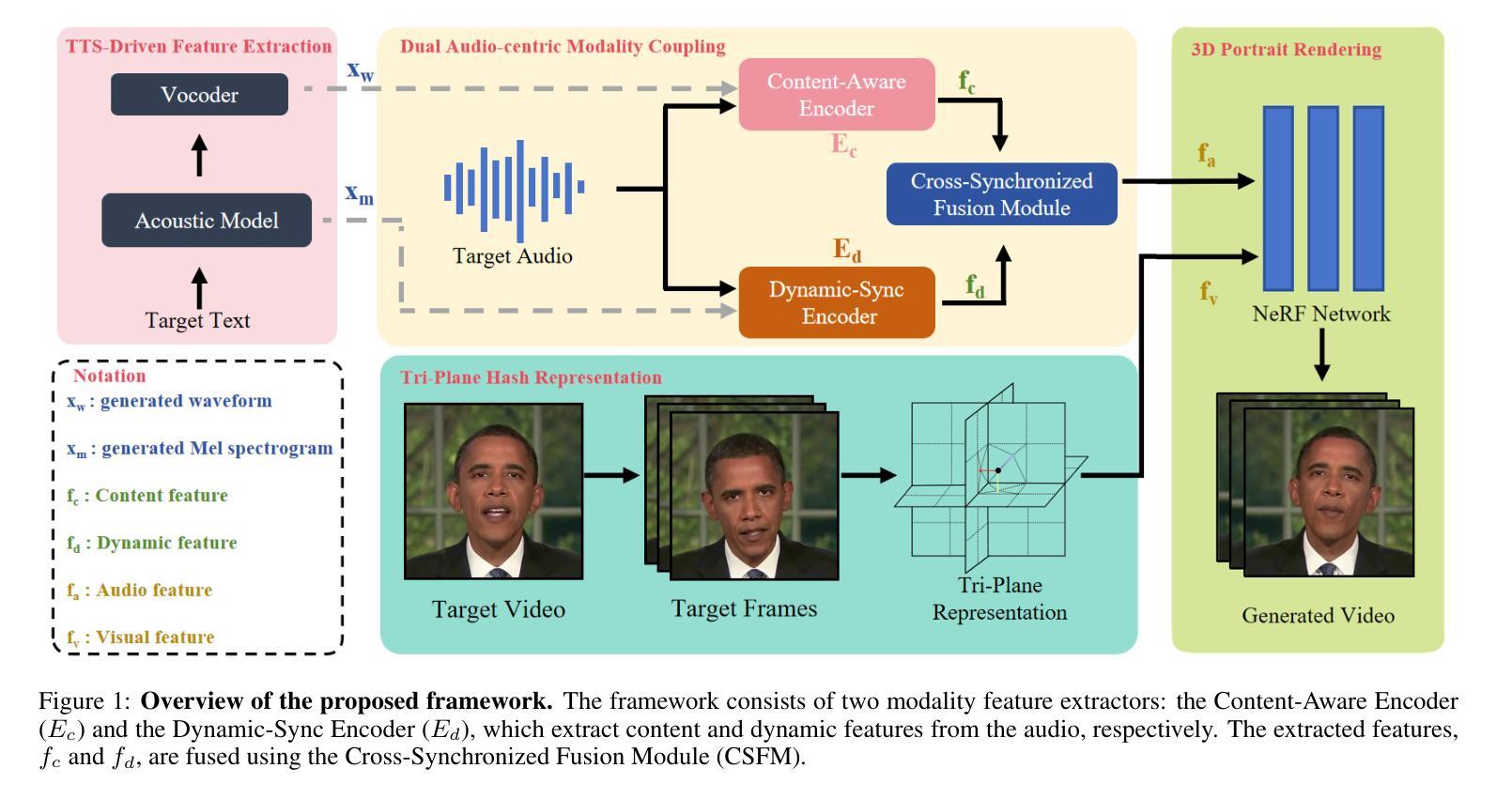
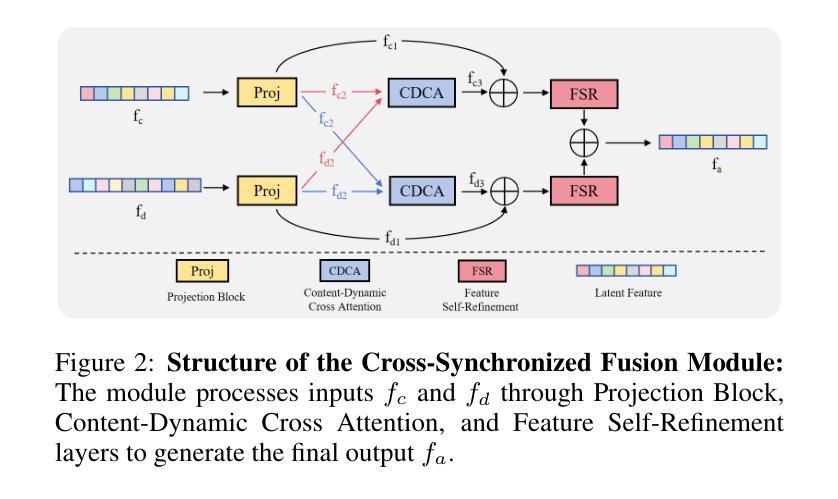
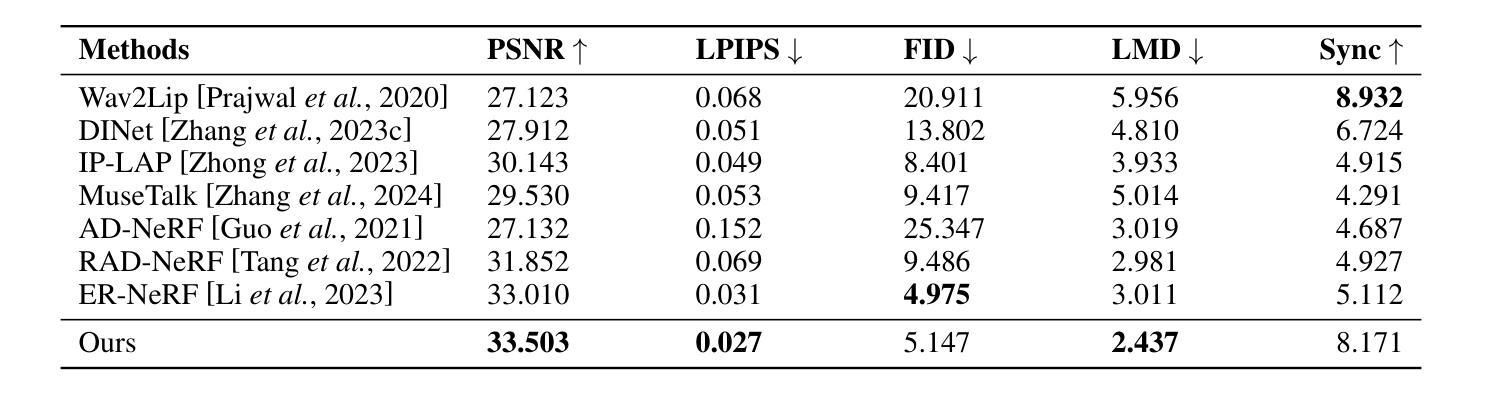
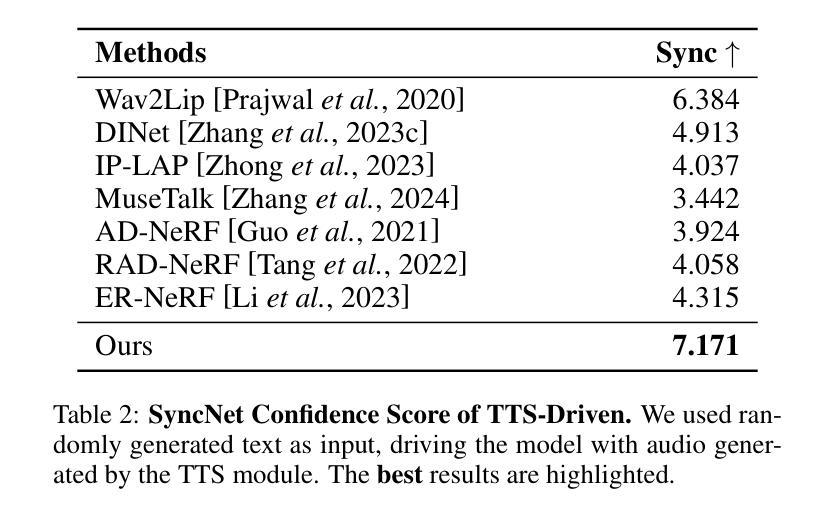
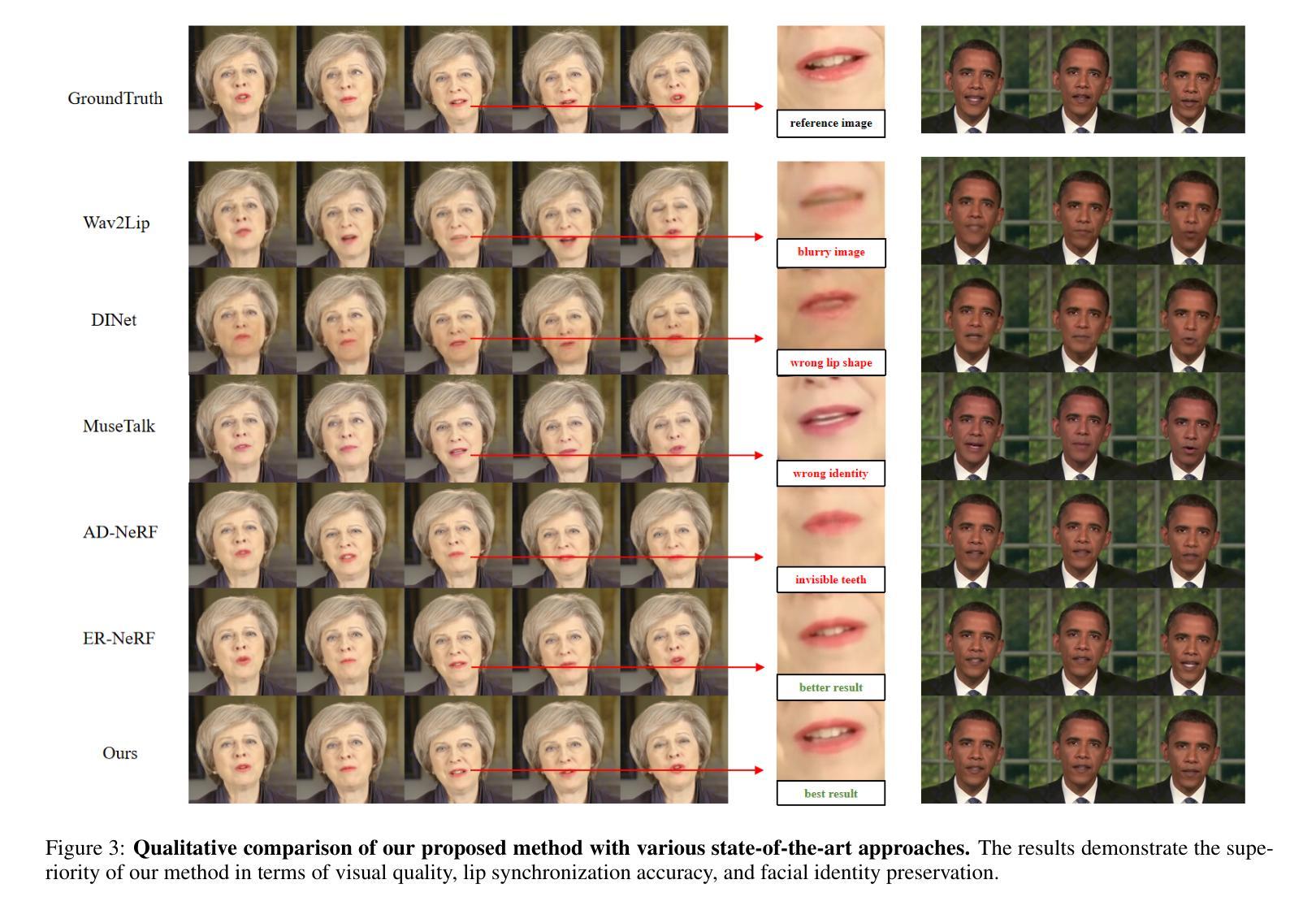
Audio-Plane: Audio Factorization Plane Gaussian Splatting for Real-Time Talking Head Synthesis
Authors:Shuai Shen, Wanhua Li, Yunpeng Zhang, Weipeng Hu, Yap-Peng Tan
Talking head synthesis has become a key research area in computer graphics and multimedia, yet most existing methods often struggle to balance generation quality with computational efficiency. In this paper, we present a novel approach that leverages an Audio Factorization Plane (Audio-Plane) based Gaussian Splatting for high-quality and real-time talking head generation. For modeling a dynamic talking head, 4D volume representation is needed. However, directly storing a dense 4D grid is impractical due to the high cost and lack of scalability for longer durations. We overcome this challenge with the proposed Audio-Plane, where the 4D volume representation is decomposed into audio-independent space planes and audio-dependent planes. This provides a compact and interpretable feature representation for talking head, facilitating more precise audio-aware spatial encoding and enhanced audio-driven lip dynamic modeling. To further improve speech dynamics, we develop a dynamic splatting method that helps the network more effectively focus on modeling the dynamics of the mouth region. Extensive experiments demonstrate that by integrating these innovations with the powerful Gaussian Splatting, our method is capable of synthesizing highly realistic talking videos in real time while ensuring precise audio-lip synchronization. Synthesized results are available in https://sstzal.github.io/Audio-Plane/.
