⚠️ 以下所有内容总结都来自于 大语言模型的能力,如有错误,仅供参考,谨慎使用
🔴 请注意:千万不要用于严肃的学术场景,只能用于论文阅读前的初筛!
💗 如果您觉得我们的项目对您有帮助 ChatPaperFree ,还请您给我们一些鼓励!⭐️ HuggingFace免费体验
2025-04-04 更新
BioAtt: Anatomical Prior Driven Low-Dose CT Denoising
Authors:Namhun Kim, UiHyun Cho
Deep-learning-based denoising methods have significantly improved Low-Dose CT (LDCT) image quality. However, existing models often over-smooth important anatomical details due to their purely data-driven attention mechanisms. To address this challenge, we propose a novel LDCT denoising framework, BioAtt. The key innovation lies in attending anatomical prior distributions extracted from the pretrained vision-language model BiomedCLIP. These priors guide the denoising model to focus on anatomically relevant regions to suppress noise while preserving clinically relevant structures. We highlight three main contributions: BioAtt outperforms baseline and attention-based models in SSIM, PSNR, and RMSE across multiple anatomical regions. The framework introduces a new architectural paradigm by embedding anatomic priors directly into spatial attention. Finally, BioAtt attention maps provide visual confirmation that the improvements stem from anatomical guidance rather than increased model complexity.
基于深度学习的去噪方法已显著提高低剂量CT(LDCT)图像质量。然而,现有模型由于其纯粹的数据驱动注意力机制,经常会过度平滑重要的解剖细节。为了解决这一挑战,我们提出了一种新型的LDCT去噪框架——BioAtt。关键创新点在于,该框架关注从预训练的视觉语言模型BiomedCLIP中提取的解剖先验分布。这些先验知识引导去噪模型关注解剖相关的区域,以在保留临床上相关结构的同时抑制噪声。我们强调了三个主要贡献:BioAtt在多个解剖区域的SSIM、PSNR和RMSE方面均优于基准模型和基于注意力的模型。该框架通过直接将解剖先验嵌入空间注意力,引入了一种新的架构范式。最后,BioAtt的注意力图从视觉上证实了改进源于解剖指导而非模型复杂度的增加。
论文及项目相关链接
PDF 14 pages
Summary
深度学习在医学图像去噪领域的应用已经显著提高了低剂量CT(LDCT)图像的质量。然而,现有模型由于纯粹的基于数据的注意力机制,往往会过度平滑重要的解剖细节。为了应对这一挑战,我们提出了一种新的LDCT去噪框架BioAtt。其核心创新之处在于引入了解剖先验分布,这些先验分布是从预训练的视觉语言模型BiomCLIP中提取的。这些先验指导去噪模型关注解剖相关的区域,从而在去噪的同时保留临床上重要的结构。
Key Takeaways
- 深度学习在低剂量CT图像去噪领域有显著改善图像质量的潜力。
- 当前模型因纯粹的基于数据的注意力机制而可能过度平滑解剖细节。
- BioAtt框架通过引入解剖先验分布解决了这一问题,这些先验分布来源于预训练的视觉语言模型BiomCLIP。
- BioAtt在多个解剖学区域的SSIM、PSNR和RMSE指标上优于基准模型和基于注意力的模型。
- BioAtt框架开创了新的架构范式,直接将解剖先验嵌入空间注意力中。
- BioAtt的注意力图可视化证实,改进源于解剖指导而非模型复杂度的增加。
- 该方法有望提高医学图像的质量和诊断的准确性。
点此查看论文截图
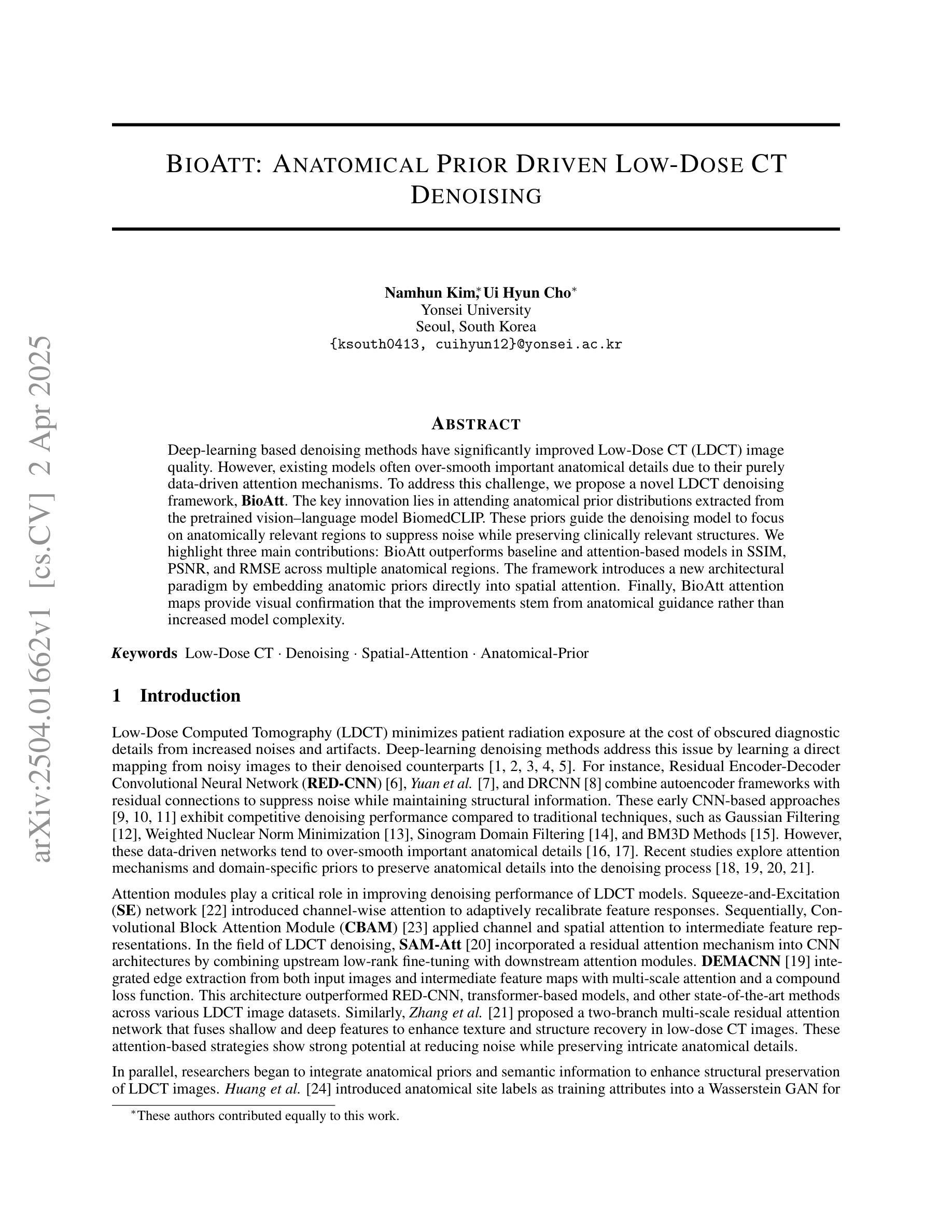
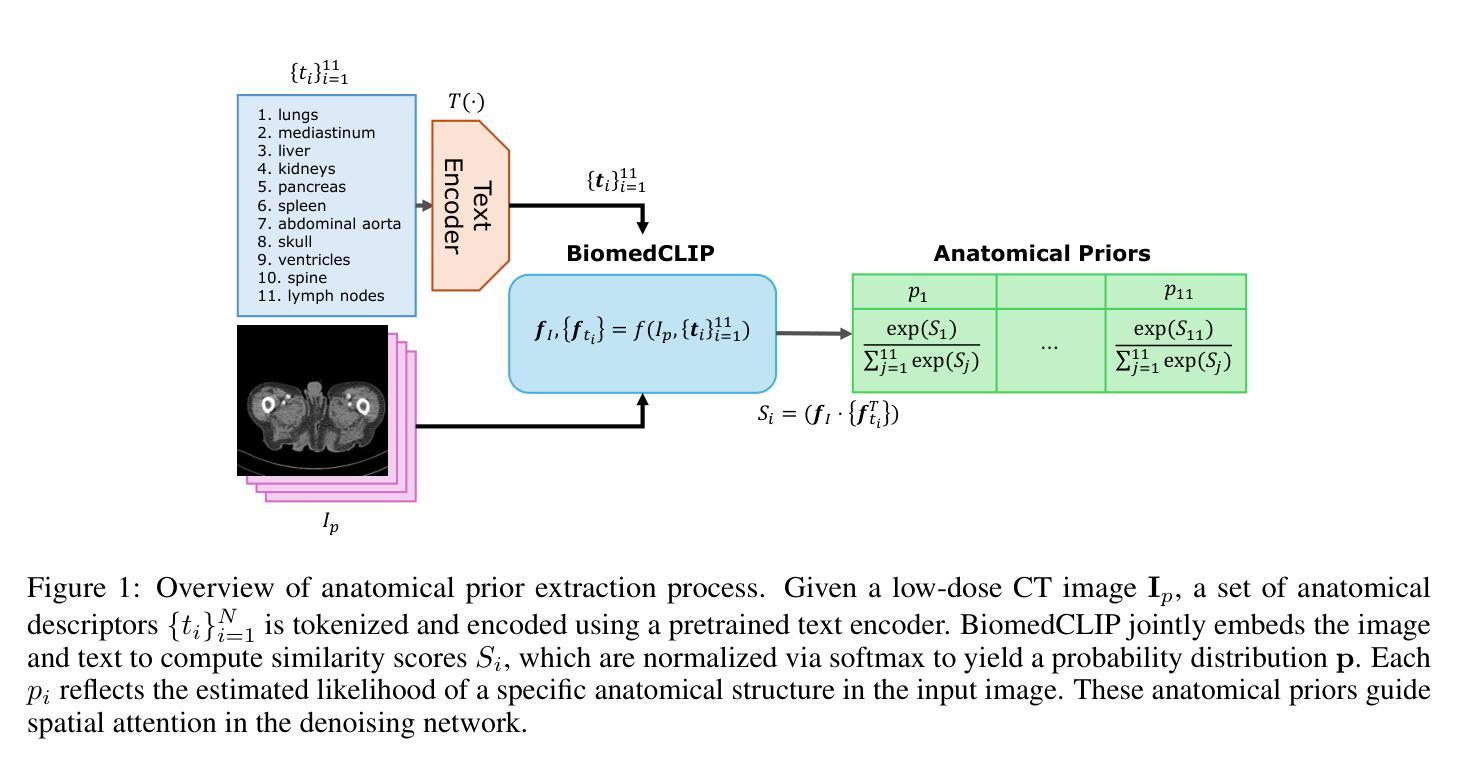
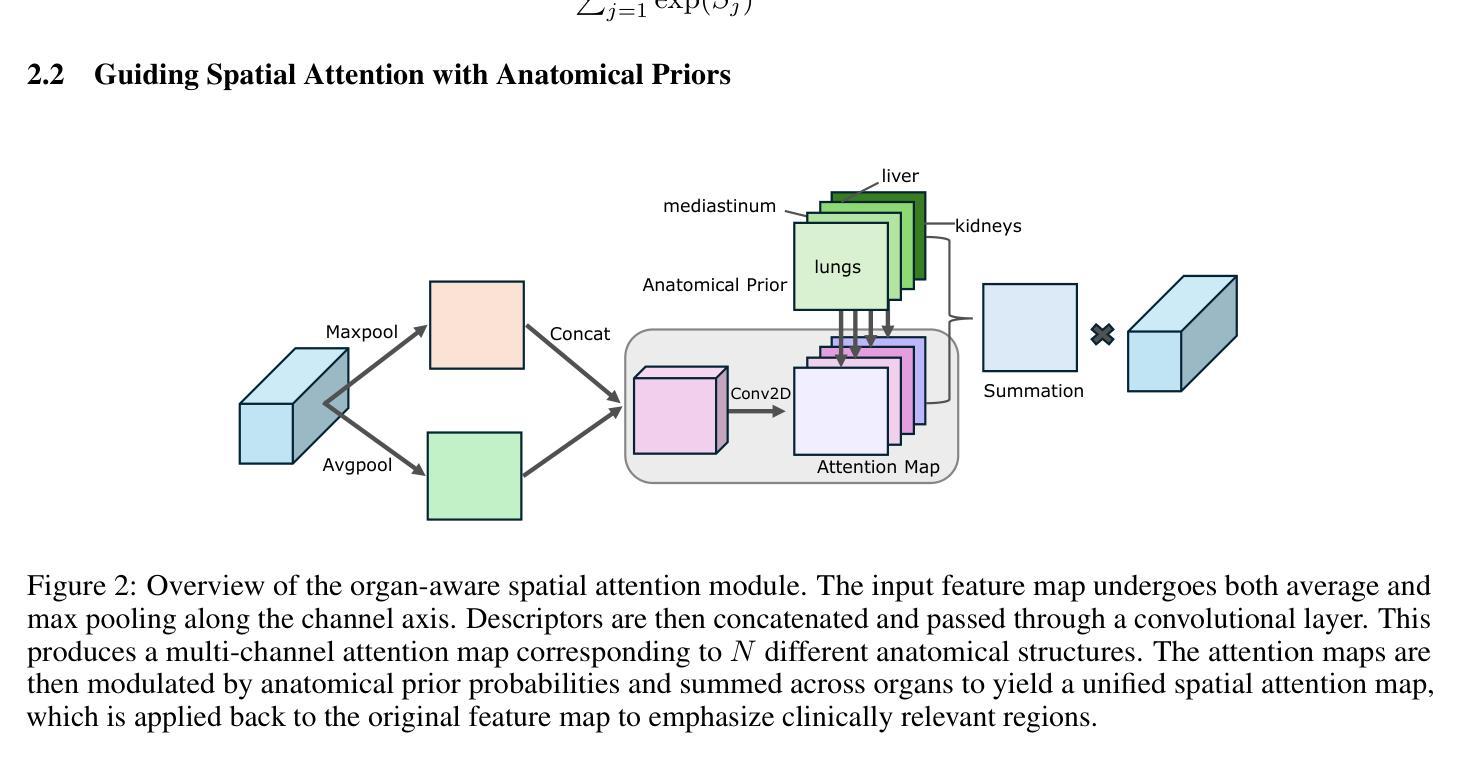
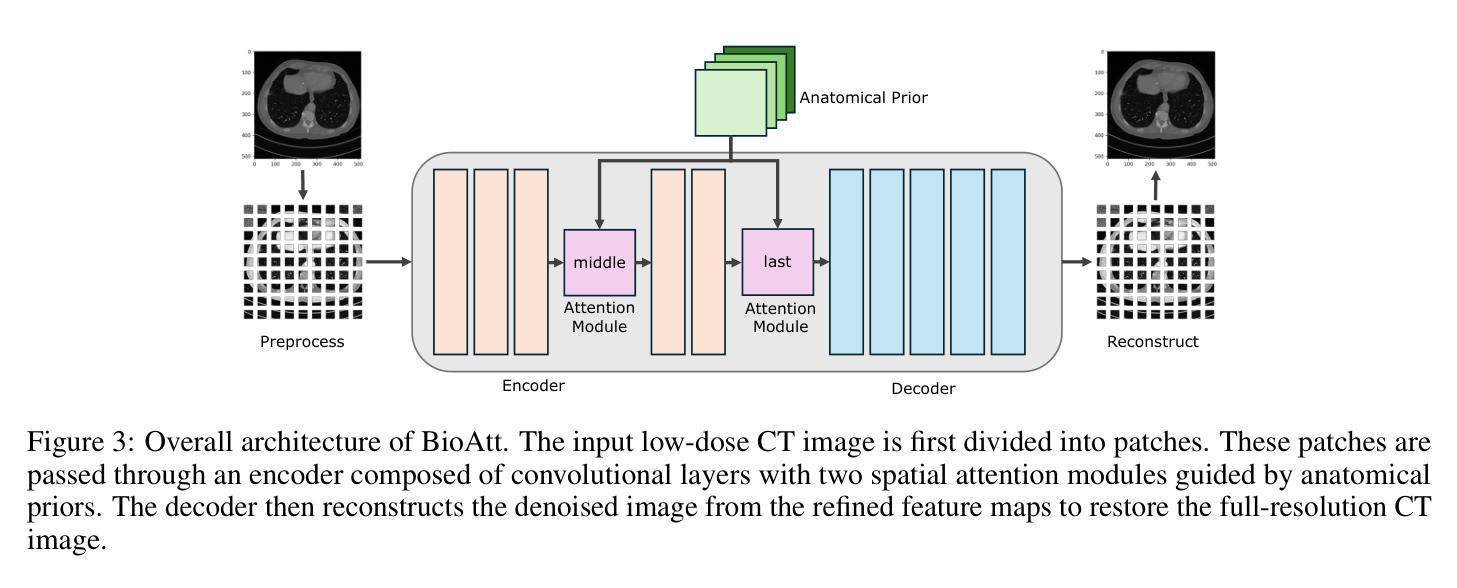
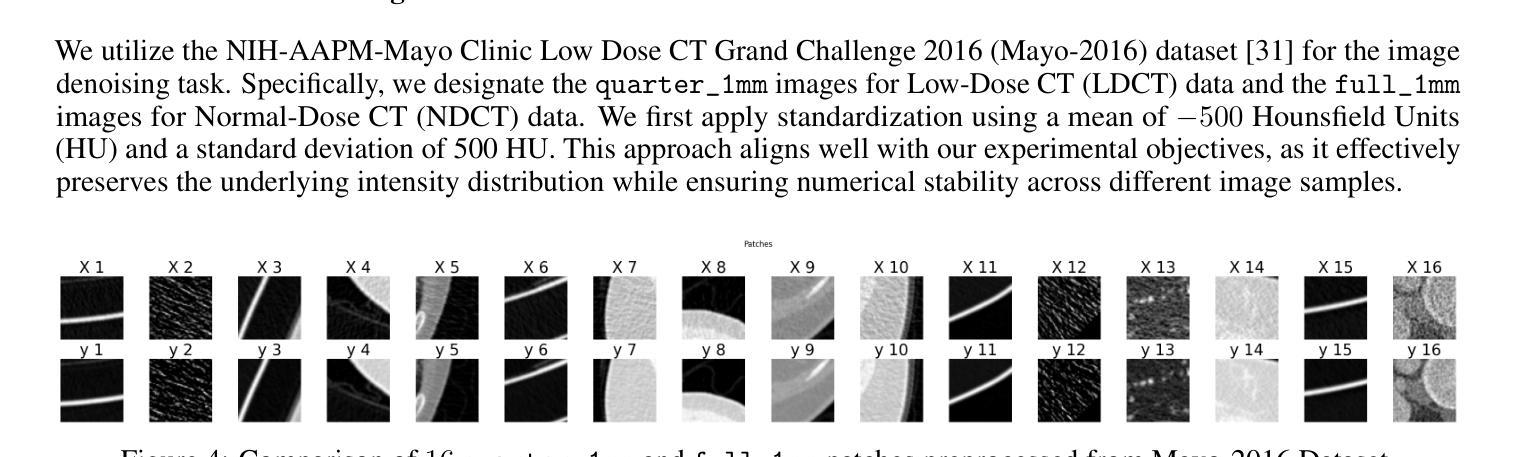
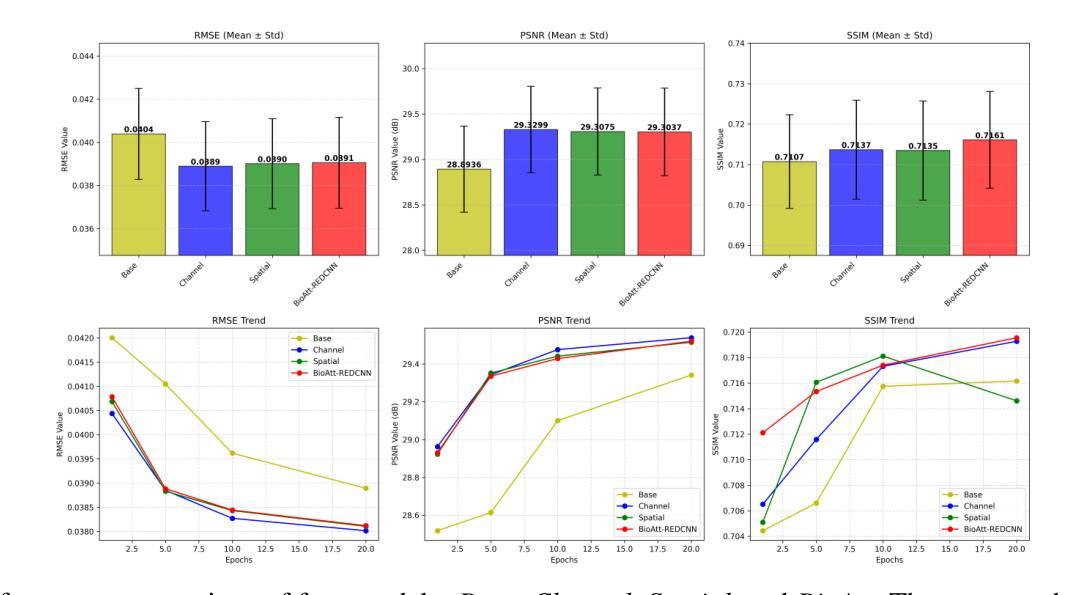
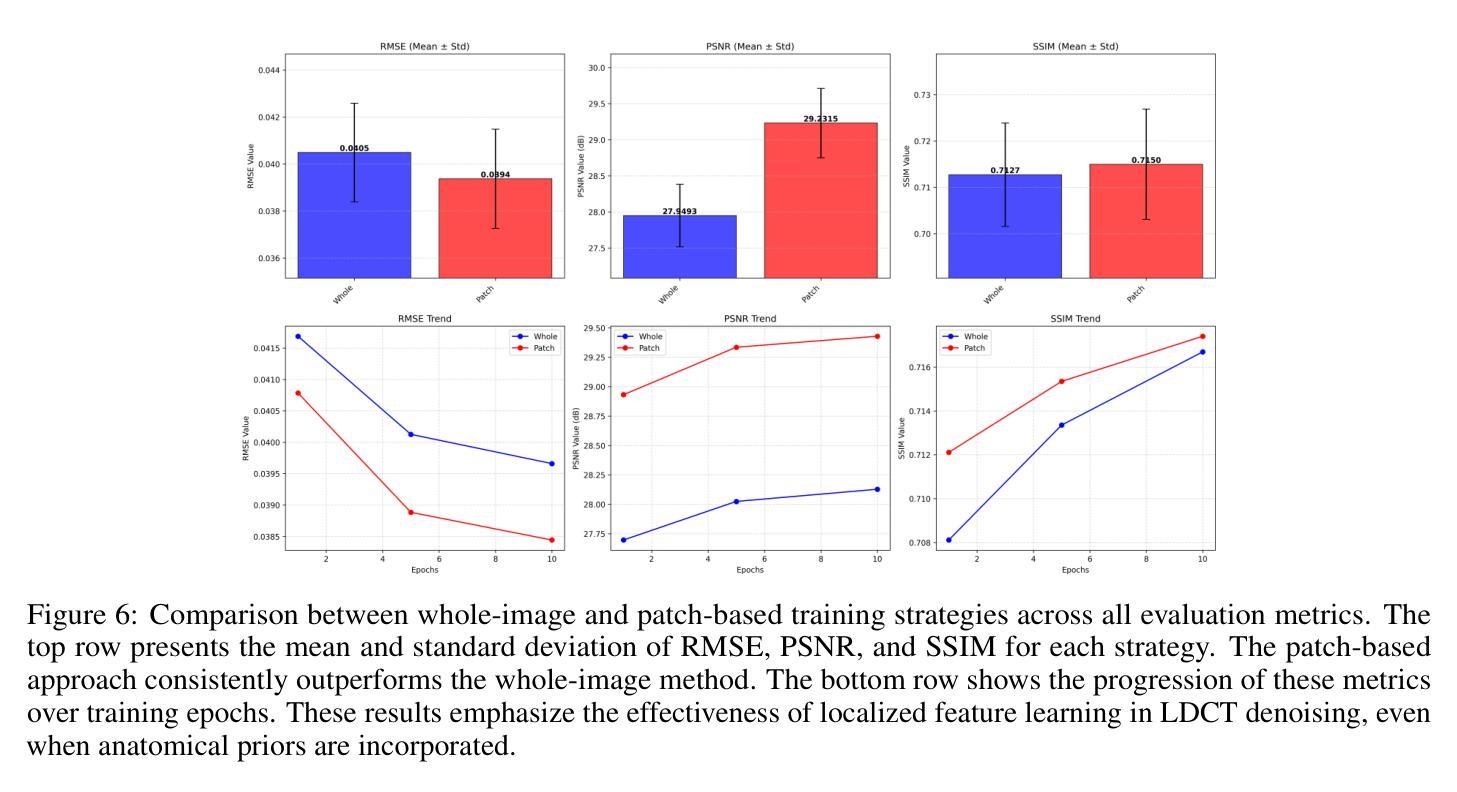
KTaO3(001) Preparation Methods in Vacuum: Effects on Surface Stoichiometry, Crystallography, and in-gap States
Authors:Andrea M. Lucero Manzano, Esteban D. Cantero, Emanuel A. Martínez, F. Y. Bruno, Esteban A. Sánchez, Oscar Grizzi
KTaO3 single crystals with different orientations are used as substrates for the epitaxial growth of thin films and/or as hosts for two-dimensional electron gases. Due to the polar nature of the KTaO3(001) surface, one can expect difficulties and challenges to arise in its preparation. Maintaining good insulating characteristics without adding undesirable in-gap electronic states, obtaining good crystalline order up to the top surface layer, a sufficiently flat surface, and complete cleanliness of the surface (without water, C or OH contaminants), are in general difficult conditions to accomplish simultaneously. Cleaving in vacuum is likely the best option for obtaining a clean surface. However, since KTaO3 is cubic and lacks a well-defined cleavage plane, this method is notsuitable for sample growth or reproducible device fabrication. Here, we systematically evaluate the effect of typical preparation methods applied on the surfaces of KTaO3(001) single crystals. In particular, we used annealing in vacuum at different temperatures, light sputtering with Ar+ ions at low energy (500 eV) followed by annealing, heavy Ar+ ion bombardment and annealing, and grazing Ar+ ion bombardment under continuous azimuthal rotation combined with both annealing in vacuum and in O2 atmosphere. Possible side effects after each treatment are evaluated by a combination of techniques, including low-energy ion scattering at forward angles, Auger electron spectroscopy, low-energy electron energy loss, X-ray photoelectron spectroscopy, low-energy electron diffraction, and time of flightsecondary ion mass spectrometry. Advantages and shortcomings of each preparation method are discussed in detail.
KTaO3单晶具有不同的取向,可用作外延生长薄膜的衬底和/或二维电子气体的宿主。由于KTaO3(001)表面的极性特征,其在制备过程中可能会出现一些困难和挑战。在保持优良的绝缘性能且不增加不必要的带隙电子态、获得良好的结晶序达到表层、具有足够平坦的表面以及表面完全清洁(无水分、碳或羟基污染物)的同时,通常很难同时实现这些条件。真空中的劈裂可能是获得清洁表面的最佳方法。然而,由于KTaO3是立方的并且没有明确的劈裂面,因此这种方法不适用于样品生长或可重复的器件制造。在这里,我们系统地评估了典型的制备方法对KTaO3(001)单晶表面的影响。特别是,我们在不同的温度下进行了真空退火处理,使用低能量(500 eV)的Ar+离子进行轻度溅射后再进行退火处理,受到重Ar+离子轰击和退火处理,以及在连续方位旋转下进行倾斜Ar+离子轰击,并结合真空和氧气氛围中的退火处理。通过结合多种技术评估每种处理后的可能副作用,包括前向角度下的低能离子散射、俄歇电子光谱、低能电子能量损失、X射线光电子光谱、低能电子衍射以及飞行时间二次离子质谱。每种制备方法的优点和缺点都进行了详细讨论。
论文及项目相关链接
PDF 28 pages, 8 figures
Summary
KTaO3单晶因其极性表面在制备过程中面临多种挑战,如保持良好绝缘性能、确保晶体结构有序至表层、获得足够平滑且清洁的表面等。本文系统评估了不同处理方法对KTaO3(001)单晶表面的影响,包括真空退火、低能Ar+离子溅射结合退火等处理方式,并讨论了各种方法的优缺点。
Key Takeaways
- KTaO3单晶因其极性表面在制备过程中存在多种挑战。
- 真空退火是常用的处理方法之一,但KTaO3缺乏明确的解理面,不适合用于样品生长和可重复器件制造。
- 低能Ar+离子溅射结合退火处理可有效改善KTaO3表面性质。
- 重离子轰击和真空及氧气环境下的退火处理也是常见的处理方式。
- 多种技术被用于评估处理后的效果,包括低能离子散射、俄歇电子光谱、低能电子能量损失等。
- 每种制备方法都有其优势和局限性,需根据实际情况选择合适的方法。
点此查看论文截图
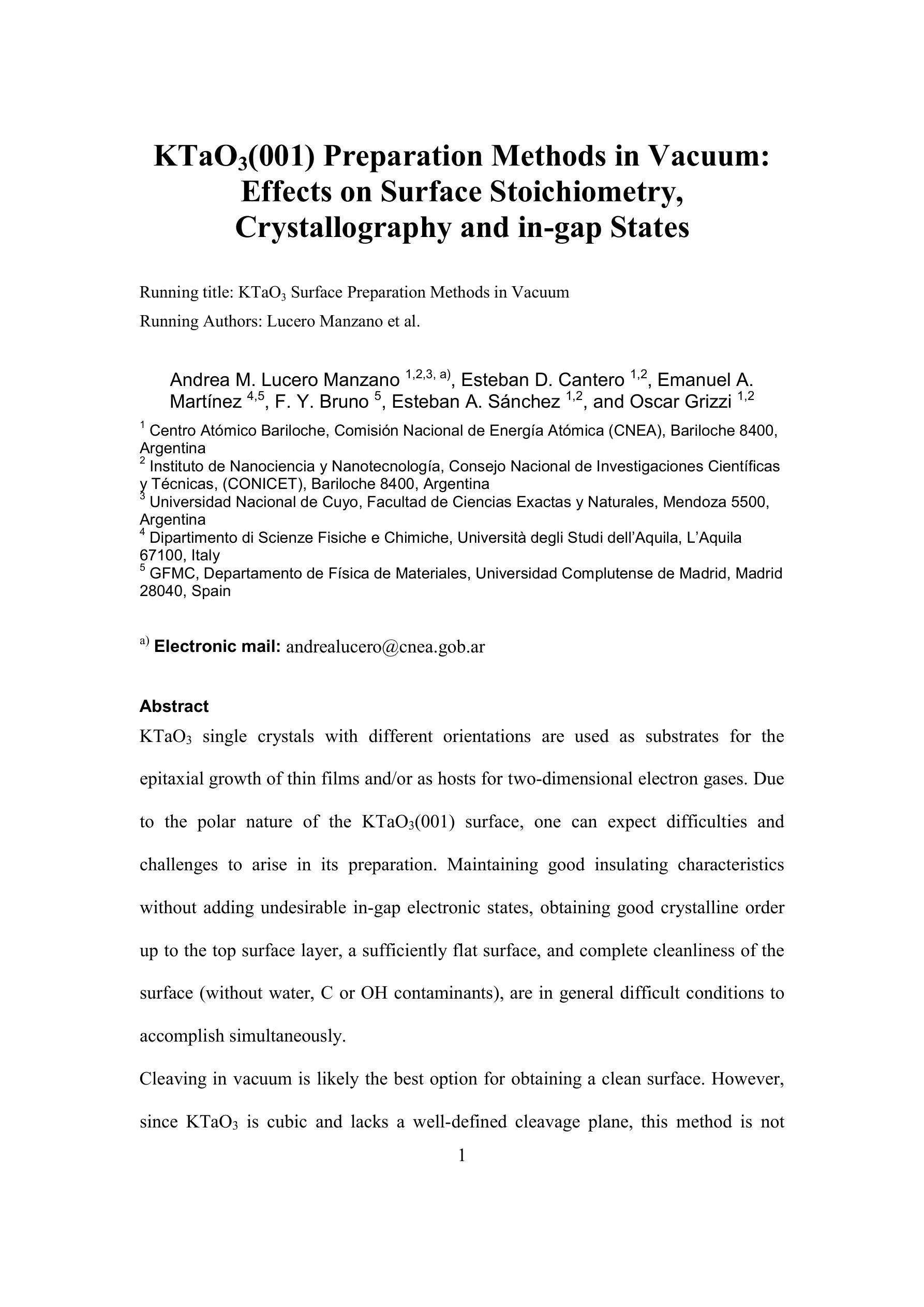
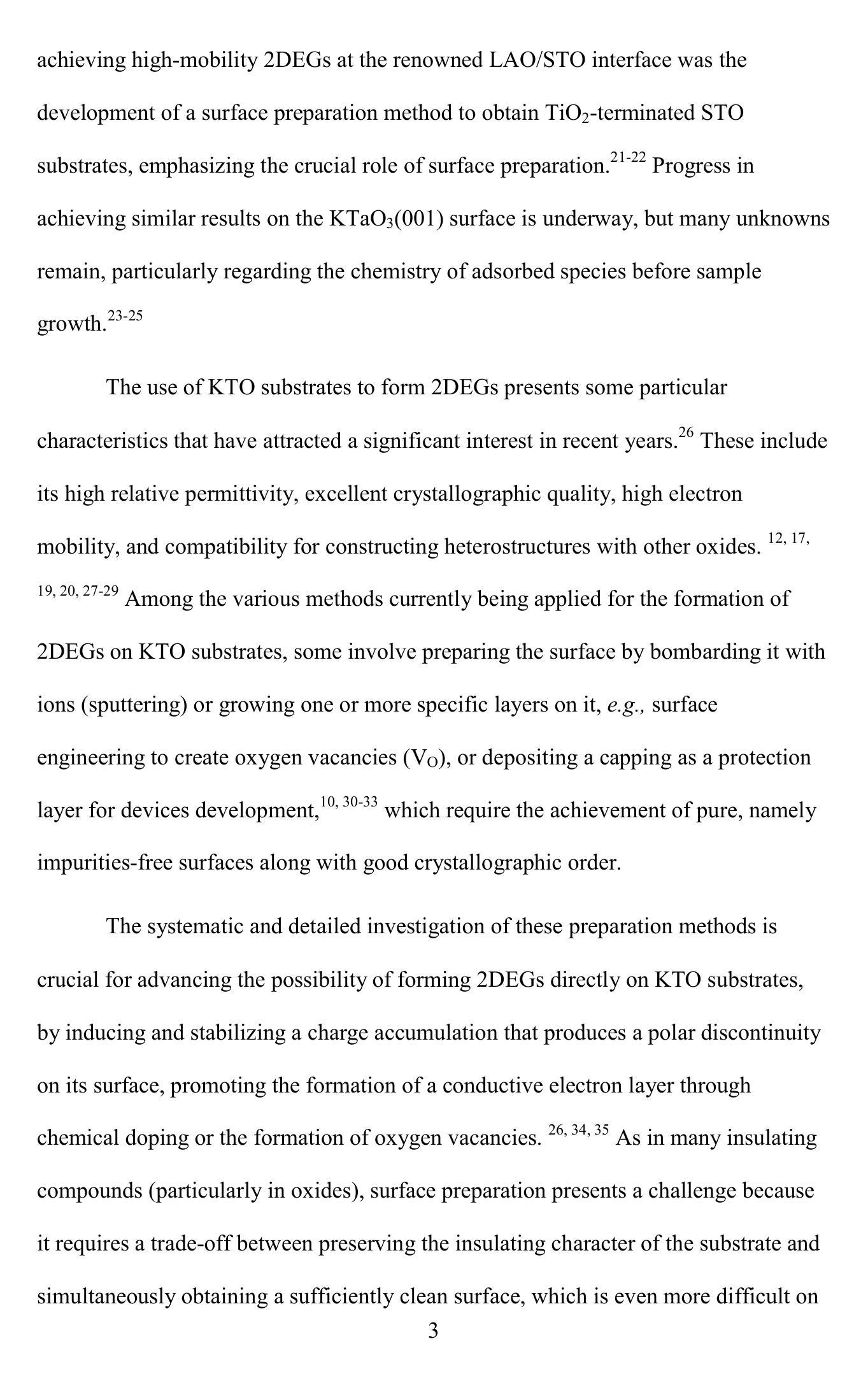
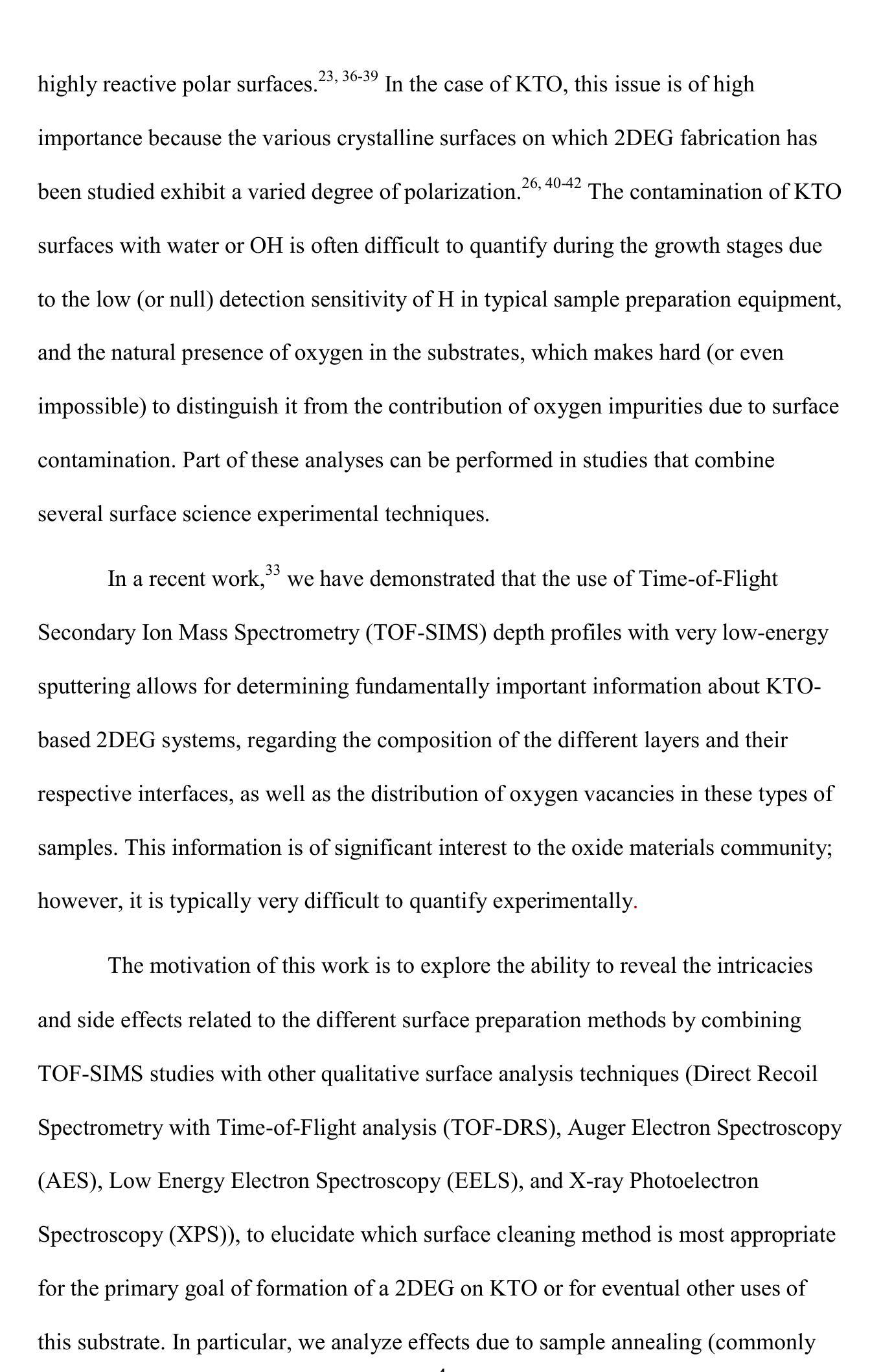
STPNet: Scale-aware Text Prompt Network for Medical Image Segmentation
Authors:Dandan Shan, Zihan Li, Yunxiang Li, Qingde Li, Jie Tian, Qingqi Hong
Accurate segmentation of lesions plays a critical role in medical image analysis and diagnosis. Traditional segmentation approaches that rely solely on visual features often struggle with the inherent uncertainty in lesion distribution and size. To address these issues, we propose STPNet, a Scale-aware Text Prompt Network that leverages vision-language modeling to enhance medical image segmentation. Our approach utilizes multi-scale textual descriptions to guide lesion localization and employs retrieval-segmentation joint learning to bridge the semantic gap between visual and linguistic modalities. Crucially, STPNet retrieves relevant textual information from a specialized medical text repository during training, eliminating the need for text input during inference while retaining the benefits of cross-modal learning. We evaluate STPNet on three datasets: COVID-Xray, COVID-CT, and Kvasir-SEG. Experimental results show that our vision-language approach outperforms state-of-the-art segmentation methods, demonstrating the effectiveness of incorporating textual semantic knowledge into medical image analysis. The code has been made publicly on https://github.com/HUANGLIZI/STPNet.
