⚠️ 以下所有内容总结都来自于 大语言模型的能力,如有错误,仅供参考,谨慎使用
🔴 请注意:千万不要用于严肃的学术场景,只能用于论文阅读前的初筛!
💗 如果您觉得我们的项目对您有帮助 ChatPaperFree ,还请您给我们一些鼓励!⭐️ HuggingFace免费体验
2025-04-04 更新
Progressive Human Motion Generation Based on Text and Few Motion Frames
Authors:Ling-An Zeng, Gaojie Wu, Ancong Wu, Jian-Fang Hu, Wei-Shi Zheng
Although existing text-to-motion (T2M) methods can produce realistic human motion from text description, it is still difficult to align the generated motion with the desired postures since using text alone is insufficient for precisely describing diverse postures. To achieve more controllable generation, an intuitive way is to allow the user to input a few motion frames describing precise desired postures. Thus, we explore a new Text-Frame-to-Motion (TF2M) generation task that aims to generate motions from text and very few given frames. Intuitively, the closer a frame is to a given frame, the lower the uncertainty of this frame is when conditioned on this given frame. Hence, we propose a novel Progressive Motion Generation (PMG) method to progressively generate a motion from the frames with low uncertainty to those with high uncertainty in multiple stages. During each stage, new frames are generated by a Text-Frame Guided Generator conditioned on frame-aware semantics of the text, given frames, and frames generated in previous stages. Additionally, to alleviate the train-test gap caused by multi-stage accumulation of incorrectly generated frames during testing, we propose a Pseudo-frame Replacement Strategy for training. Experimental results show that our PMG outperforms existing T2M generation methods by a large margin with even one given frame, validating the effectiveness of our PMG. Code is available at https://github.com/qinghuannn/PMG.