谈话头合成已成为计算机图形学和多媒体领域的一个关键研究课题,然而,大多数现有方法往往难以在生成质量和计算效率之间取得平衡。在本文中,我们提出了一种新方法,利用基于音频分解平面(Audio-Plane)的高斯溅出(Gaussian Splatting)进行高质量实时谈话头生成。为了模拟动态的谈话头,需要4D体积表示。然而,由于成本高昂和缺乏长期可扩展性,直接存储密集的4D网格并不实用。我们克服了这一挑战,采用了所提出的音频平面(Audio-Plane),其中将4D体积表示分解成与音频无关的空间平面和与音频相关的平面。这为谈话头提供了紧凑且可解释的特征表示,促进了更精确的声音感知空间编码和增强的音频驱动嘴唇动态建模。为了进一步提高语音动态效果,我们开发了一种动态溅出方法,帮助网络更有效地专注于嘴巴区域的动态建模。大量实验表明,通过将这些创新与强大的高斯溅出相结合,我们的方法能够在确保精确音频与嘴唇同步的同时,实时合成高度逼真的对话视频。合成结果可在https://sstzal.github.io/Audio-Plane/查看。
论文及项目相关链接
Summary
本文提出了一种基于音频分解平面的高斯溅泼法,用于高质量实时说话人头部的合成。针对动态说话人头部的建模,采用4D体积表示法,但直接存储密集4D网格不实际。因此,本文提出了音频分解平面,将4D体积表示分解为音频独立的空间平面和音频依赖平面,为说话头部提供紧凑且可解释的特征表示,促进更精确的音频感知空间编码和增强的音频驱动唇动态建模。实验表明,结合高斯溅泼法,该方法能实时合成高度逼真的说话视频,确保音频与嘴唇的精确同步。
Key Takeaways
- 说话头合成是计算机图形学和多媒体的关键研究领域,但平衡生成质量与计算效率是一大挑战。
- 本文提出了一种基于音频分解平面的高斯溅泼法,用于高质量实时说话头生成。
- 4D体积表示法用于建模动态说话头部,但直接存储密集4D网格不实际。
- 提出的音频分解平面解决了这一问题,将4D体积表示为音频独立和依赖的平面。
- 此方法提供了一种紧凑且可解释的特征表示,促进了更精确的音频感知空间编码和唇动态建模。
- 结合高斯溅泼法,该方法能实时合成高度逼真的说话视频。
- 合成结果可在链接查看。
点此查看论文截图
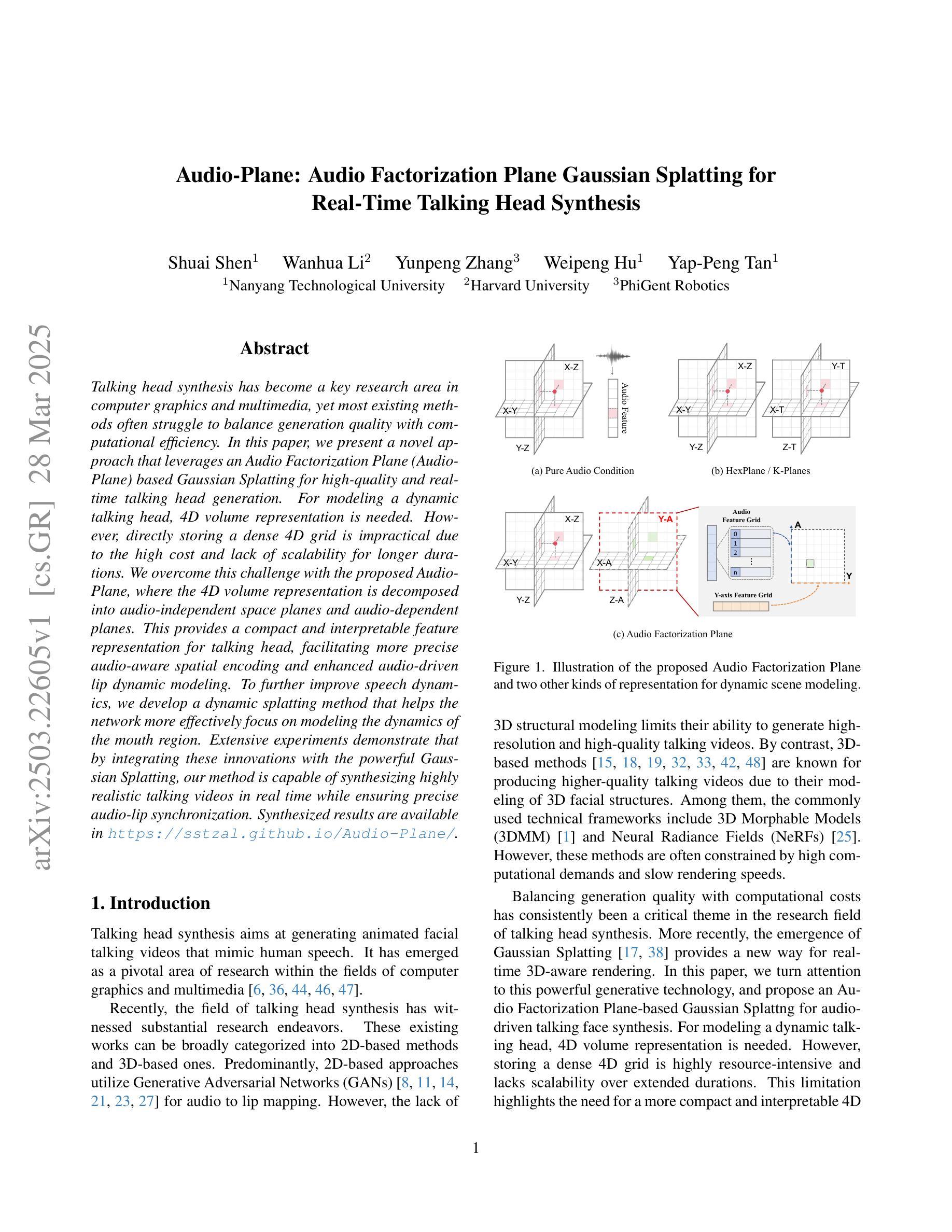
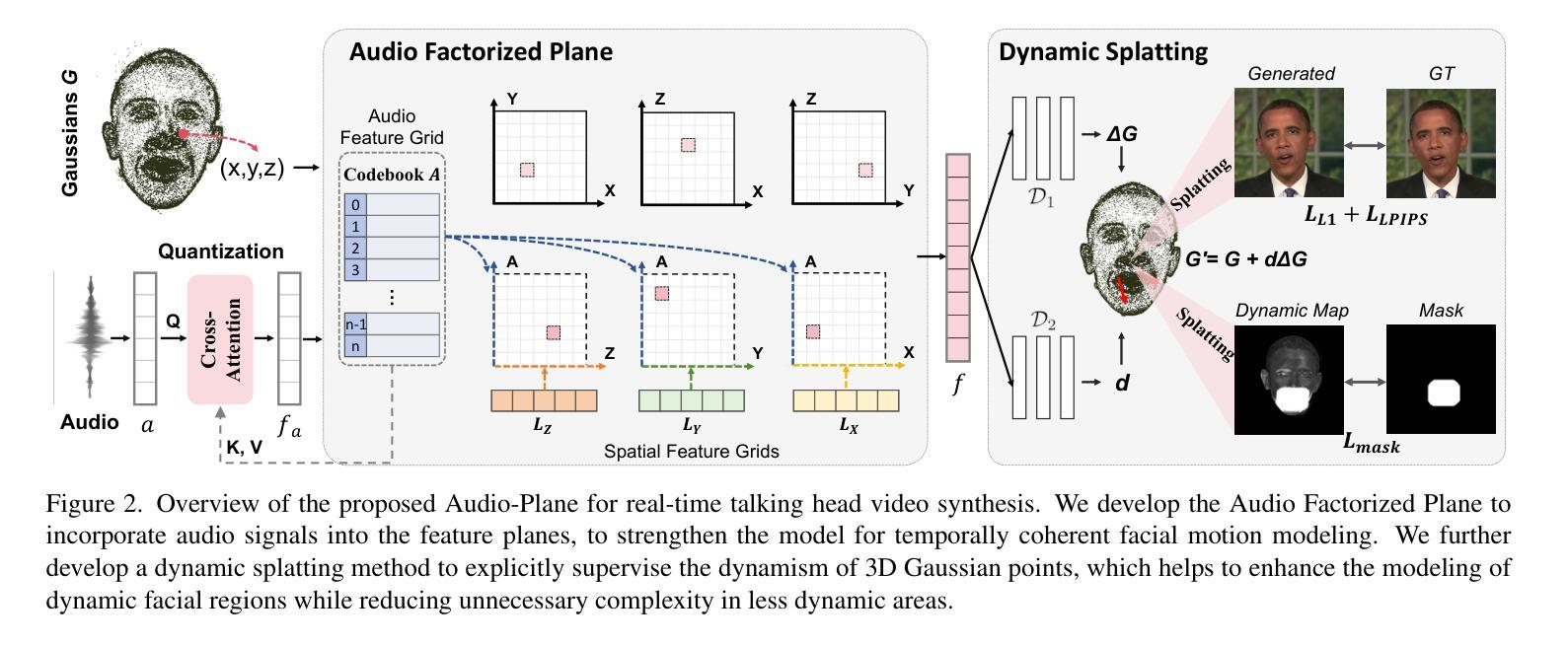
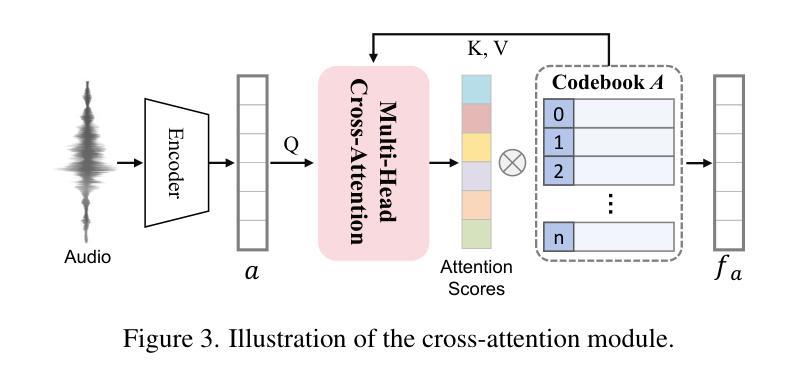
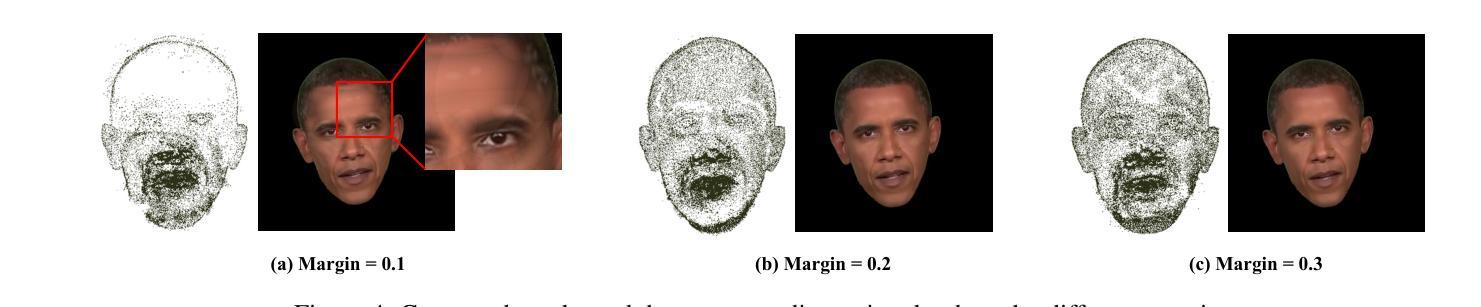
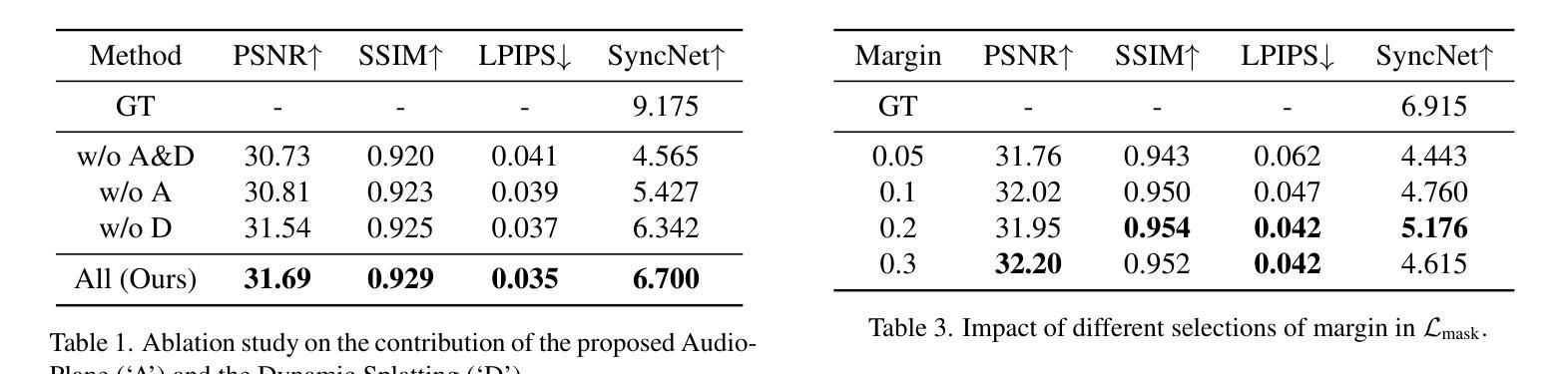
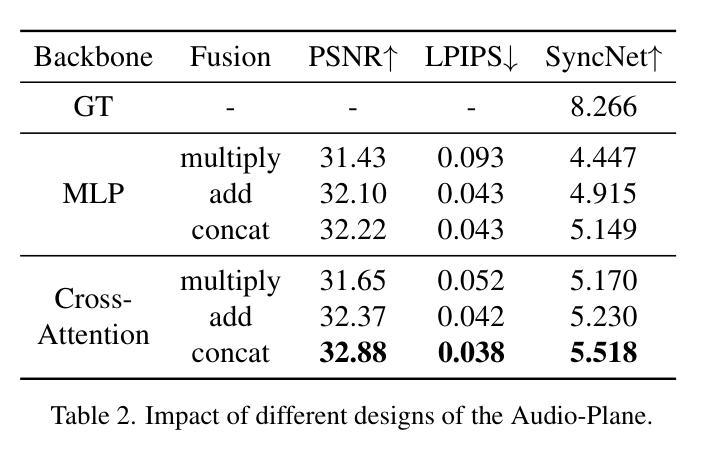
Follow Your Motion: A Generic Temporal Consistency Portrait Editing Framework with Trajectory Guidance
Authors:Haijie Yang, Zhenyu Zhang, Hao Tang, Jianjun Qian, Jian Yang
Pre-trained conditional diffusion models have demonstrated remarkable potential in image editing. However, they often face challenges with temporal consistency, particularly in the talking head domain, where continuous changes in facial expressions intensify the level of difficulty. These issues stem from the independent editing of individual images and the inherent loss of temporal continuity during the editing process. In this paper, we introduce Follow Your Motion (FYM), a generic framework for maintaining temporal consistency in portrait editing. Specifically, given portrait images rendered by a pre-trained 3D Gaussian Splatting model, we first develop a diffusion model that intuitively and inherently learns motion trajectory changes at different scales and pixel coordinates, from the first frame to each subsequent frame. This approach ensures that temporally inconsistent edited avatars inherit the motion information from the rendered avatars. Secondly, to maintain fine-grained expression temporal consistency in talking head editing, we propose a dynamic re-weighted attention mechanism. This mechanism assigns higher weight coefficients to landmark points in space and dynamically updates these weights based on landmark loss, achieving more consistent and refined facial expressions. Extensive experiments demonstrate that our method outperforms existing approaches in terms of temporal consistency and can be used to optimize and compensate for temporally inconsistent outputs in a range of applications, such as text-driven editing, relighting, and various other applications.
