⚠️ 以下所有内容总结都来自于 大语言模型的能力,如有错误,仅供参考,谨慎使用
🔴 请注意:千万不要用于严肃的学术场景,只能用于论文阅读前的初筛!
💗 如果您觉得我们的项目对您有帮助 ChatPaperFree ,还请您给我们一些鼓励!⭐️ HuggingFace免费体验
2025-04-04 更新
Dist Loss: Enhancing Regression in Few-Shot Region through Distribution Distance Constraint
Authors:Guangkun Nie, Gongzheng Tang, Shenda Hong
Imbalanced data distributions are prevalent in real-world scenarios, posing significant challenges in both imbalanced classification and imbalanced regression tasks. They often cause deep learning models to overfit in areas of high sample density (many-shot regions) while underperforming in areas of low sample density (few-shot regions). This characteristic restricts the utility of deep learning models in various sectors, notably healthcare, where areas with few-shot data hold greater clinical relevance. While recent studies have shown the benefits of incorporating distribution information in imbalanced classification tasks, such strategies are rarely explored in imbalanced regression. In this paper, we address this issue by introducing a novel loss function, termed Dist Loss, designed to minimize the distribution distance between the model’s predictions and the target labels in a differentiable manner, effectively integrating distribution information into model training. Dist Loss enables deep learning models to regularize their output distribution during training, effectively