尽管现有的文本到动作(T2M)方法可以从文本描述生成逼真的人类动作,但由于仅使用文本不足以精确描述各种姿势,因此将生成的动作与所需的姿势对齐仍然很困难。为了实现更可控的生成,一种直观的方法是允许用户输入几个描述精确所需姿势的动作帧。因此,我们探索了一个新的文本框架到动作(TF2M)生成任务,旨在从文本和少量给定的帧生成动作。直观地,一个帧与给定的帧越接近,当以这个给定帧为条件时,该帧的不确定性就越低。因此,我们提出了一种新颖的分步动作生成(PMG)方法,从不确定性较低的帧逐步生成不确定性较高的帧,分为多个阶段。在每个阶段,新的帧由文本框架引导生成器根据文本、给定帧和先前阶段生成的帧的框架感知语义来生成。此外,为了减轻测试时因不正确生成的帧的多阶段累积而导致的训练和测试之间的差距,我们提出了用于训练的伪帧替换策略。实验结果表明,我们的PMG在给定一个框架的情况下就大幅度超越了现有的T2M生成方法,验证了我们的PMG的有效性。代码可在https://github.com/qinghuannn/PMG找到。
论文及项目相关链接
PDF Accepted to IEEE Transactions on Circuits and Systems for Video Technology (TCSVT), 2025
Summary