病变的精确分割在医学图像分析和诊断中起着至关重要的作用。传统的仅依赖视觉特征的分割方法通常难以应对病变分布和大小中的固有不确定性。为了解决这些问题,我们提出了STPNet,一个基于尺度的文本提示网络,它利用视觉语言模型来提高医学图像分割的效果。我们的方法利用多尺度文本描述来引导病变定位,并采用检索分割联合学习来弥合视觉和语言模态之间的语义鸿沟。重要的是,STPNet在训练过程中从专门的医学文本存储库中检索相关的文本信息,从而在推理过程中不需要文本输入,同时保留跨模态学习的优点。我们在三个数据集上评估了STPNet:COVID-Xray、COVID-CT和Kvasir-SEG。实验结果表明,我们的视觉语言方法优于最先进的分割方法,证明了将文本语义知识融入医学图像分析中的有效性。代码已公开在https://github.com/HUANGLIZI/STPNet。
论文及项目相关链接
Summary
医学图像分析中,病灶准确分割对诊断至关重要。传统方法常因病灶分布和大小的不确定性而表现不佳。STPNet通过结合视觉和语言建模提升医学图像分割,利用多尺度文本描述引导病灶定位,通过检索分割联合学习缩小视觉与语言模态间的语义鸿沟。STPNet训练时从专用医学文本库中检索相关文本信息,推断时无需文本输入,仍保留跨模态学习的优势。在COVID-Xray、COVID-CT和Kvasir-SEG三个数据集上的实验证明,STPNet的视语言方法优于最新分割方法,突显融入文本语义知识对医学图像分析的有效性。代码已公开于https://github.com/HUANGLIZI/STPNet。
Key Takeaways
- 医学图像分析中,STPNet通过结合视觉和语言建模提升医学图像分割的准确性。
- STPNet利用多尺度文本描述来引导病灶定位,增强了模型对病灶分布和大小不确定性的处理能力。
- 通过检索分割联合学习,STPNet缩小了视觉与语言模态之间的语义鸿沟。
- STPNet在训练时从专用医学文本库中检索相关文本信息,提高模型的性能。
- 该方法在推断时无需额外的文本输入,保持了跨模态学习的优势。
- 在多个数据集上的实验证明STPNet的视语言方法优于现有的分割方法。
点此查看论文截图
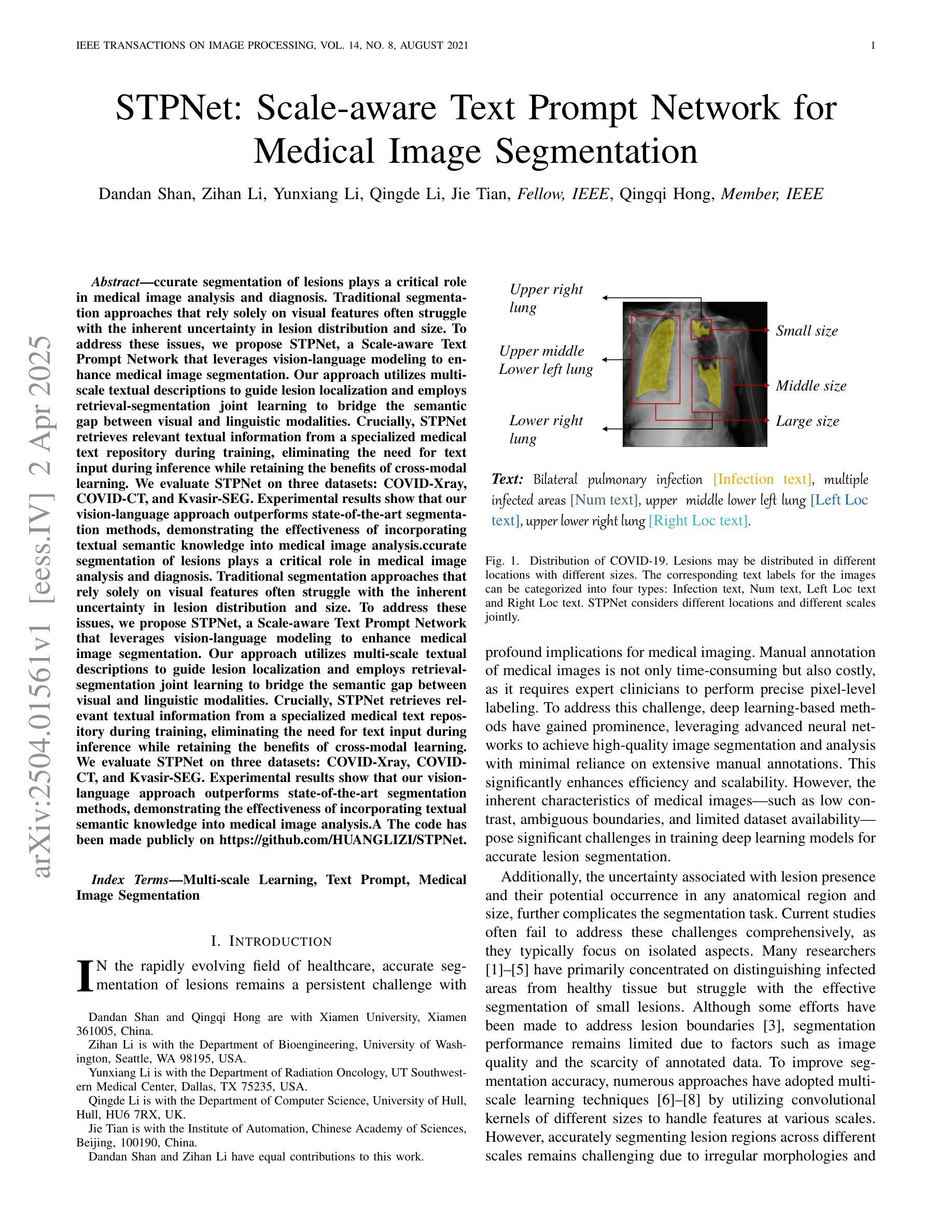
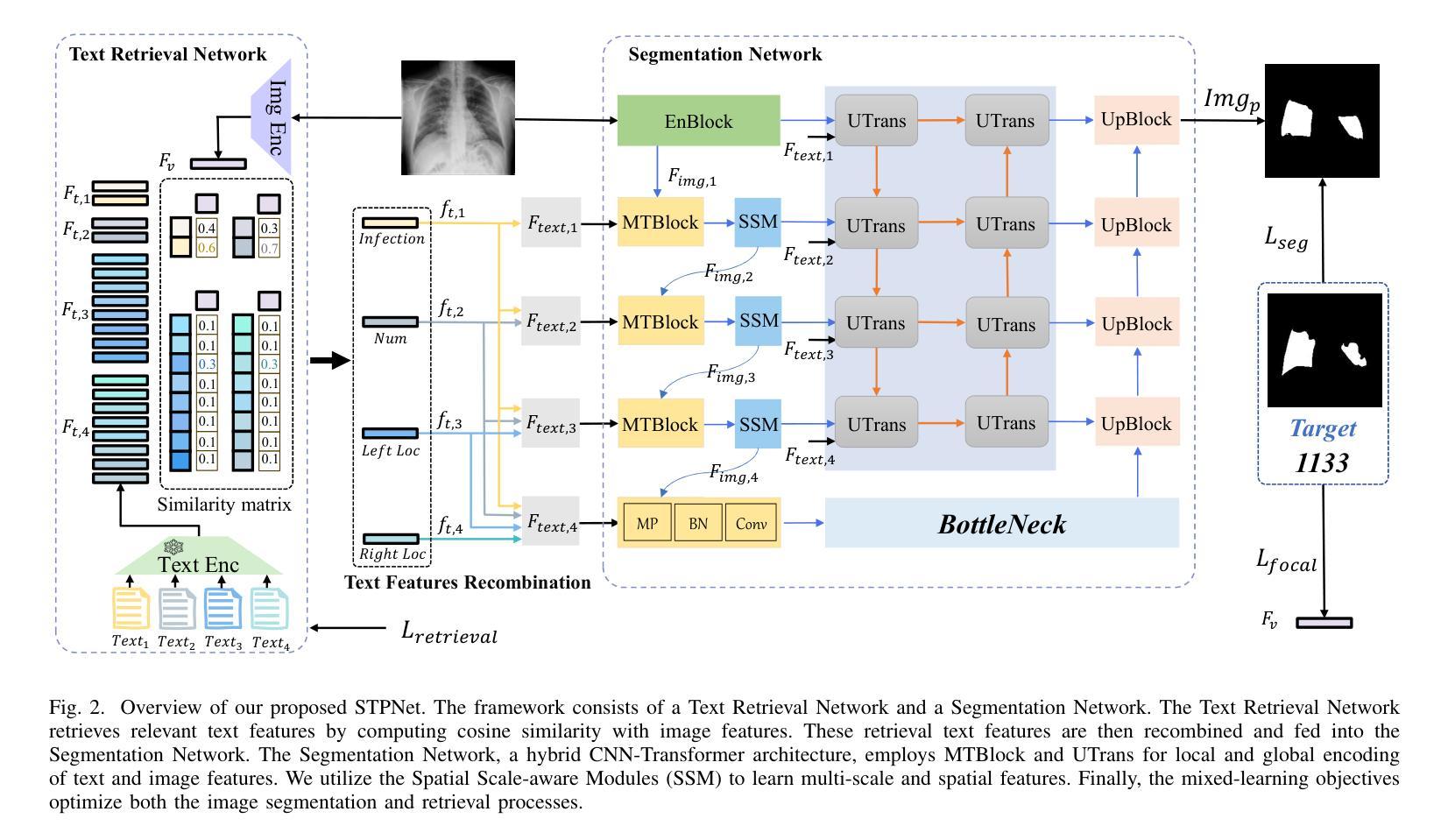
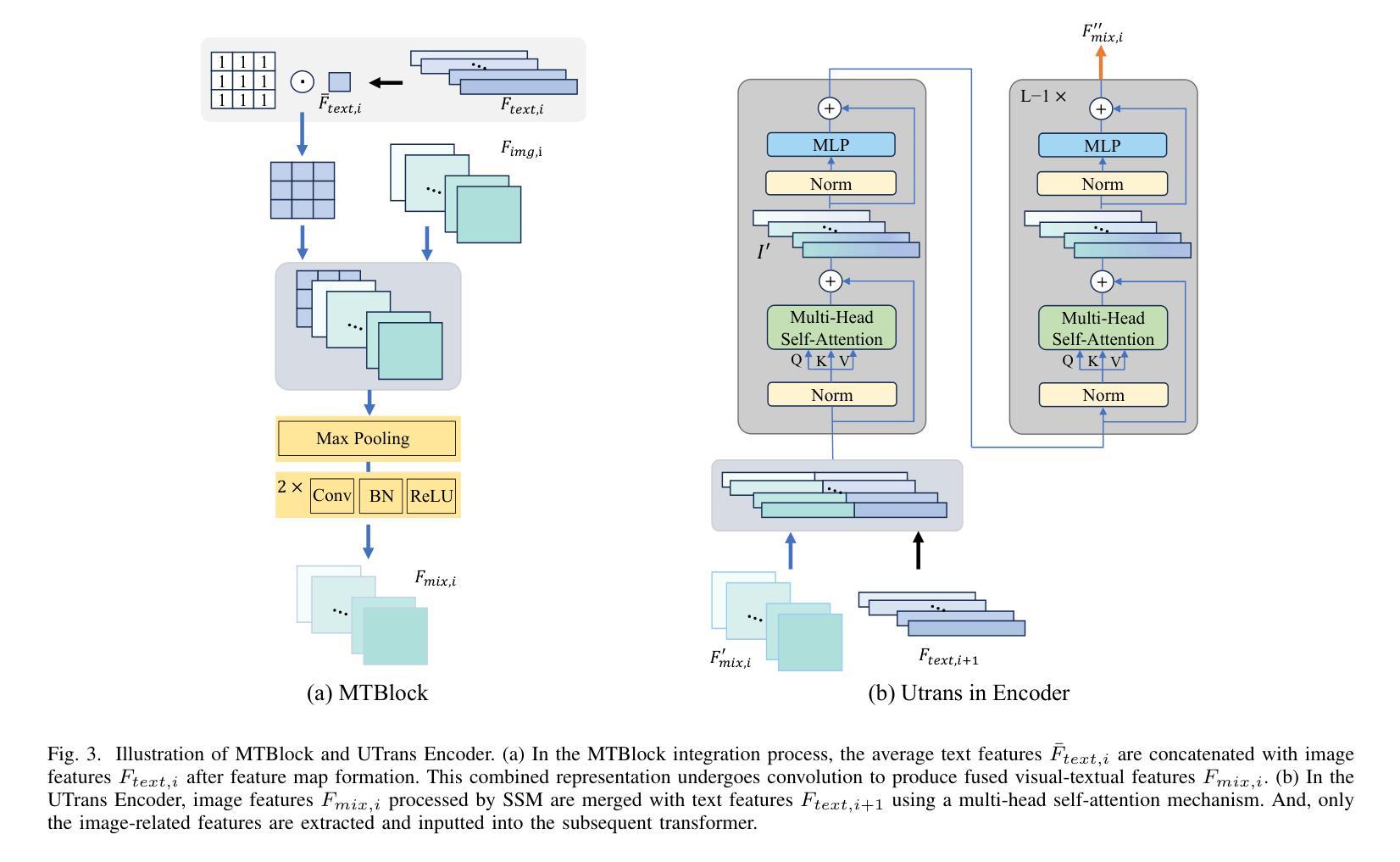
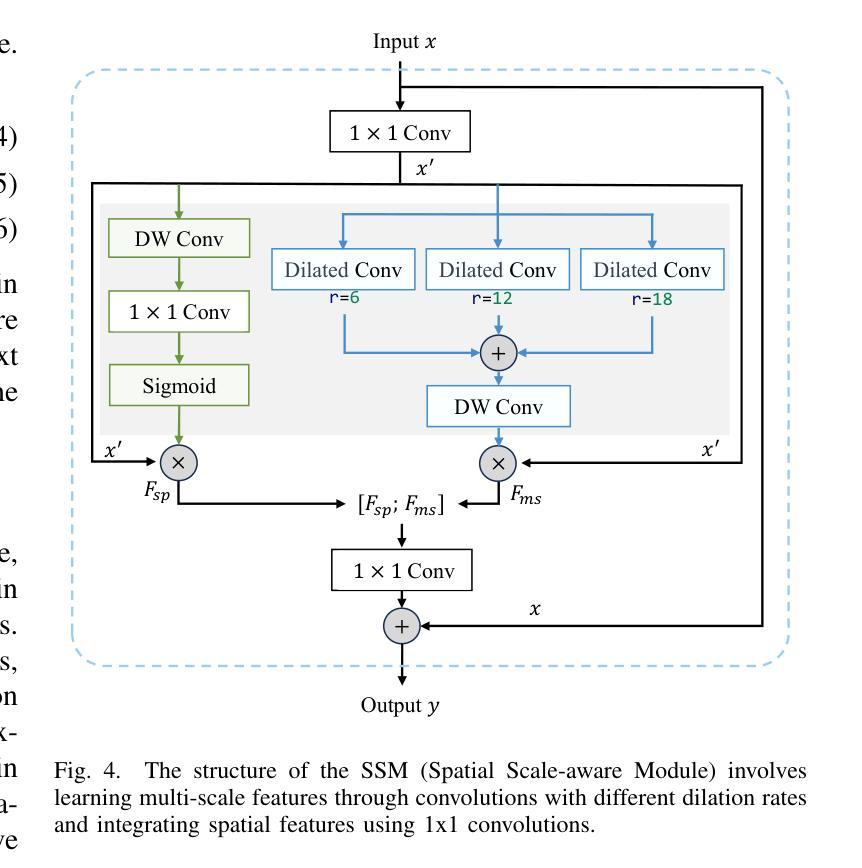
Semi-Supervised Biomedical Image Segmentation via Diffusion Models and Teacher-Student Co-Training
Authors:Luca Ciampi, Gabriele Lagani, Giuseppe Amato, Fabrizio Falchi
Supervised deep learning for semantic segmentation has achieved excellent results in accurately identifying anatomical and pathological structures in medical images. However, it often requires large annotated training datasets, which limits its scalability in clinical settings. To address this challenge, semi-supervised learning is a well-established approach that leverages both labeled and unlabeled data. In this paper, we introduce a novel semi-supervised teacher-student framework for biomedical image segmentation, inspired by the recent success of generative models. Our approach leverages denoising diffusion probabilistic models (DDPMs) to generate segmentation masks by progressively refining noisy inputs conditioned on the corresponding images. The teacher model is first trained in an unsupervised manner using a cycle-consistency constraint based on noise-corrupted image reconstruction, enabling it to generate informative semantic masks. Subsequently, the teacher is integrated into a co-training process with a twin-student network. The student learns from ground-truth labels when available and from teacher-generated pseudo-labels otherwise, while the teacher continuously improves its pseudo-labeling capabilities. Finally, to further enhance performance, we introduce a multi-round pseudo-label generation strategy that iteratively improves the pseudo-labeling process. We evaluate our approach on multiple biomedical imaging benchmarks, spanning multiple imaging modalities and segmentation tasks. Experimental results show that our method consistently outperforms state-of-the-art semi-supervised techniques, highlighting its effectiveness in scenarios with limited annotated data. The code to replicate our experiments can be found at https://github.com/ciampluca/diffusion_semi_supervised_biomedical_image_segmentation
监督深度学习在医学图像准确识别解剖和病理结构方面取得了优异的结果。然而,它通常需要大量带注释的训练数据集,这在临床环境中限制了其可扩展性。为了解决这一挑战,半监督学习是一种利用有标签和无标签数据的方法。在本文中,我们介绍了一种受生成模型最新成功启发的生物医学图像分割的半监督教师-学生框架。我们的方法利用去噪扩散概率模型(DDPM)逐步细化基于相应图像的噪声输入,以生成分割掩膜。教师模型首先使用基于噪声损坏图像重建的循环一致性约束进行无监督训练,使其能够生成信息丰富的语义掩膜。随后,教师被集成到一个与学生网络共同训练的过程中。当学生可以使用真实标签进行学习时,他们就从真实标签中学习;否则,他们就由教师生成的伪标签进行学习,而教师则不断改善其伪标签生成能力。最后,为了进一步提高性能,我们引入了一种多轮伪标签生成策略,该策略通过迭代改进伪标签生成过程。我们在多个生物医学成像基准测试上对我们的方法进行了评估,涵盖了多种成像模式和分割任务。实验结果表明,我们的方法始终优于最新的半监督技术,突显了其在有限标注数据场景中的有效性。我们的实验代码可在 https://github.com/ciampluca/diffusion_semi_supervised_biomedical_image_segmentation 找到。
论文及项目相关链接
Summary
本文介绍了一种基于半监督教师-学生框架的生物医学图像分割方法,该方法利用去噪扩散概率模型(DDPMs)生成分割掩膜,通过逐步细化噪声输入并基于相应图像进行条件化。教师模型首先通过无监督方式训练,使用基于噪声破坏图像重建的循环一致性约束来生成语义掩膜。随后,教师模型与双学生网络进行协同训练过程。学生模型在有真实标签时学习,没有时则依靠教师生成的伪标签。为进一步提高性能,引入多轮伪标签生成策略,迭代改进伪标签过程。在多个生物医学成像基准测试上的实验结果表明,该方法在有限标注数据场景下表现优异,优于其他先进的半监督技术。
Key Takeaways
- 监督深度学习在医学图像分割中表现优秀,但需大量标注数据,限制了其在临床环境中的可扩展性。
- 半监督学习利用标注和非标注数据,是解决这个问题的一种途径。
- 本文提出了一种基于教师-学生框架的半监督生物医学图像分割方法,利用去噪扩散概率模型(DDPMs)生成分割掩膜。
- 教师模型通过无监督方式训练,使用循环一致性约束生成语义掩膜。
- 学生模型从真实标签和伪标签中学习,教师模型不断提高其伪标签生成能力。
- 引入多轮伪标签生成策略,提高性能。
点此查看论文截图
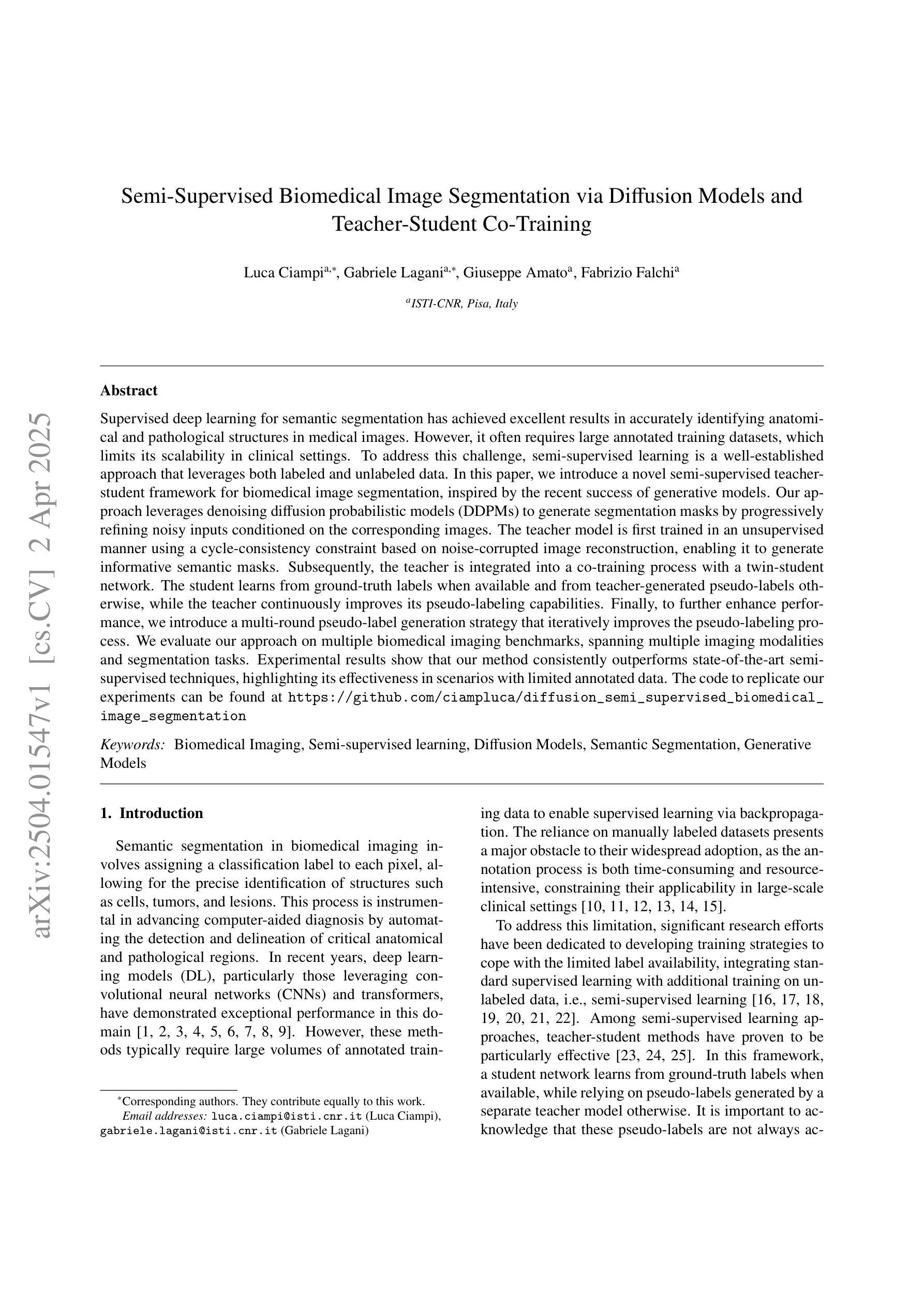
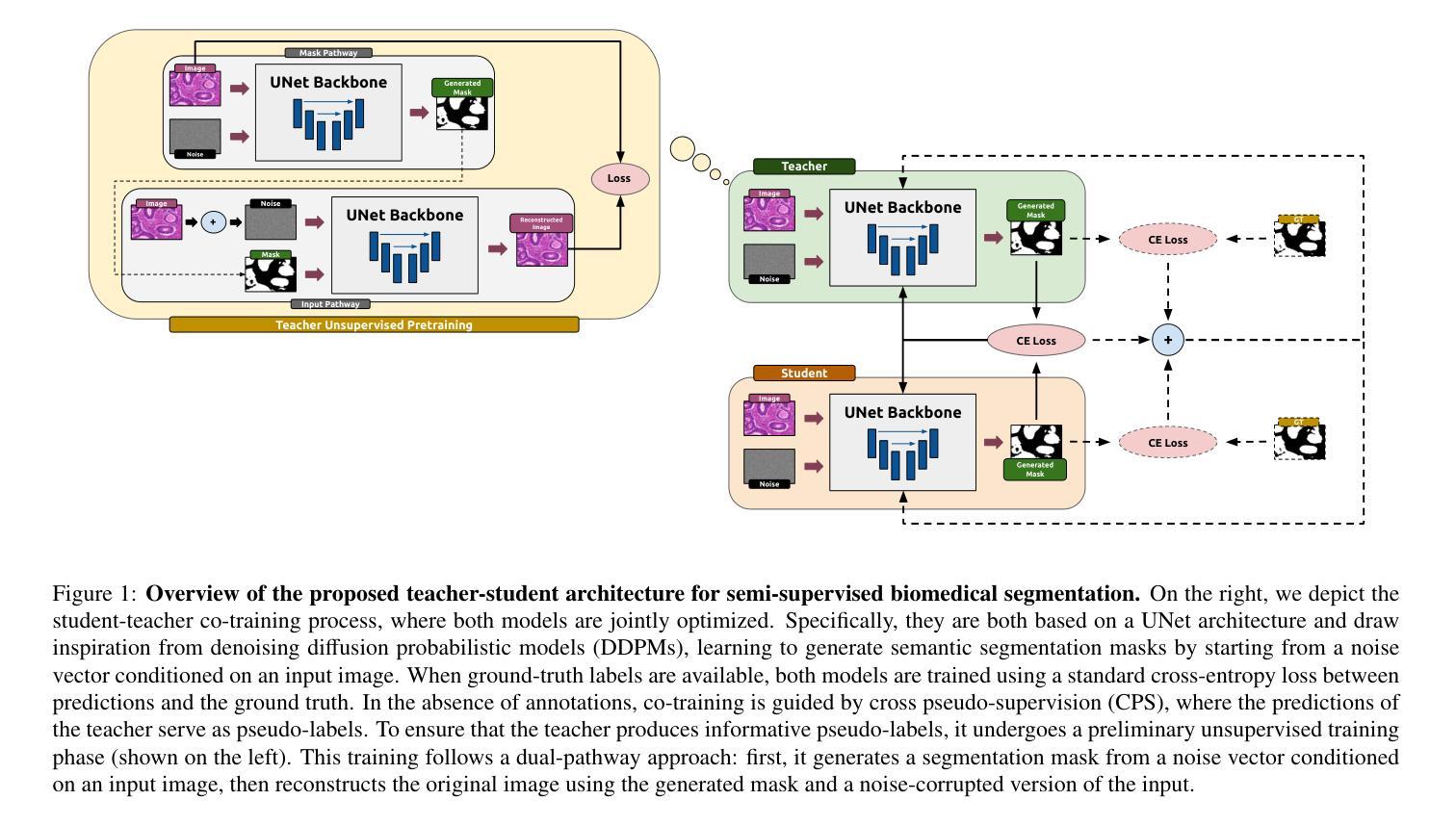
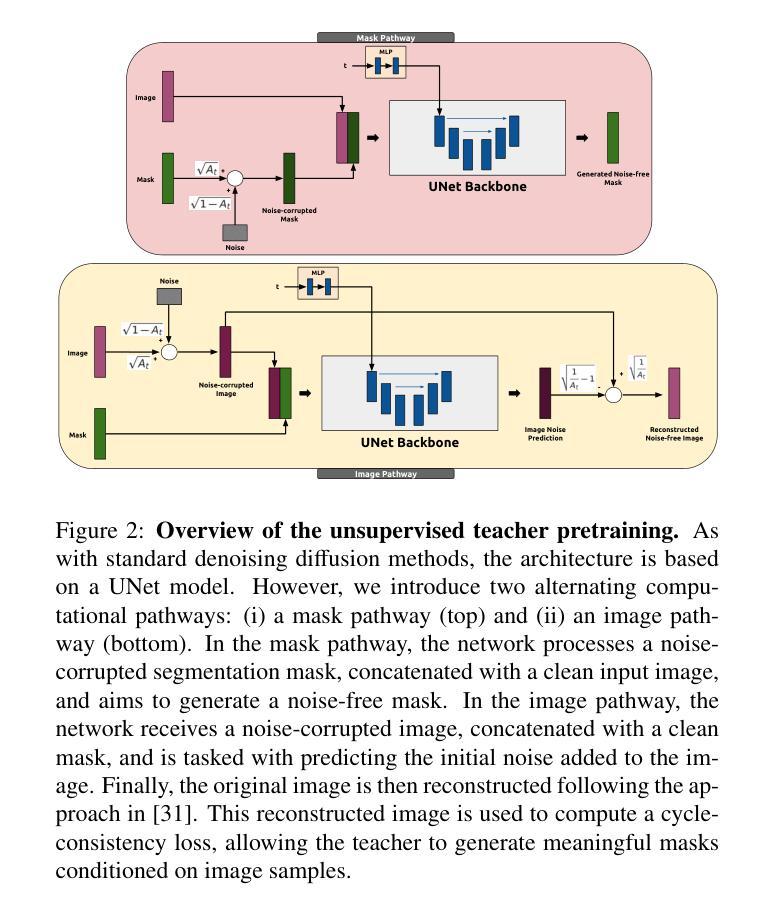
FUSION: Frequency-guided Underwater Spatial Image recOnstructioN
Authors:Jaskaran Singh Walia, Shravan Venkatraman, Pavithra LK
Underwater images suffer from severe degradations, including color distortions, reduced visibility, and loss of structural details due to wavelength-dependent attenuation and scattering. Existing enhancement methods primarily focus on spatial-domain processing, neglecting the frequency domain’s potential to capture global color distributions and long-range dependencies. To address these limitations, we propose FUSION, a dual-domain deep learning framework that jointly leverages spatial and frequency domain information. FUSION independently processes each RGB channel through multi-scale convolutional kernels and adaptive attention mechanisms in the spatial domain, while simultaneously extracting global structural information via FFT-based frequency attention. A Frequency Guided Fusion module integrates complementary features from both domains, followed by inter-channel fusion and adaptive channel recalibration to ensure balanced color distributions. Extensive experiments on benchmark datasets (UIEB, EUVP, SUIM-E) demonstrate that FUSION achieves state-of-the-art performance, consistently outperforming existing methods in reconstruction fidelity (highest PSNR of 23.717 dB and SSIM of 0.883 on UIEB), perceptual quality (lowest LPIPS of 0.112 on UIEB), and visual enhancement metrics (best UIQM of 3.414 on UIEB), while requiring significantly fewer parameters (0.28M) and lower computational complexity, demonstrating its suitability for real-time underwater imaging applications.