预训练条件扩散模型在图像编辑方面表现出显著潜力。然而,它们在时间连续性方面常常面临挑战,特别是在头部说话领域,面部表情的连续变化增加了难度。这些问题源于单独编辑的图像和编辑过程中固有的时间连续性丧失。在本文中,我们介绍了Follow Your Motion(FYM),这是一个保持肖像编辑中时间连续性的通用框架。具体来说,给定由预训练的3D高斯拼接模型渲染的肖像图像,我们首先开发了一种扩散模型,该模型直觉地、固有地学习从第一帧到每一后续帧不同尺度和像素坐标的运动轨迹变化。这种方法确保了时间上不一致的编辑化身继承了从渲染化身继承的运动信息。其次,为了保持头部说话中精细表情的时间连续性,我们提出了一种动态加权注意力机制。该机制在空间地标点上分配较高的权重系数,并根据地标损失动态更新这些权重,从而实现更一致和更精细的面部表情。大量实验表明,我们的方法在时间连续性方面优于现有方法,并可用于优化和补偿一系列应用中时间上不一致的输出,如文本驱动编辑、重新照明和其他各种应用。
论文及项目相关链接
PDF https://anonymous-hub1127.github.io/FYM.github.io/
Summary
本文提出了一种基于动态重建加权注意力机制的临时一致性框架Follow Your Motion(FYM),用于肖像编辑中的说话头生成。该框架使用预训练的3D高斯喷射模型渲染的肖像图像,通过开发一种扩散模型来学习和保持不同尺度和像素坐标的运动轨迹变化,确保编辑后的化身在时间上的连续性。此外,还引入了一种动态加权注意力机制,以维持精细表情的时间一致性。实验证明,该方法在时间上的一致性和精细表情的处理上优于现有方法。
Key Takeaways
- 介绍了基于预训练条件扩散模型的说话头生成面临的挑战。这类挑战主要由于对单独图像独立编辑时的时序一致性损失所导致。特别是在面部表情连续变化的情境中,挑战更加严重。
- 提出了一种新的通用框架Follow Your Motion (FYM),旨在维护肖像编辑中的时序一致性。通过开发扩散模型并使其学习与特定时间节点相关的运动轨迹变化,该框架解决了时序不一致的问题。
- FYM框架利用预训练的3D高斯喷射模型进行肖像图像渲染,并利用动态重建加权注意力机制确保面部表情的时间连续性。该机制能够动态更新权重系数,从而实现更一致和精细的面部表情处理。
- 该方法可以通过文本驱动编辑等多种应用来优化和改进时序不一致的输出结果。此外,它还适用于其他领域,如重光照等。
点此查看论文截图
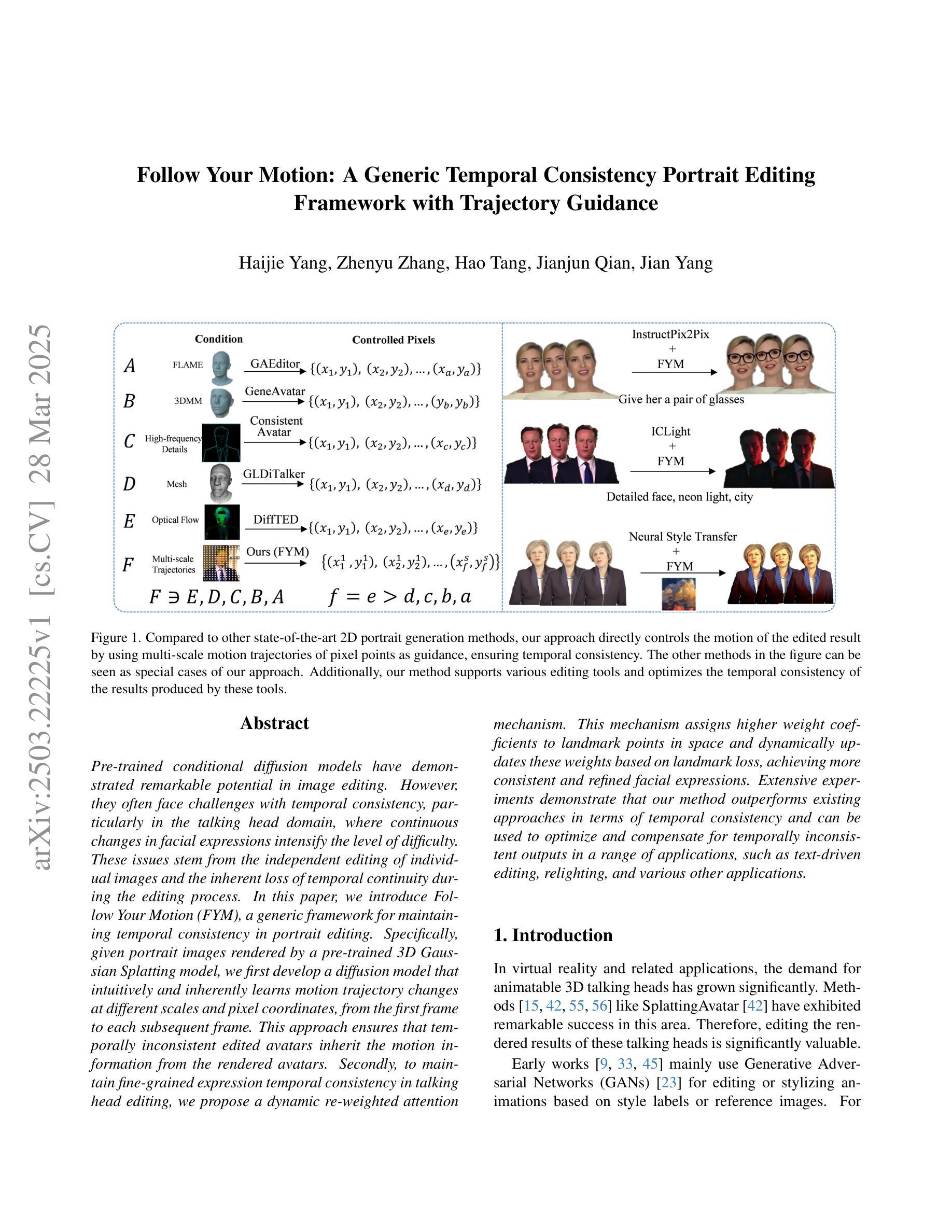
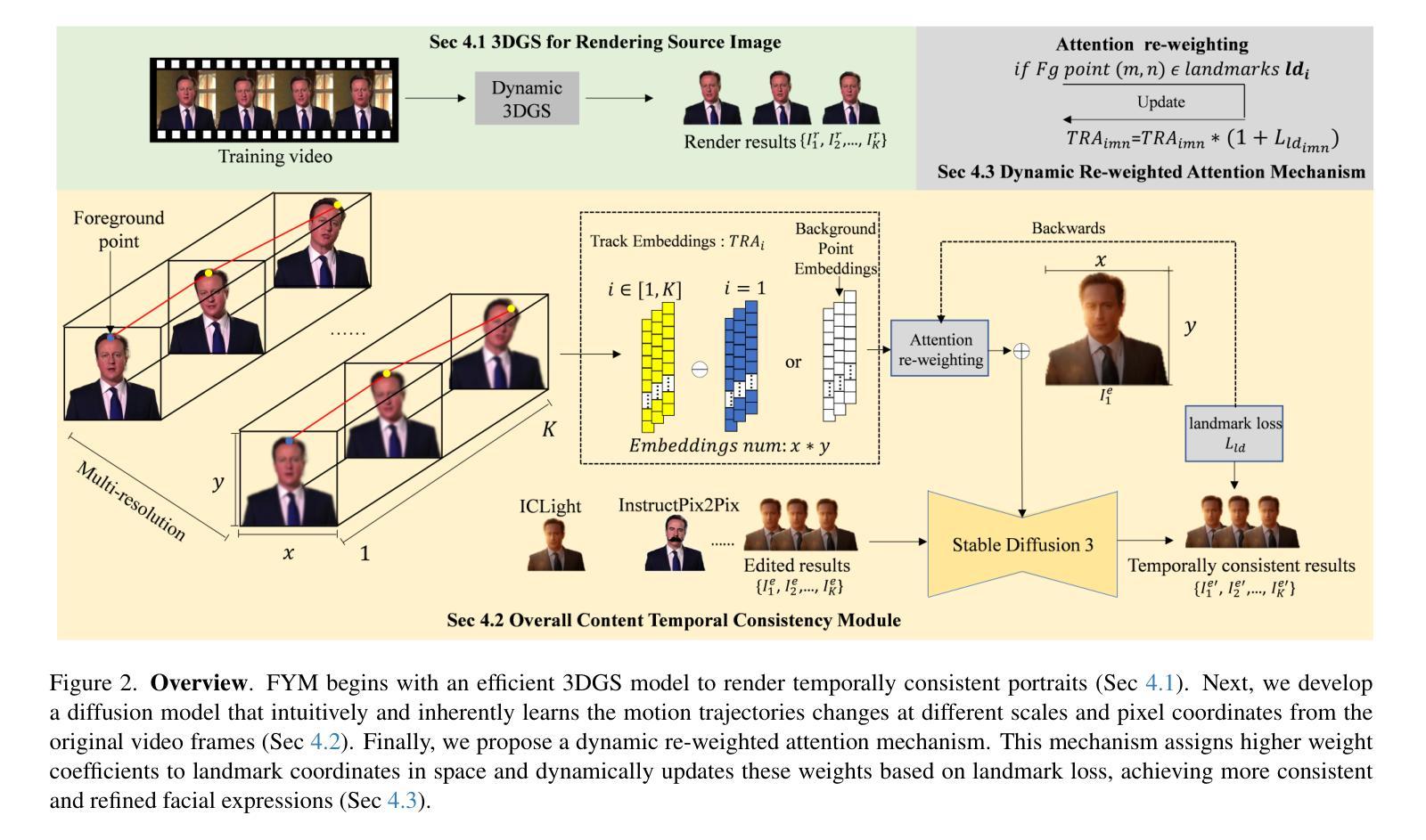
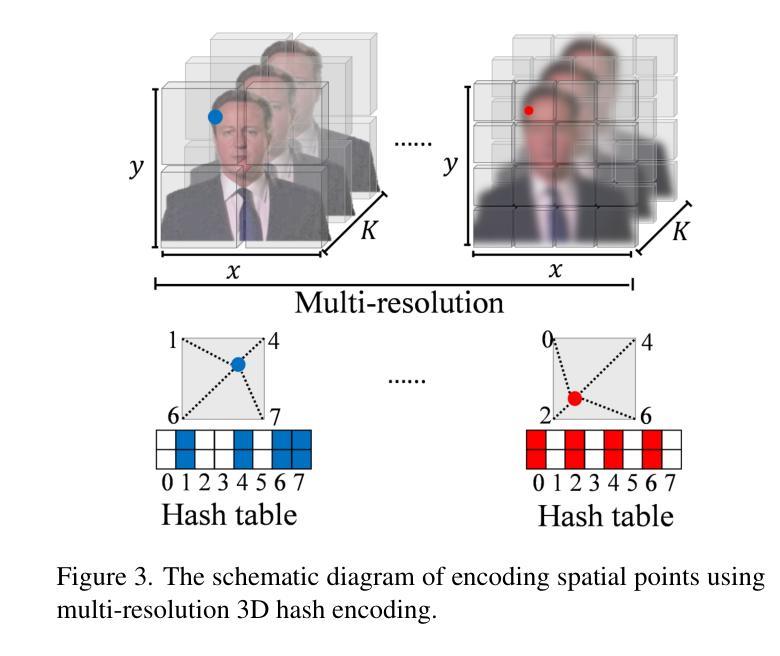
Audio-driven Gesture Generation via Deviation Feature in the Latent Space
Authors:Jiahui Chen, Yang Huan, Runhua Shi, Chanfan Ding, Xiaoqi Mo, Siyu Xiong, Yinong He
Gestures are essential for enhancing co-speech communication, offering visual emphasis and complementing verbal interactions. While prior work has concentrated on point-level motion or fully supervised data-driven methods, we focus on co-speech gestures, advocating for weakly supervised learning and pixel-level motion deviations. We introduce a weakly supervised framework that learns latent representation deviations, tailored for co-speech gesture video generation. Our approach employs a diffusion model to integrate latent motion features, enabling more precise and nuanced gesture representation. By leveraging weakly supervised deviations in latent space, we effectively generate hand gestures and mouth movements, crucial for realistic video production. Experiments show our method significantly improves video quality, surpassing current state-of-the-art techniques.