本文探讨了文本到动作(T2M)方法的局限性,即仅通过文本描述生成的动作难以与期望的姿势对齐。为此,文章提出了一种新的文本框架到动作(TF2M)生成任务,旨在从文本和少量给定框架生成动作。文章还提出了一种渐进动作生成(PMG)方法,该方法可分阶段从不确定性较低的框架开始生成动作,逐步生成不确定性较高的框架。实验结果表明,与现有的T2M生成方法相比,即使在只有一个给定框架的情况下,PMG也能大幅度提高性能。
Key Takeaways
- 文本到动作(T2M)方法存在难度,难以将生成的动作与期望的姿势对齐。
- 引入新的文本框架到动作(TF2M)生成任务,旨在结合文本和少量给定框架来生成动作。
- 提出渐进动作生成(PMG)方法,分阶段从确定性较高的框架开始生成动作。
- PMG方法利用文本框架指导生成器,以及前一阶段生成的框架。
- 为了缩小训练与测试之间的差距,采用伪框架替换策略进行训练。
- 实验结果表明,PMG方法在只有一个给定框架的情况下,性能优于现有的T2M生成方法。
点此查看论文截图
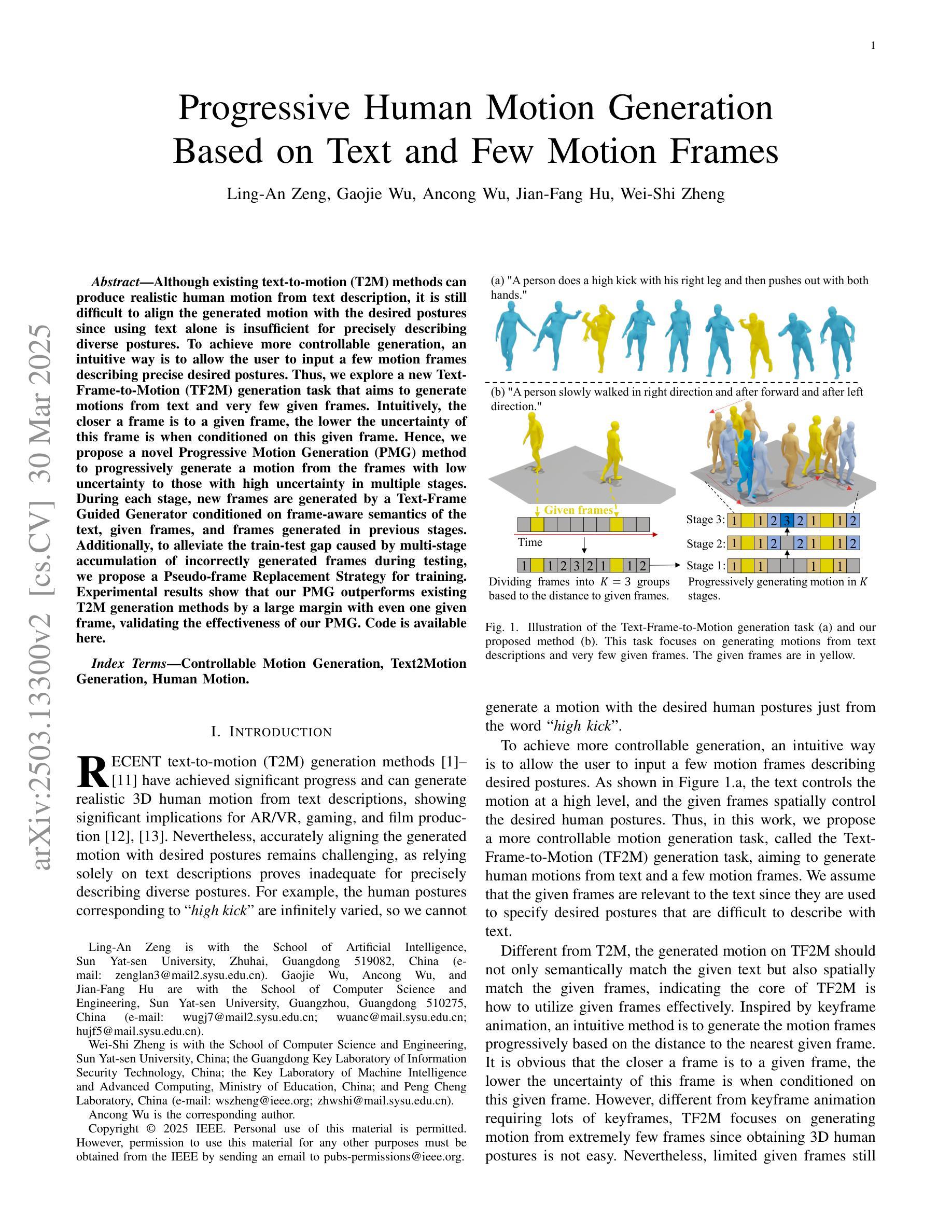
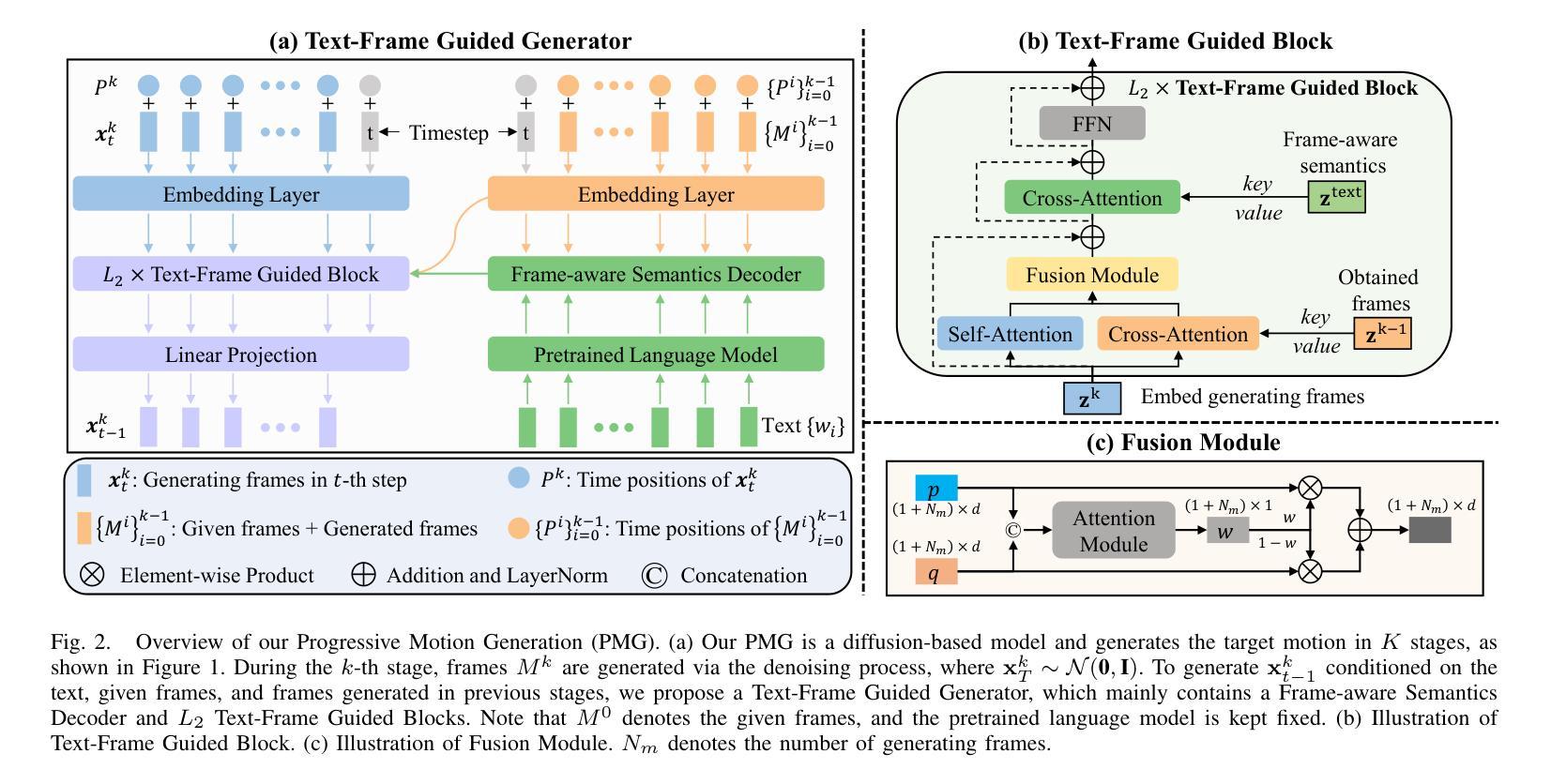
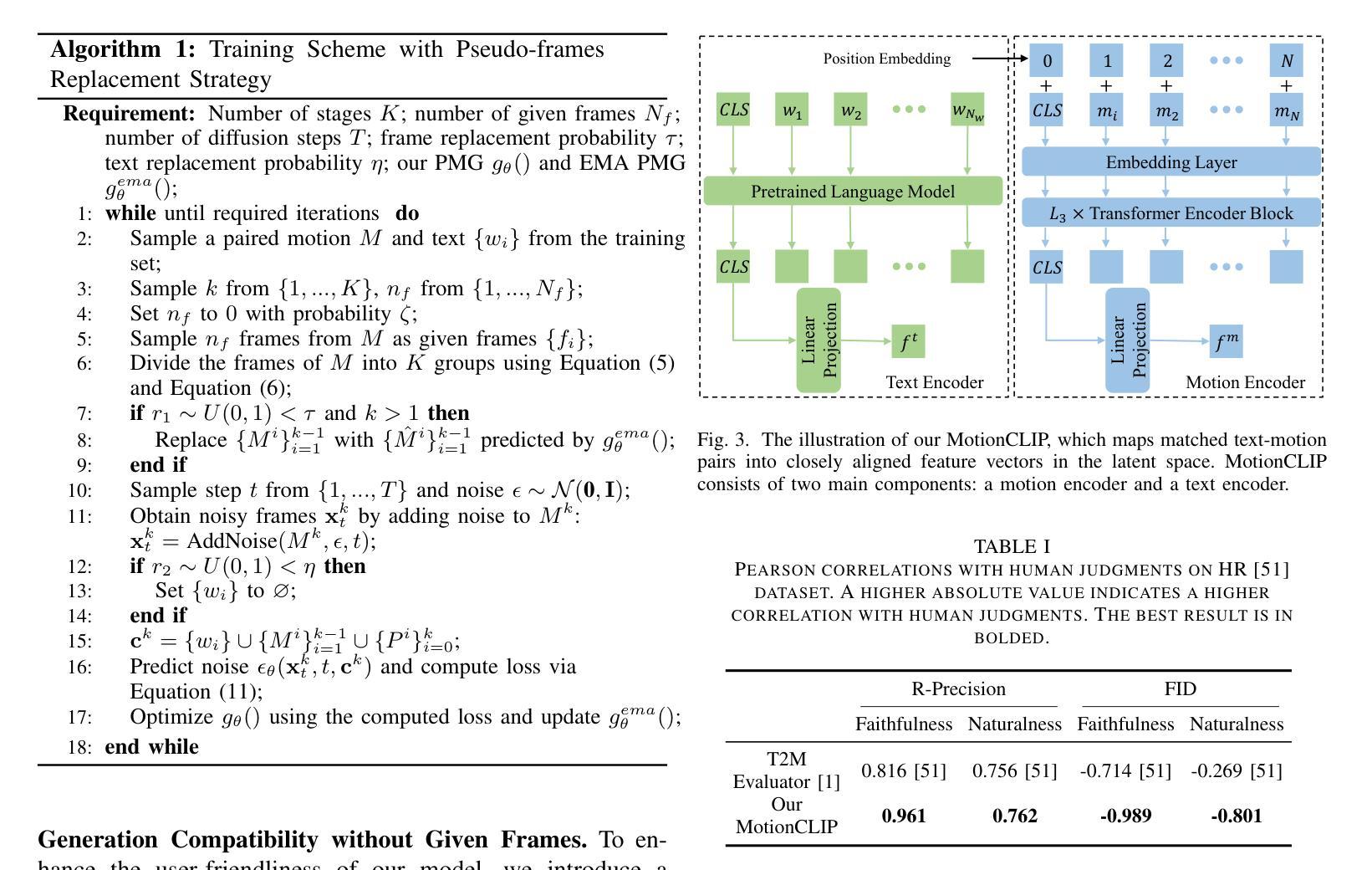
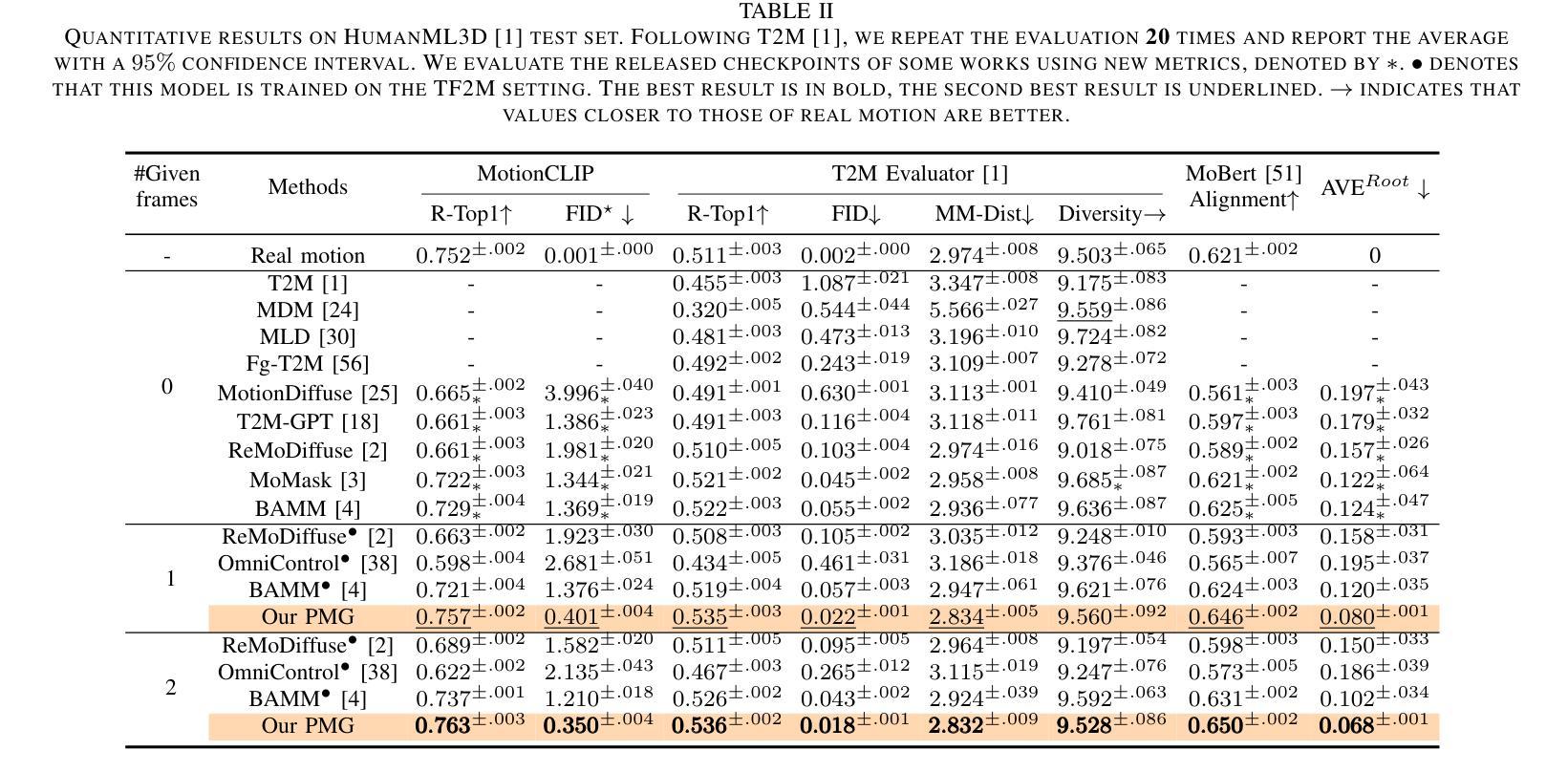