水下图像受到严重的退化影响,包括色彩失真、能见度降低以及由于波长相关的衰减和散射导致的结构细节损失。现有的增强方法主要集中在空间域处理上,忽略了频率域在捕捉全局颜色分布和长距离依赖方面的潜力。为了解决这些局限性,我们提出了FUSION,这是一个双域深度学习框架,它联合利用空间域和频率域信息。FUSION在空间域中通过多尺度卷积核和自适应注意力机制独立处理每个RGB通道,同时利用基于FFT的频率注意力提取全局结构信息。频率引导融合模块整合两个域中的互补特征,然后进行跨通道融合和自适应通道再校准,以确保颜色分布的平衡。在UIEB、EUVP和SUIM-E等基准数据集上的大量实验表明,FUSION达到了最先进的性能,在重建保真度、感知质量和视觉增强指标方面均优于现有方法(在UIEB上最高PSNR为23.717 dB,SSIM为0.883;在UIEB上最低LPIPS为0.112;在UIEB上最佳UIQM为3.414),同时所需参数大大减少(仅0.28M)且计算复杂度较低,显示出其适用于实时水下成像应用。
论文及项目相关链接
Summary
本文提出一种名为FUSION的深度学习框架,该框架结合空间域和频域信息,针对水下图像的颜色失真、可见度降低和结构细节丢失等问题进行增强处理。通过多尺度卷积核和自适应注意力机制在空间域独立处理每个RGB通道,同时利用FFT-based频率注意力提取全局结构信息。实验证明,FUSION在基准数据集上实现了最佳性能,具有较少的参数和较低的计算复杂度,适合实时水下成像应用。
Key Takeaways
- 水下图像存在严重的失真问题,包括颜色失真、可见度降低和结构细节丢失。
- 现有增强方法主要关注空间域处理,忽略了频域在捕捉全局颜色分布和长距离依赖方面的潜力。
- FUSION框架结合空间域和频域信息,通过多尺度卷积核和自适应注意力机制处理水下图像。
- FUSION利用FFT-based频率注意力提取全局结构信息,并通过频率引导融合模块整合两个领域的特征。
- FUSION在多个基准数据集上实现了最佳性能,包括UIEB、EUVP和SUIM-E。
- FUSION在重建保真度、感知质量、视觉增强指标方面均表现出卓越性能。
点此查看论文截图
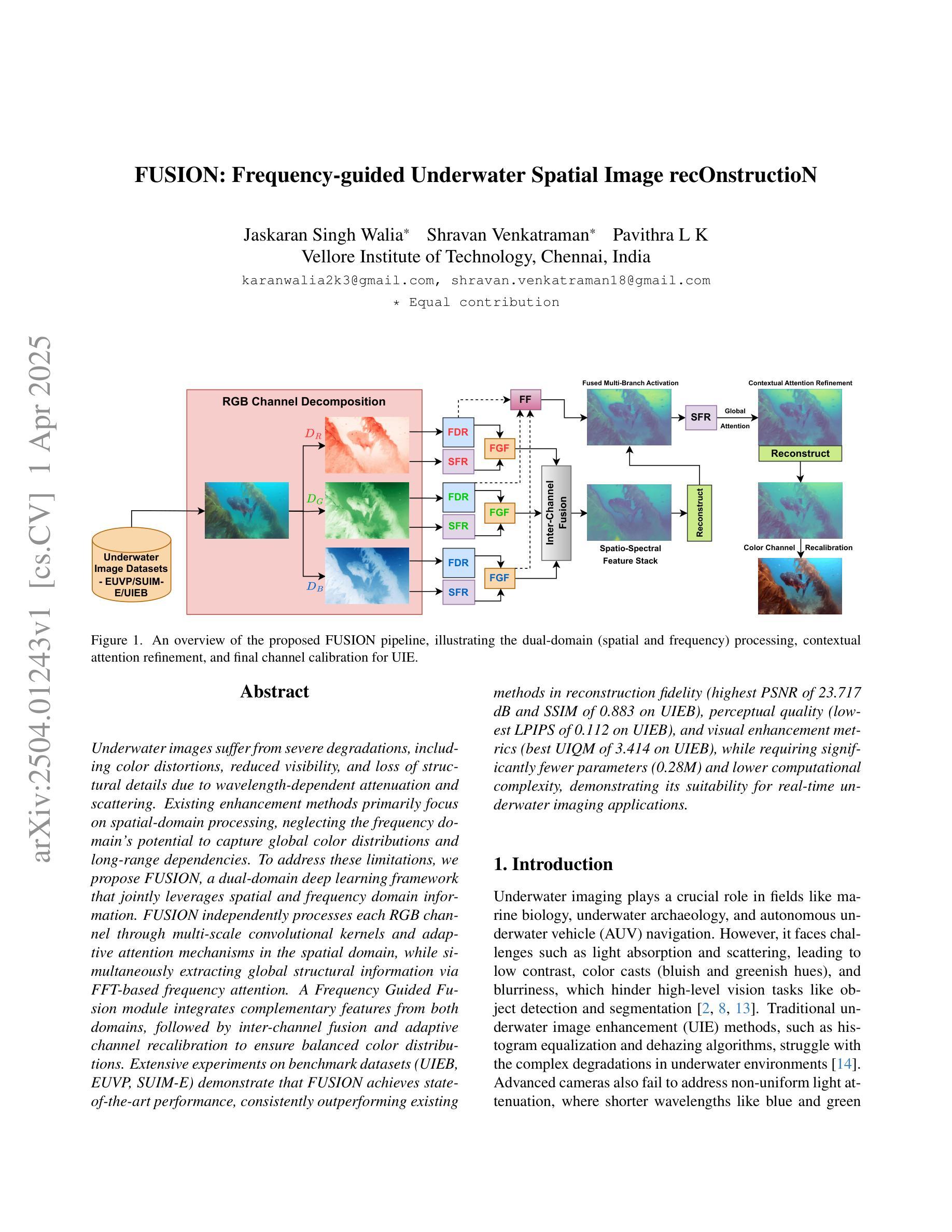
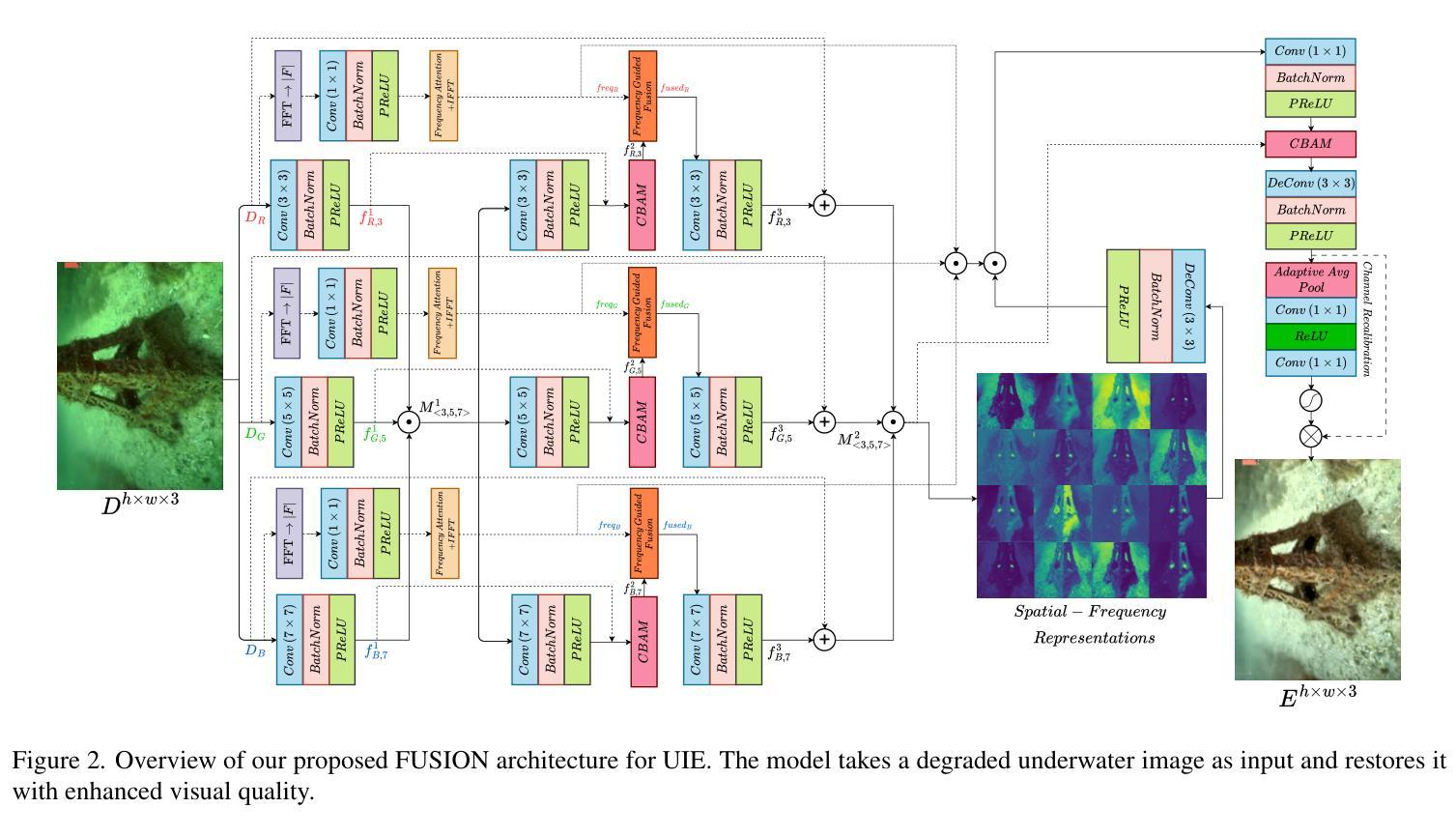
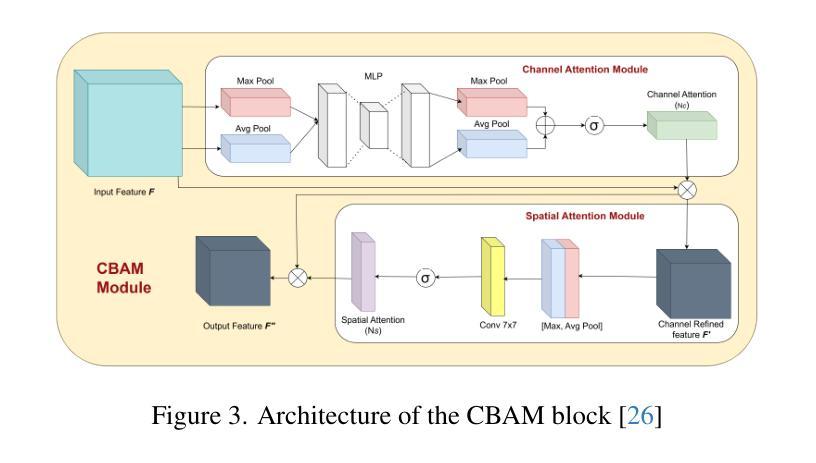
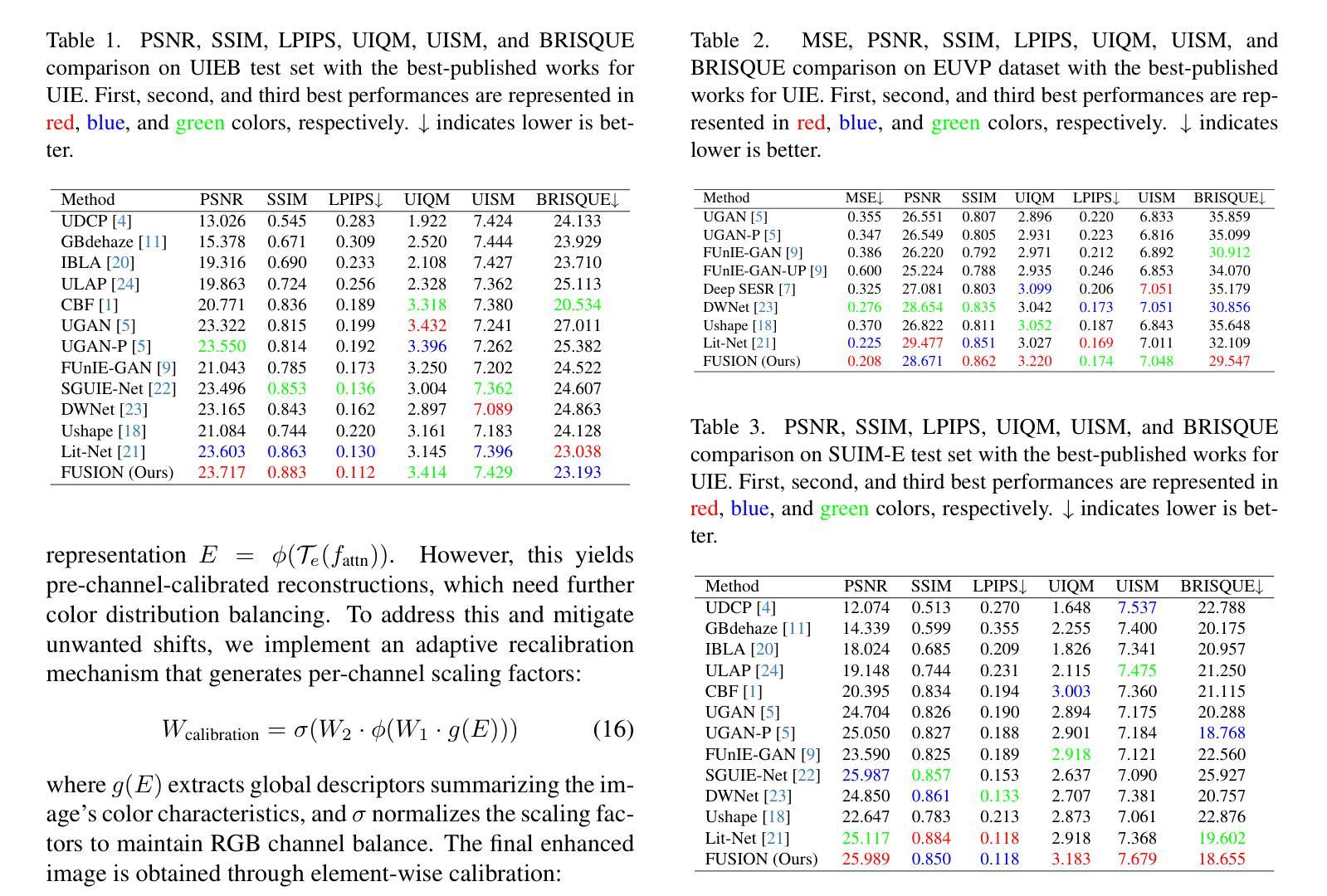
An Integrated AI-Enabled System Using One Class Twin Cross Learning (OCT-X) for Early Gastric Cancer Detection
Authors:Xian-Xian Liu, Yuanyuan Wei, Mingkun Xu, Yongze Guo, Hongwei Zhang, Huicong Dong, Qun Song, Qi Zhao, Wei Luo, Feng Tien, Juntao Gao, Simon Fong
Early detection of gastric cancer, a leading cause of cancer-related mortality worldwide, remains hampered by the limitations of current diagnostic technologies, leading to high rates of misdiagnosis and missed diagnoses. To address these challenges, we propose an integrated system that synergizes advanced hardware and software technologies to balance speed-accuracy. Our study introduces the One Class Twin Cross Learning (OCT-X) algorithm. Leveraging a novel fast double-threshold grid search strategy (FDT-GS) and a patch-based deep fully convolutional network, OCT-X maximizes diagnostic accuracy through real-time data processing and seamless lesion surveillance. The hardware component includes an all-in-one point-of-care testing (POCT) device with high-resolution imaging sensors, real-time data processing, and wireless connectivity, facilitated by the NI CompactDAQ and LabVIEW software. Our integrated system achieved an unprecedented diagnostic accuracy of 99.70%, significantly outperforming existing models by up to 4.47%, and demonstrated a 10% improvement in multirate adaptability. These findings underscore the potential of OCT-X as well as the integrated system in clinical diagnostics, offering a path toward more accurate, efficient, and less invasive early gastric cancer detection. Future research will explore broader applications, further advancing oncological diagnostics. Code is available at https://github.com/liu37972/Multirate-Location-on-OCT-X-Learning.git.
胃癌是全球癌症相关死亡的主要原因之一,其早期检测仍受到当前诊断技术局限的阻碍,导致误诊和漏诊率较高。为了应对这些挑战,我们提出了一种协同先进硬件和软件技术的集成系统,以平衡速度和准确性。本研究介绍了单类双交叉学习(OCT-X)算法。该算法利用新型快速双阈值网格搜索策略(FDT-GS)和基于补丁的深度全卷积网络,通过实时数据处理和无缝病变监测,最大限度地提高诊断准确性。硬件组件包括具有高清成像传感器、实时数据处理和无线连接的一体化即时检测(POCT)设备,由NI CompactDAQ和LabVIEW软件提供支持。我们的集成系统取得了前所未有的99.70%的诊断准确率,比现有模型高出4.47%,并在多速率适应性方面提高了10%。这些发现强调了OCT-X及其在临床诊断中的集成系统的潜力,为更准确、高效和非侵入性的早期胃癌检测提供了途径。未来的研究将探索更广泛的应用,进一步推动肿瘤诊断的进步。相关代码可在https://github.com/liu37972/Multirate-Location-on-OCT-X-Learning.git上找到。
论文及项目相关链接
PDF 26 pages, 4 figures, 6 tables
Summary
本文提出一种集成系统,结合了先进的软硬件技术,以提高胃癌早期诊断的准确性和速度。研究中引入了One Class Twin Cross Learning(OCT-X)算法,通过快速双阈值网格搜索策略(FDT-GS)和基于补丁的深度全卷积网络,最大化诊断准确性,并实现实时数据处理和无缝病变监测。硬件组件包括具有高清成像传感器、实时数据处理和无线连接的一体化即时检测(POCT)设备,软件支持NI CompactDAQ和LabVIEW。该集成系统实现了99.70%的诊断准确性,较现有模型提高了高达4.47%,并在多率适应性方面提高了10%。这表明OCT-X及集成系统在临床诊疗中的潜力,可更准确、高效、侵入性较小地检测早期胃癌。未来的研究将探索更广泛的应用,进一步推进肿瘤诊断。
Key Takeaways
- 当前胃癌诊断技术存在局限性,导致高误诊率。
- 提出了一个集成系统,结合先进软硬件技术,提高诊断速度和准确性。
- 引入了One Class Twin Cross Learning(OCT-X)算法,通过FDT-GS和基于补丁的深度学习网络,优化诊断。
- 系统中包含高清成像传感器、实时数据处理和无线连接的POCT设备。
- 系统实现了99.70%的诊断准确性,较现有技术有显著改进。
- 系统在多率适应性方面提高10%,显示其在不同情境下的稳健性。
点此查看论文截图
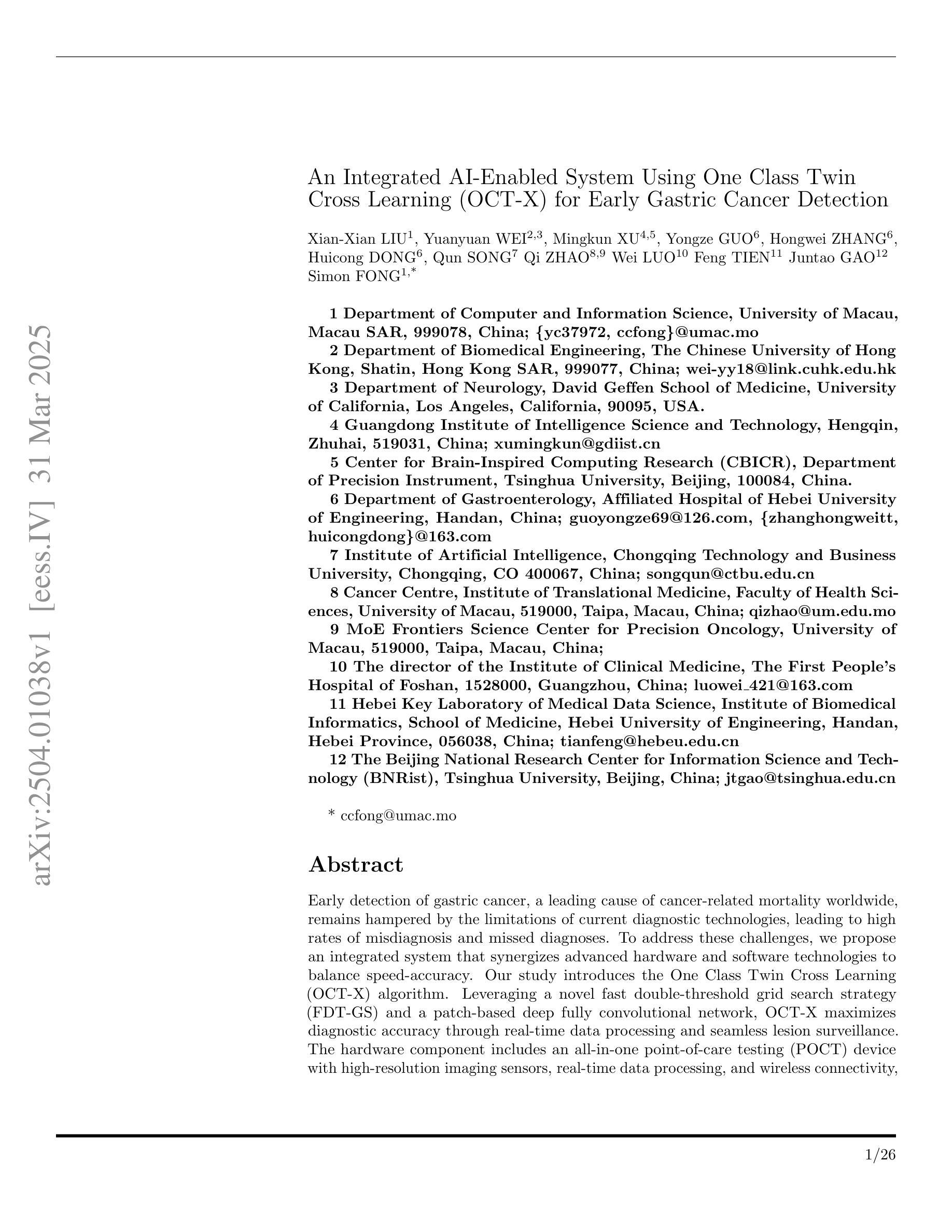
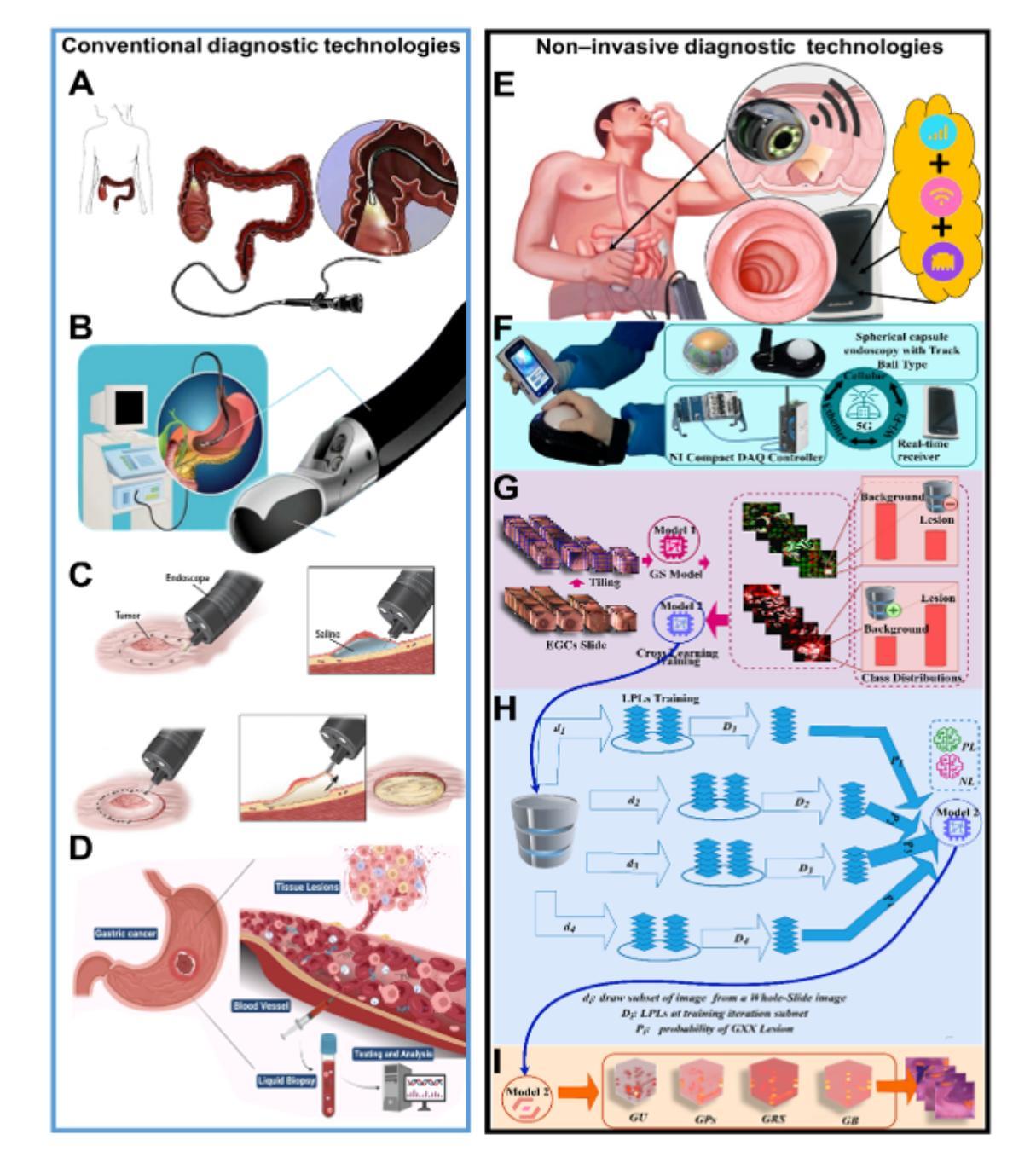
GKAN: Explainable Diagnosis of Alzheimer’s Disease Using Graph Neural Network with Kolmogorov-Arnold Networks
Authors:Tianqi Ding, Dawei Xiang, Keith E Schubert, Liang Dong
Alzheimer’s Disease (AD) is a progressive neurodegenerative disorder that poses significant diagnostic challenges due to its complex etiology. Graph Convolutional Networks (GCNs) have shown promise in modeling brain connectivity for AD diagnosis, yet their reliance on linear transformations limits their ability to capture intricate nonlinear patterns in neuroimaging data. To address this, we propose GCN-KAN, a novel single-modal framework that integrates Kolmogorov-Arnold Networks (KAN) into GCNs to enhance both diagnostic accuracy and interpretability. Leveraging structural MRI data, our model employs learnable spline-based transformations to better represent brain region interactions. Evaluated on the Alzheimer’s Disease Neuroimaging Initiative (ADNI) dataset, GCN-KAN outperforms traditional GCNs by 4-8% in classification accuracy while providing interpretable insights into key brain regions associated with AD. This approach offers a robust and explainable tool for early AD diagnosis.
阿尔茨海默症(AD)是一种进展性的神经退行性疾病,由于其复杂的病因,给诊断带来了很大的挑战。图卷积网络(GCNs)在AD诊断的脑连接建模中显示出潜力,但它们对线性转换的依赖限制了捕捉神经成像数据中复杂非线性模式的能力。为了解决这一问题,我们提出了GCN-KAN这一新型单模态框架,它将Kolmogorov-Arnold网络(KAN)集成到GCNs中,以提高诊断准确性和可解释性。我们的模型利用结构磁共振成像数据,采用可学习的基于样条的转换来更好地表示脑区相互作用。在阿尔茨海默症神经影像学倡议(ADNI)数据集上评估的GCN-KAN,在分类准确率上比传统GCNs高出4-8%,同时提供了关于与AD相关的关键脑区的可解释见解。这种方法为早期AD诊断提供了稳健和可解释的工具。
论文及项目相关链接
PDF 12 pages, 4 figures, under review of The Southwest Data Science Conference (SDSC 2025)
Summary
针对阿尔茨海默病(AD)诊断的挑战,提出一种结合图卷积网络(GCN)和柯尔莫哥洛夫-阿诺尔德网络(KAN)的新型单模态框架GCN-KAN。通过结构磁共振成像数据,该模型采用可学习的样条基变换,更好地表示脑区交互,提高诊断准确性和可解释性,为早期AD诊断提供稳健且可解释的工具。
Key Takeaways
- 阿尔茨海默病(AD)是一种进展性的神经退行性疾病,诊断具有挑战性。
- 图卷积网络(GCN)在AD诊断的脑连接建模中展现出潜力,但受限于对非线性模式的捕捉能力。
- 提出GCN-KAN框架,整合柯尔莫哥洛夫-阿诺尔德网络(KAN)以增强诊断准确性和可解释性。
- GCN-KAN框架使用结构磁共振成像数据,并采用可学习的样条基变换,更好地表示脑区交互。
- 在阿尔茨海默病神经影像学倡议(ADNI)数据集上评估,GCN-KAN相比传统GCN在分类准确度上提高了4-8%。
- GCN-KAN不仅能提高诊断准确性,还能提供关于AD相关关键脑区的可解释见解。
点此查看论文截图
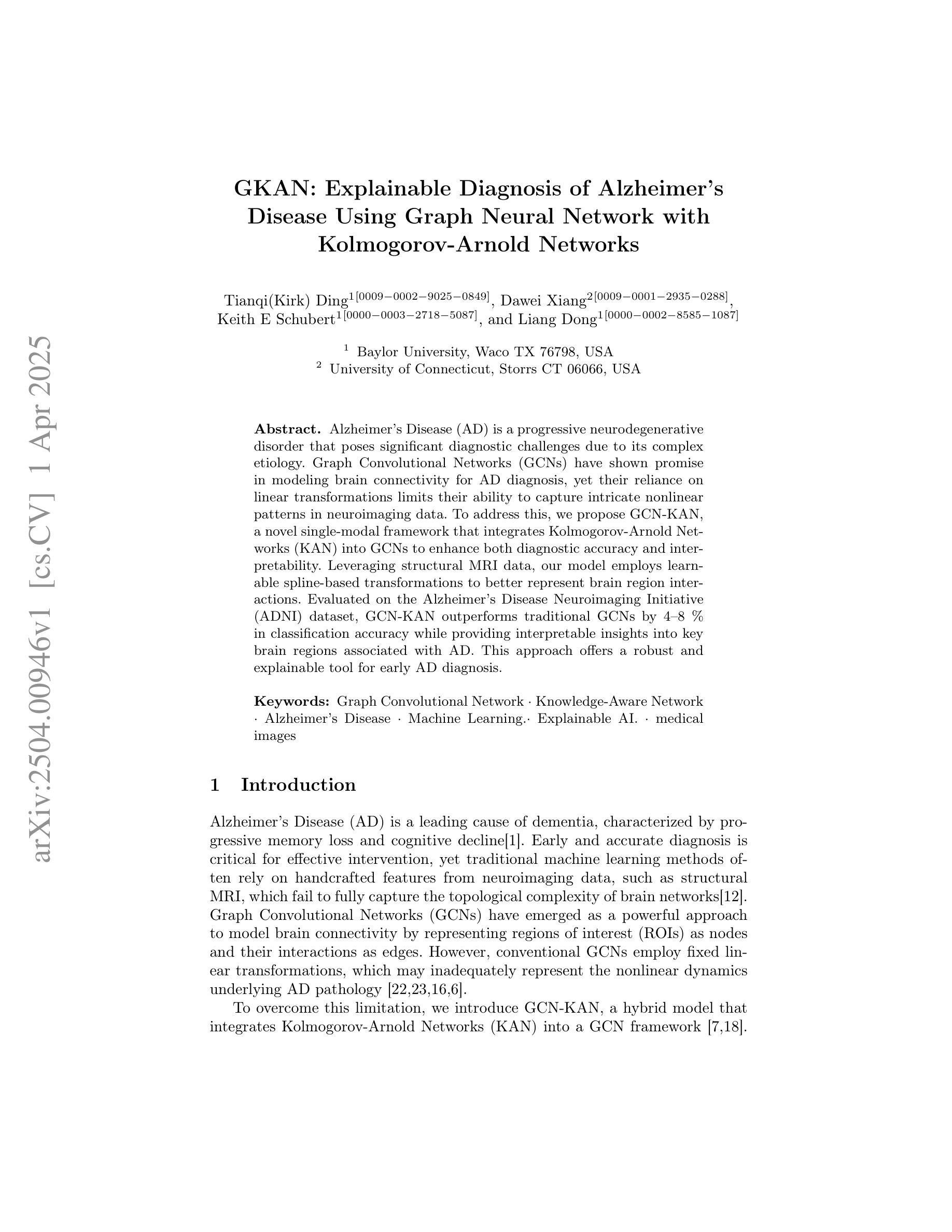
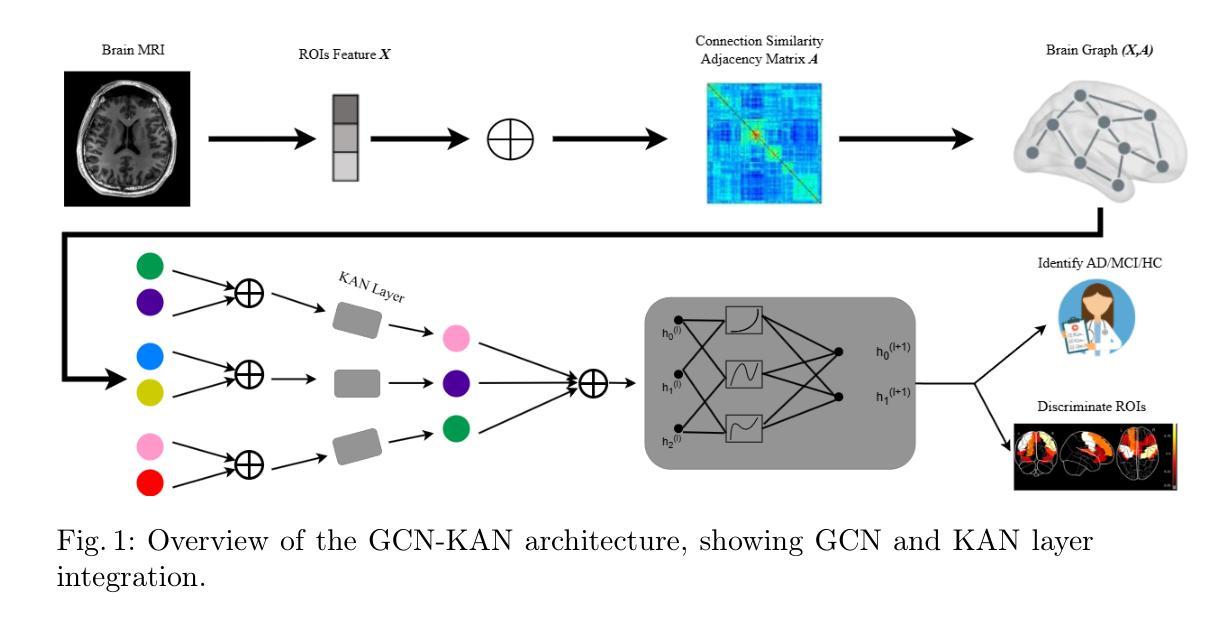
Graph Classification and Radiomics Signature for Identification of Tuberculous Meningitis
Authors:Snigdha Agarwal, Ganaraja V H, Neelam Sinha, Abhilasha Indoria, Netravathi M, Jitender Saini
Introduction: Tuberculous meningitis (TBM) is a serious brain infection caused by Mycobacterium tuberculosis, characterized by inflammation of the meninges covering the brain and spinal cord. Diagnosis often requires invasive lumbar puncture (LP) and cerebrospinal fluid (CSF) analysis. Objectives: This study aims to classify TBM patients using T1-weighted (T1w) non-contrast Magnetic Resonance Imaging (MRI) scans. We hypothesize that specific brain regions, such as the interpeduncular cisterns, bone, and corpus callosum, contain visual markers that can non-invasively distinguish TBM patients from healthy controls. We propose a novel Pixel-array Graphs Classifier (PAG-Classifier) that leverages spatial relationships between neighbouring 3D pixels in a graph-based framework to extract significant features through eigen decomposition. These features are then used to train machine learning classifiers for effective patient classification. We validate our approach using a radiomics-based methodology, classifying TBM patients based on relevant radiomics features. Results: We utilized an internal dataset consisting of 52 scans, 32 from confirmed TBM patients based on mycobacteria detection in CSF, and 20 from healthy individuals. We achieved a 5-fold cross-validated average F1 score of 85.71% for cistern regions with our PAG-Classifier and 92.85% with the radiomics features classifier, surpassing current state-of-the-art benchmarks by 15% and 22%, respectively. However, bone and corpus callosum regions showed poor classification effectiveness, with average F1 scores below 50%. Conclusion: Our study suggests that algorithms like the PAG-Classifier serve as effective tools for non-invasive TBM analysis, particularly by targeting the interpeduncular cistern. Findings indicate that the bone and corpus callosum regions lack distinctive patterns for differentiation.