手势对于增强伴随语音的交流、提供视觉重点以及补充口头互动至关重要。尽管先前的研究主要集中在点级运动或完全监督的数据驱动方法上,但我们专注于伴随语音的手势,主张采用弱监督学习和像素级运动偏差。我们引入了一个弱监督框架,用于学习潜在表示偏差,该框架专为伴随语音的手势视频生成而设计。我们的方法采用扩散模型来整合潜在运动特征,从而实现更精确和细微的手势表示。通过利用潜在空间中的弱监督偏差,我们可以有效地生成对真实视频制作至关重要的手势和口型运动。实验表明,我们的方法显著提高了视频质量,超越了当前最先进的技术。
论文及项目相关链接
PDF 6 pages, 5 figures
Summary
本文重点研究通过微弱监督学习的方式进行语音伴随手势的视频生成。通过引入扩散模型整合潜在运动特征,实现精准且细腻的手势表达。利用潜在空间的微弱监督偏差,有效生成手部和口部动作,对真实视频制作至关重要。实验证明,该方法显著提高视频质量,超越现有技术。
Key Takeaways
- 文中指出手势在伴随语音交流中的重要性,可增强视觉强调和补充语言交互。
- 与先前集中在点状运动或完全监督数据驱动方法的研究不同,该文重点关注伴随语音的手势,提倡微弱监督学习和像素级运动偏差。
- 提出一个微弱监督框架来学习潜在表示的偏差,专门为伴随语音的手势视频生成量身定制。
- 采用扩散模型整合潜在运动特征,实现更精确和细致的手势表示。
- 利用潜在空间的微弱监督偏差有效生成手部和口部动作,这对现实视频制作至关重要。
- 实验结果显示,该方法在视频质量上表现出显著改进,超越了当前最新技术。
点此查看论文截图
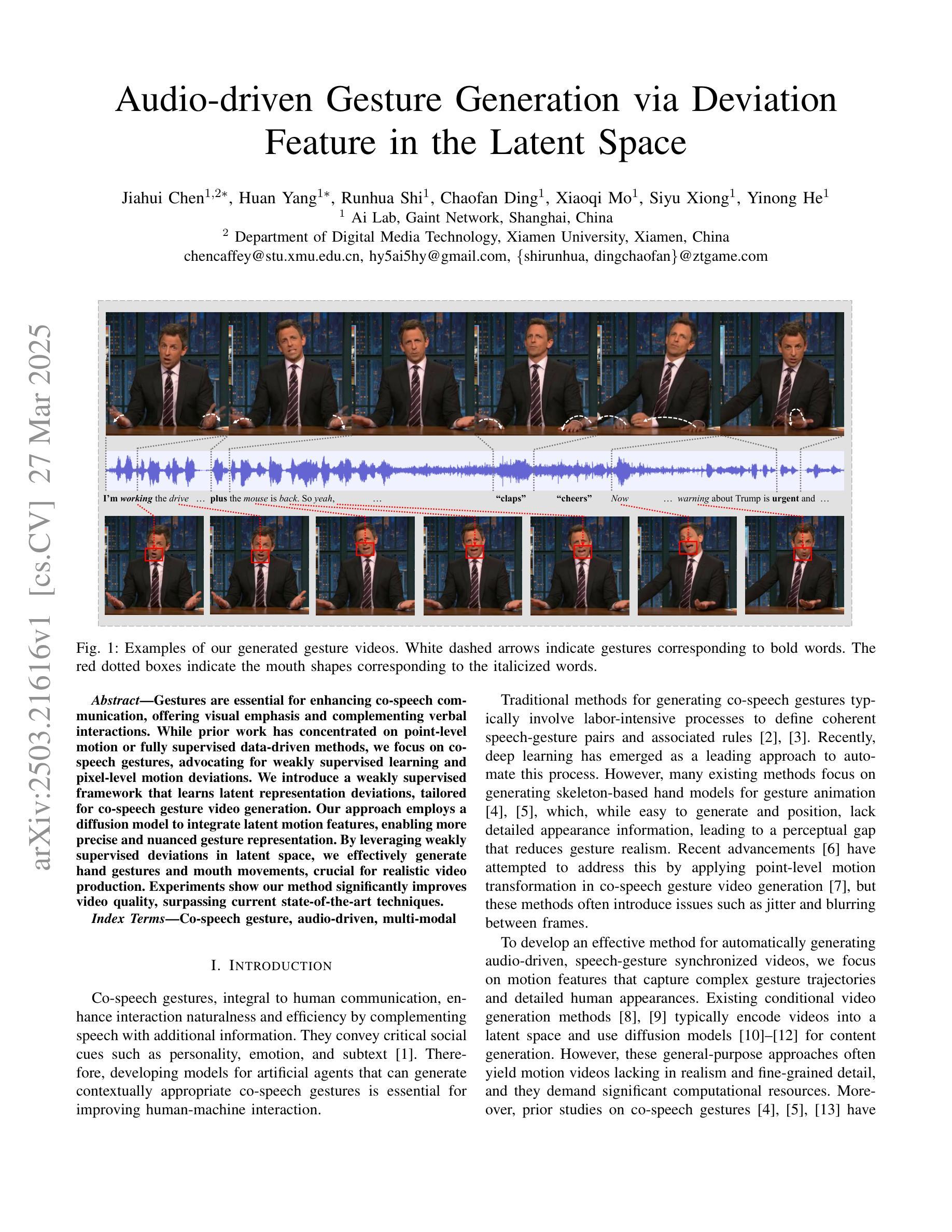
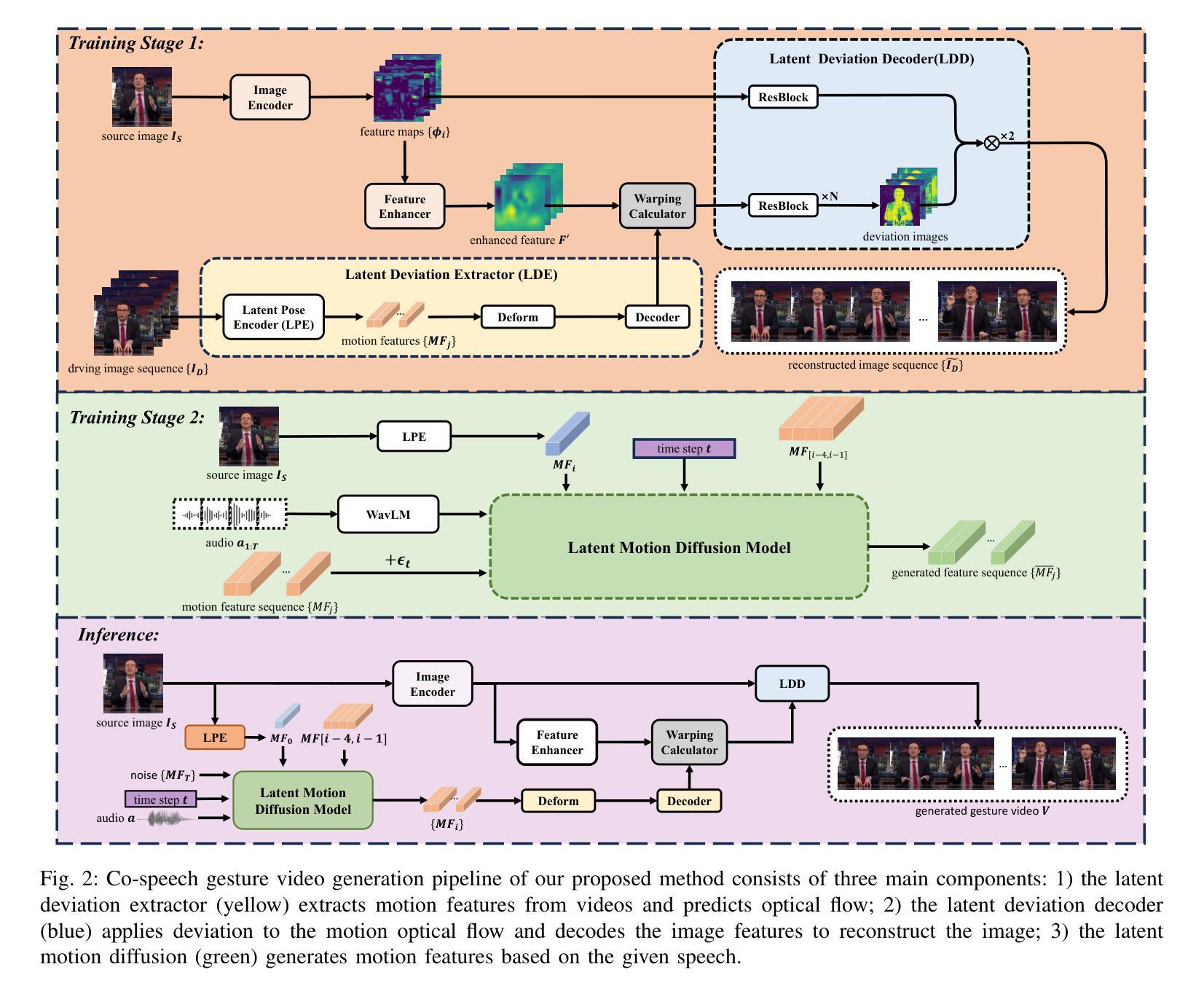
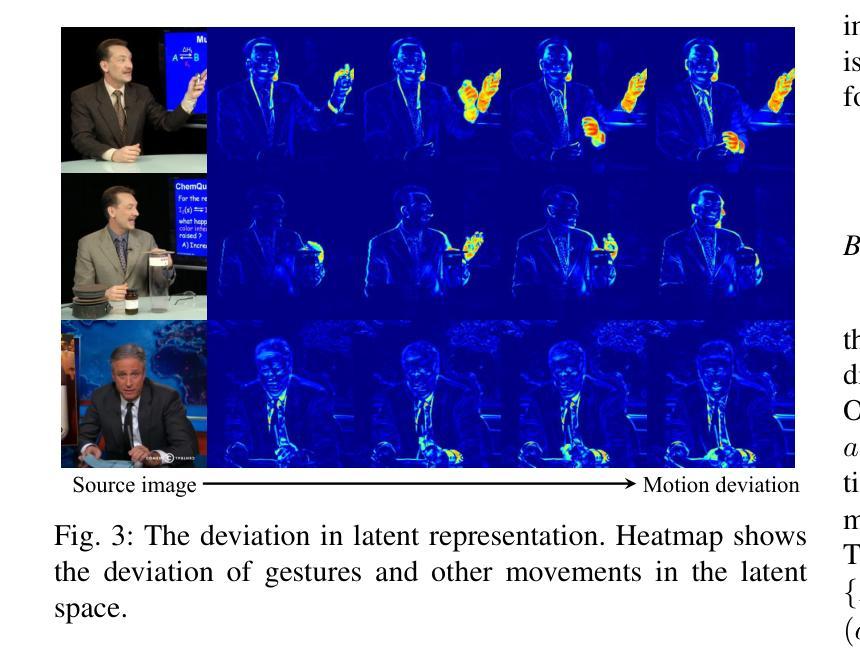
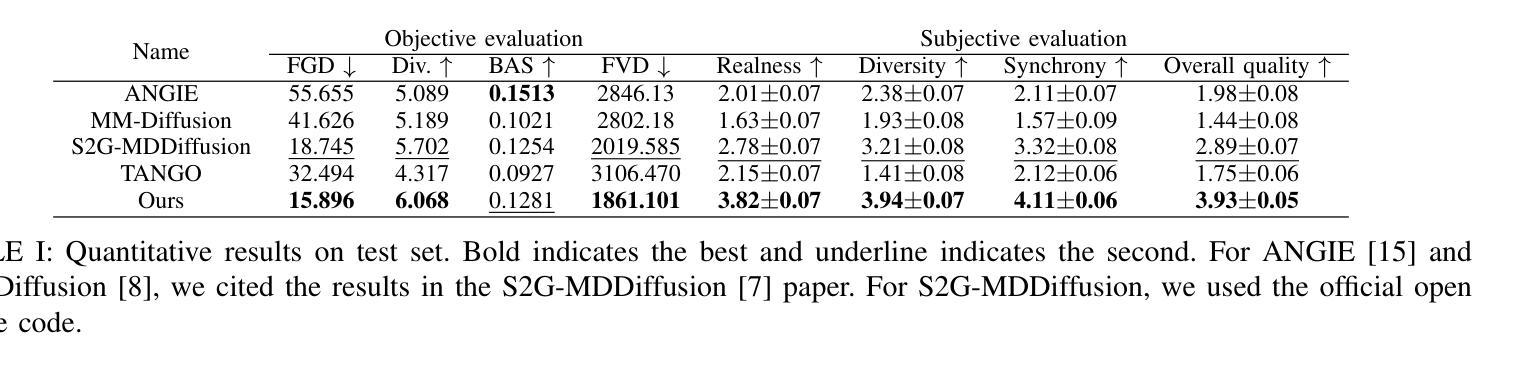
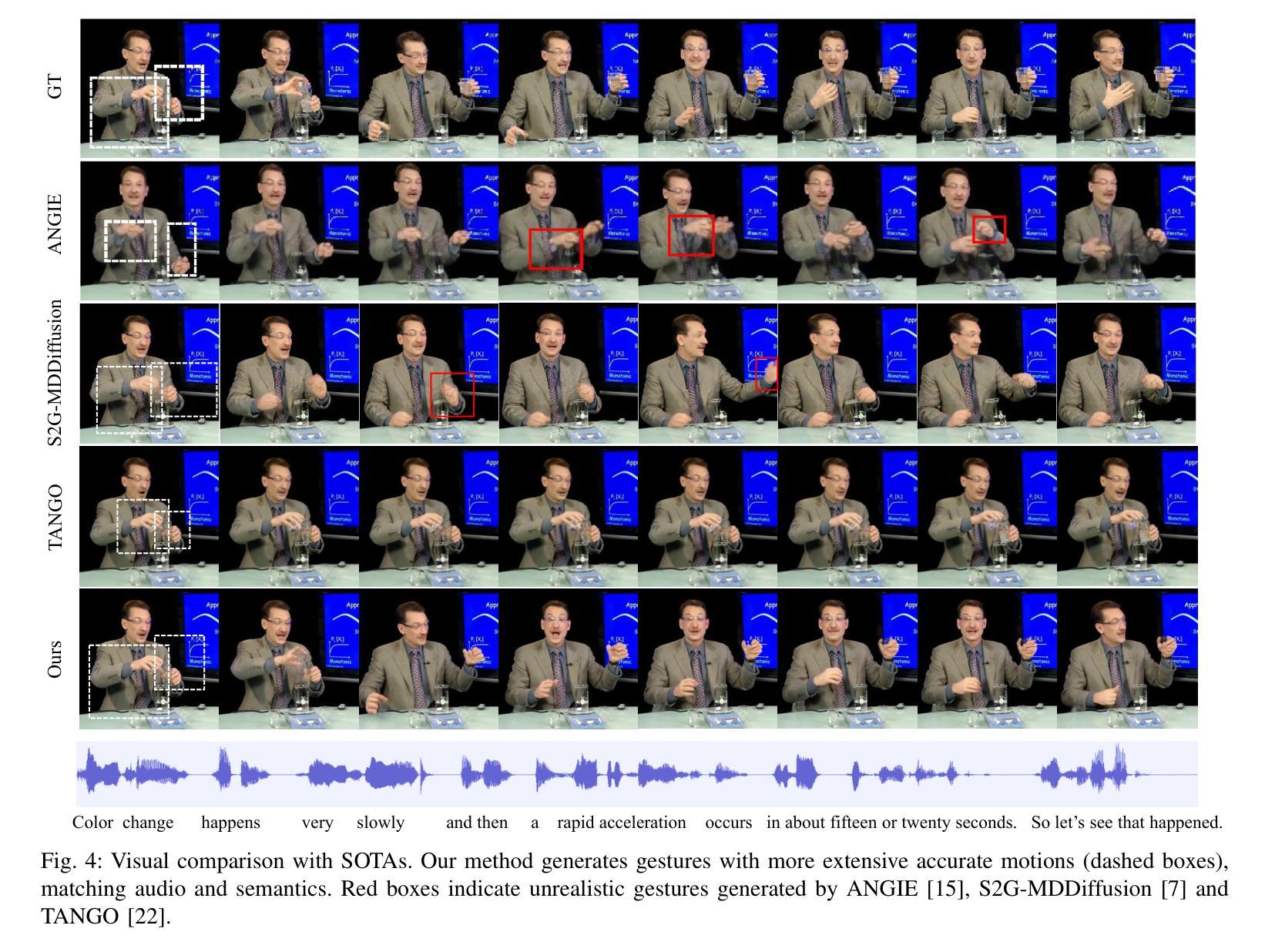
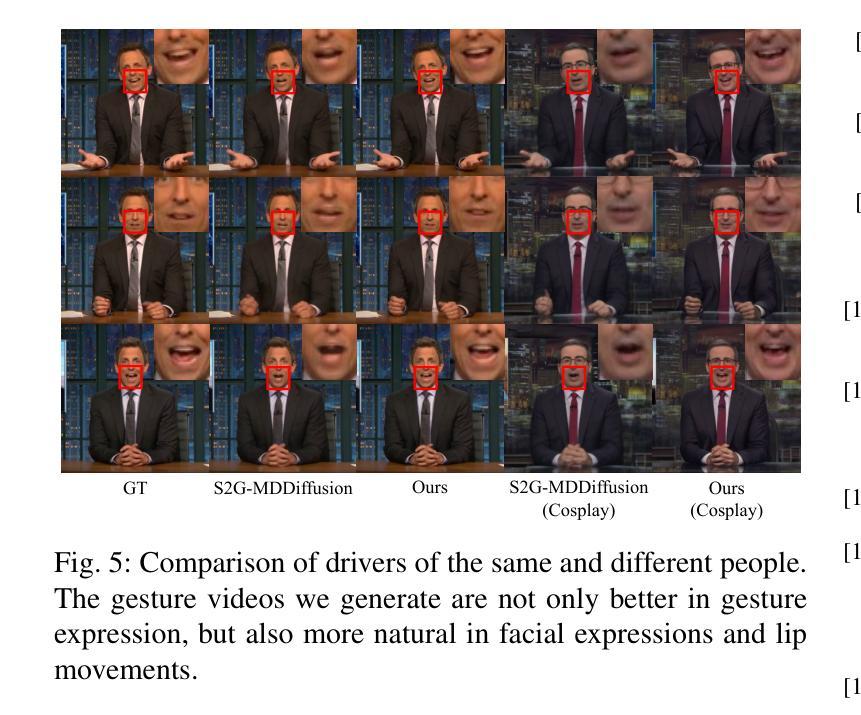

Perceptually Accurate 3D Talking Head Generation: New Definitions, Speech-Mesh Representation, and Evaluation Metrics
Authors:Lee Chae-Yeon, Oh Hyun-Bin, Han EunGi, Kim Sung-Bin, Suekyeong Nam, Tae-Hyun Oh
Recent advancements in speech-driven 3D talking head generation have made significant progress in lip synchronization. However, existing models still struggle to capture the perceptual alignment between varying speech characteristics and corresponding lip movements. In this work, we claim that three criteria – Temporal Synchronization, Lip Readability, and Expressiveness – are crucial for achieving perceptually accurate lip movements. Motivated by our hypothesis that a desirable representation space exists to meet these three criteria, we introduce a speech-mesh synchronized representation that captures intricate correspondences between speech signals and 3D face meshes. We found that our learned representation exhibits desirable characteristics, and we plug it into existing models as a perceptual loss to better align lip movements to the given speech. In addition, we utilize this representation as a perceptual metric and introduce two other physically grounded lip synchronization metrics to assess how well the generated 3D talking heads align with these three criteria. Experiments show that training 3D talking head generation models with our perceptual loss significantly improve all three aspects of perceptually accurate lip synchronization. Codes and datasets are available at https://perceptual-3d-talking-head.github.io/.
近期语音驱动的3D动态头部生成技术的进展在唇同步方面取得了重大突破。然而,现有模型在捕捉不同语音特征和相应唇部运动之间的感知对齐方面仍存在困难。在这项工作中,我们认为时间同步、唇可读性和表现力这三个标准是实现感知准确唇部运动的关键。受存在一个符合这三个标准的理想表示空间的假设的驱动,我们引入了一种语音网格同步表示,该表示捕捉了语音信号和3D面部网格之间的精细对应关系。我们发现,我们学到的表示具有理想的特性,我们将其插入现有模型作为感知损失,以更好地将唇部运动与给定语音对齐。此外,我们利用这种表示作为感知度量,并引入其他两个基于物理的唇同步度量标准,以评估生成的3D动态头部与这三个标准的对齐程度。实验表明,使用我们的感知损失训练3D动态头部生成模型可以显著提高这三个方面的感知准确唇同步。相关代码和数据集可在https://perceptual-3d-talking-head.github.io/找到。