引言:结核性脑膜炎(TBM)是由结核分枝杆菌引起的一种严重的大脑感染,其特征在于大脑和脊髓的脑膜发炎。诊断通常需要侵入性的腰椎穿刺(LP)和脑脊液(CSF)分析。目标:本研究旨在利用T1加权(T1w)非对比磁共振成像(MRI)扫描对TBM患者进行分类。我们假设特定的脑区,如脚间池、骨骼和胼胝体,存在视觉标记,可以无创地区分TBM患者和健康对照者。我们提出了一种新型的像素阵列图分类器(PAG-Classifier),它利用基于图的框架中相邻3D像素之间的空间关系,通过特征分解提取重要特征。然后,这些特征被用来训练机器学习分类器,以实现有效的患者分类。我们使用基于放射组学的方法验证我们的方法,根据相关的放射学特征对TBM患者进行分类。结果:我们使用内部数据集,包括52次扫描,其中32次来自经脑脊液中细菌检测确认的TBM患者,以及20次来自健康个体的扫描。使用我们的PAG-Classifier,在池区域实现了平均F1分数为85.71%的5倍交叉验证,使用放射学特征分类器达到了92.85%,分别比当前最新技术高出15%和22%。然而,骨骼和胼胝体区域的分类效果较差,平均F1分数低于50%。结论:我们的研究表明,像PAG-Classifier这样的算法可以作为无创TBM分析的有效工具,特别是通过针对脚间池进行分析。研究结果表明,骨骼和胼胝体区域缺乏明显的差异模式来进行区分。
论文及项目相关链接
PDF 19 pages, 6 figures, 3 tables
摘要
结核性脑膜炎(TBM)是由结核分枝杆菌引起的一种严重的脑部感染,表现为脑和脊髓膜炎症。本研究旨在利用T1加权(T1w)非对比磁共振成像(MRI)扫描对TBM患者进行分类。研究假设特定脑区如脚间池、骨骼和胼胝体包含视觉标记,能无创地区分TBM患者和健康对照者。研究提出了一种新的像素阵列图分类器(PAG-Classifier),它利用图框架中相邻3D像素的空间关系来提取特征,并通过特征分解进行训练机器学习分类器,以有效地对患者进行分类。研究使用基于放射组学的方法验证其方法的有效性。结果:研究使用了由52次扫描组成的内部数据集,其中包括根据脑脊液中结核杆菌检测结果确诊的32名TBM患者和20名健康个体。使用PAG-Classifier在池区域的5倍交叉验证平均F1分数为85.71%,使用放射学特征分类器的F1分数为92.85%,分别比目前最先进的基准高出15%和22%。然而,骨骼和胼胝体区域的分类效果较差,平均F1分数低于50%。结论:本研究表明,像PAG-Classifier这样的算法可作为无创分析TBM的有效工具,特别是在针对脚间池区域时。研究结果表明,骨骼和胼胝体区域缺乏明显的模式进行区分。
要点概括
- TBM是一种由结核分枝杆菌引起的严重脑部感染。
- 本研究旨在利用T1加权非对比磁共振成像(MRI)扫描分类TBM患者。
- 研究假设特定脑区包含视觉标记,能区分TBM患者和健康者。
- 提出一种新的像素阵列图分类器(PAG-Classifier)进行无创患者分类。
- 使用放射组学方法进行验证,取得较高分类准确率。
- PAG-Classifier在池区域的分类效果较好,但在骨骼和胼胝体区域的分类效果较差。
- 研究结果支持使用算法如PAG-Classifier进行无创TBM分析。
点此查看论文截图
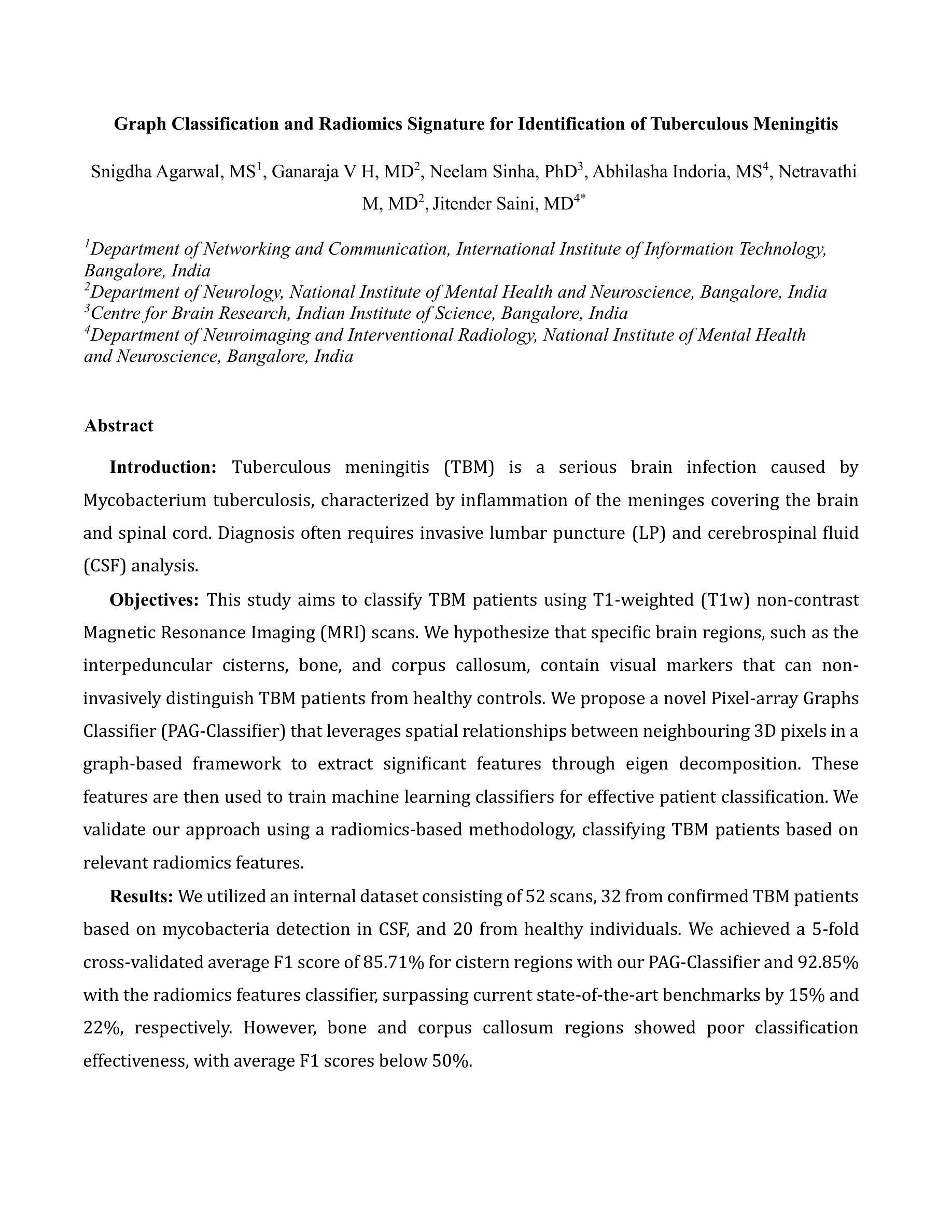
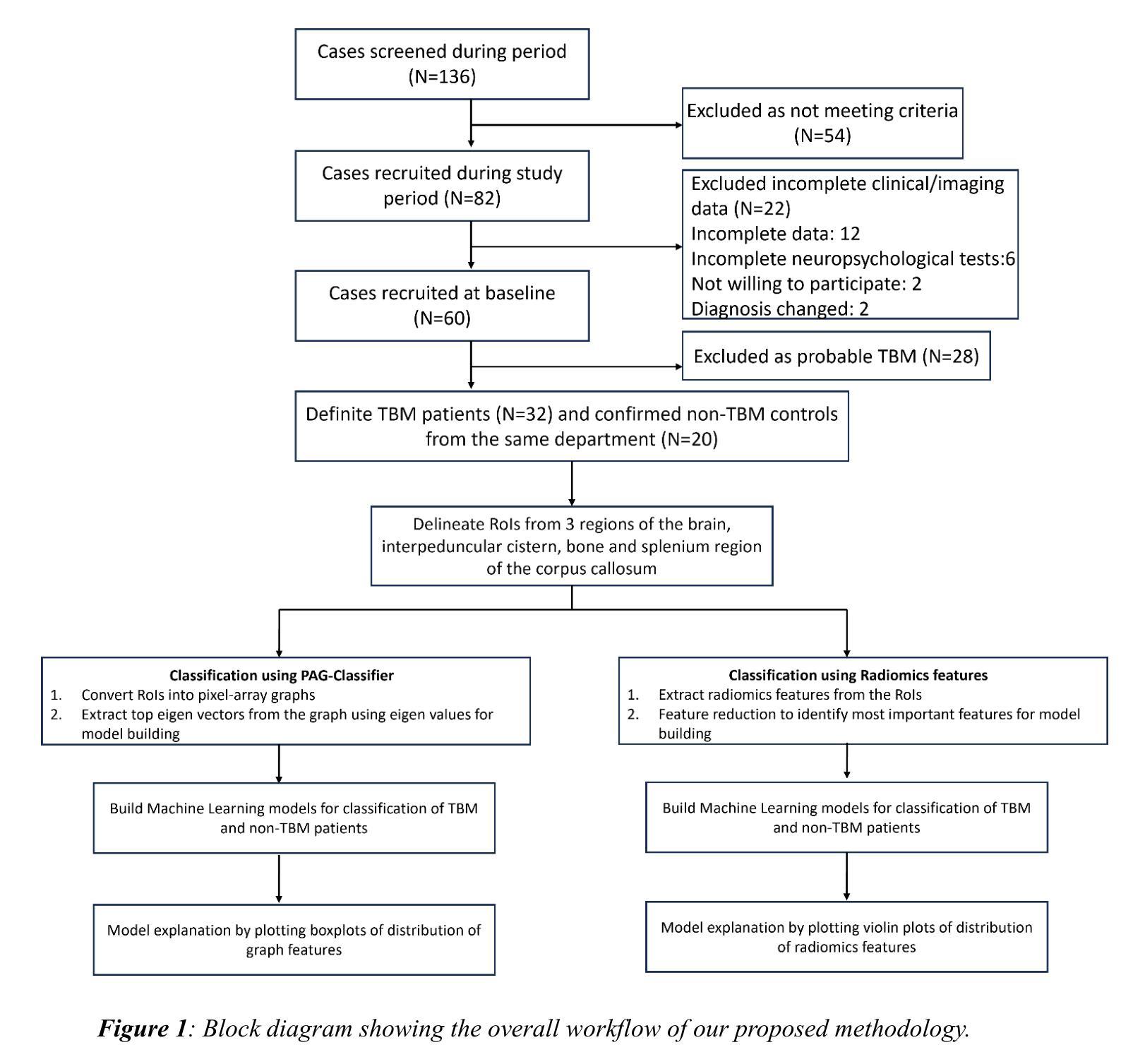
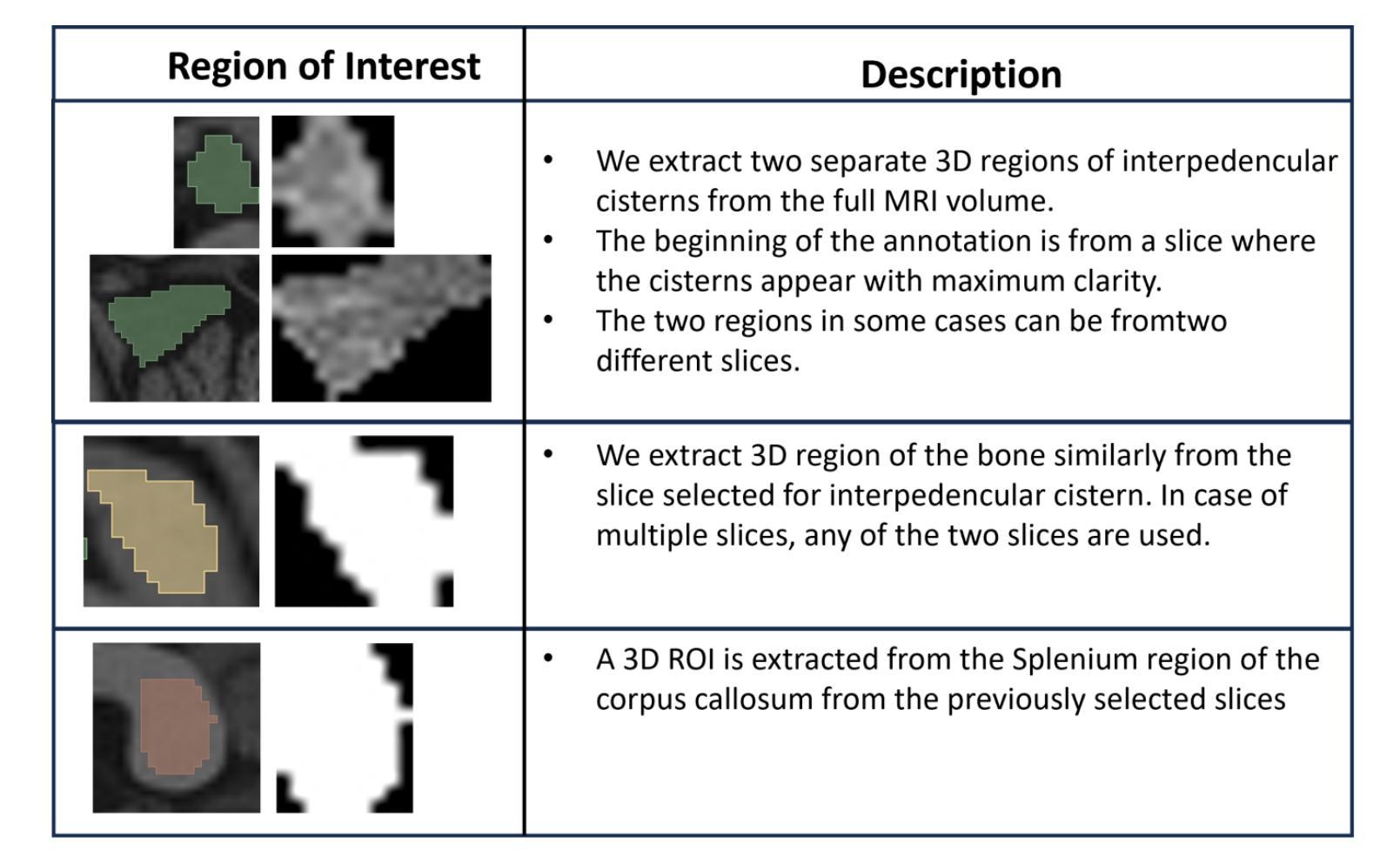
Balancing Multi-Target Semi-Supervised Medical Image Segmentation with Collaborative Generalist and Specialists
Authors:You Wang, Zekun Li, Lei Qi, Qian Yu, Yinghuan Shi, Yang Gao
Despite the promising performance achieved by current semi-supervised models in segmenting individual medical targets, many of these models suffer a notable decrease in performance when tasked with the simultaneous segmentation of multiple targets. A vital factor could be attributed to the imbalanced scales among different targets: during simultaneously segmenting multiple targets, large targets dominate the loss, leading to small targets being misclassified as larger ones. To this end, we propose a novel method, which consists of a Collaborative Generalist and several Specialists, termed CGS. It is centered around the idea of employing a specialist for each target class, thus avoiding the dominance of larger targets. The generalist performs conventional multi-target segmentation, while each specialist is dedicated to distinguishing a specific target class from the remaining target classes and the background. Based on a theoretical insight, we demonstrate that CGS can achieve a more balanced training. Moreover, we develop cross-consistency losses to foster collaborative learning between the generalist and the specialists. Lastly, regarding their intrinsic relation that the target class of any specialized head should belong to the remaining classes of the other heads, we introduce an inter-head error detection module to further enhance the quality of pseudo-labels. Experimental results on three popular benchmarks showcase its superior performance compared to state-of-the-art methods.
尽管当前半监督模型在分割单个医学目标方面表现出良好的性能,但当这些模型被要求同时分割多个目标时,其性能会出现显著下降。这背后的一个重要因素可以归结为不同目标之间的尺度不平衡:在同时分割多个目标时,大目标会主导损失,导致小目标被错误地分类为大目标。因此,我们提出了一种新方法,它由一位通用专家和几位专业人士组成,称为CGS。它以学生为中心的理念是为每个目标类别配备一名专家,从而避免了大型目标的主导地位。通用专家执行常规的多目标分割,而每个专家都致力于将其特定的目标类别与其余类别和背景区分开来。基于理论上的见解,我们证明了CGS可以实现更平衡的训练。此外,我们还开发了交叉一致性损失以促进通用专家和专业人士之间的协作学习。最后,考虑到任何专业头部的目标类别应属于其他头部的剩余类别的内在关系,我们引入了跨头部错误检测模块来进一步提高伪标签的质量。在三个流行基准测试上的实验结果表明,与最先进的方法相比,其性能卓越。
论文及项目相关链接
Summary
当前半监督模型在医学图像分割单目标任务中表现良好,但在多目标任务中存在性能下降的问题。主要是由于不同目标之间的尺度不平衡,大目标在同时分割多个目标时占据主导地位,导致小目标被误分类为大目标。为此,我们提出了一种新方法CGS(协作通才和专家系统)。该方法采用一个通用模型处理多目标分割任务,同时为每个目标类别设置一个专门的专家模型。我们提出跨一致性损失以促进协作学习,并针对专家模型的特殊类别与剩余类别之间的关系引入了交叉头误差检测模块以提高伪标签质量。在三个流行基准测试上的实验结果表明,该方法相较于现有方法具有优越性能。
Key Takeaways
- 当前半监督模型在多目标医学图像分割任务中性能下降的原因是不同目标之间的尺度不平衡。
- CGS方法旨在解决该问题,通过为每个目标类别设置专门的专家模型来避免大目标的支配地位。
- CGS中的通才模型负责多目标分割任务,而专家模型则专注于识别特定的目标类别与其他类别及背景之间的差异。
- 提出跨一致性损失以促进通才模型和专家模型之间的协作学习。
- 针对专家模型之间的特殊类别关系引入了交叉头误差检测模块以提高伪标签质量。
- CGS方法在三个流行基准测试上的实验结果表明其性能优于现有方法。
点此查看论文截图
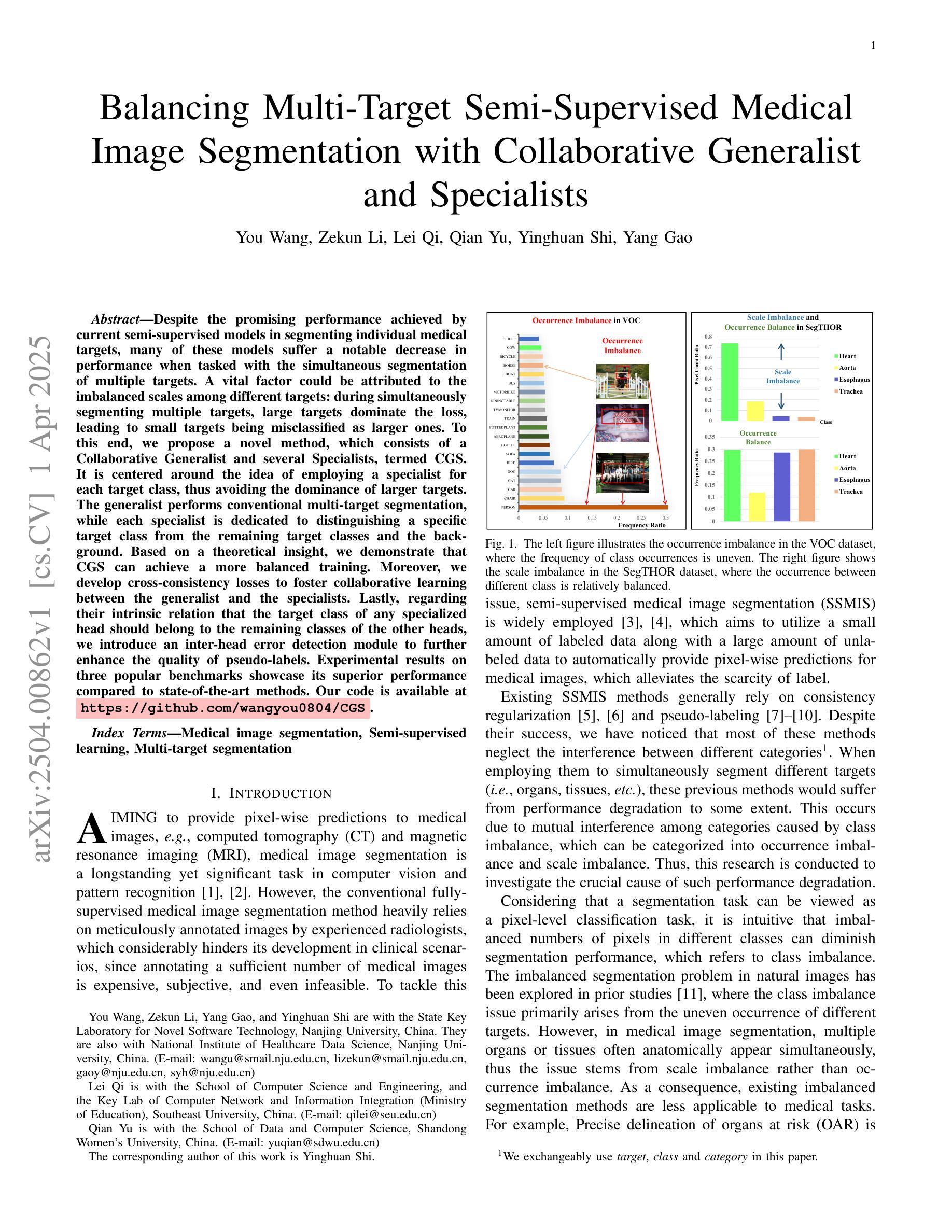
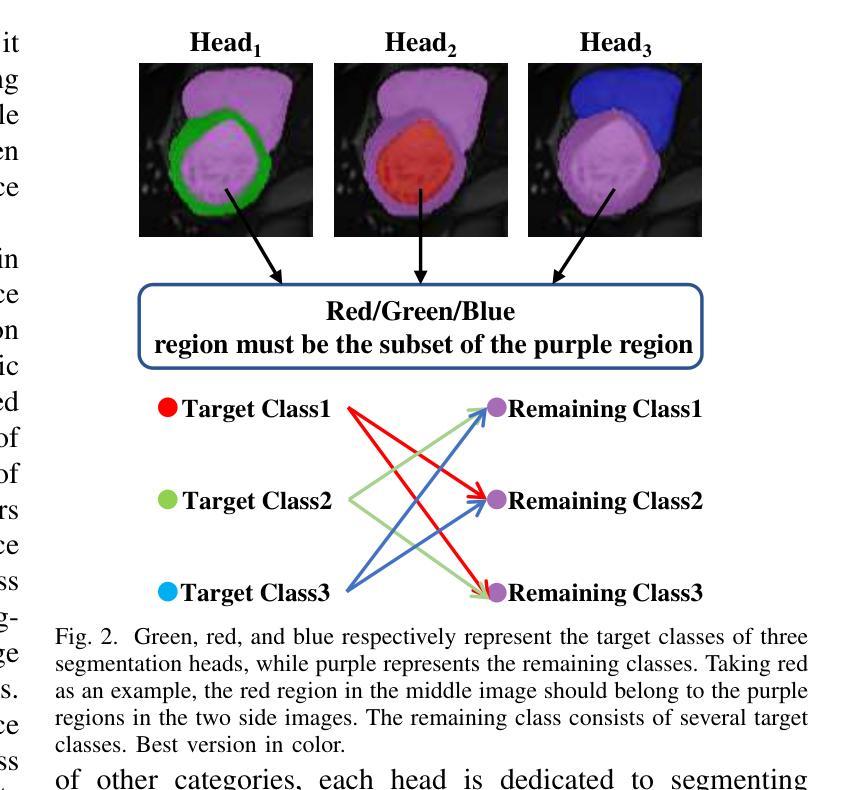
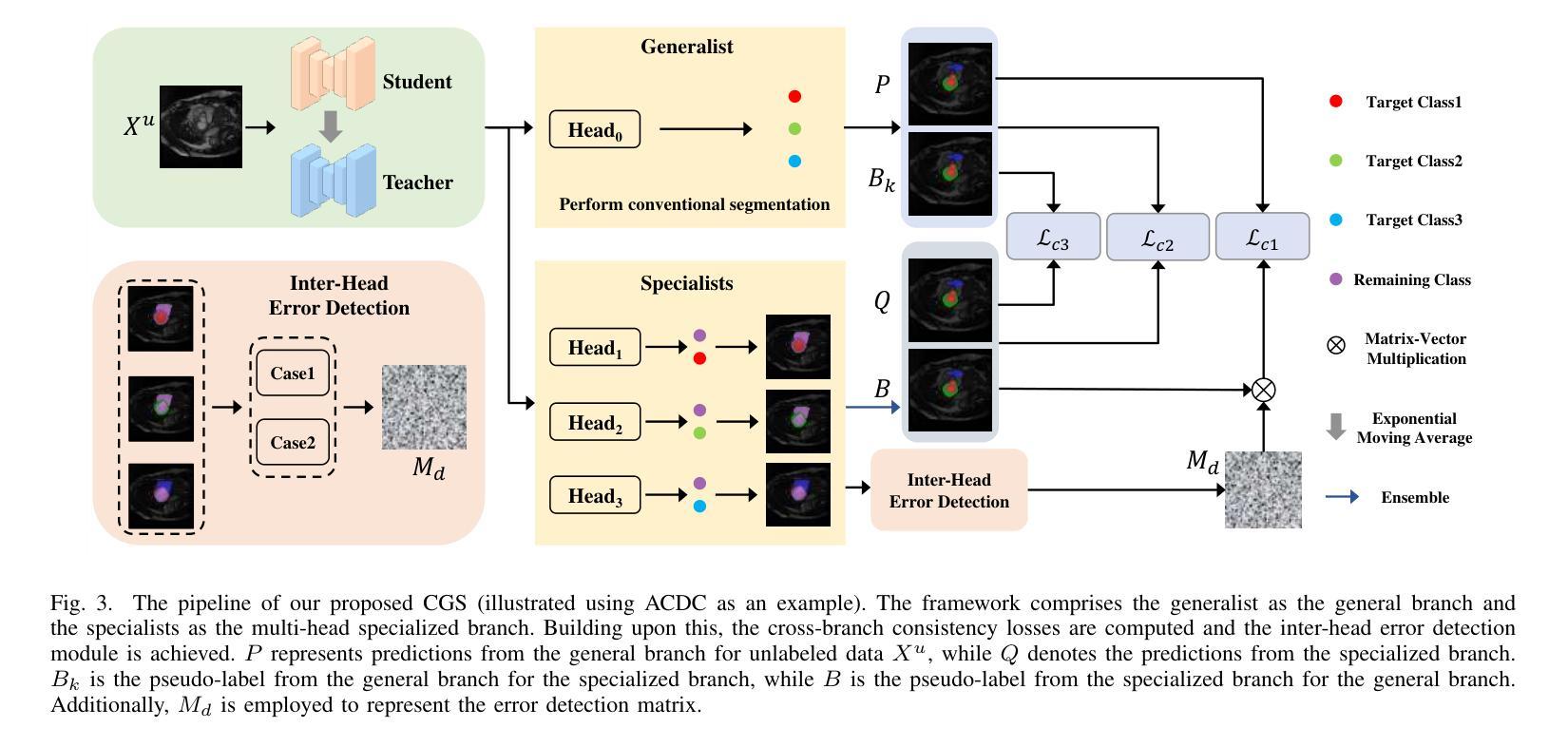
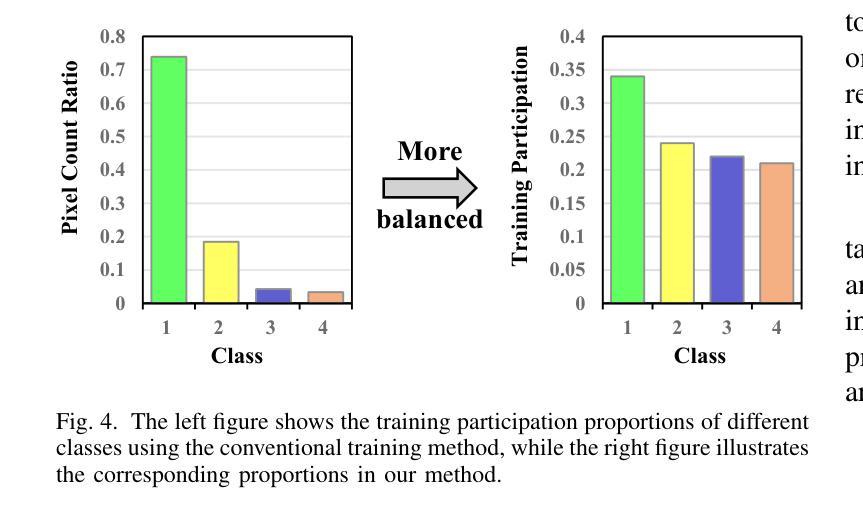
SCFANet: Style Distribution Constraint Feature Alignment Network For Pathological Staining Translation
Authors:Zetong Chen, Yuzhuo Chen, Hai Zhong, Xu Qiao
Immunohistochemical (IHC) staining serves as a valuable technique for detecting specific antigens or proteins through antibody-mediated visualization. However, the IHC staining process is both time-consuming and costly. To address these limitations, the application of deep learning models for direct translation of cost-effective Hematoxylin and Eosin (H&E) stained images into IHC stained images has emerged as an efficient solution. Nevertheless, the conversion from H&E to IHC images presents significant challenges, primarily due to alignment discrepancies between image pairs and the inherent diversity in IHC staining style patterns. To overcome these challenges, we propose the Style Distribution Constraint Feature Alignment Network (SCFANet), which incorporates two innovative modules: the Style Distribution Constrainer (SDC) and Feature Alignment Learning (FAL). The SDC ensures consistency between the generated and target images’ style distributions while integrating cycle consistency loss to maintain structural consistency. To mitigate the complexity of direct image-to-image translation, the FAL module decomposes the end-to-end translation task into two subtasks: image reconstruction and feature alignment. Furthermore, we ensure pathological consistency between generated and target images by maintaining pathological pattern consistency and Optical Density (OD) uniformity. Extensive experiments conducted on the Breast Cancer Immunohistochemical (BCI) dataset demonstrate that our SCFANet model outperforms existing methods, achieving precise transformation of H&E-stained images into their IHC-stained counterparts. The proposed approach not only addresses the technical challenges in H&E to IHC image translation but also provides a robust framework for accurate and efficient stain conversion in pathological analysis.
免疫组织化学(IHC)染色是一种通过抗体介导的可视化检测特定抗原或蛋白质的有价值的技术。然而,IHC染色过程既耗时又成本高。为了解决这些局限性,应用深度学习模型将成本效益高的苏木精-伊红(H&E)染色图像直接翻译为IHC染色图像已成为一种高效的解决方案。然而,从H&E到IHC图像的转换存在重大挑战,主要是由于图像对之间的对齐差异和IHC染色风格模式的固有多样性。为了克服这些挑战,我们提出了风格分布约束特征对齐网络(SCFANet),它包含两个创新模块:风格分布约束器(SDC)和特征对齐学习(FAL)。SDC确保生成图像和目标图像之间风格分布的一致性,同时结合循环一致性损失以保持结构一致性。为了减轻直接图像到图像翻译的复杂性,FAL模块将端到端的翻译任务分解为两个子任务:图像重建和特征对齐。此外,我们通过保持病理模式的一致性和光学密度(OD)的均匀性,确保生成图像和目标图像之间的病理一致性。在乳腺癌免疫组织化学(BCI)数据集上进行的广泛实验表明,我们的SCFANet模型优于现有方法,实现了H&E染色图像到IHC染色图像的精确转换。所提出的方法不仅解决了H&E到IHC图像转换中的技术挑战,而且为病理分析中的准确和高效染色转换提供了稳健的框架。
论文及项目相关链接
Summary
基于免疫组织化学染色在病理分析中的重要性及其时间成本和费用高昂的问题,深度学习模型被应用于将成本较低的苏木精-伊红(H&E)染色图像直接转化为免疫组织化学染色图像。本文提出了Style Distribution Constraint Feature Alignment Network(SCFANet),其中包括Style Distribution Constrainer(SDC)和Feature Alignment Learning(FAL)两个创新模块,以确保转化图像的风格分布一致性、结构一致性及病理模式一致性和光学密度均匀性。在乳腺癌免疫组织化学(BCI)数据集上的实验表明,SCFANet模型较现有方法表现更优,能精确地将H&E染色图像转化为IHC染色图像。
Key Takeaways
- 免疫组织化学染色(IHC)在病理分析中很重要,但过程耗时且成本高。
- 直接将成本较低的的苏木精-伊红(H&E)染色图像转化为免疫组织化学染色图像是有效的解决方案。
- SCFANet模型包括SDC和FAL两个模块,确保转化图像的风格、结构和病理模式的一致性。
- 在乳腺癌免疫组织化学(BCI)数据集上的实验表明,SCFANet模型表现优异。
点此查看论文截图
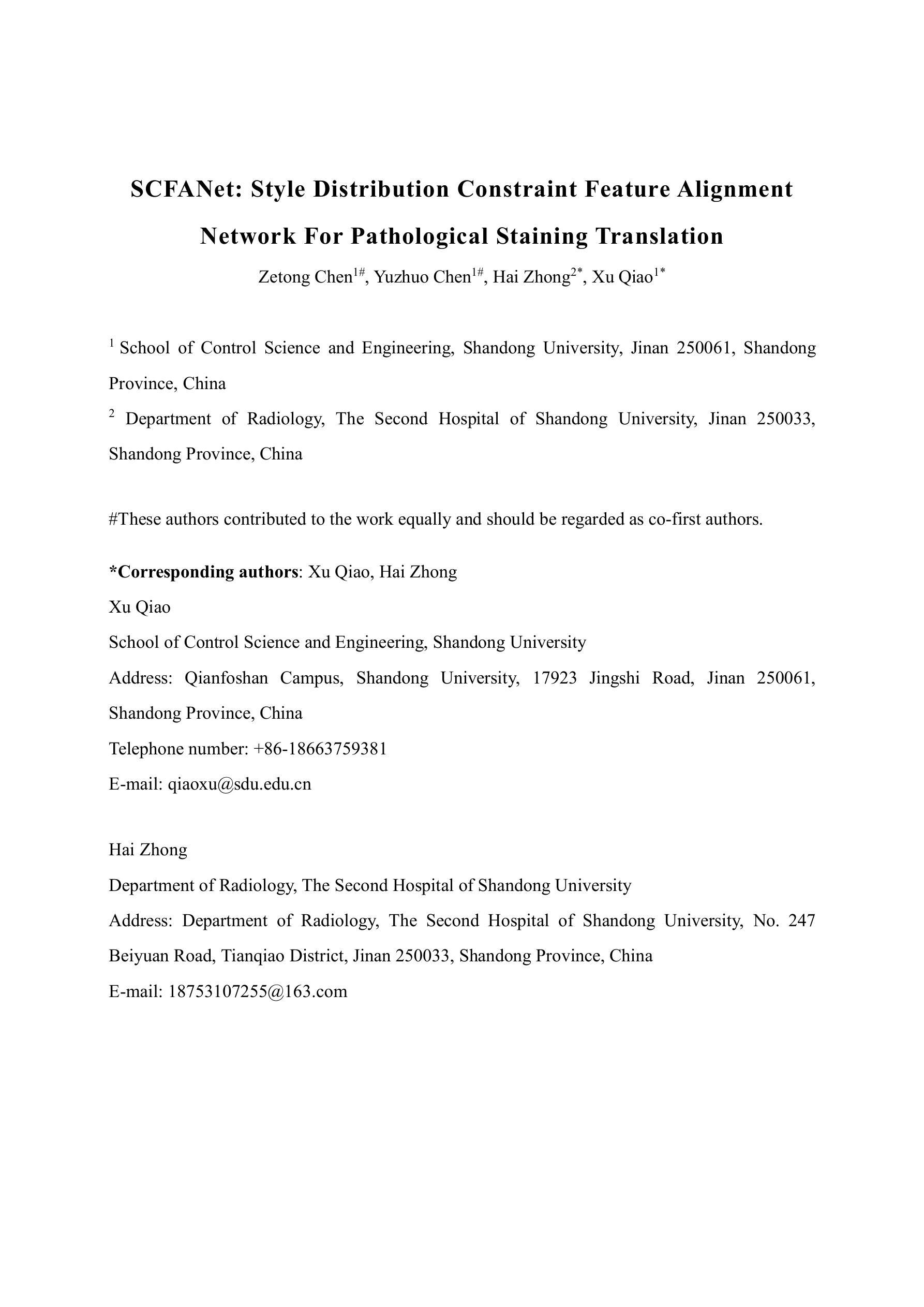
Hybrid Global-Local Representation with Augmented Spatial Guidance for Zero-Shot Referring Image Segmentation
Authors:Ting Liu, Siyuan Li
Recent advances in zero-shot referring image segmentation (RIS), driven by models such as the Segment Anything Model (SAM) and CLIP, have made substantial progress in aligning visual and textual information. Despite these successes, the extraction of precise and high-quality mask region representations remains a critical challenge, limiting the full potential of RIS tasks. In this paper, we introduce a training-free, hybrid global-local feature extraction approach that integrates detailed mask-specific features with contextual information from the surrounding area, enhancing mask region representation. To further strengthen alignment between mask regions and referring expressions, we propose a spatial guidance augmentation strategy that improves spatial coherence, which is essential for accurately localizing described areas. By incorporating multiple spatial cues, this approach facilitates more robust and precise referring segmentation. Extensive experiments on standard RIS benchmarks demonstrate that our method significantly outperforms existing zero-shot RIS models, achieving substantial performance gains. We believe our approach advances RIS tasks and establishes a versatile framework for region-text alignment, offering broader implications for cross-modal understanding and interaction. Code is available at https://github.com/fhgyuanshen/HybridGL .
在零样本图像分割(RIS)领域,最近由Segment Anything Model(SAM)和CLIP等模型推动的进展,在视觉和文本信息的对齐方面取得了重大进展。然而,尽管取得了这些成功,提取精确高质量的掩膜区域表示仍然是一个关键挑战,限制了RIS任务的全潜力。在本文中,我们介绍了一种无训练、混合全局局部特征提取方法,该方法将详细的掩膜特定特征与周围区域的上文信息相结合,增强了掩膜区域表示。为了进一步加强对掩膜区域和参照表达之间的对齐,我们提出了一种空间引导增强策略,提高了空间连贯性,这对于准确定位描述区域至关重要。通过融入多种空间线索,该方法促进了更稳健、更精确的参照分割。在标准RIS基准测试上的大量实验表明,我们的方法显著优于现有的零样本RIS模型,实现了显著的性能提升。我们相信我们的方法推动了RIS任务的发展,并建立了一个通用的区域文本对齐框架,为跨模态理解和交互提供了更广泛的启示。代码可在https://github.com/fhgyuanshen/HybridGL获取。
论文及项目相关链接
PDF accepted to CVPR2025
Summary
近期,零射击图像分割(RIS)领域在Segment Anything Model(SAM)和CLIP等模型的推动下取得了显著进展,但在生成精确高质量掩膜区域表示方面仍存在挑战,限制了RIS任务的潜力。本文提出了一种无训练、混合全局局部特征提取方法,该方法结合了掩膜特定特征与周围区域上下文信息,增强了掩膜区域表示。为进一步强化掩膜区域和引用表达之间的对齐,我们提出了空间引导增强策略,提高了空间连贯性,这对于准确定位描述区域至关重要。通过融入多种空间线索,该方法能更稳健、精确地执行引用分割。在标准RIS基准测试上的大量实验表明,我们的方法显著优于现有零射击RIS模型,实现了性能的大幅提升。我们相信,该方法推动了RIS任务的发展,为区域文本对齐建立了通用框架,对跨模态理解和交互具有更广泛的意义。
Key Takeaways
- 零射击图像分割(RIS)领域存在生成精确高质量掩膜区域表示的挑战。
- 提出了无训练、混合全局局部特征提取方法,结合掩膜特定特征与上下文信息。
- 提出了空间引导增强策略,强化掩膜区域和引用表达之间的对齐,提高空间连贯性。
- 通过融入多种空间线索,方法能更稳健、精确地执行引用分割。
- 在标准RIS基准测试上,该方法显著优于现有模型,实现性能大幅提升。
- 该方法推动了RIS任务的发展,为区域文本对齐建立了通用框架。
- 对跨模态理解和交互具有更广泛的意义。
点此查看论文截图
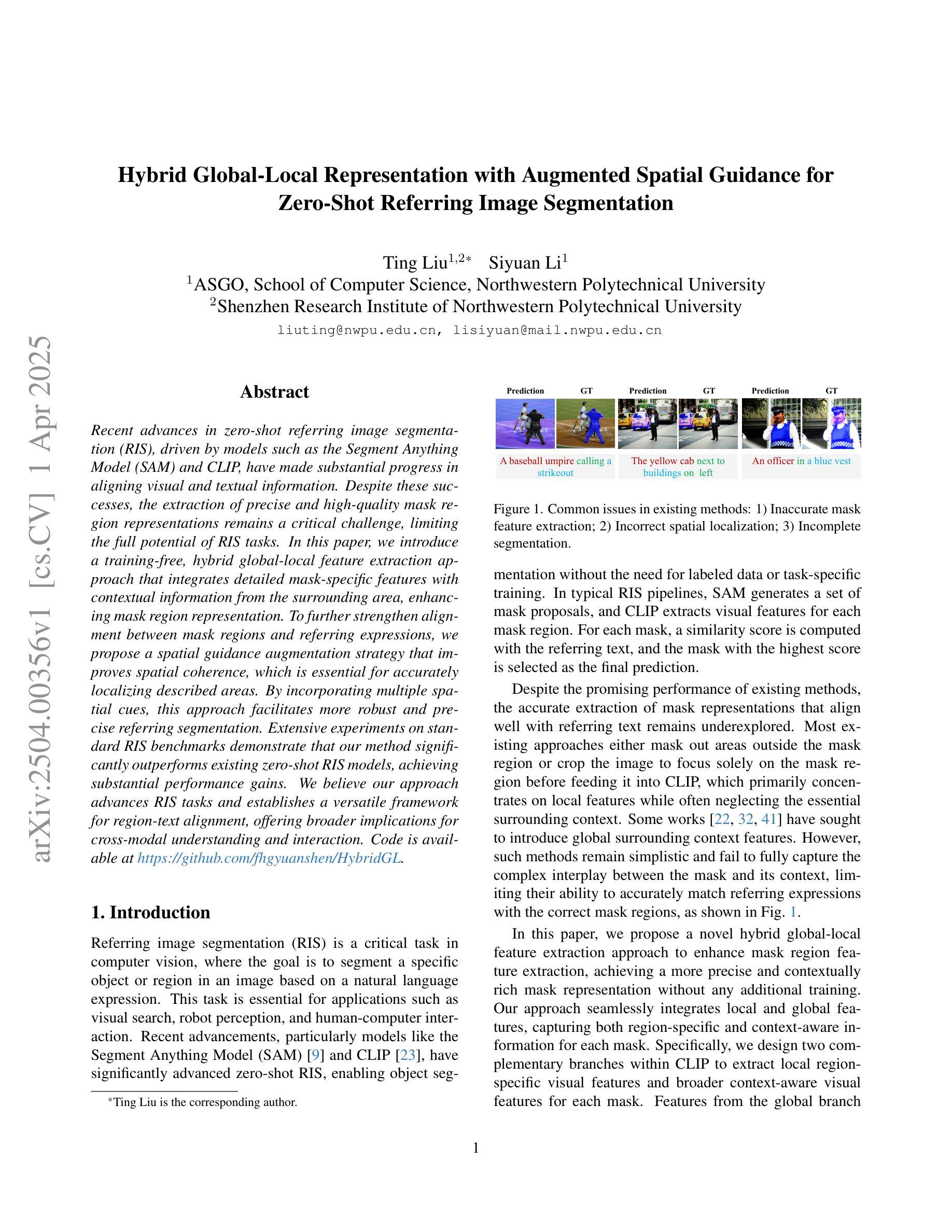
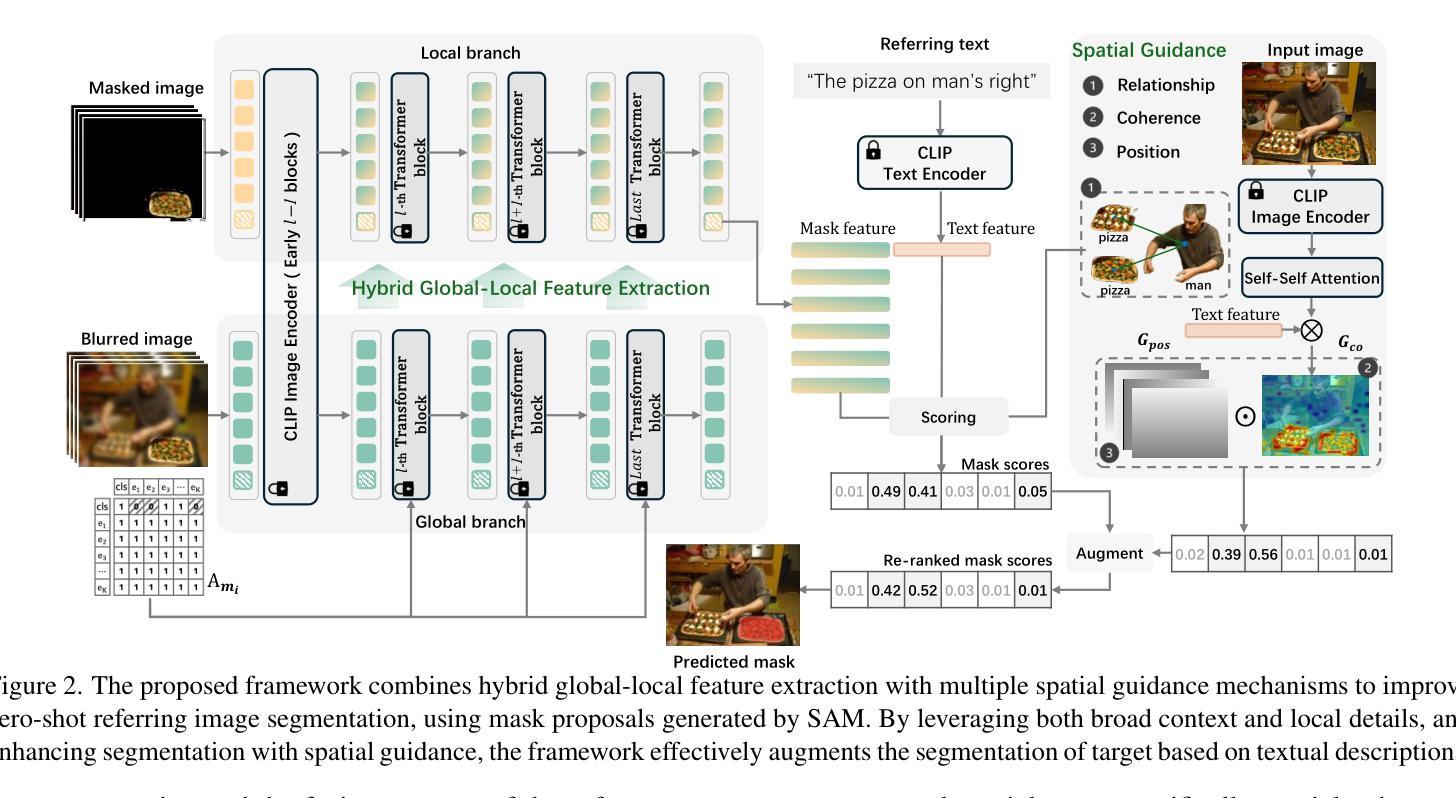
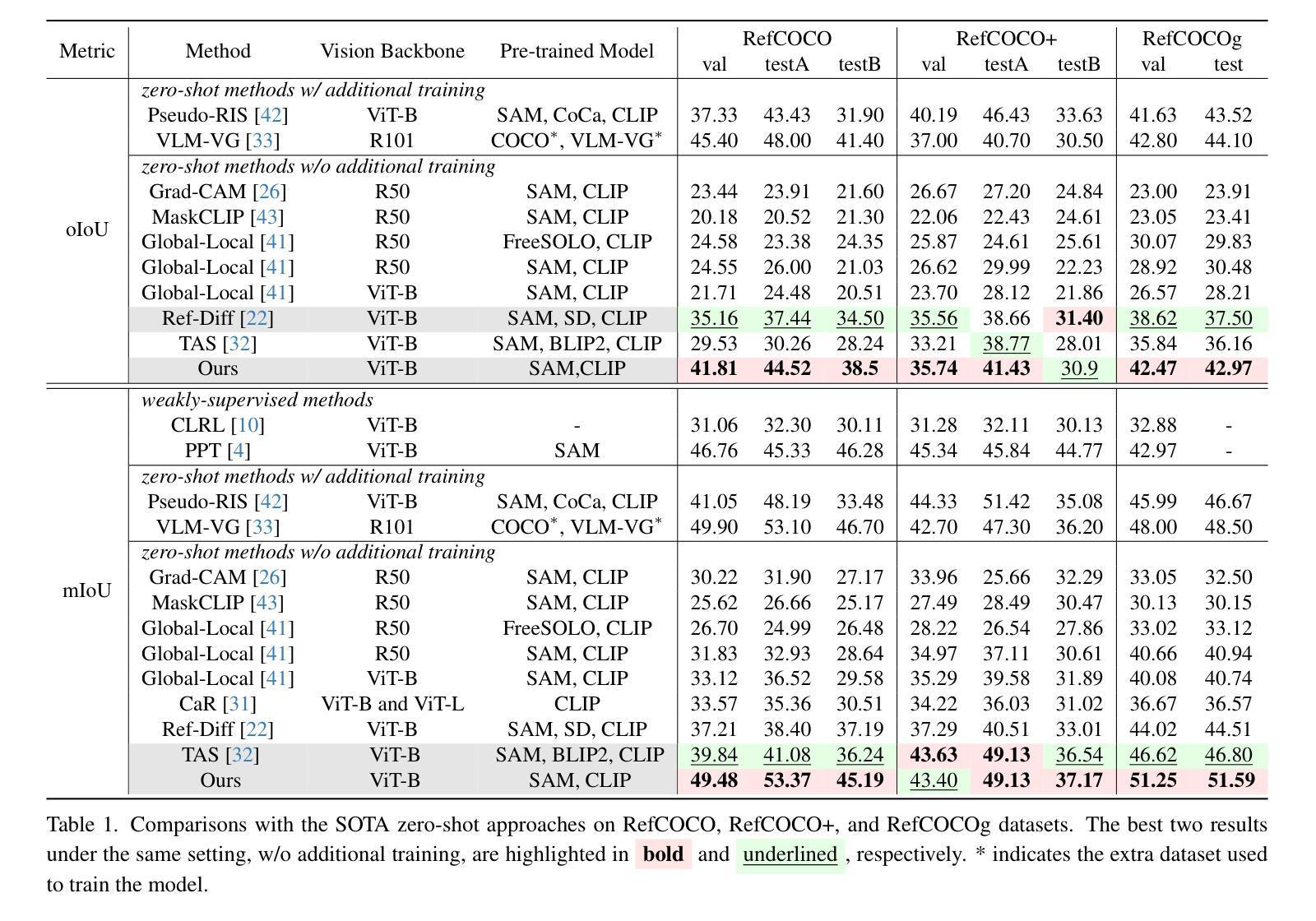
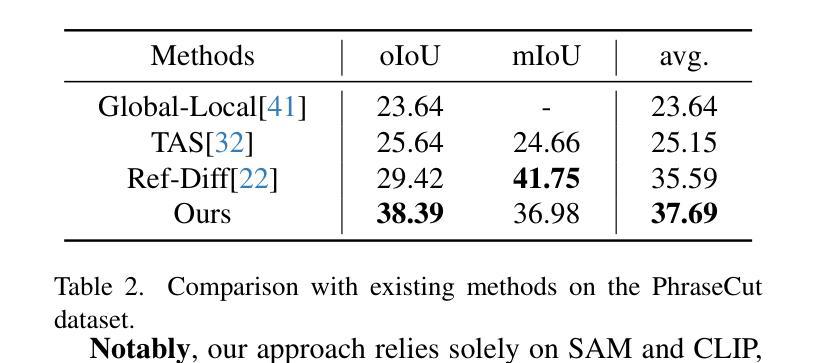
Deconver: A Deconvolutional Network for Medical Image Segmentation
Authors:Pooya Ashtari, Shahryar Noei, Fateme Nateghi Haredasht, Jonathan H. Chen, Giuseppe Jurman, Aleksandra Pizurica, Sabine Van Huffel
While convolutional neural networks (CNNs) and vision transformers (ViTs) have advanced medical image segmentation, they face inherent limitations such as local receptive fields in CNNs and high computational complexity in ViTs. This paper introduces Deconver, a novel network that integrates traditional deconvolution techniques from image restoration as a core learnable component within a U-shaped architecture. Deconver replaces computationally expensive attention mechanisms with efficient nonnegative deconvolution (NDC) operations, enabling the restoration of high-frequency details while suppressing artifacts. Key innovations include a backpropagation-friendly NDC layer based on a provably monotonic update rule and a parameter-efficient design. Evaluated across four datasets (ISLES’22, BraTS’23, GlaS, FIVES) covering both 2D and 3D segmentation tasks, Deconver achieves state-of-the-art performance in Dice scores and Hausdorff distance while reducing computational costs (FLOPs) by up to 90% compared to leading baselines. By bridging traditional image restoration with deep learning, this work offers a practical solution for high-precision segmentation in resource-constrained clinical workflows. The project is available at https://github.com/pashtari/deconver.
虽然卷积神经网络(CNNs)和视觉转换器(ViTs)已经推动了医学图像分割的进展,但它们也面临一些固有的局限性,例如CNN中的局部感受野和ViT中的高计算复杂度。本文介绍了Deconver,这是一种新型网络,它将图像恢复中的传统反卷积技术集成到一个U形架构中的核心可学习组件。Deconver用高效的非负反卷积(NDC)操作取代了计算昂贵的注意力机制,能够在恢复高频细节的同时抑制伪影。主要创新包括基于可证明的单调更新规则的逆向传播友好的NDC层以及参数高效的设计。在涵盖二维和三维分割任务的四个数据集(ISLES’22、BraTS’23、GlaS、FIVES)上进行了评估,Deconver在Dice得分和Hausdorff距离方面达到了最新技术水平,与领先的基线相比,计算成本(FLOPs)降低了高达90%。通过桥接传统图像恢复与深度学习,这项工作为资源受限的临床工作流程中的高精度分割提供了实用解决方案。该项目可在https://github.com/pashtari/deconver获取。
论文及项目相关链接
PDF 12 pages, 6 figures, 5 tables
Summary
本文提出一种名为Deconver的新型网络,它结合了传统图像恢复中的反卷积技术,并将其作为U型架构中的核心可学习组件。Deconver用高效的非负反卷积(NDC)操作取代了计算成本高昂的注意力机制,能够恢复高频细节并抑制伪影。在四个数据集上的评估表明,Deconver在Dice得分和Hausdorff距离上达到了最新水平,同时降低了高达90%的计算成本。这项研究架起了传统图像恢复与深度学习之间的桥梁,为资源受限的临床工作流程提供了高精度分割的实用解决方案。
Key Takeaways
- Deconver网络结合了传统图像恢复中的反卷积技术,并作为U型架构中的核心组件。
- Deconver用非负反卷积(NDC)操作取代高计算复杂度的注意力机制。
- NDC操作具有可证明的单调更新规则和高效的参数设计。
- Deconver在四个数据集上的表现达到了最新水平,包括2D和3D分割任务。
- 该网络在Dice得分和Hausdorff距离方面表现出色。
- Deconver相比主流基线降低了高达90%的计算成本(FLOPs)。
点此查看论文截图
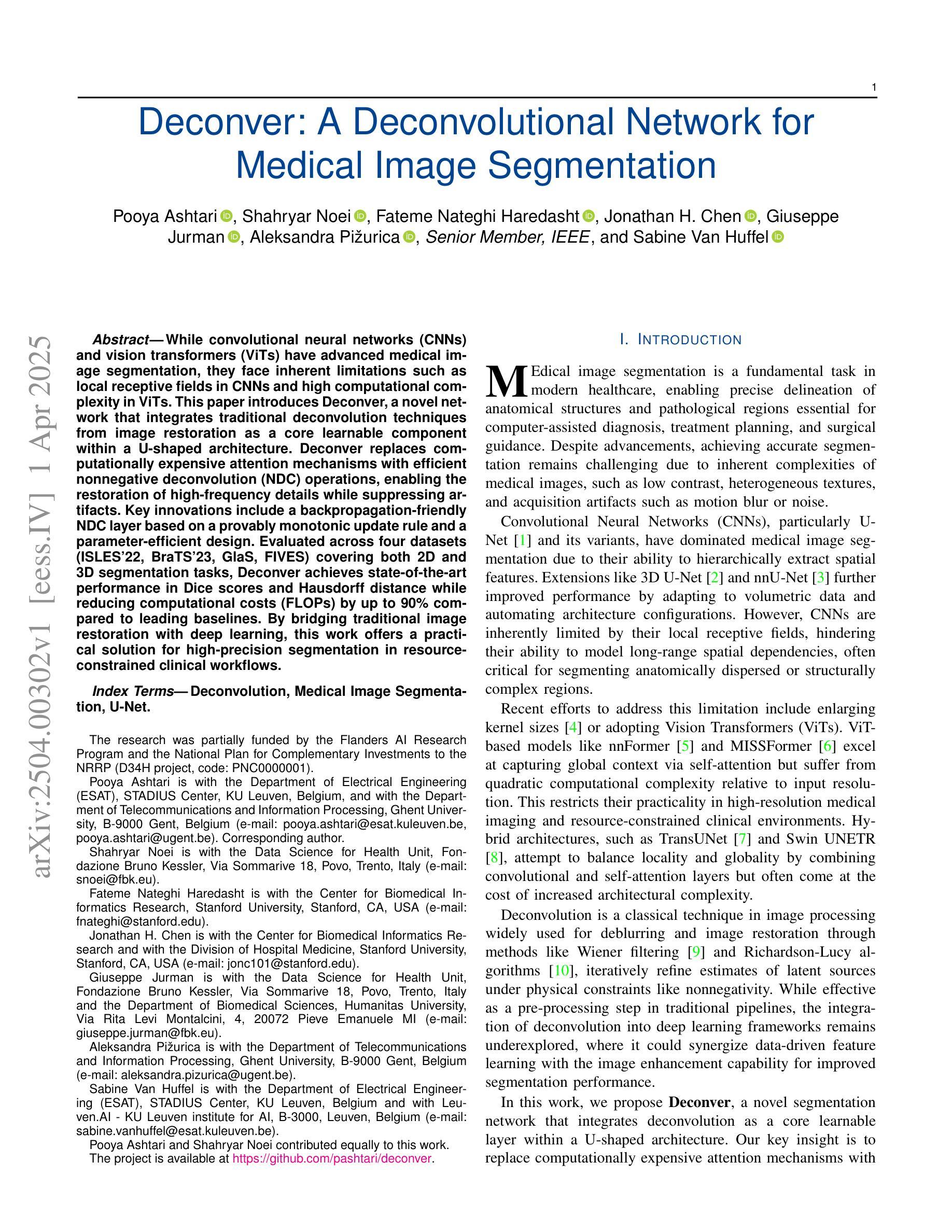
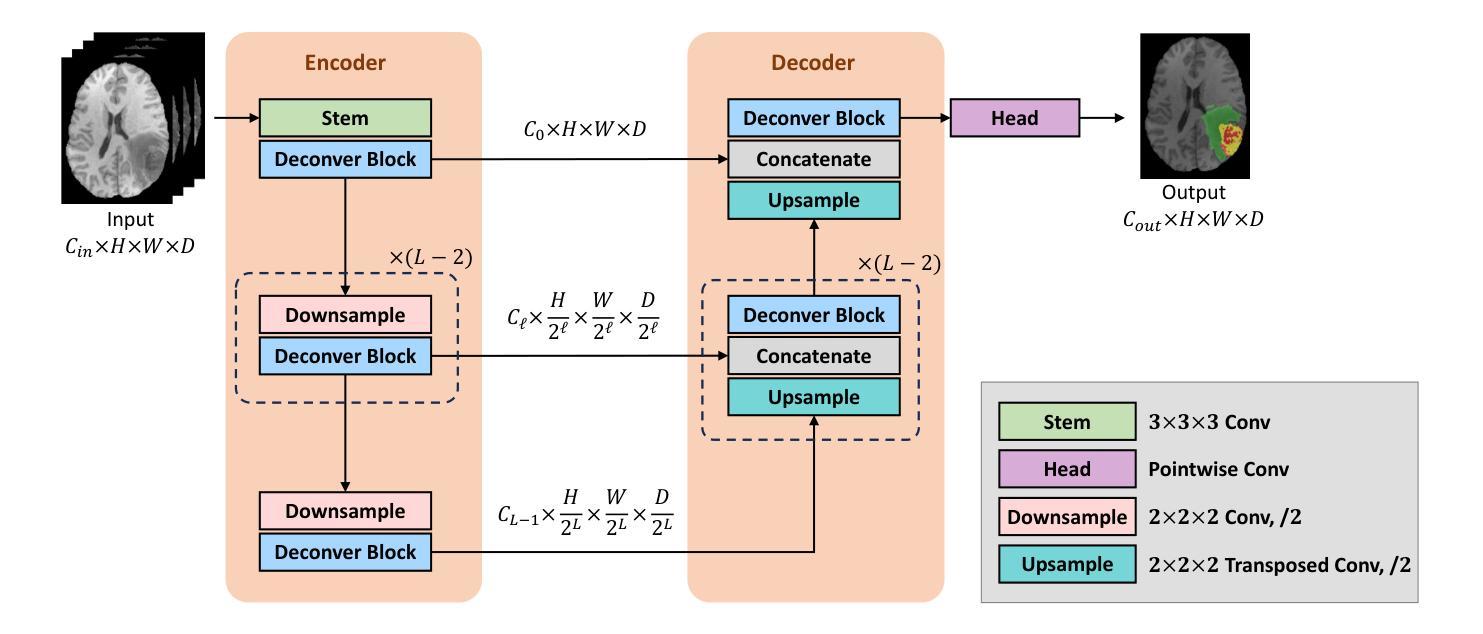
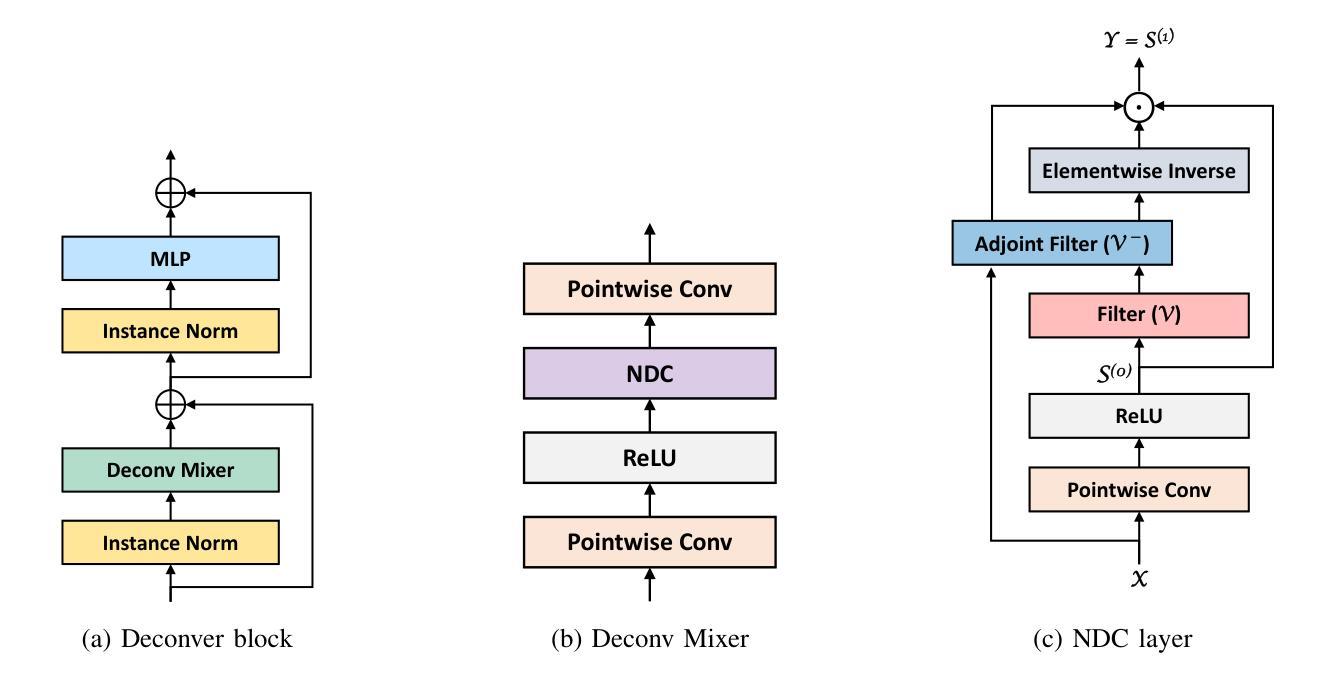
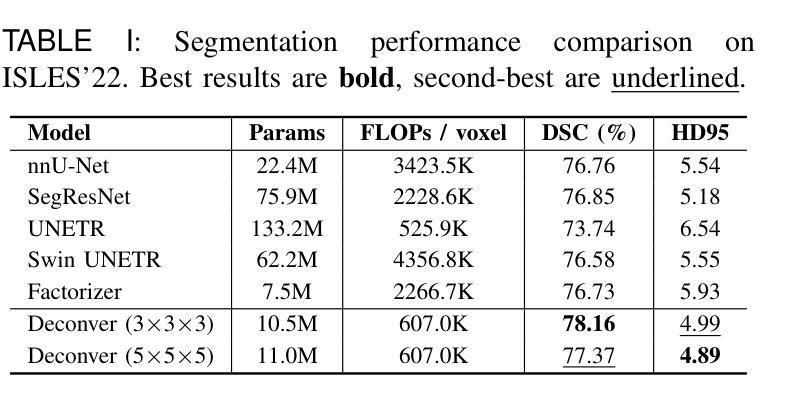
DiffDenoise: Self-Supervised Medical Image Denoising with Conditional Diffusion Models
Authors:Basar Demir, Yikang Liu, Xiao Chen, Eric Z. Chen, Lin Zhao, Boris Mailhe, Terrence Chen, Shanhui Sun
Many self-supervised denoising approaches have been proposed in recent years. However, these methods tend to overly smooth images, resulting in the loss of fine structures that are essential for medical applications. In this paper, we propose DiffDenoise, a powerful self-supervised denoising approach tailored for medical images, designed to preserve high-frequency details. Our approach comprises three stages. First, we train a diffusion model on noisy images, using the outputs of a pretrained Blind-Spot Network as conditioning inputs. Next, we introduce a novel stabilized reverse sampling technique, which generates clean images by averaging diffusion sampling outputs initialized with a pair of symmetric noises. Finally, we train a supervised denoising network using noisy images paired with the denoised outputs generated by the diffusion model. Our results demonstrate that DiffDenoise outperforms existing state-of-the-art methods in both synthetic and real-world medical image denoising tasks. We provide both a theoretical foundation and practical insights, demonstrating the method’s effectiveness across various medical imaging modalities and anatomical structures.