论文及项目相关链接
PDF CVPR 2025. Project page: https://perceptual-3d-talking-head.github.io/
Summary
本文探讨了近期语音驱动的三维动态头部生成技术的进展,特别是在唇部同步方面的成果。文章指出,现有模型在捕捉不同语音特征与唇部运动的感知对齐方面仍存在困难。为此,文章提出了三大关键标准——时间同步、唇可读性和表现力,并引入了一种语音网格同步表示法,该表示法能够捕捉语音信号与三维面部网格之间的精细对应关系。通过将这种表示法作为感知损失插入现有模型中,可以更好地对齐唇部运动与给定语音。此外,文章还利用这种表示法作为感知指标,并引入另外两个基于物理的唇部同步指标来评估生成的3D动态头部与三大标准的对齐程度。实验表明,使用本文的感知损失训练3D动态头部生成模型,可显著提高唇部同步的感知准确性。
Key Takeaways
- 现有模型在捕捉语音特征与唇部运动感知对齐方面存在困难。
- 提出三大关键标准:时间同步、唇可读性和表现力。
- 引入一种语音网格同步表示法,捕捉语音信号与三维面部网格的对应关系。
- 将感知损失插入现有模型,提高唇部运动与语音的对齐程度。
- 利用感知指标评估生成的3D动态头部的质量。
- 引入两个基于物理的唇部同步指标来全面评估生成结果。
点此查看论文截图
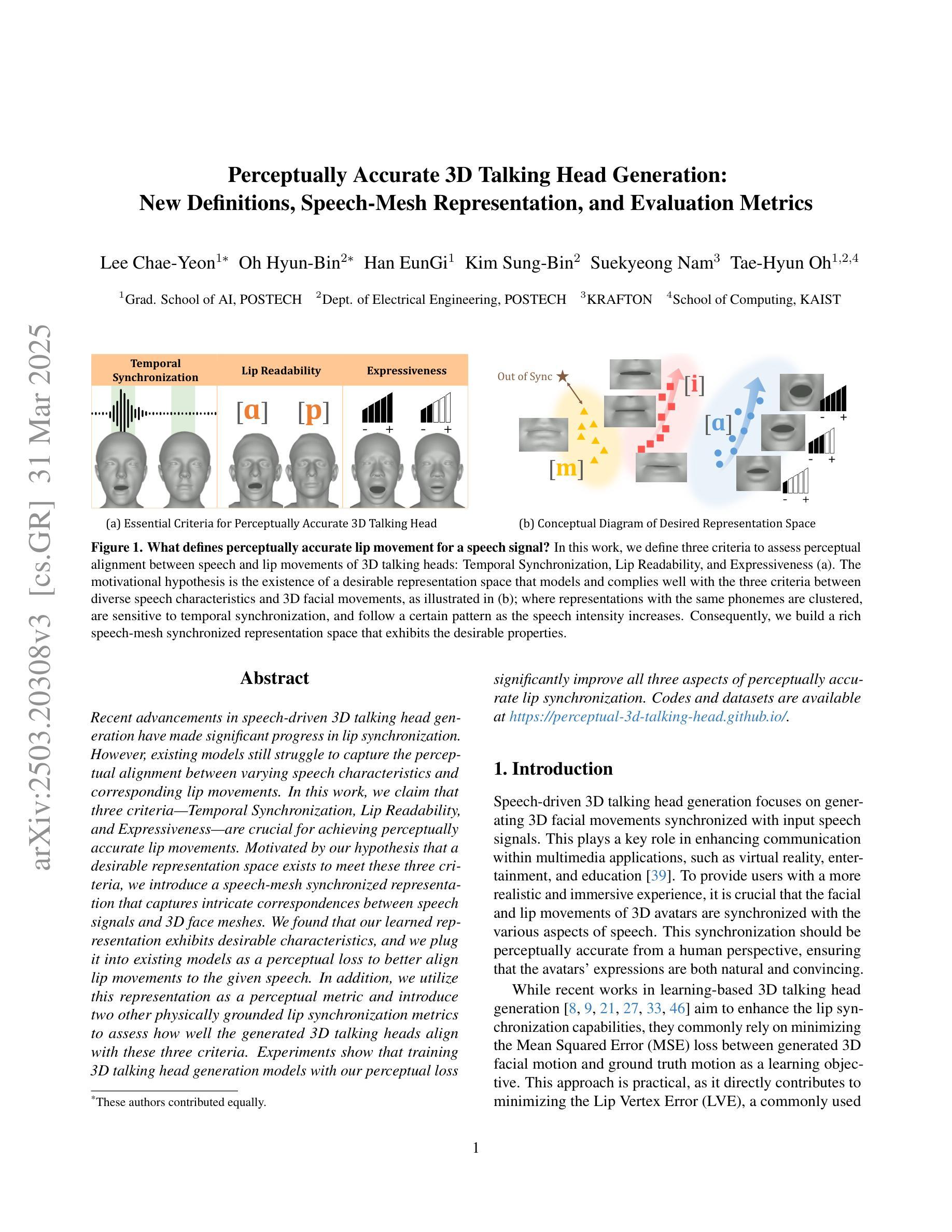
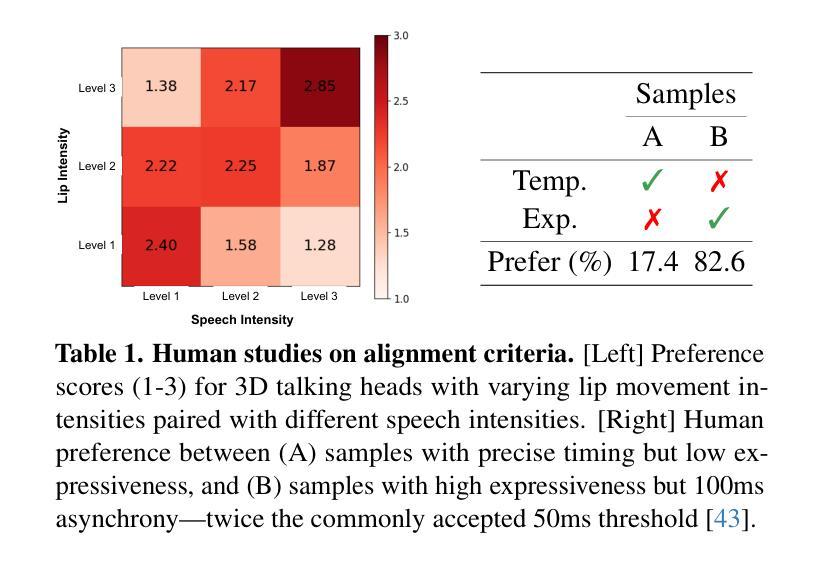
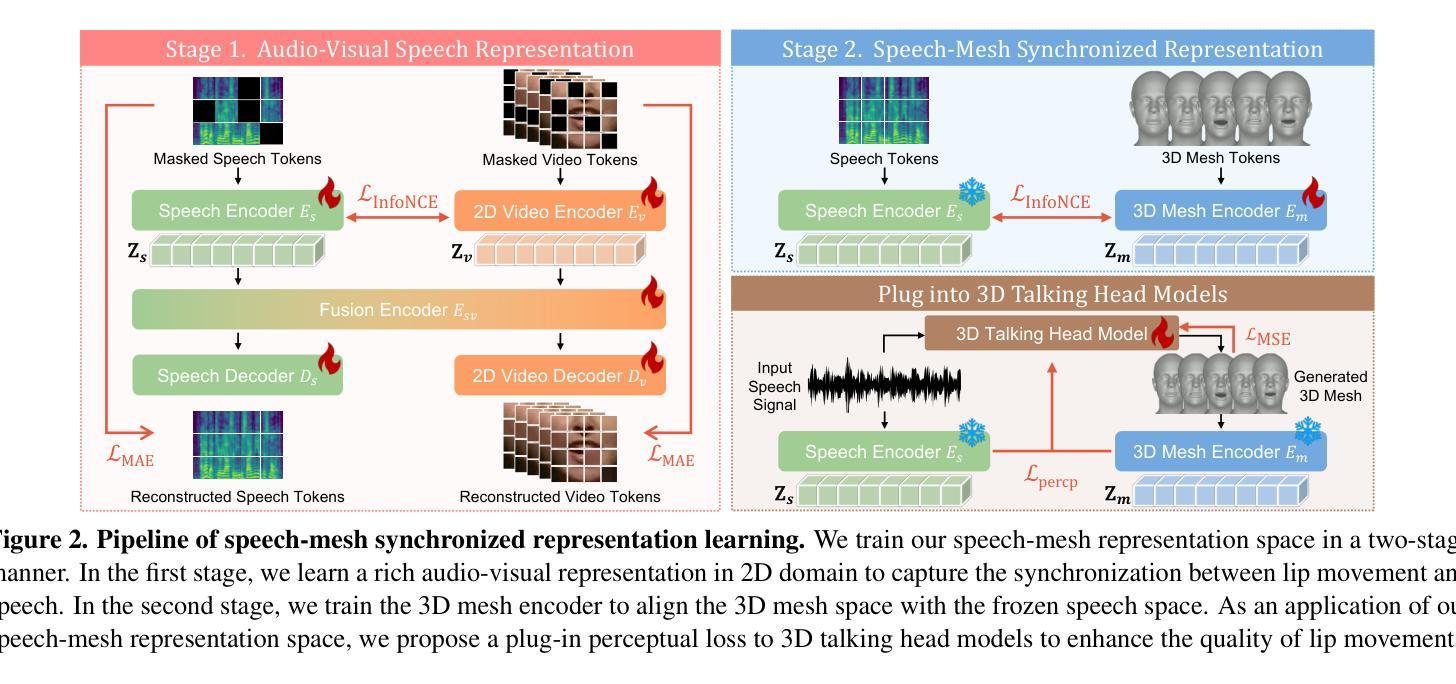
MagicDistillation: Weak-to-Strong Video Distillation for Large-Scale Few-Step Synthesis
Authors:Shitong Shao, Hongwei Yi, Hanzhong Guo, Tian Ye, Daquan Zhou, Michael Lingelbach, Zhiqiang Xu, Zeke Xie
Recently, open-source video diffusion models (VDMs), such as WanX, Magic141 and HunyuanVideo, have been scaled to over 10 billion parameters. These large-scale VDMs have demonstrated significant improvements over smaller-scale VDMs across multiple dimensions, including enhanced visual quality and more natural motion dynamics. However, these models face two major limitations: (1) High inference overhead: Large-scale VDMs require approximately 10 minutes to synthesize a 28-step video on a single H100 GPU. (2) Limited in portrait video synthesis: Models like WanX-I2V and HunyuanVideo-I2V often produce unnatural facial expressions and movements in portrait videos. To address these challenges, we propose MagicDistillation, a novel framework designed to reduce inference overhead while ensuring the generalization of VDMs for portrait video synthesis. Specifically, we primarily use sufficiently high-quality talking video to fine-tune Magic141, which is dedicated to portrait video synthesis. We then employ LoRA to effectively and efficiently fine-tune the fake DiT within the step distillation framework known as distribution matching distillation (DMD). Following this, we apply weak-to-strong (W2S) distribution matching and minimize the discrepancy between the fake data distribution and the ground truth distribution, thereby improving the visual fidelity and motion dynamics of the synthesized videos. Experimental results on portrait video synthesis demonstrate the effectiveness of MagicDistillation, as our method surpasses Euler, LCM, and DMD baselines in both FID/FVD metrics and VBench. Moreover, MagicDistillation, requiring only 4 steps, also outperforms WanX-I2V (14B) and HunyuanVideo-I2V (13B) on visualization and VBench. Our project page is https://magicdistillation.github.io/MagicDistillation/.
最近,开源视频扩散模型(VDMs),如WanX、Magic141和HunyuanVideo,已被扩展到超过10亿参数。这些大规模VDMs在多个维度上相对于小规模VDMs表现出了显著改进,包括增强的视觉质量和更自然的运动动态。然而,这些模型面临两大局限:(1)推理开销高:大规模VDMs在单个H100 GPU上合成一个28步的视频大约需要10分钟。(2)肖像视频合成受限:如WanX-I2V和HunyuanVideo-I2V等模型在肖像视频中经常产生不自然的面部表情和动作。为了解决这些挑战,我们提出了MagicDistillation,这是一个新颖框架,旨在降低推理开销,同时确保VDM在肖像视频合成的泛化能力。具体来说,我们主要使用高质量谈话视频对Magic141进行微调,Magic141是专门用于肖像视频合成的。然后,我们采用LoRA在称为分布匹配蒸馏(DMD)的步骤蒸馏框架内有效地对假DiT进行微调。接下来,我们应用弱到强(W2S)分布匹配,并最小化假数据分布与真实数据分布之间的差异,从而提高合成视频的视觉保真度和运动动态。肖像视频合成的实验结果证明了MagicDistillation的有效性,我们的方法在FID/FVD指标和VBench上都超越了Euler、LCM和DMD基线。此外,MagicDistillation仅需4步,还在可视化和VBench上超越了WanX-I2V(14B)和HunyuanVideo-I2V(13B)。我们的项目页面是https://magicdistillation.github.io/MagicDistillation/。
论文及项目相关链接
Summary
大型开源视频扩散模型(VDMs)如WanX、Magic141和HunyuanVideo已扩展至超过十亿参数,它们在视频质量及运动动态方面表现优越,但存在推理开销大及肖像视频合成受限等缺点。为应对这些挑战,提出MagicDistillation框架,通过高质量视频谈话数据对Magic141进行微调,结合LoRA及分布匹配蒸馏(DMD)等方法,改善合成视频的视觉逼真度和运动动态。实验结果显示,MagicDistillation在肖像视频合成上表现优于Euler、LCM和DMD,仅需四步即能超越WanX-I2V和HunyuanVideo-I2V。
Key Takeaways
- 大型开源视频扩散模型(VDMs)已在视觉质量和自然运动方面取得显著改进。
- 这些模型面临高推理开销和肖像视频合成受限的挑战。
- MagicDistillation框架旨在减少推理开销并确保VDM在肖像视频合成的泛化能力。
- MagicDistillation使用高质量视频谈话数据对Magic141进行微调。
- 结合LoRA和DMD,MagicDistillation提高了合成视频的视觉逼真度和运动动态。
- 实验结果表明,MagicDistillation在肖像视频合成上优于其他方法。
点此查看论文截图
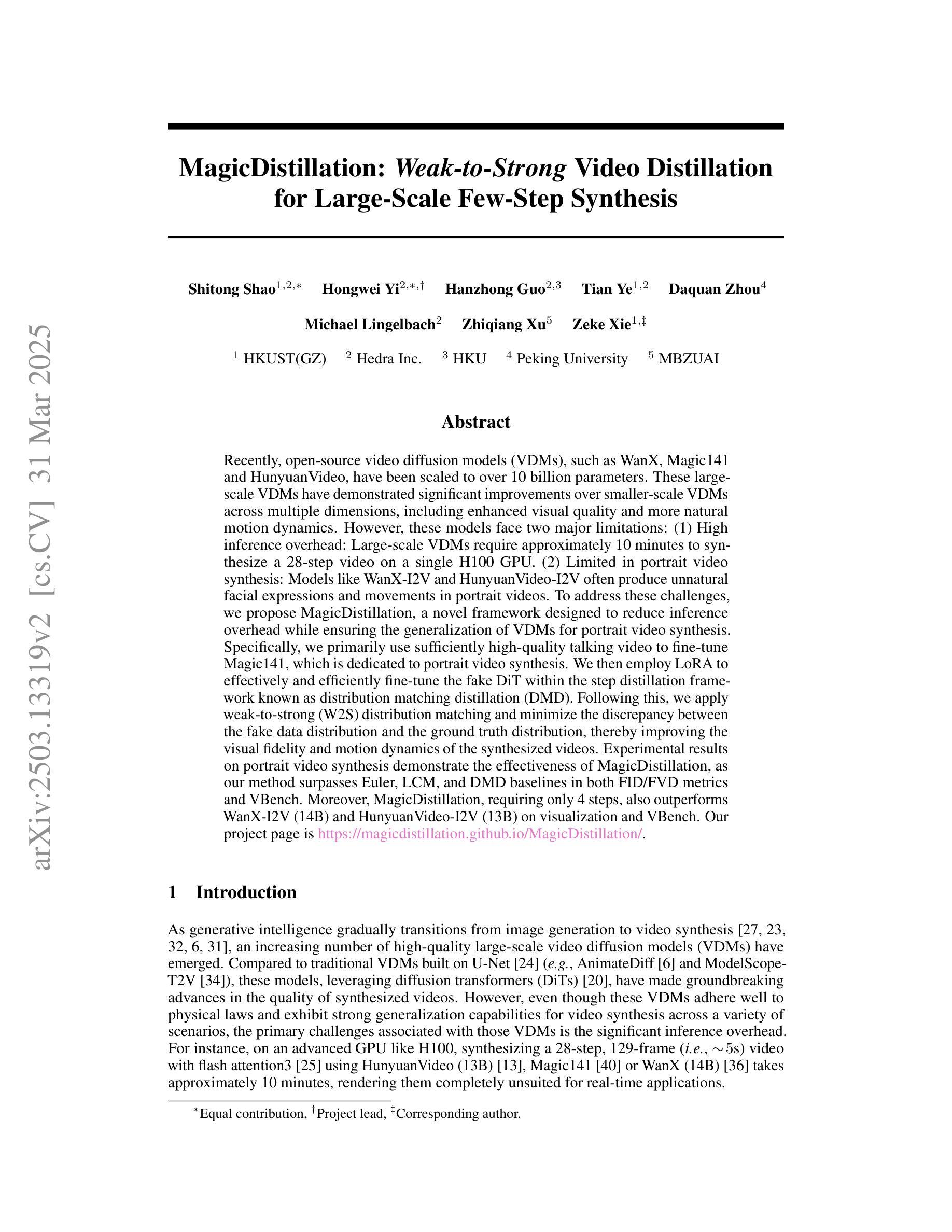
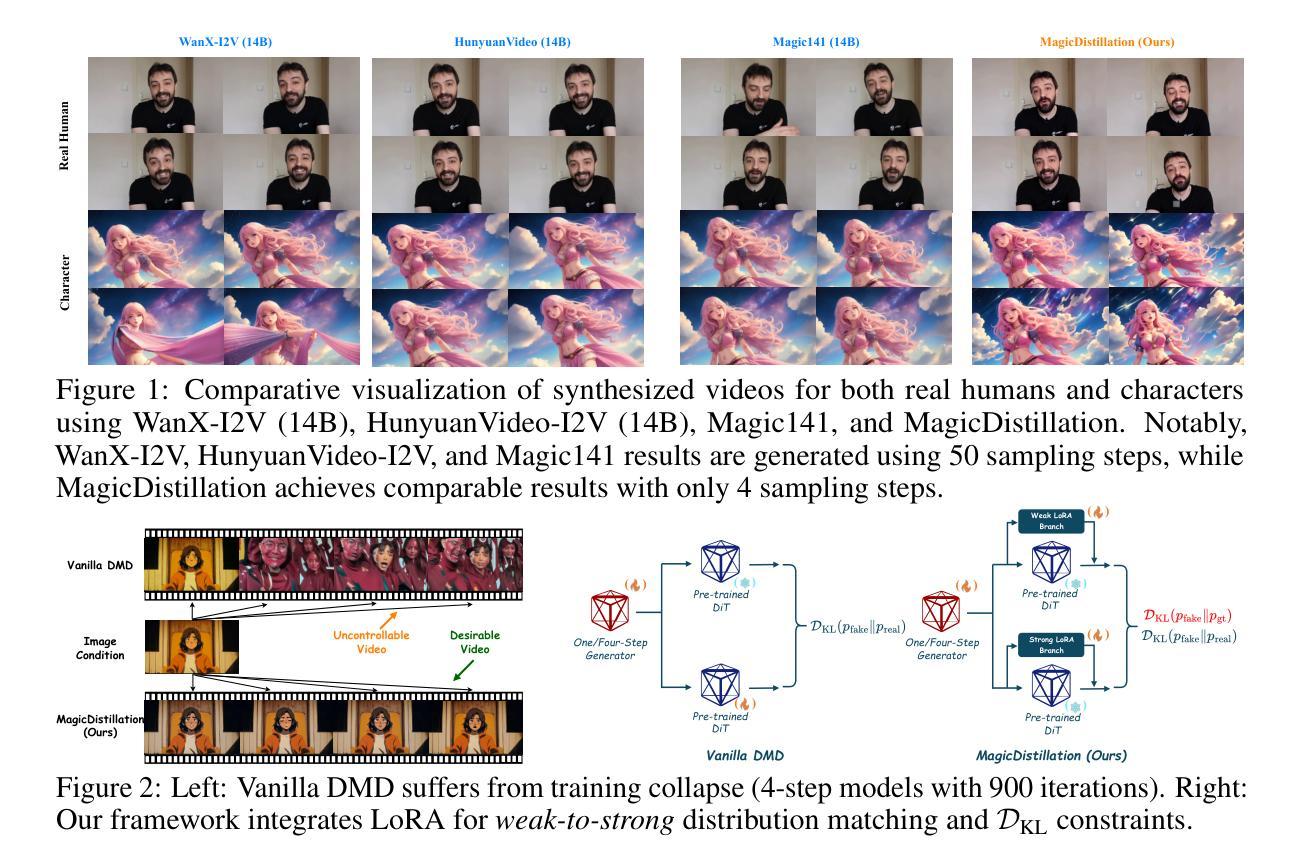
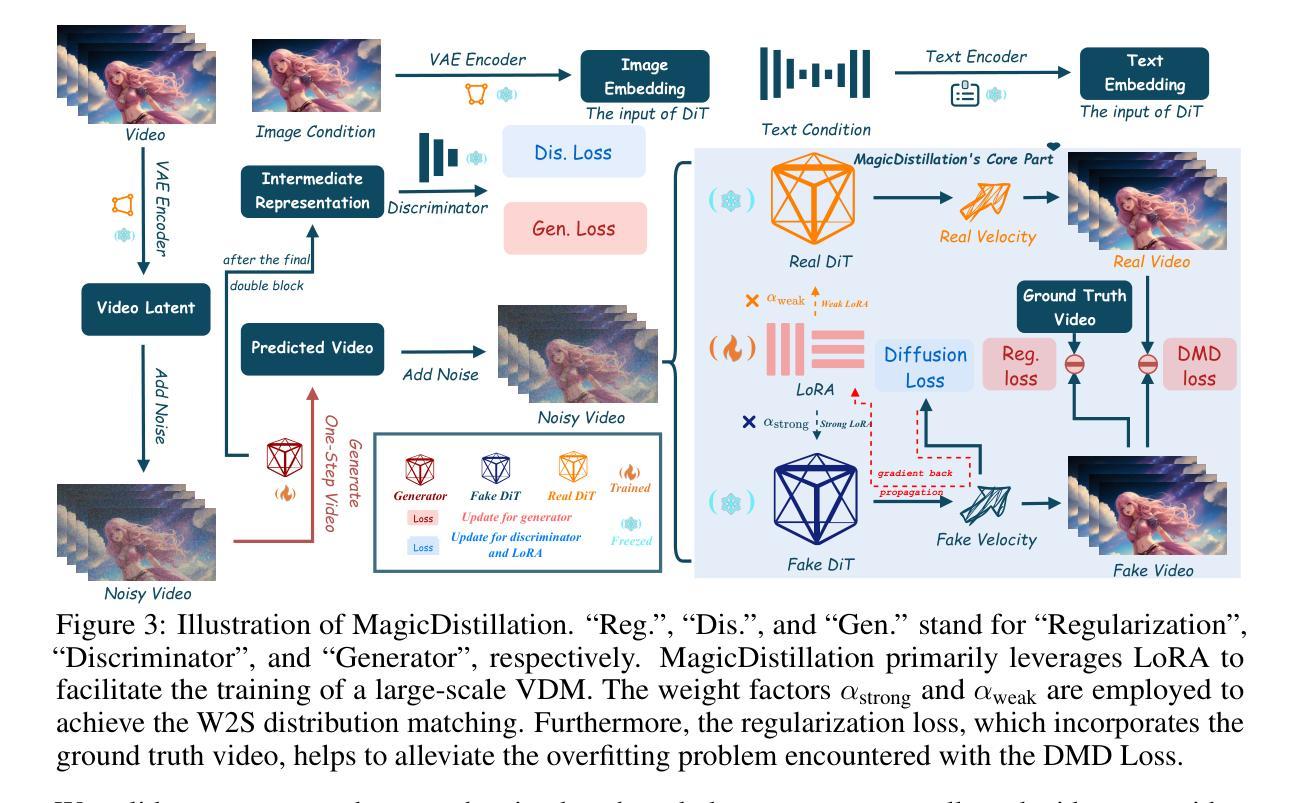
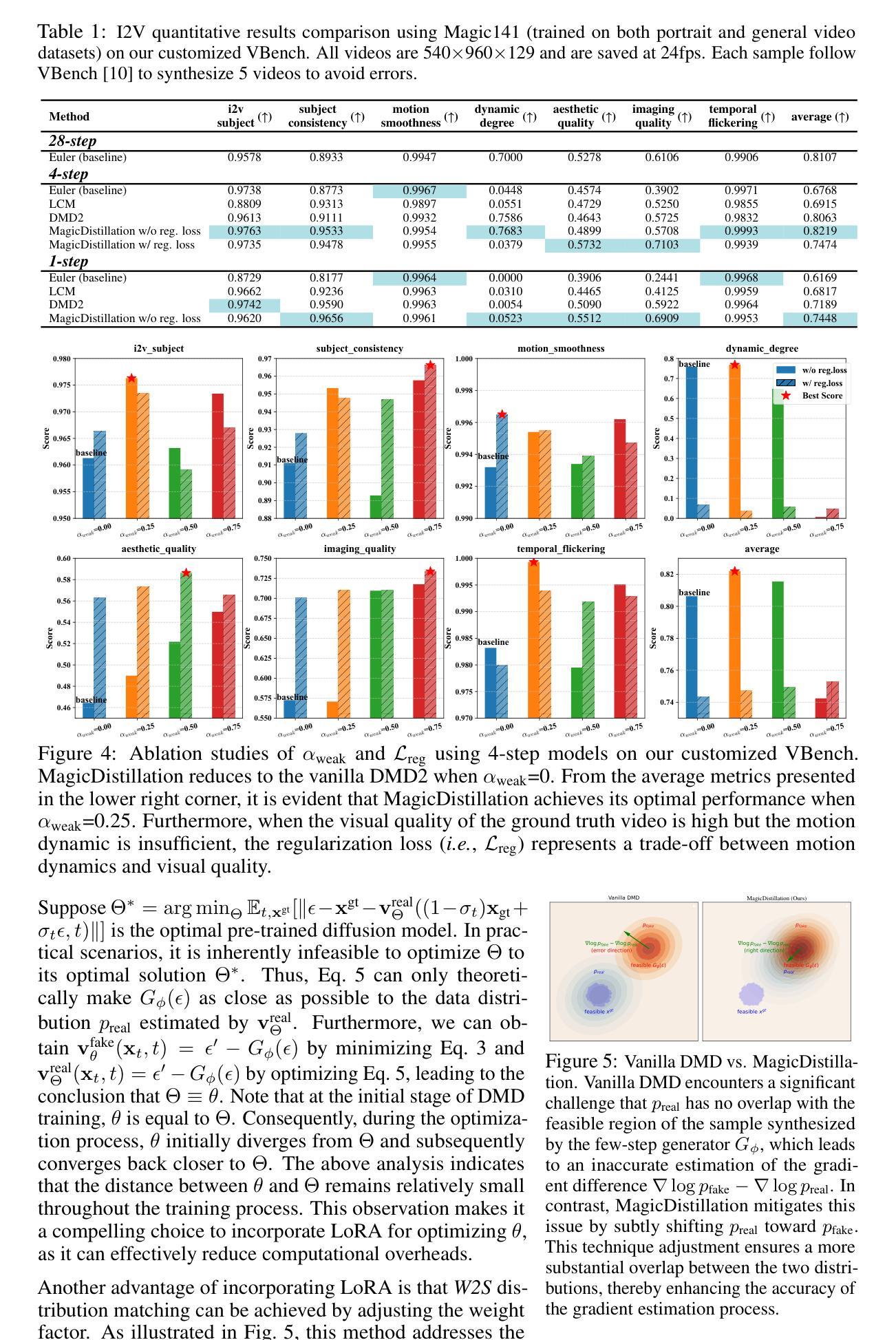