近年来已经提出了许多自监督的去噪方法。然而,这些方法往往会使图像过于平滑,导致丢失对医疗应用至关重要的精细结构。在本文中,我们提出了一种针对医学图像的强大自监督去噪方法——DiffDenoise,旨在保留高频细节。我们的方法包括三个阶段。首先,我们使用预训练盲点网络的输出作为条件输入,在噪声图像上训练扩散模型。接下来,我们引入了一种新型稳定的反向采样技术,该技术通过平均由一对对称噪声初始化的扩散采样输出生成干净图像。最后,我们使用与扩散模型生成的降噪输出配对的噪声图像训练有监督的去噪网络。我们的结果表明,DiffDenoise在合成和现实世界中的医学图像去噪任务中均优于现有最先进的去噪方法。我们既提供了理论基础,也提供了实践见解,证明了该方法在各种医学成像模式和解剖结构中的有效性。
论文及项目相关链接
Summary
本文提出了一种针对医学图像的强大的自监督去噪方法——DiffDenoise,旨在保留高频细节。该方法包括三个阶段:训练扩散模型、引入稳定反向采样技术,以及训练监督去噪网络。实验结果表明,DiffDenoise在合成和真实世界医学图像去噪任务中均优于现有最先进的方法,并展示了该方法在不同医学成像模态和解剖结构中的有效性。
Key Takeaways
- 提出的DiffDenoise是一种针对医学图像的自监督去噪方法,旨在保留高频细节。
- 方法包括三个阶段:训练扩散模型、引入稳定反向采样技术,以及训练监督去噪网络。
- 扩散模型使用带噪声图像进行训练,并以预训练盲点网络的输出作为条件输入。
- 引入了一种新型稳定的反向采样技术,该技术通过平均扩散采样输出(初始化为一对对称噪声)来生成干净图像。
- 使用由扩散模型生成的去噪输出来训练监督去噪网络。
- 实验结果表明,DiffDenoise在合成和真实世界医学图像去噪任务中均表现优异。
点此查看论文截图
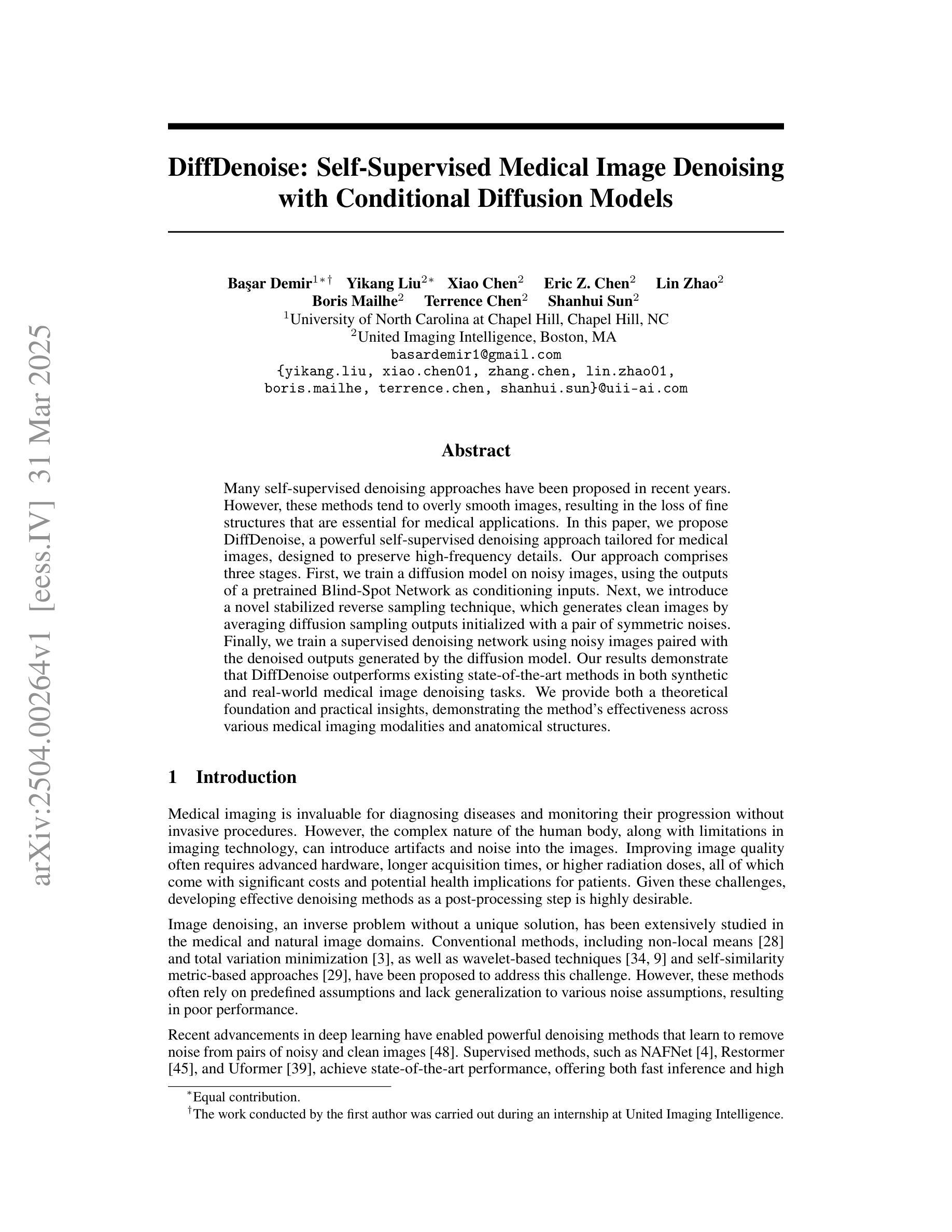
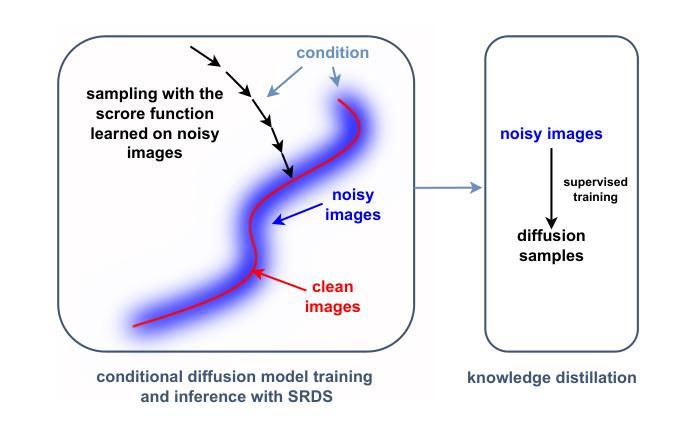
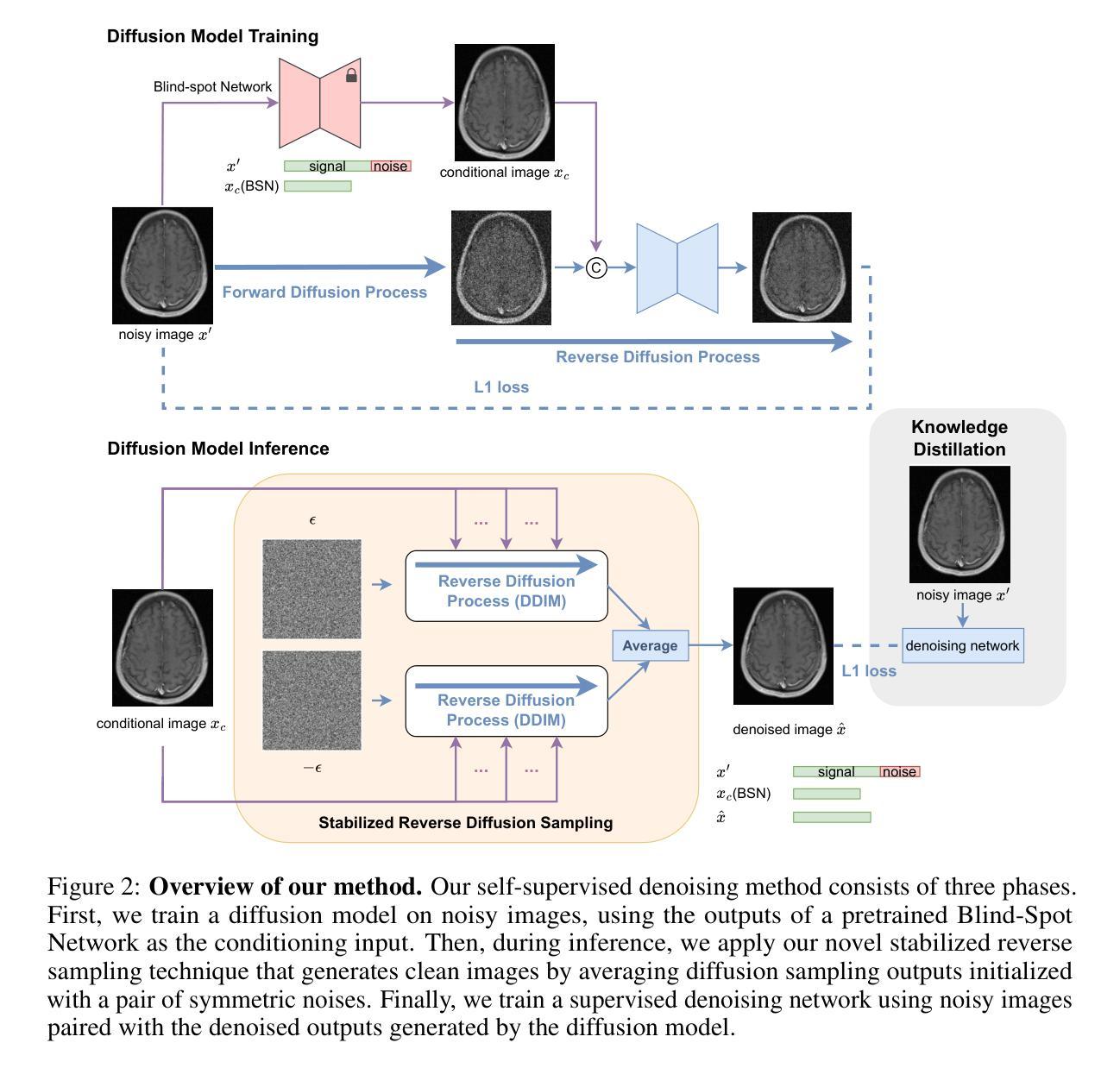
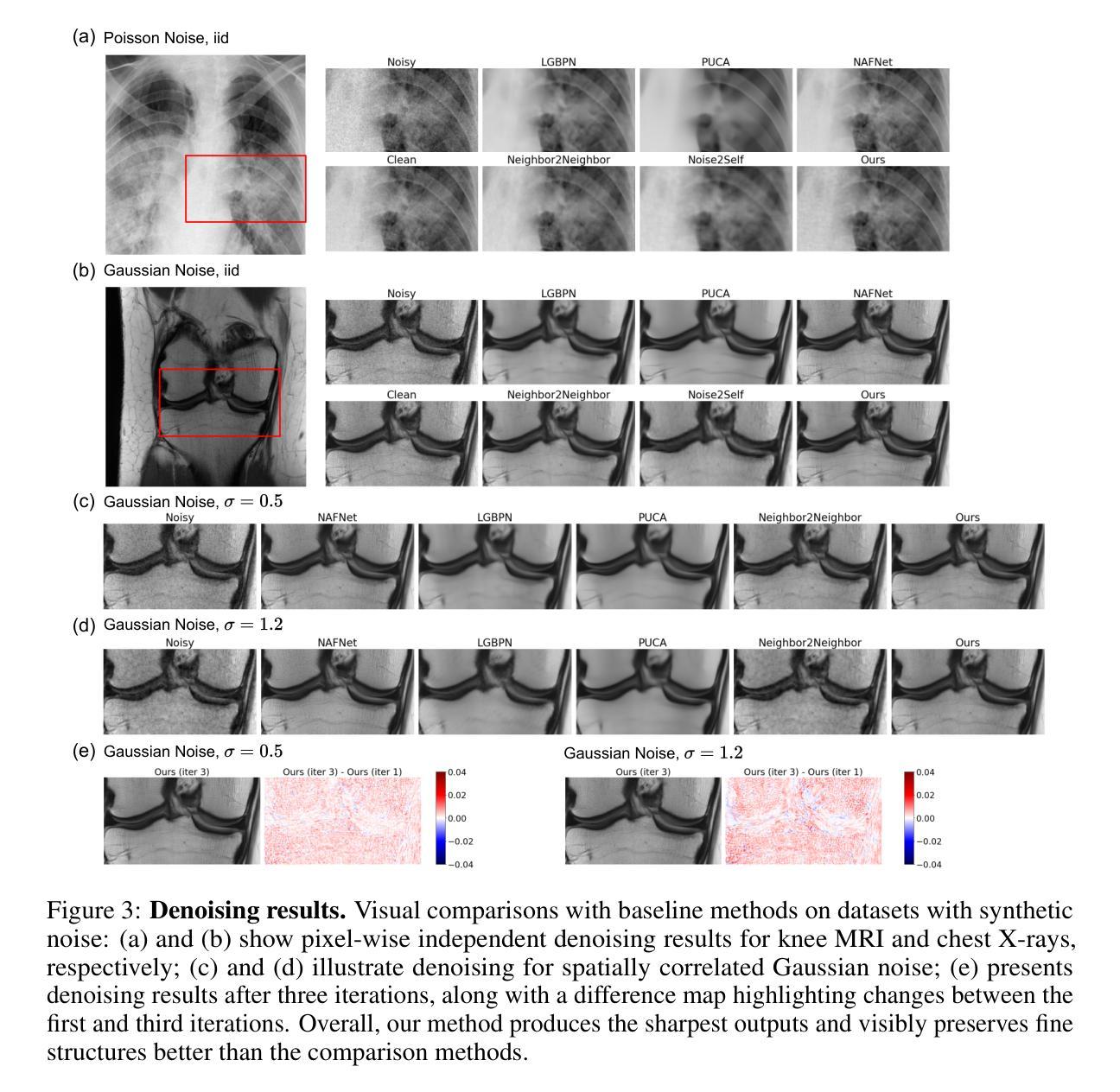
Adapting Vision Foundation Models for Real-time Ultrasound Image Segmentation
Authors:Xiaoran Zhang, Eric Z. Chen, Lin Zhao, Xiao Chen, Yikang Liu, Boris Maihe, James S. Duncan, Terrence Chen, Shanhui Sun
We propose a novel approach that adapts hierarchical vision foundation models for real-time ultrasound image segmentation. Existing ultrasound segmentation methods often struggle with adaptability to new tasks, relying on costly manual annotations, while real-time approaches generally fail to match state-of-the-art performance. To overcome these limitations, we introduce an adaptive framework that leverages the vision foundation model Hiera to extract multi-scale features, interleaved with DINOv2 representations to enhance visual expressiveness. These enriched features are then decoded to produce precise and robust segmentation. We conduct extensive evaluations on six public datasets and one in-house dataset, covering both cardiac and thyroid ultrasound segmentation. Experiments show that our approach outperforms state-of-the-art methods across multiple datasets and excels with limited supervision, surpassing nnUNet by over 20% on average in the 1% and 10% data settings. Our method achieves $\sim$77 FPS inference speed with TensorRT on a single GPU, enabling real-time clinical applications.
我们提出了一种新型方法,该方法将层次化的视觉基础模型应用于实时超声图像分割。现有的超声分割方法往往难以适应新任务,且依赖于昂贵的手动标注,而实时方法通常无法达到最新技术的性能水平。为了克服这些局限性,我们引入了一个自适应框架,该框架利用视觉基础模型Hiera提取多尺度特征,并与DINOv2表示交替使用,以增强视觉表现力。这些丰富的特征随后被解码以产生精确且稳定的分割。我们在六个公开数据集和一个内部数据集上进行了广泛评估,涵盖了心脏和甲状腺超声分割。实验表明,我们的方法在多数据集上的表现优于最新技术,并且在监督有限的情况下表现尤为出色,在1%和10%的数据设置下平均超过nnUNet约20%。我们的方法在单个GPU上使用TensorRT可达到约每秒77帧的推理速度,适用于实时临床应用。
论文及项目相关链接
摘要
提出一种新型方法,采用层次视觉基础模型进行实时超声图像分割。现有超声分割方法在新任务适应性方面存在困难,依赖昂贵的手动标注,而实时方法一般无法达到最新技术水平。为克服这些局限,我们引入了一种自适应框架,利用视觉基础模型Hiera提取多尺度特征,与DINOv2表示法交替出现,增强视觉表现力。这些丰富的特征随后被解码以产生精确且稳健的分割。我们在六个公开数据集和一个内部数据集上进行广泛评估,涵盖心脏和甲状腺超声分割。实验表明,我们的方法在多个数据集上优于最新技术,并在有限监督下表现出色,在1%和10%的数据设置下平均超出nnUNet 20%以上。我们的方法使用TensorRT在单个GPU上实现~77 FPS的推理速度,适用于实时临床应用。
要点
- 提出一种基于层次视觉基础模型的实时超声图像分割新方法。
- 现有超声分割方法存在新任务适应困难和依赖手动标注的问题。
- 引入自适应框架,结合Hiera和DINOv2技术提取多尺度特征和增强视觉表现力。
- 在多个数据集上进行广泛评估,涵盖心脏和甲状腺超声分割。
- 与最新技术相比,该方法在多个数据集上表现优越,尤其在有限监督环境下。
- 在1%和10%数据设置下,该方法平均超出nnUNet 20%以上。
点此查看论文截图
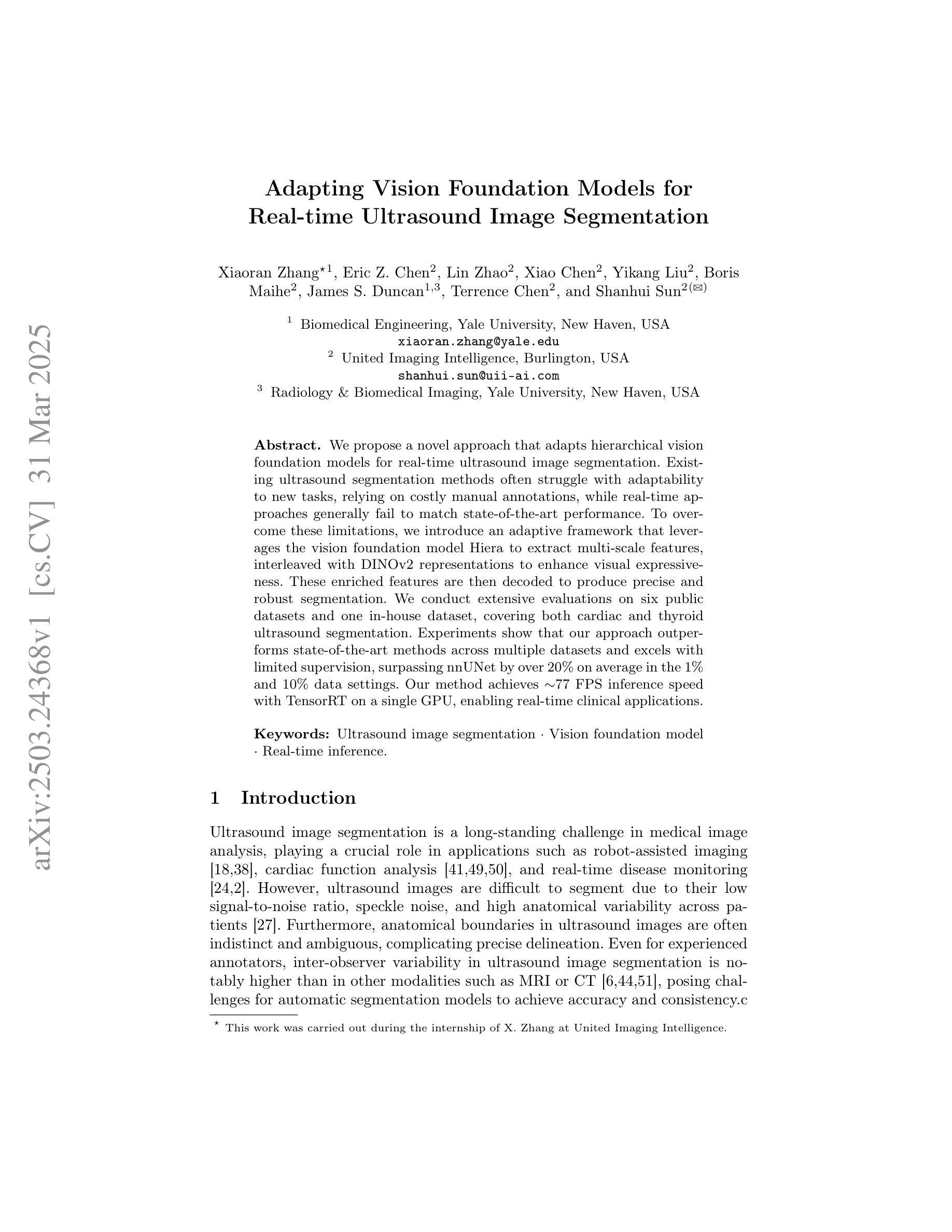
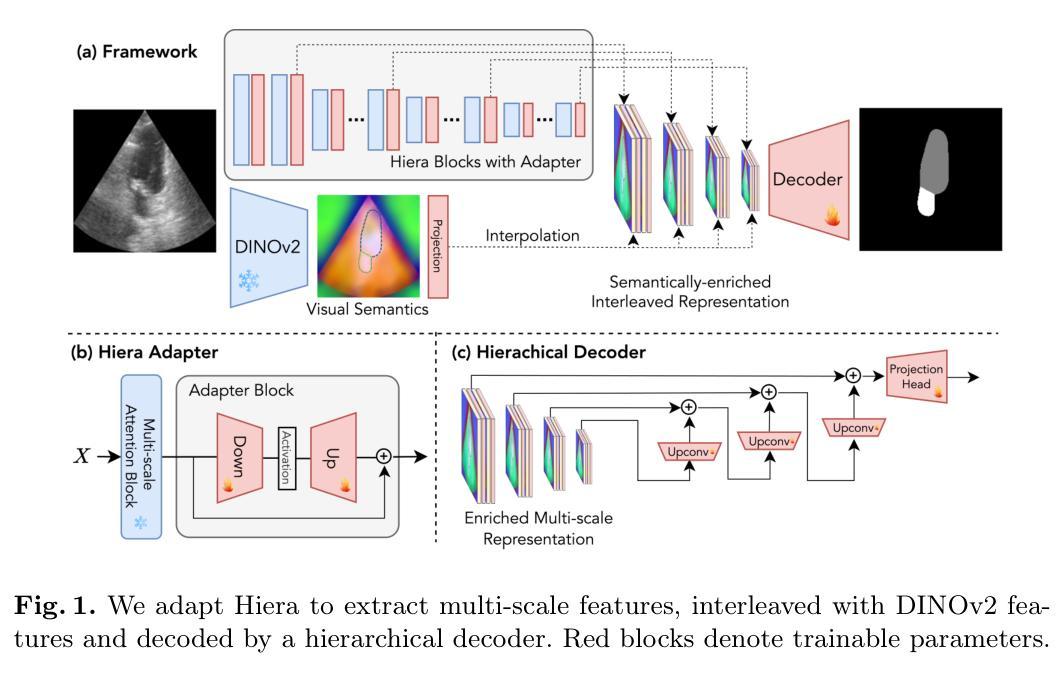
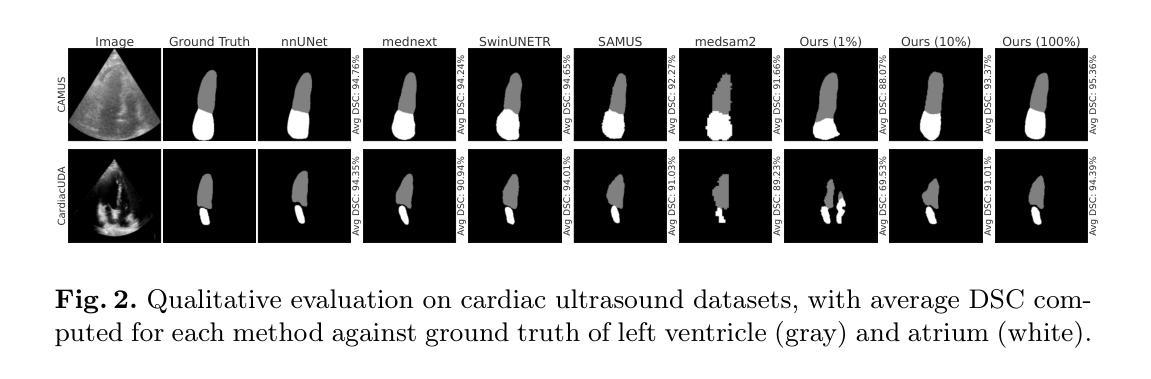
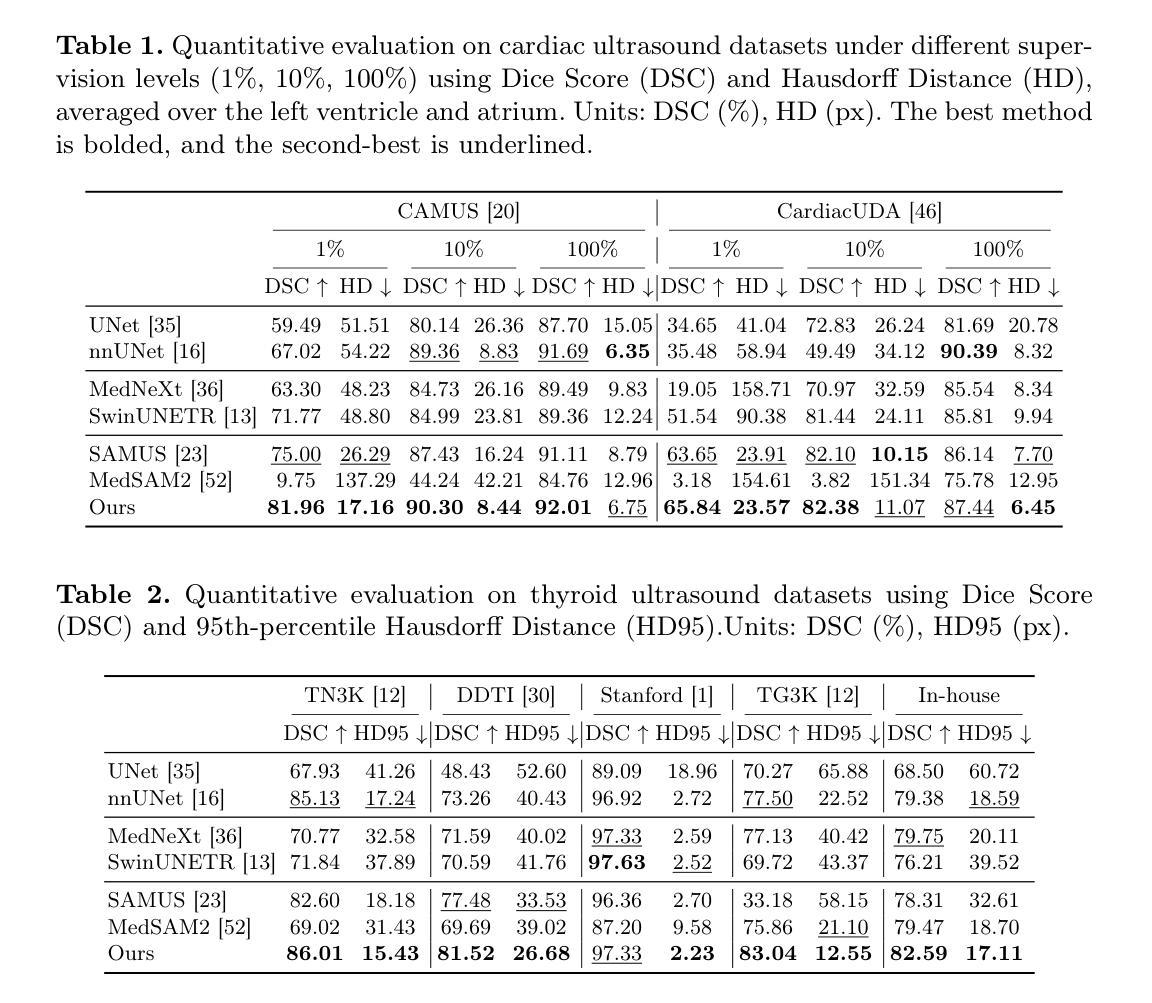
IMPACT: A Generic Semantic Loss for Multimodal Medical Image Registration
Authors:Valentin Boussot, Cédric Hémon, Jean-Claude Nunes, Jason Downling, Simon Rouzé, Caroline Lafond, Anaïs Barateau, Jean-Louis Dillenseger
Image registration is fundamental in medical imaging, enabling precise alignment of anatomical structures for diagnosis, treatment planning, image-guided treatment or longitudinal monitoring. This work introduces IMPACT (Image Metric with Pretrained model-Agnostic Comparison for Transmodality registration), a generic semantic similarity metric designed for seamless integration into diverse image registration frameworks (such as Elastix and Voxelmorph). It compares deep learning-based features extracted from medical images without requiring task-specific training, ensuring broad applicability across various modalities. By leveraging the features of the large-scale pretrained TotalSegmentator models and the ability to integrate Segment Anything Model (SAM) and other large-scale segmentation networks, this approach offers significant advantages. It provides robust, scalable, and efficient solutions for multimodal image registration. The IMPACT loss was evaluated on five challenging registration tasks involving thoracic CT/CBCT, and pelvic MR/CT datasets. Quantitative metrics, such as Target Registration Error and Dice Similarity Coefficient, demonstrated significant improvements in anatomical alignment compared to baseline methods. Qualitative analyses further confirmed the increased robustness of the proposed metric in the face of noise, artifacts, and modality variations. IMPACT’s versatility and efficiency make it a valuable tool for advancing registration performance in clinical and research applications, addressing critical challenges in multimodal medical imaging.
医学影像中的图像配准是实现精确解剖结构对齐的关键技术,对于诊断、治疗计划、图像引导治疗和纵向监测都具有重要意义。本研究引入了IMPACT(针对跨模态配准的预训练模型通用语义相似性度量指标),这是一种通用语义相似性度量指标,旨在无缝集成到多种图像配准框架(如Elastix和VoxelMorph)中。它比较医学图像中深度学习的特征提取结果,无需特定任务的训练,确保在不同模态的广泛应用中的适用性。通过利用大规模预训练的TotalSegmentator模型的特性以及集成Segment Anything Model(SAM)和其他大规模分割网络的能力,该方法具有显著优势。它为多模态图像配准提供了稳健、可扩展和高效的解决方案。IMPACT损失在涉及胸部CT/CBCT和盆腔MR/CT数据集的五个具有挑战性的配准任务上进行了评估。通过目标注册误差和Dice相似系数等定量指标,与基准方法相比,在解剖结构对齐方面显示出显著改善。定性分析进一步证实了所提出指标在面对噪声、伪影和模态变化时的增强稳健性。IMPACT的通用性和效率使其成为推动临床和研究应用中配准性能提高的有价值工具,解决了多模态医学影像中的关键挑战。
论文及项目相关链接
PDF Submitted to IEEE Transactions on Pattern Analysis and Machine Intelligence (TPAMI). This is a preprint version and has not been peer-reviewed
Summary
医学图像配准对于诊断、治疗计划、图像引导治疗和长期监测等至关重要。本文提出一种名为IMPACT的通用语义相似性度量方法,可无缝集成到多种图像配准框架中。该方法利用深度学习从医学图像中提取的特征,无需特定任务训练,可在各种模态之间广泛应用。通过与大型预训练TotalSegmentator模型的特性和整合Segment Anything Model等其他大型分割网络的能力相结合,该方法提供了稳健、可扩展和高效的跨模态图像配准解决方案。在五个具有挑战性的注册任务上评估IMPACT损失,定量和定性分析均证明了其在解剖学对齐方面的显著改进。
Key Takeaways
- 图像配准在医学成像中具有重要作用,用于诊断、治疗计划、图像引导治疗和长期监测。
- IMPACT是一种通用的语义相似性度量方法,旨在解决图像配准问题。
- IMPACT可以无缝集成到不同的图像配准框架中,如Elastix和Voxelmorph。
- 该方法利用深度学习从医学图像中提取特征,无需特定任务训练,适用于多种模态。
- IMPACT利用大型预训练模型(如TotalSegmentator)的特性,并可与Segment Anything Model等其他大型分割网络结合使用。
- 在多个挑战性注册任务上评估IMPACT损失,结果显示其在解剖学对齐方面较基线方法有显著改善。
点此查看论文截图
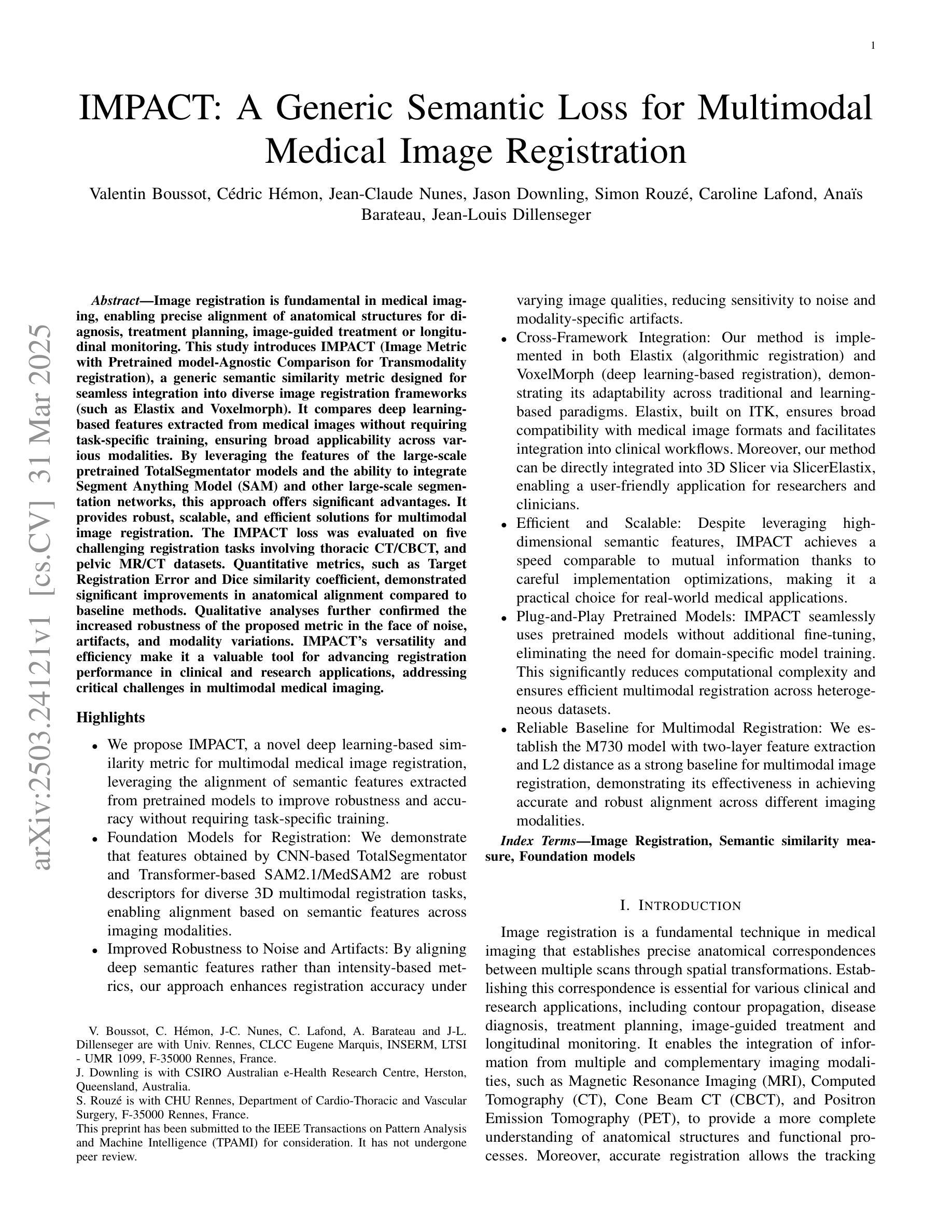
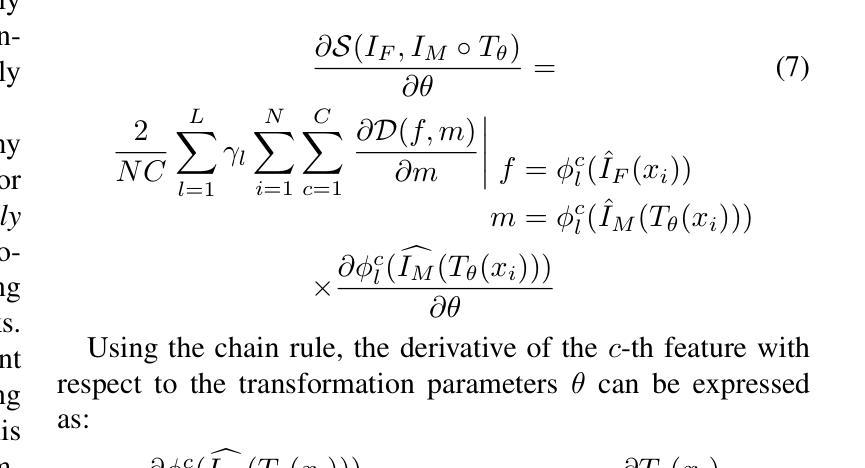
Learned Image Compression and Restoration for Digital Pathology
Authors:SeonYeong Lee, EonSeung Seong, DongEon Lee, SiYeoul Lee, Yubin Cho, Chunsu Park, Seonho Kim, MinKyung Seo, YoungSin Ko, MinWoo Kim
Digital pathology images play a crucial role in medical diagnostics, but their ultra-high resolution and large file sizes pose significant challenges for storage, transmission, and real-time visualization. To address these issues, we propose CLERIC, a novel deep learning-based image compression framework designed specifically for whole slide images (WSIs). CLERIC integrates a learnable lifting scheme and advanced convolutional techniques to enhance compression efficiency while preserving critical pathological details. Our framework employs a lifting-scheme transform in the analysis stage to decompose images into low- and high-frequency components, enabling more structured latent representations. These components are processed through parallel encoders incorporating Deformable Residual Blocks (DRB) and Recurrent Residual Blocks (R2B) to improve feature extraction and spatial adaptability. The synthesis stage applies an inverse lifting transform for effective image reconstruction, ensuring high-fidelity restoration of fine-grained tissue structures. We evaluate CLERIC on a digital pathology image dataset and compare its performance against state-of-the-art learned image compression (LIC) models. Experimental results demonstrate that CLERIC achieves superior rate-distortion (RD) performance, significantly reducing storage requirements while maintaining high diagnostic image quality. Our study highlights the potential of deep learning-based compression in digital pathology, facilitating efficient data management and long-term storage while ensuring seamless integration into clinical workflows and AI-assisted diagnostic systems. Code and models are available at: https://github.com/pnu-amilab/CLERIC.
数字病理图像在医学诊断中起着至关重要的作用,但其超高的分辨率和庞大的文件大小给存储、传输和实时可视化带来了重大挑战。为了解决这些问题,我们提出了CLERIC,这是一种基于深度学习、专为全幻灯片图像(WSI)设计的图像压缩框架。CLERIC集成了一种可学习的提升方案和先进的卷积技术,以提高压缩效率,同时保留关键的病理细节。我们的框架在分析阶段采用提升方案变换,将图像分解为低频和高频成分,以实现更结构化的潜在表示。这些成分通过包含可变形残差块(DRB)和循环残差块(R2B)的并行编码器进行处理,以改善特征提取和空间适应性。合成阶段应用逆向提升变换进行有效的图像重建,确保精细的纹理组织结构的保真恢复。我们在数字病理学图像数据集上评估了CLERIC的性能,并将其与最先进的学习图像压缩(LIC)模型进行了比较。实验结果表明,CLERIC在速率失真(RD)性能上表现优越,在保持高诊断图像质量的同时显著减少了存储需求。我们的研究突出了基于深度学习的压缩在数字病理学中的潜力,有助于实现高效的数据管理和长期存储,同时确保无缝集成到临床工作流程和人工智能辅助诊断系统中。代码和模型可在https://github.com/pnu-amilab/CLERIC获取。
论文及项目相关链接
Summary
基于深度学习的图像压缩框架CLERIC,专为全幻灯片图像(WSIs)设计,解决了数字病理图像超高分辨率和大文件尺寸带来的存储、传输和实时可视化挑战。CLERIC采用可学习的提升方案和先进的卷积技术,提升压缩效率的同时保留关键病理细节。实验结果表明,CLERIC在速率失真(RD)性能上表现优越,能显著降低存储需求同时保持高诊断图像质量。
Key Takeaways
- 数字病理图像在医学诊断中起关键作用,但其超高分辨率和大文件尺寸带来存储、传输和可视化挑战。
- CLERIC是一个基于深度学习的专门用于全幻灯片图像(WSIs)的压缩框架。
- CLERIC采用可学习的提升方案,将图像分解为低频和高频成分,实现更结构化的潜在表示。
- 框架中的并行编码器采用可变残差块和循环残差块,改善特征提取和空间适应性。
- 合成阶段应用反向提升变换,实现有效图像重建,恢复精细的组织结构。
- 实验结果表明,CLERIC在速率失真性能上优于现有的学习图像压缩模型。
点此查看论文截图
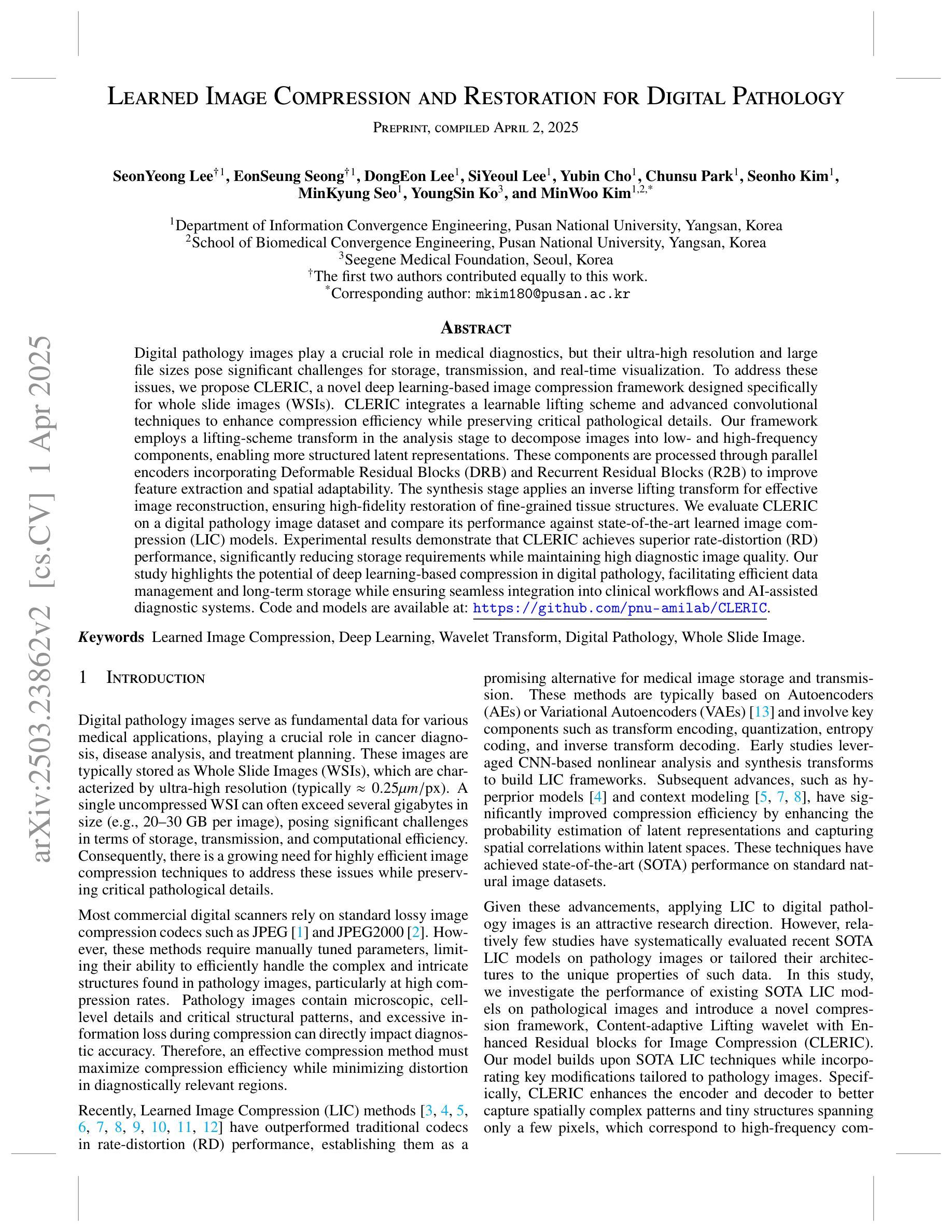
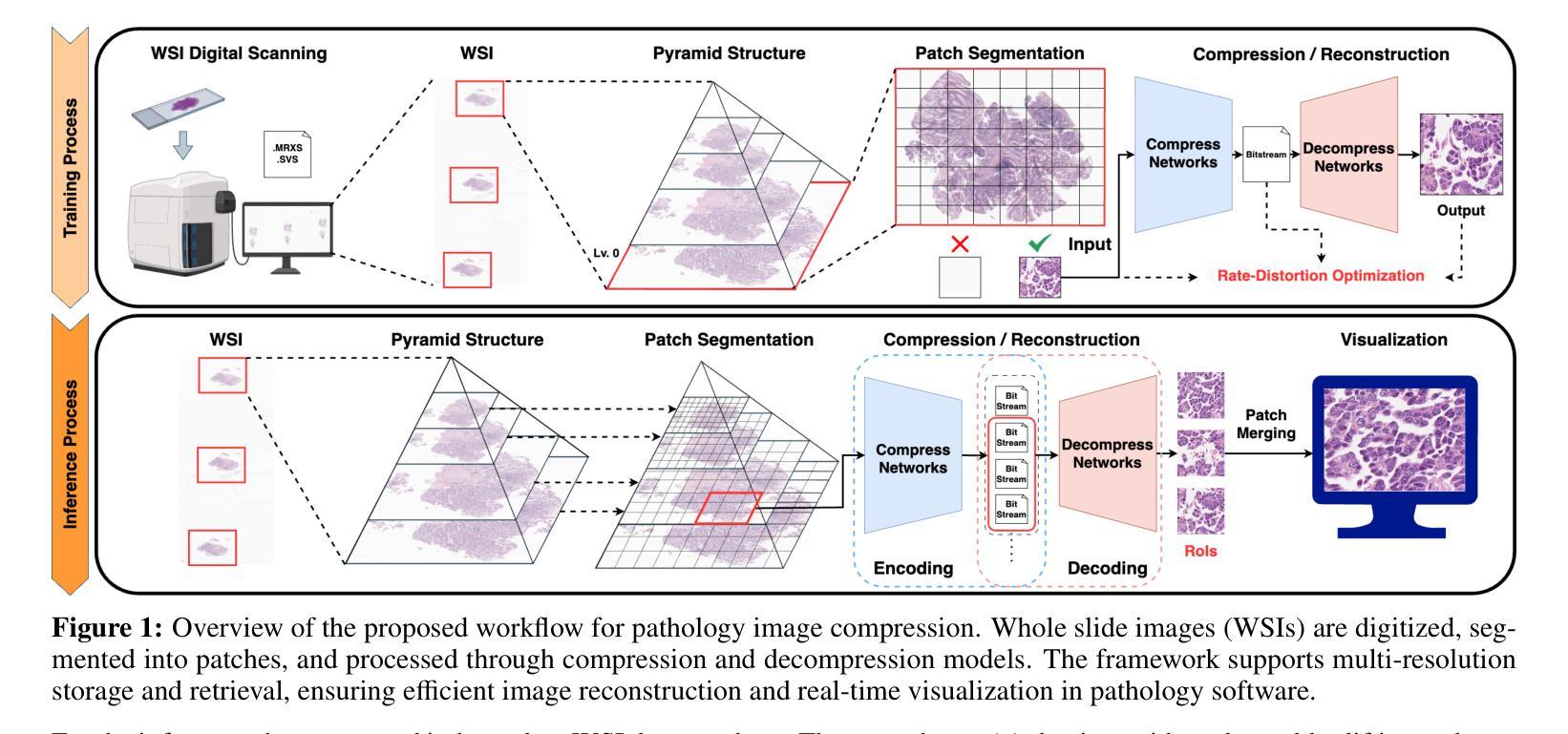
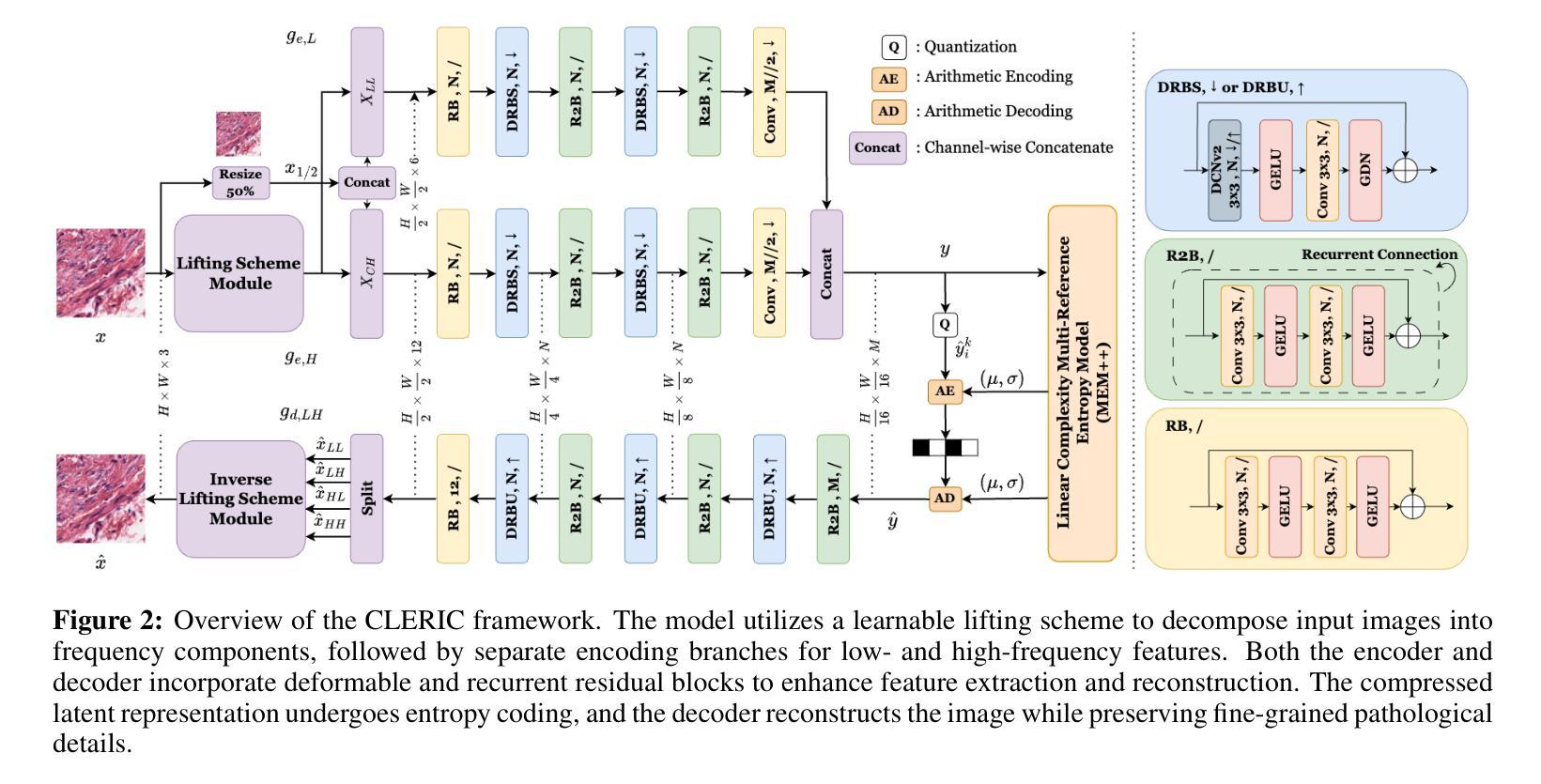
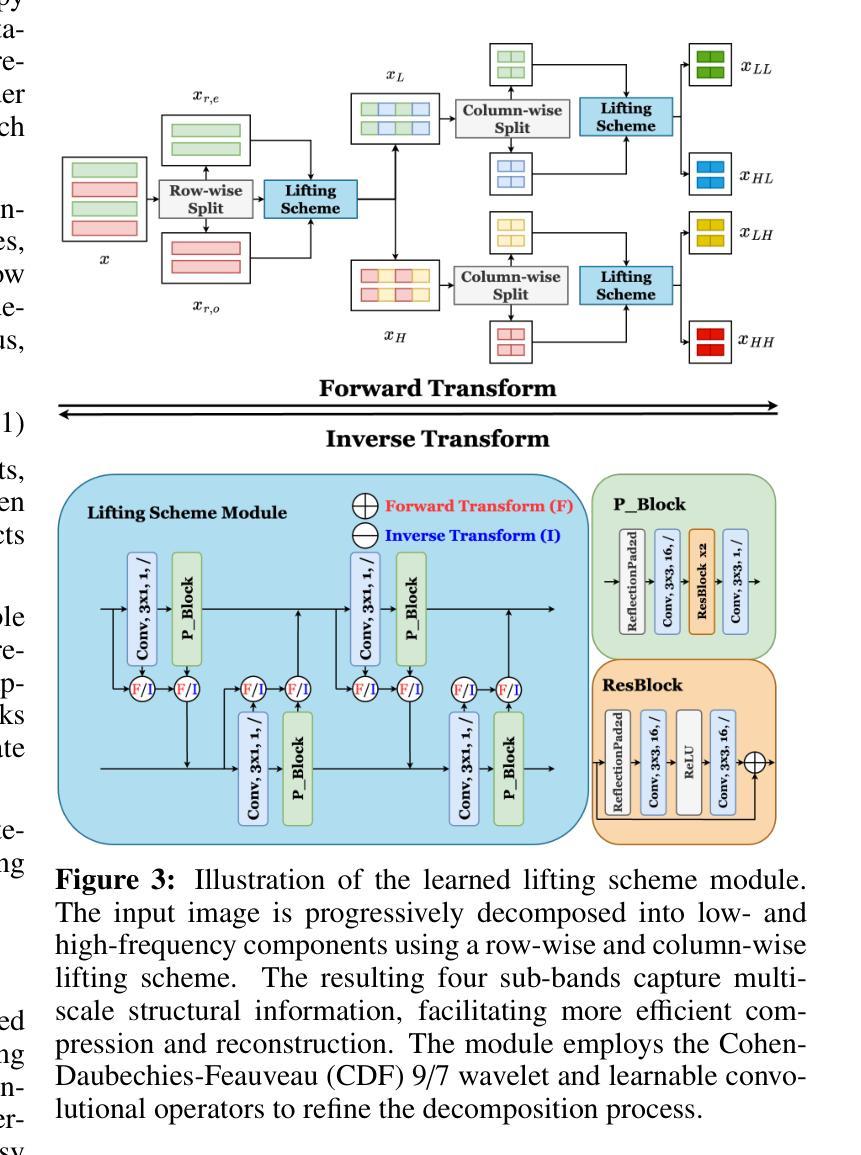
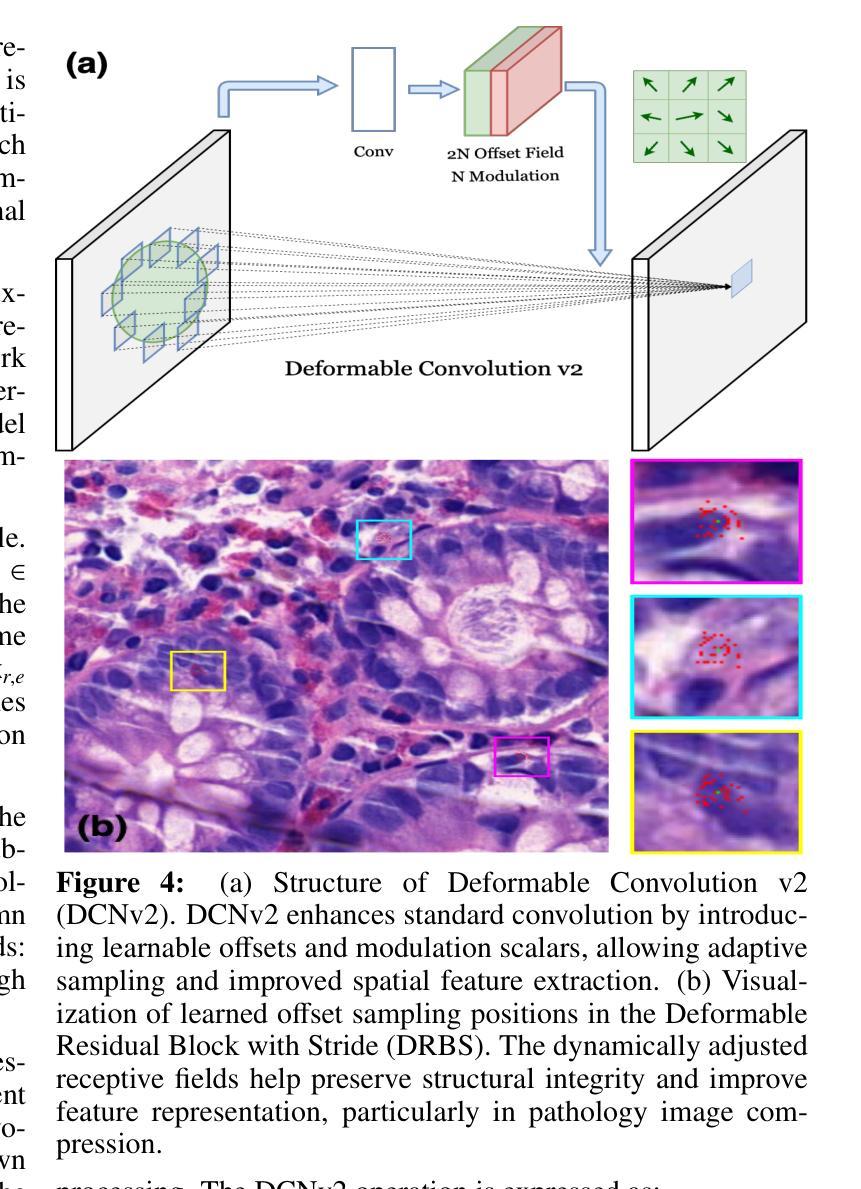
WaveFormer: A 3D Transformer with Wavelet-Driven Feature Representation for Efficient Medical Image Segmentation
Authors:Md Mahfuz Al Hasan, Mahdi Zaman, Abdul Jawad, Alberto Santamaria-Pang, Ho Hin Lee, Ivan Tarapov, Kyle See, Md Shah Imran, Antika Roy, Yaser Pourmohammadi Fallah, Navid Asadizanjani, Reza Forghani
Transformer-based architectures have advanced medical image analysis by effectively modeling long-range dependencies, yet they often struggle in 3D settings due to substantial memory overhead and insufficient capture of fine-grained local features. We address these limitations with WaveFormer, a novel 3D-transformer that: i) leverages the fundamental frequency-domain properties of features for contextual representation, and ii) is inspired by the top-down mechanism of the human visual recognition system, making it a biologically motivated architecture. By employing discrete wavelet transformations (DWT) at multiple scales, WaveFormer preserves both global context and high-frequency details while replacing heavy upsampling layers with efficient wavelet-based summarization and reconstruction. This significantly reduces the number of parameters, which is critical for real-world deployment where computational resources and training times are constrained. Furthermore, the model is generic and easily adaptable to diverse applications. Evaluations on BraTS2023, FLARE2021, and KiTS2023 demonstrate performance on par with state-of-the-art methods while offering substantially lower computational complexity.
基于Transformer的架构通过有效地建模长程依赖关系,已经推动了医学图像分析的发展。然而,它们在3D环境中往往面临巨大的内存开销和精细局部特征捕捉不足的问题。为了解决这些局限性,我们提出了WaveFormer,这是一种新型的3D Transformer,它:(i)利用特征的基本频域属性进行上下文表示;(ii)受到人类视觉识别系统自上而下机制的启发,是一种具有生物动机的架构。通过采用多尺度的离散小波变换(DWT),WaveFormer在保留全局上下文和高频细节的同时,用高效的基于小波的总览和重建替代了大量的上采样层。这显著减少了参数数量,对于计算资源和训练时间受限的实际情况下的部署至关重要。此外,该模型具有通用性,可轻松适应多种应用。在BraTS2023、FLARE2021和KiTS2023上的评估表明,其性能与最先进的方法相当,同时计算复杂度大大降低。
论文及项目相关链接
Summary
波前形成器(WaveFormer)是一种新型3D变压器架构,它通过利用特征的频域属性进行上下文表示,并借鉴人类视觉识别系统的自上而下机制,解决了现有变压器在3D设置中面临的内存开销大、精细局部特征捕捉不足的问题。通过采用多尺度离散小波变换(DWT),WaveFormer在保留全局上下文和高频细节的同时,以高效的小波为基础进行摘要和重建,替代了繁重的上采样层。这显著减少了参数数量,对于计算资源和训练时间受限的实际部署环境至关重要。此外,该模型通用且易于适应多种应用。在BraTS2023、FLARE2021和KiTS2023上的评估表明,其性能与最先进的方法相当,但计算复杂度大大降低。
Key Takeaways
- WaveFormer是一种新型的3D变压器架构,旨在解决现有变压器在医疗图像分析中的局限性。
- 该架构利用特征的频域属性进行上下文表示,借鉴了人类视觉识别系统的自上而下机制。
- 通过采用离散小波变换(DWT),WaveFormer能够同时保留全局上下文和高频细节。
- WaveFormer通过高效的小波摘要和重建替代了繁重的上采样层,显著减少了参数数量。
- 这种架构在计算资源和训练时间受限的实际部署环境中具有优势。
- WaveFormer在多个医疗图像分析评估中表现出与最先进方法相当的性能。
点此查看论文截图
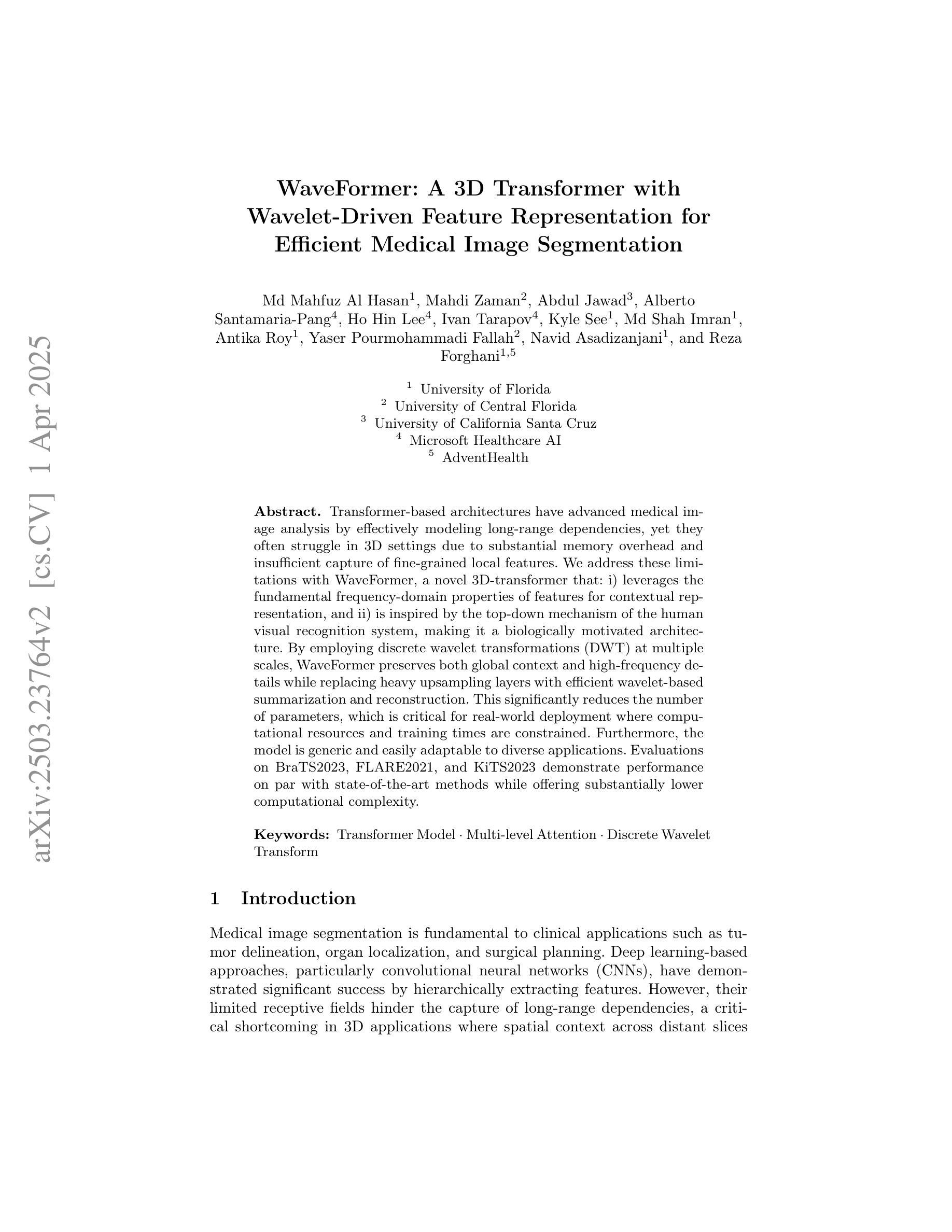
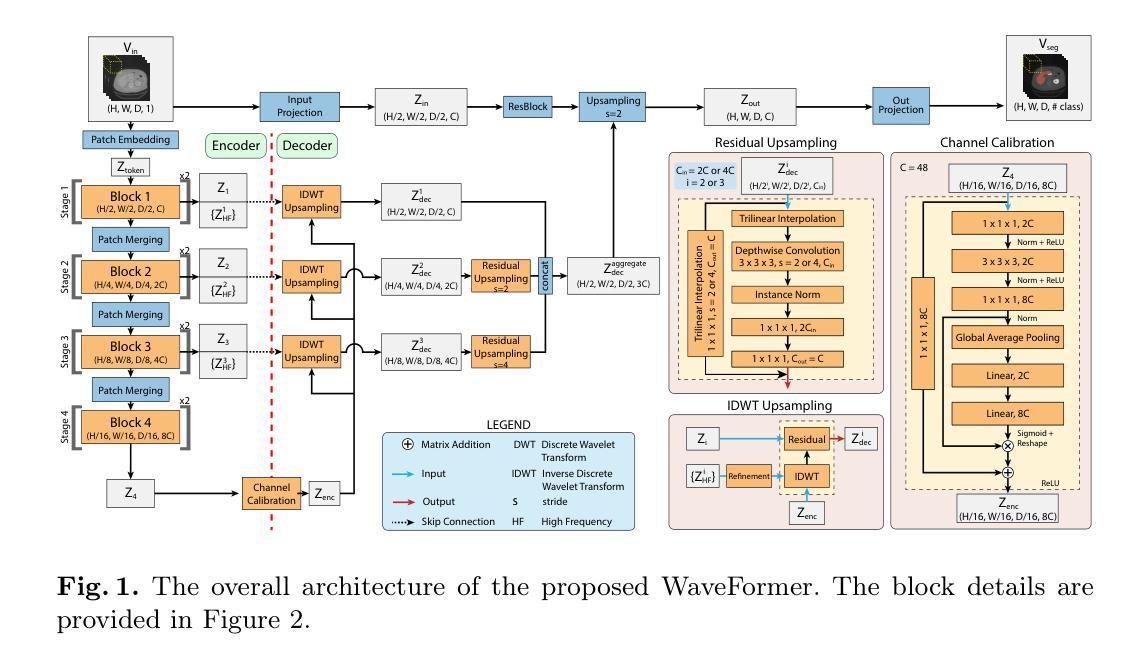
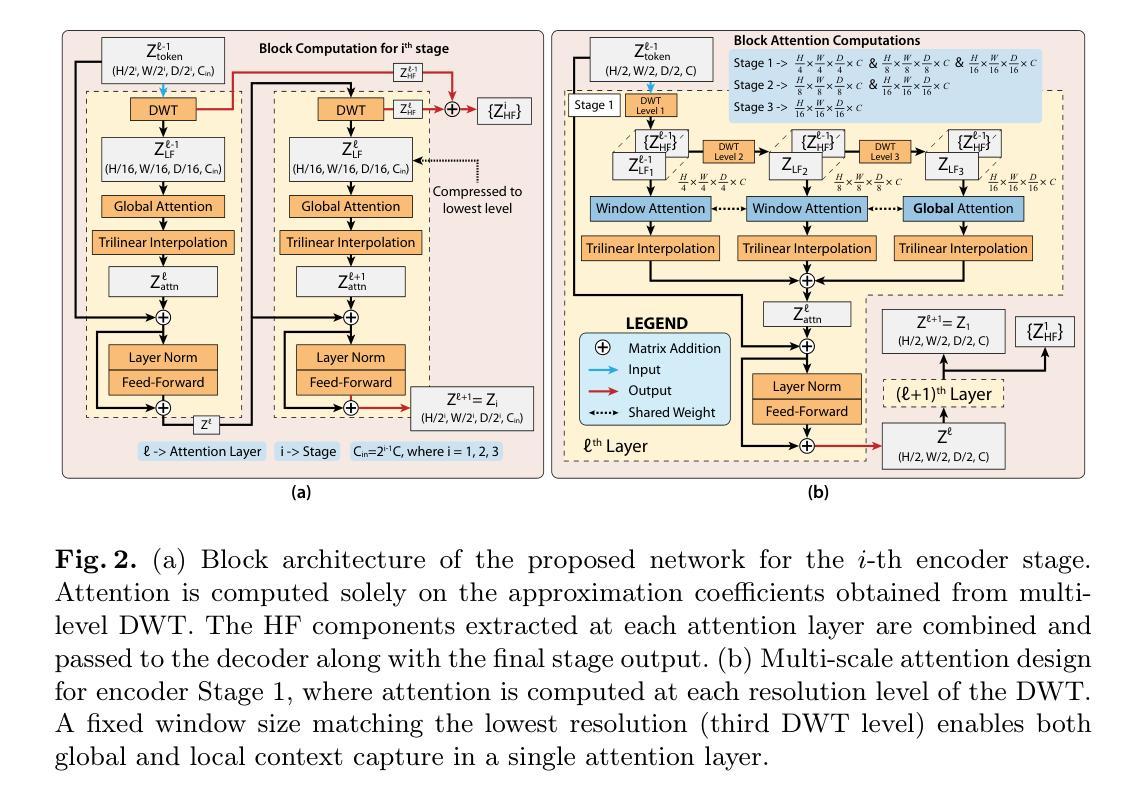
BiPVL-Seg: Bidirectional Progressive Vision-Language Fusion with Global-Local Alignment for Medical Image Segmentation
Authors:Rafi Ibn Sultan, Hui Zhu, Chengyin Li, Dongxiao Zhu
Medical image segmentation typically relies solely on visual data, overlooking the rich textual information clinicians use for diagnosis. Vision-language models attempt to bridge this gap, but existing approaches often process visual and textual features independently, resulting in weak cross-modal alignment. Simple fusion techniques fail due to the inherent differences between spatial visual features and sequential text embeddings. Additionally, medical terminology deviates from general language, limiting the effectiveness of off-the-shelf text encoders and further hindering vision-language alignment. We propose BiPVL-Seg, an end-to-end framework that integrates vision-language fusion and embedding alignment through architectural and training innovations, where both components reinforce each other to enhance medical image segmentation. BiPVL-Seg introduces bidirectional progressive fusion in the architecture, which facilitates stage-wise information exchange between vision and text encoders. Additionally, it incorporates global-local contrastive alignment, a training objective that enhances the text encoder’s comprehension by aligning text and vision embeddings at both class and concept levels. Extensive experiments on diverse medical imaging benchmarks across CT and MR modalities demonstrate BiPVL-Seg’s superior performance when compared with state-of-the-art methods in complex multi-class segmentation. Source code is available in this GitHub repository.