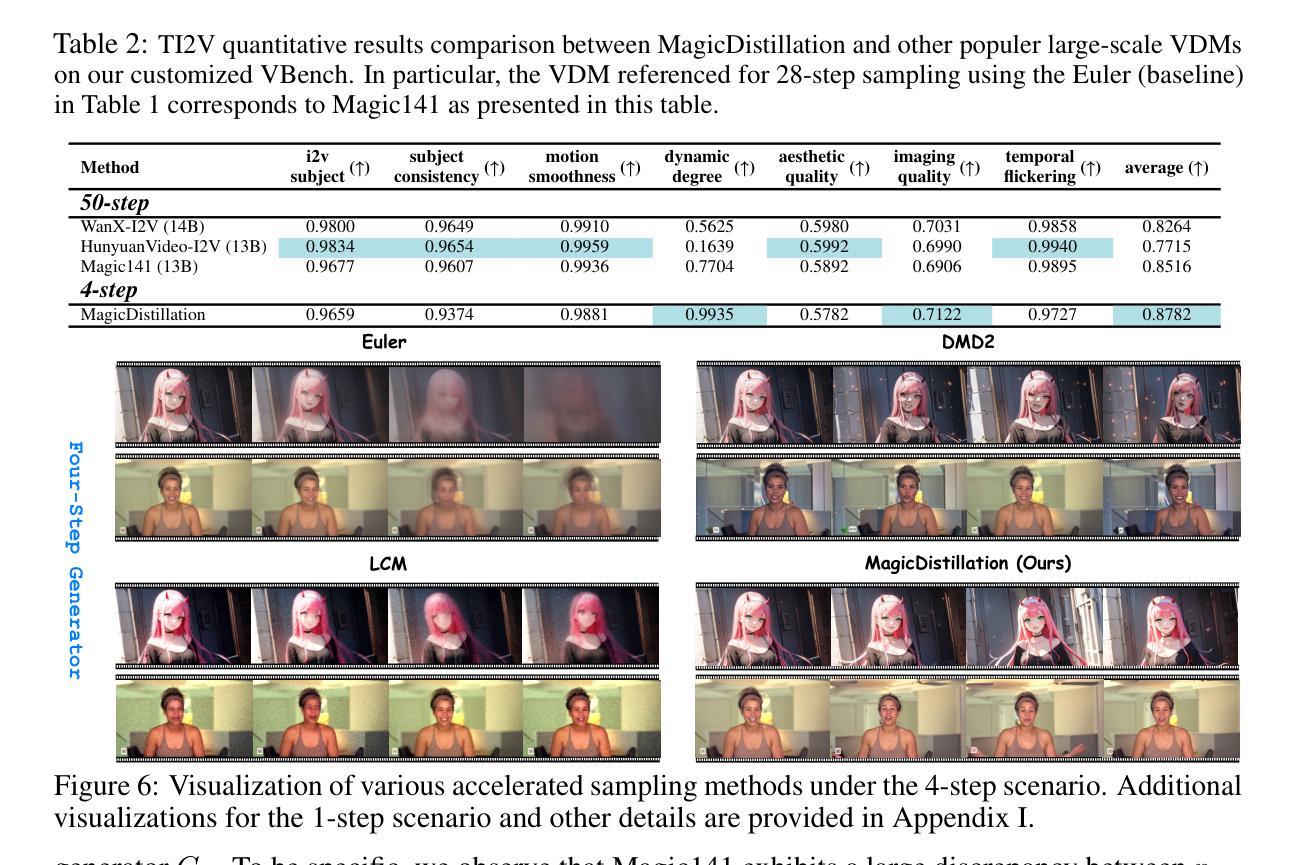
GMTalker: Gaussian Mixture-based Audio-Driven Emotional Talking Video Portraits
Authors:Yibo Xia, Lizhen Wang, Xiang Deng, Xiaoyan Luo, Yunhong Wang, Yebin Liu
Synthesizing high-fidelity and emotion-controllable talking video portraits, with audio-lip sync, vivid expressions, realistic head poses, and eye blinks, has been an important and challenging task in recent years. Most existing methods suffer in achieving personalized and precise emotion control, smooth transitions between different emotion states, and the generation of diverse motions. To tackle these challenges, we present GMTalker, a Gaussian mixture-based emotional talking portraits generation framework. Specifically, we propose a Gaussian mixture-based expression generator that can construct a continuous and disentangled latent space, achieving more flexible emotion manipulation. Furthermore, we introduce a normalizing flow-based motion generator pretrained on a large dataset with a wide-range motion to generate diverse head poses, blinks, and eyeball movements. Finally, we propose a personalized emotion-guided head generator with an emotion mapping network that can synthesize high-fidelity and faithful emotional video portraits. Both quantitative and qualitative experiments demonstrate our method outperforms previous methods in image quality, photo-realism, emotion accuracy, and motion diversity.
近年来,合成高保真和情绪可控的说话视频肖像已经成为一项重要且具有挑战性的任务,这些视频需要具有音频-唇部同步、生动的表情、真实的头部姿势和眨眼等特征。大多数现有方法在实现个性化、精确的情绪控制、不同情绪状态之间的平滑过渡以及生成各种动作方面存在困难。为了应对这些挑战,我们提出了GMTalker,这是一个基于高斯混合的情绪交谈肖像生成框架。具体来说,我们提出了一个基于高斯混合的表情生成器,能够构建一个连续且分离的潜在空间,实现更灵活的情绪操控。此外,我们还引入了一个基于归一化流的运动生成器,该生成器在包含大范围运动的大型数据集上进行预训练,以生成各种头部姿势、眨眼和眼球运动。最后,我们提出了一个个性化的情绪引导头部生成器,它包含一个情感映射网络,能够合成高保真和真实的情绪视频肖像。定量和定性实验均表明,我们的方法在图像质量、逼真度、情绪准确性和运动多样性方面优于以前的方法。
论文及项目相关链接
PDF Project page: https://bob35buaa.github.io/GMTalker. This work has been submitted to the IEEE journal for possible publication
Summary
本文介绍了一种基于高斯混合的情感对话肖像生成框架GMTalker,用于合成高保真、情感可控的说话视频肖像。该框架通过基于高斯混合的表情生成器构建连续且解耦的潜在空间,实现更灵活的情感操控。同时,引入基于归一化流的运动生成器,在大量数据预训练的基础上生成多样化的头部姿态、眨眼和眼球运动。此外,还提出了个性化情感引导的头部生成器,通过情感映射网络合成高保真和情感真实的视频肖像。实验表明,该方法在图像质量、真实感、情感准确性和运动多样性方面均优于先前的方法。
Key Takeaways
- GMTalker是一个基于高斯混合的情感对话肖像生成框架,旨在合成高保真、情感可控的说话视频肖像。
- 提出了基于高斯混合的表情生成器,构建连续且解耦的潜在空间,实现更灵活的情感操控。
- 引入基于归一化流的运动生成器,可以生成多样化的头部姿态、眨眼和眼球运动。
- 提出了个性化情感引导的头部生成器,通过情感映射网络合成真实且高保真的视频肖像。
- 该方法在图像质量、真实感、情感准确性和运动多样性方面表现优异,优于先前的方法。
- GMTalker框架具有广泛的应用前景,可以用于电影特效、游戏开发、虚拟偶像等领域。
点此查看论文截图
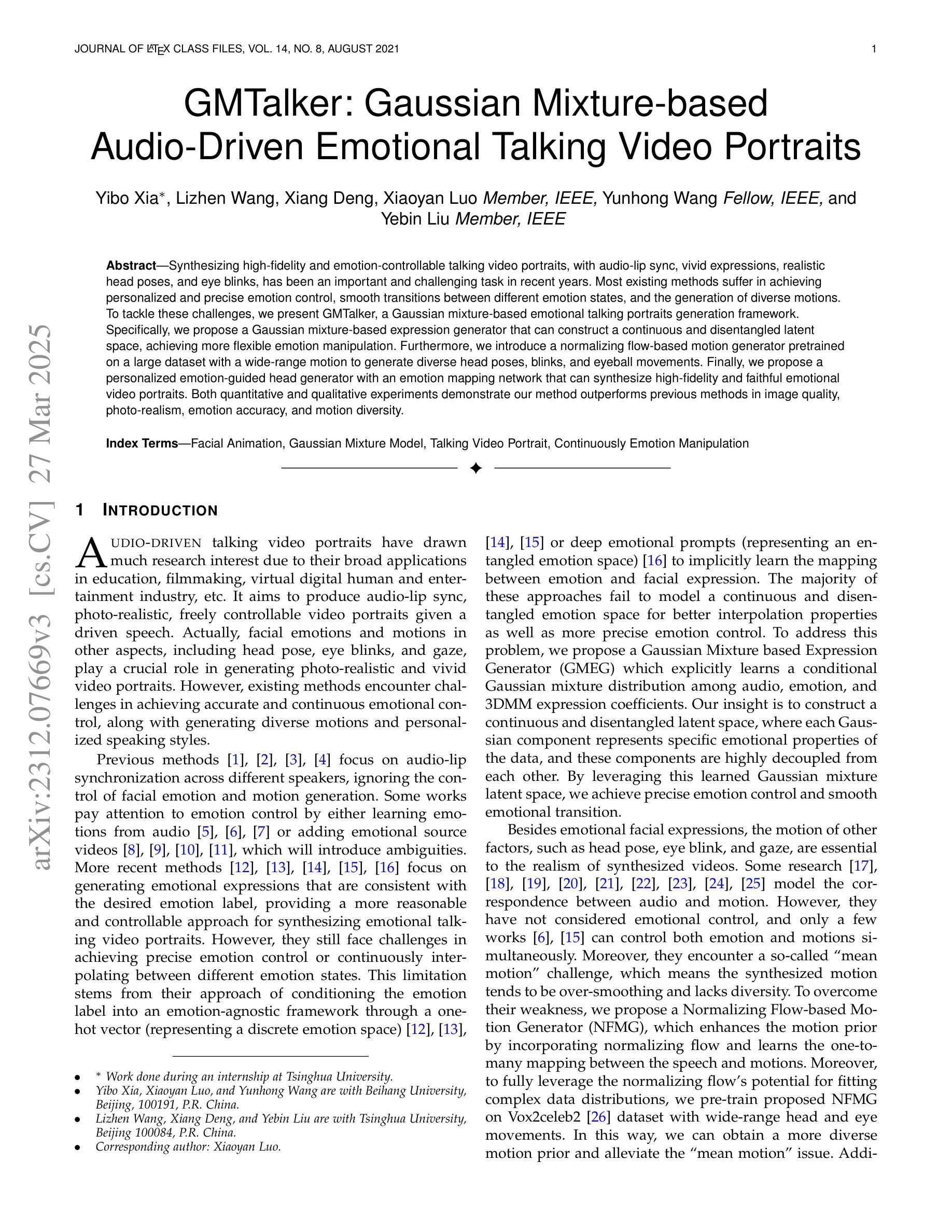
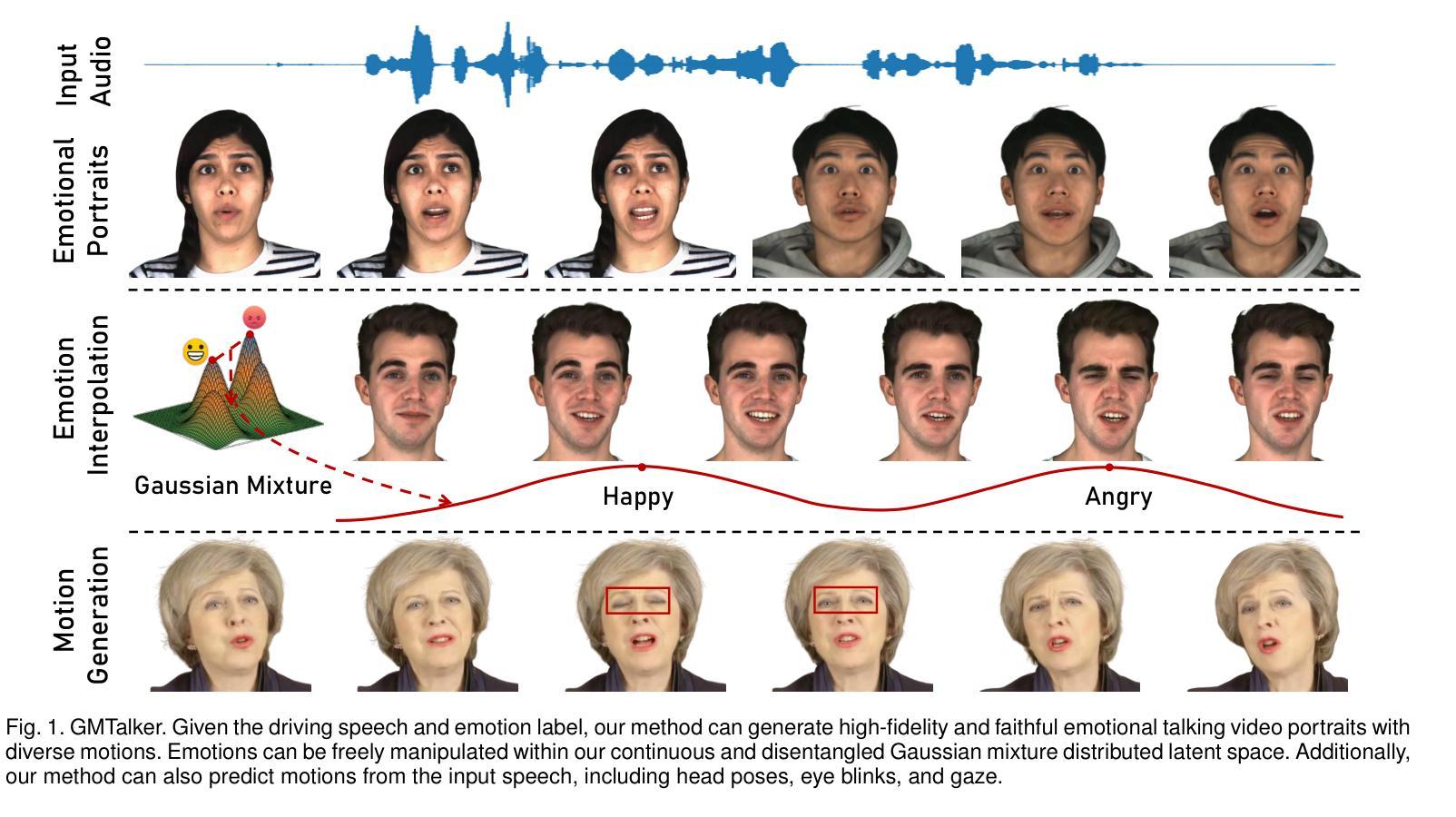
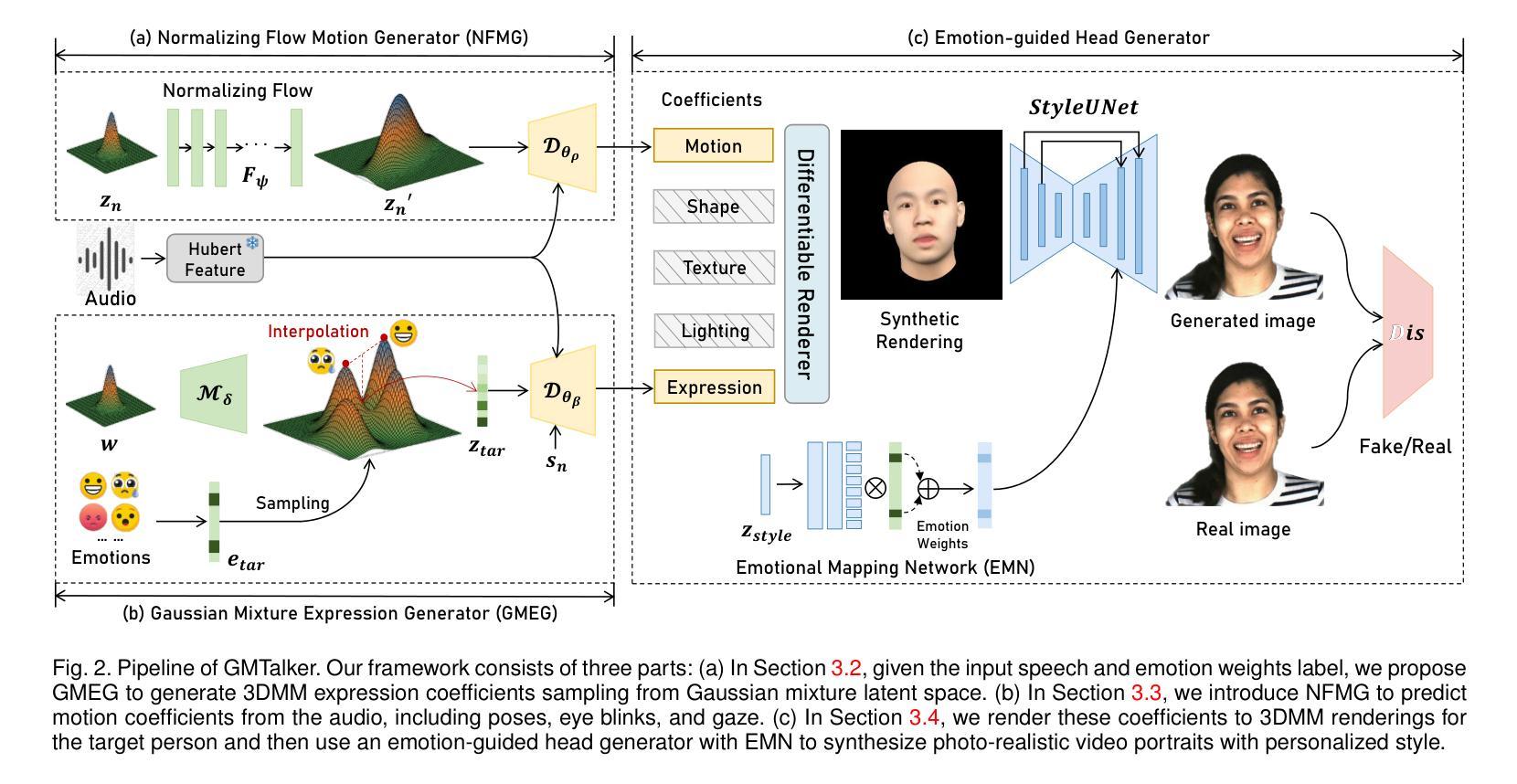
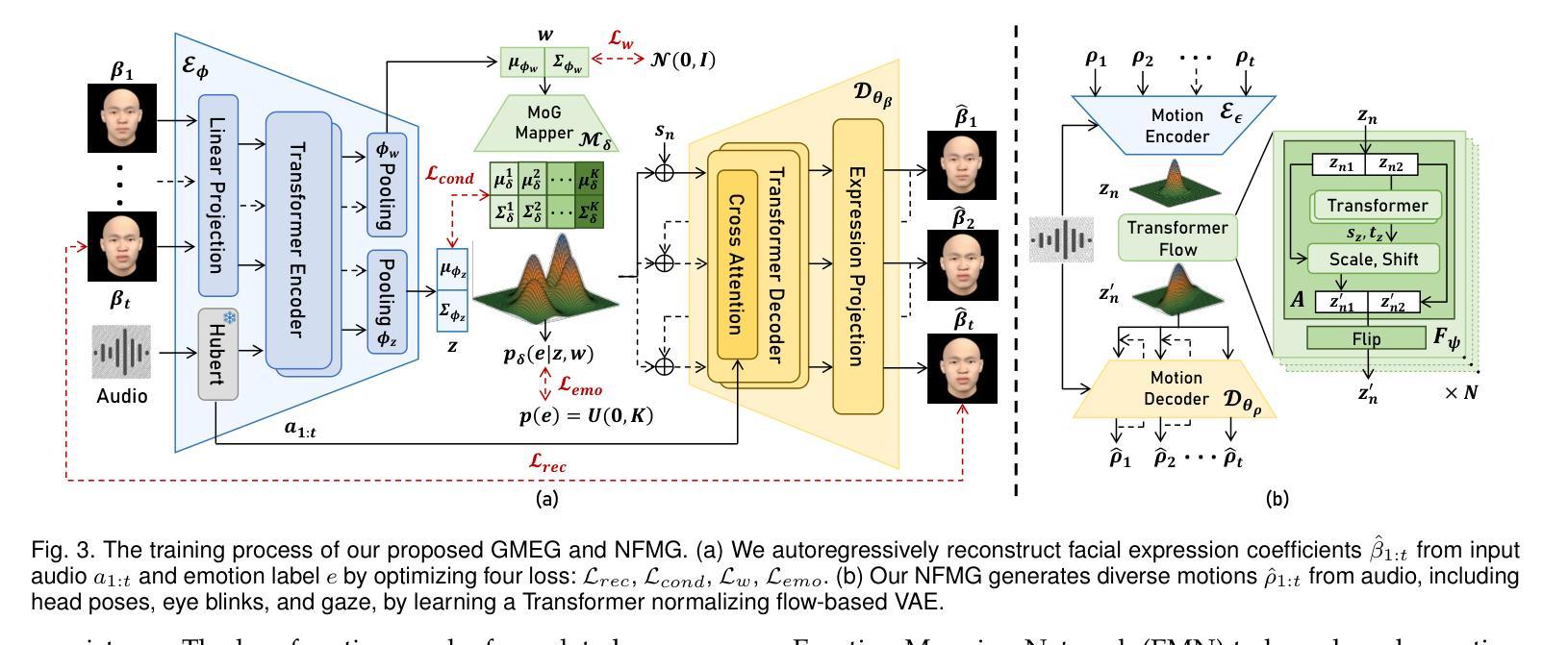