医学图像分割通常仅依赖于视觉数据,忽略了临床医生用于诊断的丰富文本信息。视觉语言模型试图弥合这一鸿沟,但现有方法通常独立处理视觉和文本特征,导致跨模态对齐较弱。简单的融合技术由于空间视觉特征和序列文本嵌入之间的固有差异而失败。此外,医学术语与通用语言有偏差,限制了现成的文本编码器的有效性,进一步阻碍了视觉语言对齐。我们提出了BiPVL-Seg,这是一个端到端的框架,通过架构和训练创新整合视觉语言融合和嵌入对齐,两个组件相互增强,以提高医学图像分割。BiPVL-Seg在架构中引入了双向渐进融合,有利于促进视觉和文本编码器之间的阶段性信息交换。此外,它结合了全局局部对比对齐这一训练目标,通过在对齐文本和视觉嵌入时实现类和概念级别的对齐,增强了文本编码器的理解能力。在CT和MR模态的多样化医学影像基准测试上的广泛实验表明,与最新方法相比,BiPVL-Seg在复杂的多类分割中表现出卓越的性能。源代码可在GitHub仓库中找到。
论文及项目相关链接
Summary
医疗图像分割通常仅依赖视觉数据,忽略了临床医生用于诊断的丰富文本信息。本研究提出BiPVL-Seg框架,通过架构和训练创新,实现视觉与语言的融合与嵌入对齐,以提高医疗图像分割的准确性。BiPVL-Seg引入双向渐进融合技术,便于视觉和文本编码器之间的阶段性信息交换。同时采用全局局部对比对齐训练目标,提高文本编码器的理解能力,通过对齐文本和视觉嵌入的类别和概念级别。在CT和MR多种医学影像基准测试上的实验表明,BiPVL-Seg相较于最先进的方法在多类复杂分割中表现优越。
Key Takeaways
- 医疗图像分割主要依赖视觉数据,但忽略文本信息在临床诊断中的重要性。
- BiPVL-Seg是一个创新的端到端框架,旨在提高医疗图像分割的性能。
- BiPVL-Seg实现了视觉与语言的融合与嵌入对齐。
- 该框架引入了双向渐进融合技术,便于视觉和文本编码器之间的信息交换。
- 采用全局局部对比对齐训练目标,提高文本编码器的理解能力。
- BiPVL-Seg在多种医学影像基准测试上的表现优于现有最先进的方法。
- BiPVL-Seg的源代码已公开在GitHub上。
点此查看论文截图
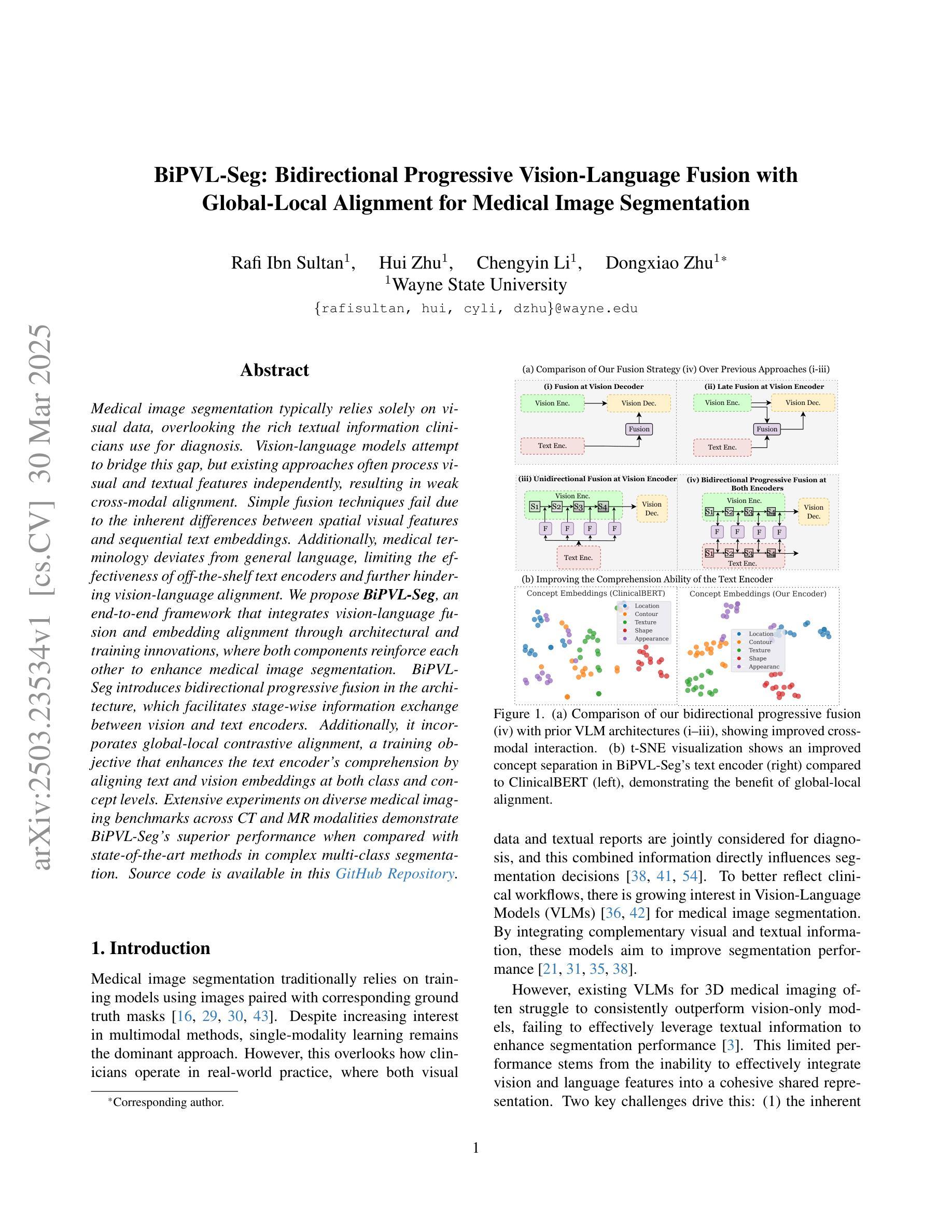
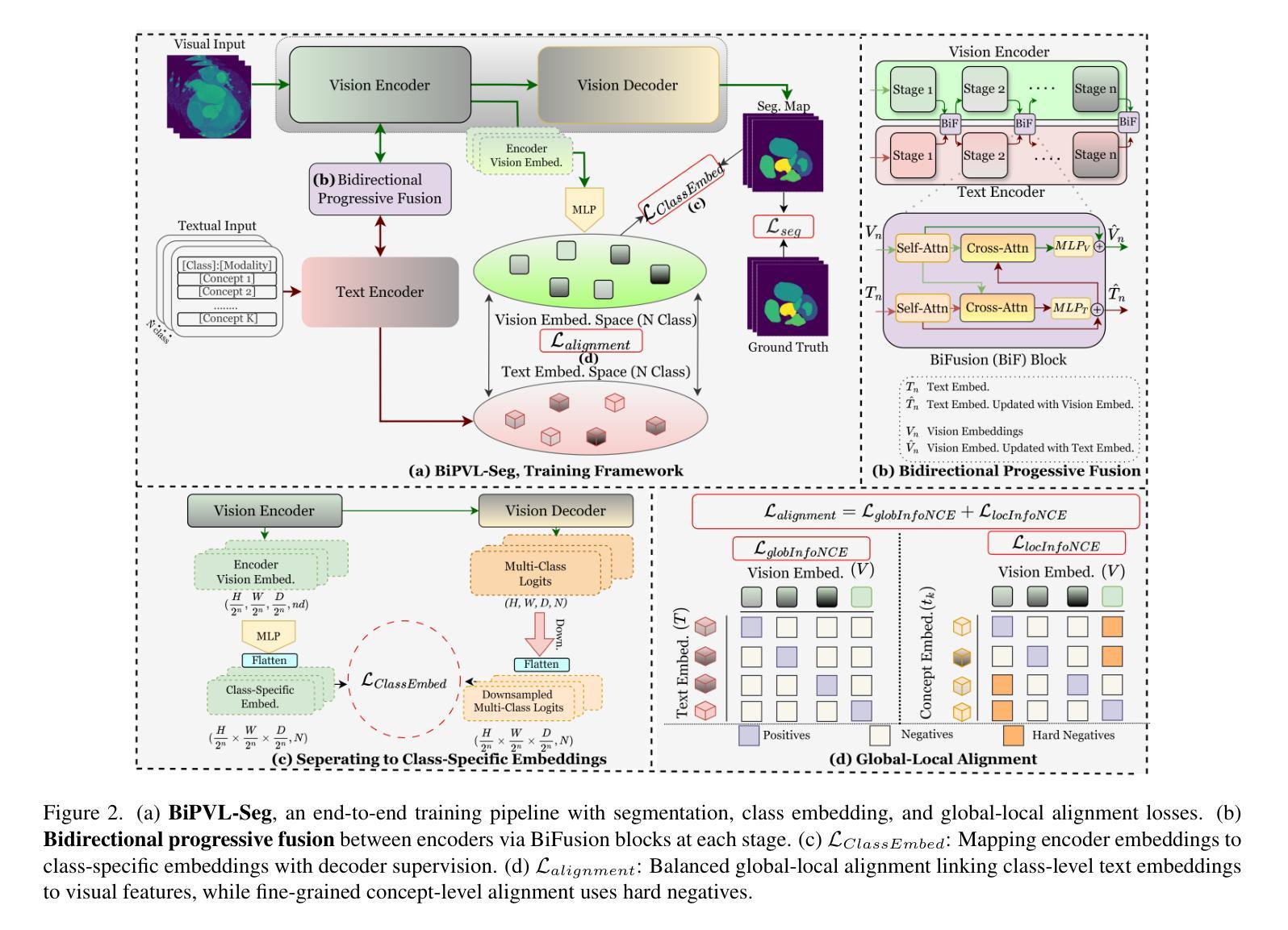
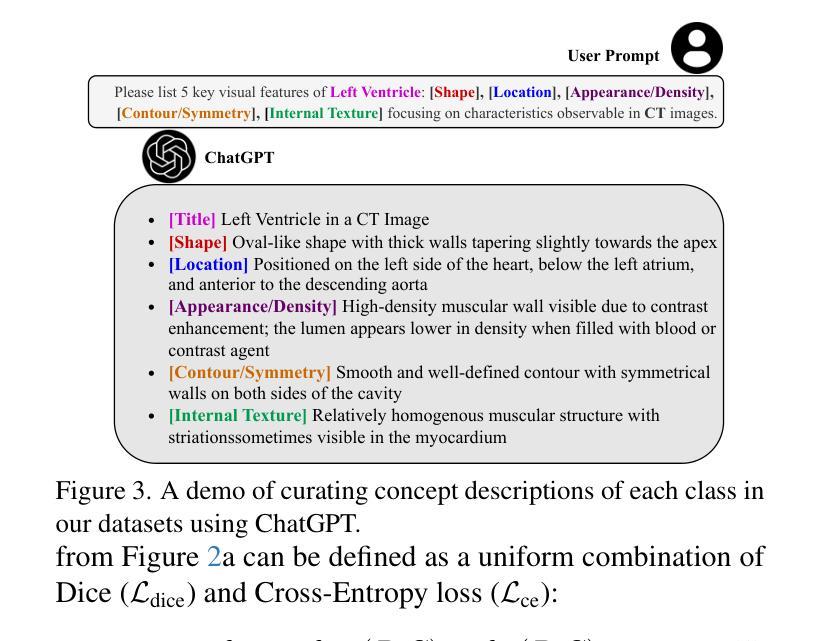
Federated Self-Supervised Learning for One-Shot Cross-Modal and Cross-Imaging Technique Segmentation
Authors:Siladittya Manna, Suresh Das, Sayantari Ghosh, Saumik Bhattacharya
Decentralized federated learning enables learning of data representations from multiple sources without compromising the privacy of the clients. In applications like medical image segmentation, where obtaining a large annotated dataset from a single source is a distressing problem, federated self-supervised learning can provide some solace. In this work, we push the limits further by exploring a federated self-supervised one-shot segmentation task representing a more data-scarce scenario. We adopt a pre-existing self-supervised few-shot segmentation framework CoWPro and adapt it to the federated learning scenario. To the best of our knowledge, this work is the first to attempt a self-supervised few-shot segmentation task in the federated learning domain. Moreover, we consider the clients to be constituted of data from different modalities and imaging techniques like MR or CT, which makes the problem even harder. Additionally, we reinforce and improve the baseline CoWPro method using a fused dice loss which shows considerable improvement in performance over the baseline CoWPro. Finally, we evaluate this novel framework on a completely unseen held-out part of the local client dataset. We observe that the proposed framework can achieve performance at par or better than the FedAvg version of the CoWPro framework on the held-out validation dataset.
去中心化联邦学习能够在不损害客户端隐私的情况下,从多个来源学习数据表示。在医学图像分割等应用中,从单一来源获取大量标注数据集是一个令人头疼的问题,联邦自监督学习可以提供一些慰藉。在这项工作中,我们通过探索联邦自监督一次性分割任务,进一步拓展了界限,这代表了数据更加稀缺的场景。我们采用预先存在的自监督少样本分割框架CoWPro,并将其适应于联邦学习场景。据我们所知,这是首次尝试在联邦学习领域进行自监督少样本分割任务。此外,我们认为客户端由不同模态和数据成像技术(如MR或CT)构成,这使得问题更加复杂。另外,我们使用融合Dice损失来加强和改进基线CoWPro方法,与基线CoWPro相比,这在性能上显示出显著改进。最后,我们在本地客户端数据集的完全未见过的保留部分上评估了这种新型框架。我们发现,在保留的验证数据集上,所提出的框架的性能可以达到或超过CoWPro框架的FedAvg版本。
论文及项目相关链接
Summary
本摘要探讨了分布式联邦学习在医疗图像分割领域的应用。通过采用联邦自监督学习,能够在数据稀缺的情况下从多个源学习数据表示,同时保护客户端隐私。研究团队首次尝试在联邦学习领域进行自监督少样本分割任务,并改进了现有的CoWPro框架,以适应不同模态和数据采集技术的客户端数据。此外,采用融合Dice损失方法,在性能上取得了显著提升,并在本地客户端数据集的未见部分进行了评估,表现出良好的性能。
Key Takeaways
- 分布式联邦学习允许从多个源学习数据表示,同时保护客户端隐私。
- 在医疗图像分割等应用中,联邦自监督学习是解决单一来源大数据集获取困难的有效方法。
- 研究首次尝试在联邦学习领域进行自监督少样本分割任务。
- 改进了现有的CoWPro框架以适应联邦学习场景。
- 考虑到不同模态和数据采集技术的客户端数据,增加了问题的复杂性。
- 采用融合Dice损失方法,显著提高了性能。
点此查看论文截图
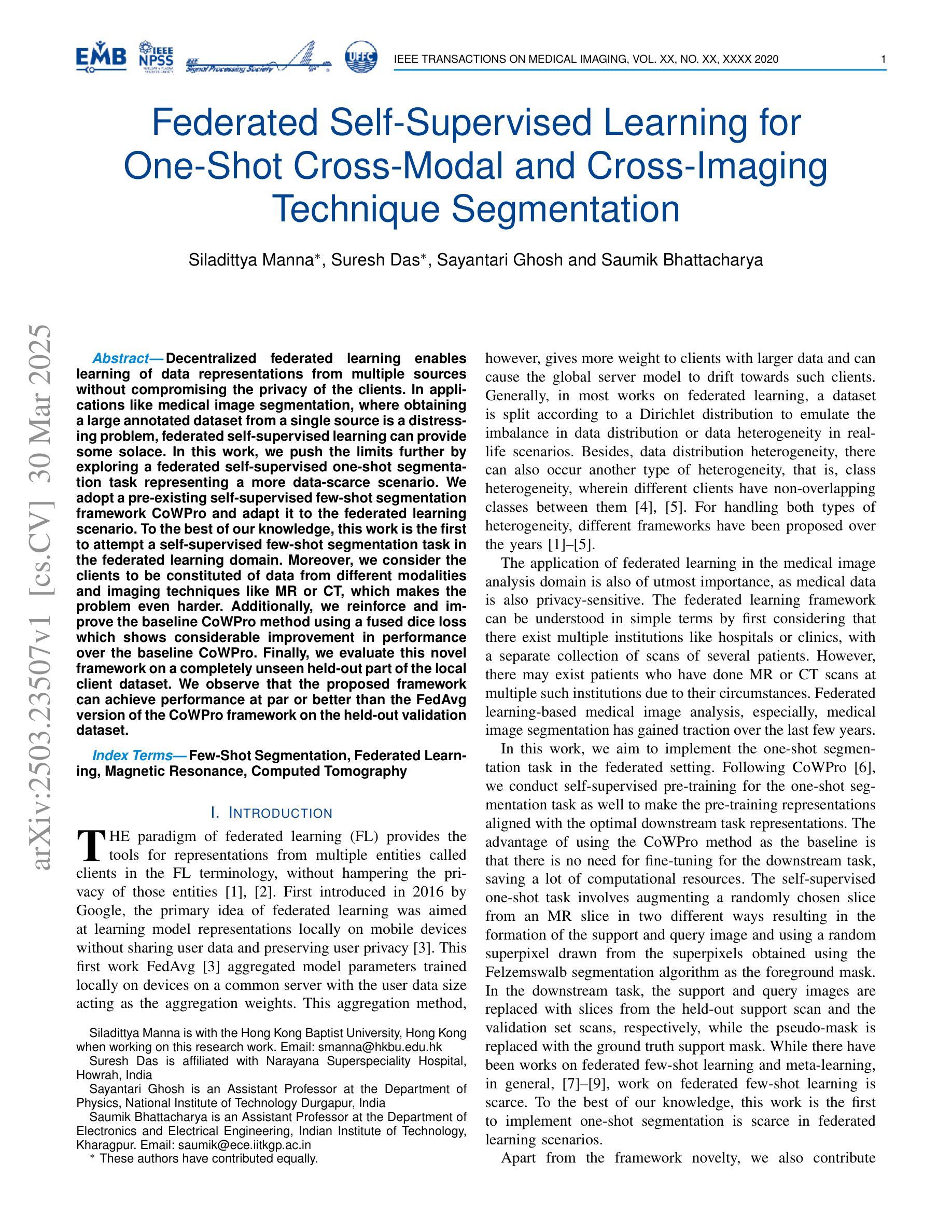
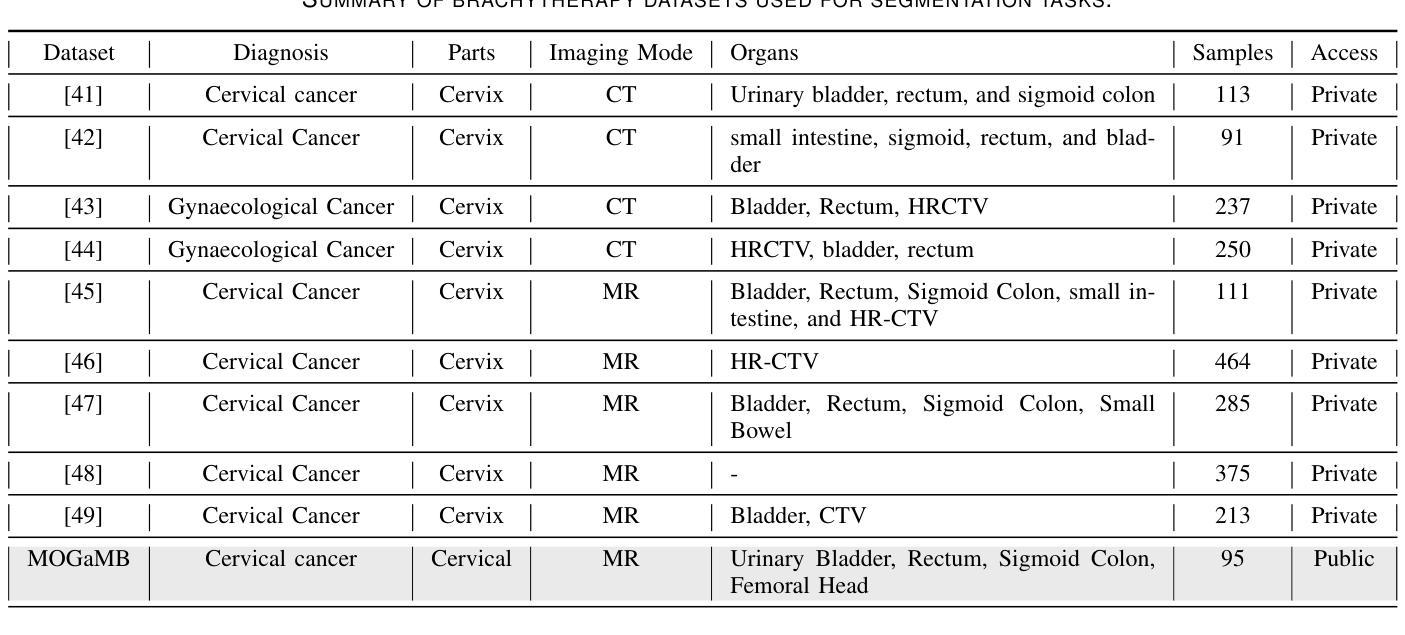
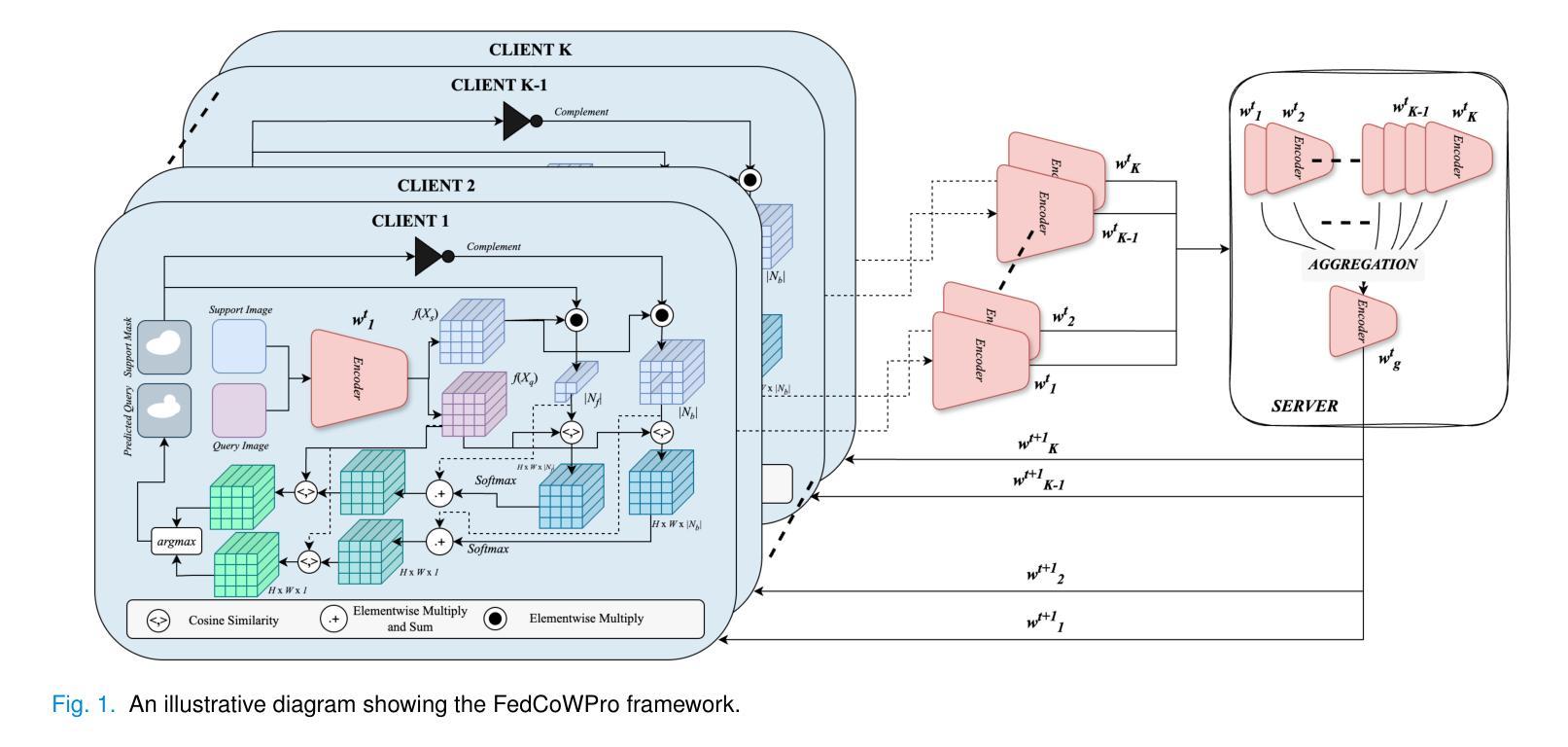
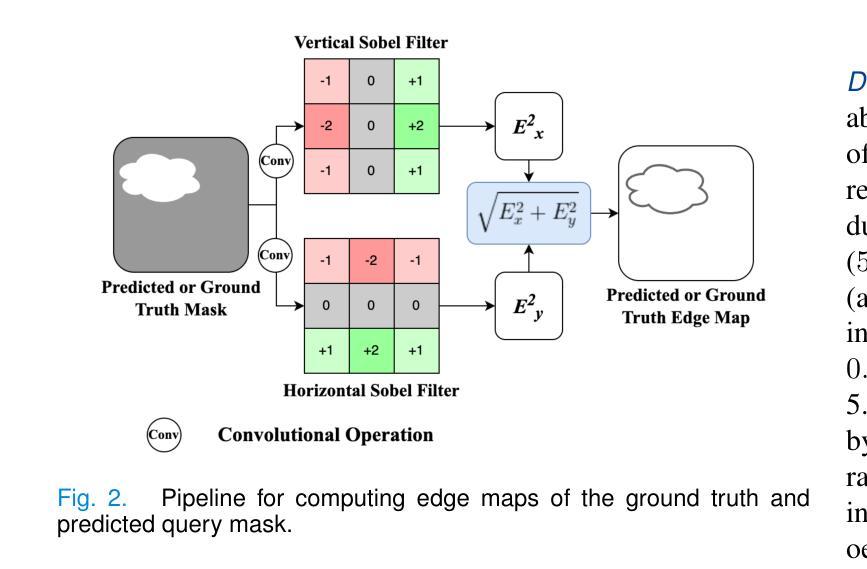
Evolutionary Prompt Optimization Discovers Emergent Multimodal Reasoning Strategies in Vision-Language Models
Authors:Sid Bharthulwar, John Rho, Katrina Brown
We present a framework for optimizing prompts in vision-language models to elicit multimodal reasoning without model retraining. Using an evolutionary algorithm to guide prompt updates downstream of visual tasks, our approach improves upon baseline prompt-updating algorithms, which lack evolution-style “survival of the fittest” iteration. Crucially, we find this approach enables the language model to independently discover progressive problem-solving techniques across several evolution generations. For example, the model reasons that to “break down” visually complex spatial tasks, making a tool call to a Python interpreter to perform tasks (such as cropping, image segmentation, or saturation changes) would improve performance significantly. Our experimentation shows that explicitly evoking this “tool calling” call, via system-level XML $…\texttt{
我们提出了一种优化视觉语言模型提示的框架,以激发多模态推理而无需重新训练模型。我们使用进化算法来指导视觉任务下游的提示更新,我们的方法改进了基线提示更新算法,后者缺乏进化式的“适者生存”迭代。关键的是,我们发现这种方法使语言模型能够在多个进化代数中独立地发现渐进的问题解决技术。例如,模型推理出为了“分解”视觉上复杂的空间任务,调用Python解释器执行一些任务(如裁剪、图像分割或饱和度更改)将大大提高性能。我们的实验表明,通过系统级别的XML
<tool>
…<tool>
标签显式触发这种“工具调用”,可以有效地标记语言模型访问Python解释器以生成相关程序,从而产生高级的多模态功能。此功能可以提炼成系统级的提示,在推理时间时提高性能,我们的实验表明在某些视觉任务上相对改进了约50%。下游性能是在MathVista、M3CoT和GeoBench-VLM数据集的任务中进行训练和评估的。重要的是,我们的方法表明,进化提示优化可以引导语言模型进行自推理发现,从而导致任务间的零样本泛化能力得到提高。
论文及项目相关链接
PDF Published at ICLR 2025 Workshop on Reasoning and Planning for LLMs
Summary
该研究提出了一种优化视觉语言模型提示的框架,无需重新训练模型即可激发多模态推理。该研究采用进化算法引导视觉任务下游的提示更新,改进了基线提示更新算法。进化式的“适者生存”迭代使语言模型能够独立发现渐进的问题解决技术,并通过系统级别的提示标签,如XML中的
Key Takeaways
- 研究提出了一种优化视觉语言模型提示的框架,以激发多模态推理,而无需重新训练模型。
- 采用进化算法引导视觉任务下游的提示更新,改进了基线提示更新算法。
- 语言模型能够独立发现渐进的问题解决技术,并通过系统级别的提示标签调用Python解释器执行特定任务。
- 通过系统级提示(如XML中的
和 标签),可以有效提高语言模型在推理时间内的性能。 - 实验表明,该框架在MathVista、M3CoT和GeoBench-VLM数据集上实现了显著的性能改进,相对改进约为50%。
- 进化提示优化有助于语言模型的自我推理发现,提高了跨任务的零样本泛化能力。
- 该框架具有广泛的应用潜力,可以应用于其他视觉语言任务中。
点此查看论文截图
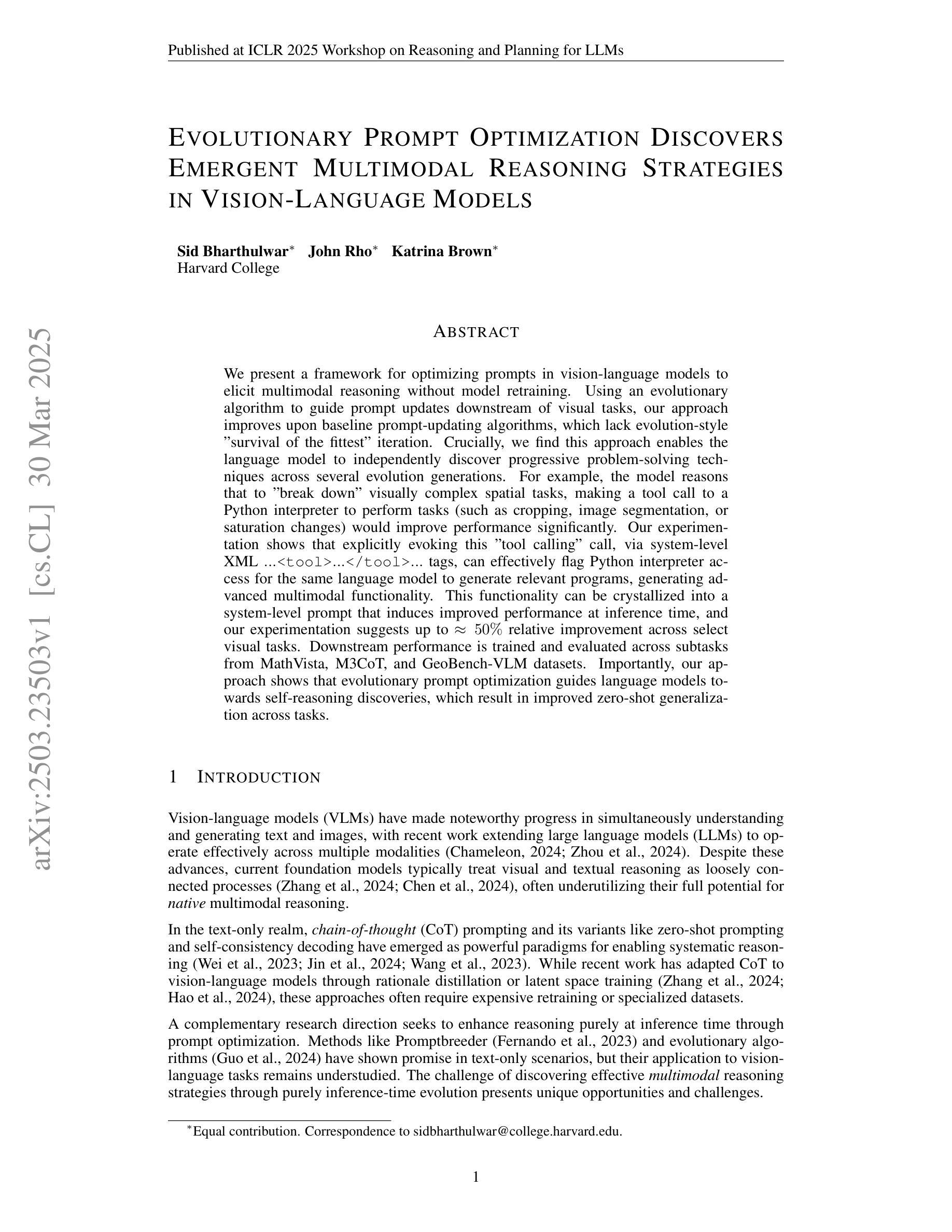
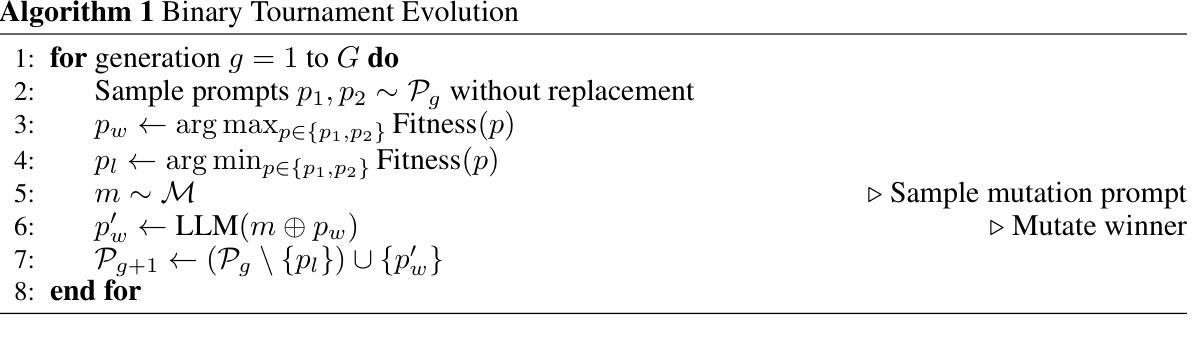
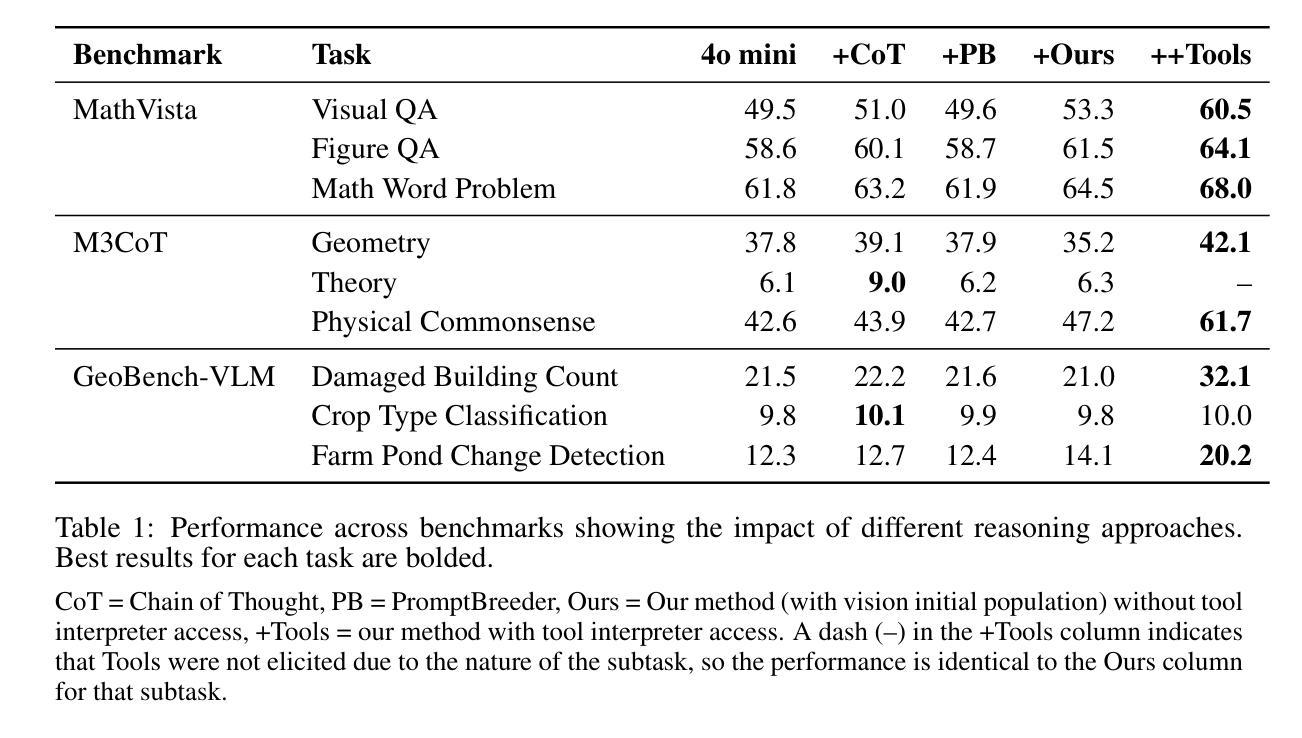