enhancing their focus on few-shot regions. We have conducted extensive experiments across three datasets spanning computer vision and healthcare: IMDB-WIKI-DIR, AgeDB-DIR, and ECG-Ka-DIR. The results demonstrate that Dist Loss effectively mitigates the negative impact of imbalanced data distribution on model performance, achieving state-of-the-art results in sparse data regions. Furthermore, Dist Loss is easy to integrate, complementing existing methods.
不平衡数据分布在实际场景中普遍存在,给不平衡分类和不平衡回归任务带来了重大挑战。它们经常导致深度学习模型在高样本密度区域(多镜头区域)过度拟合,而在低样本密度区域(少镜头区域)表现不佳。这一特点限制了深度学习模型在各行业的应用价值,尤其在医疗领域,少镜头数据区域具有更大的临床意义。虽然最近的研究表明在不平衡分类任务中融入分布信息具有优势,但在不平衡回归中很少探索此类策略。本文旨在解决这个问题,通过引入一种新型损失函数(称为Dist Loss),旨在以可区分的方式最小化模型预测与目标标签之间的分布距离,有效地将分布信息集成到模型训练中。Dist Loss使深度学习模型能够在训练过程中规范其输出分布,有效提高对少镜头区域的关注度。我们在跨越计算机视觉和医疗领域的三个数据集上进行了广泛实验:IMDB-WIKI-DIR、AgeDB-DIR和ECG-Ka-DIR。结果表明,Dist Loss有效减轻了不平衡数据分布对模型性能的负面影响,在稀疏数据区域取得了最先进的成果。此外,Dist Loss易于集成,可以辅助现有方法。
论文及项目相关链接
Summary
深度学习模型在处理不平衡数据分布时面临挑战,特别是在分类和回归任务中。新提出的Dist Loss损失函数能够最小化模型预测与目标标签之间的分布距离,提高模型在样本稀少区域的性能。实验结果表明,Dist Loss能有效缓解不平衡数据分布对模型性能的负面影响,实现稀疏数据区域的最佳性能。
Key Takeaways
- 不平衡数据分布在现实场景中普遍存在,对深度学习的分类和回归任务带来挑战。
- 深度学习模型在样本密集区域容易过拟合,而在样本稀少区域表现不佳。
- Dist Loss是一种新型损失函数,旨在最小化模型预测与目标标签之间的分布距离。
- Dist Loss能够使深度学习模型在训练过程中正则化其输出分布,从而提高对样本稀少区域的关注。
- Dist Loss易于集成,可以与其他方法相结合。
- 实验结果表明,Dist Loss能有效提高模型在稀疏数据区域的性能。
点此查看论文截图
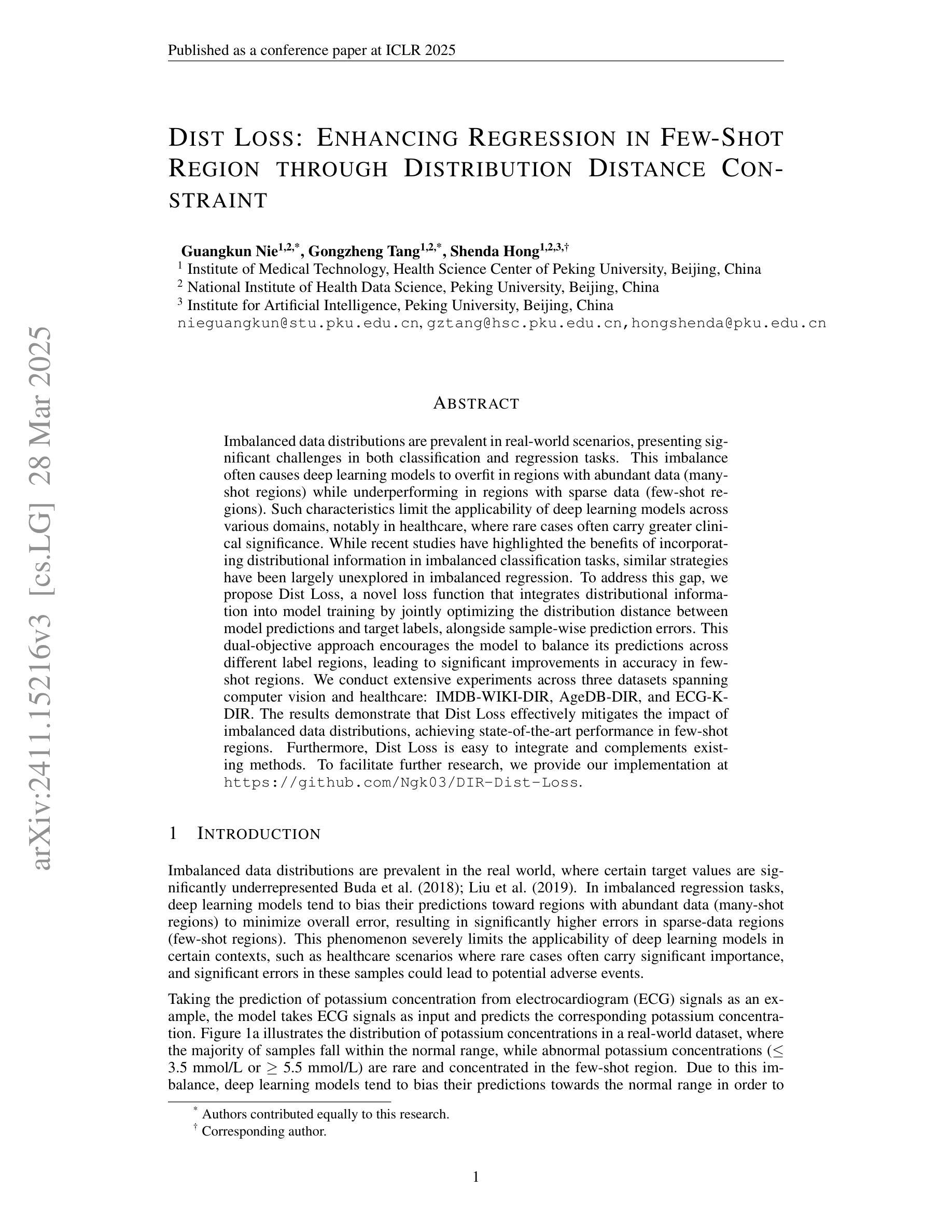
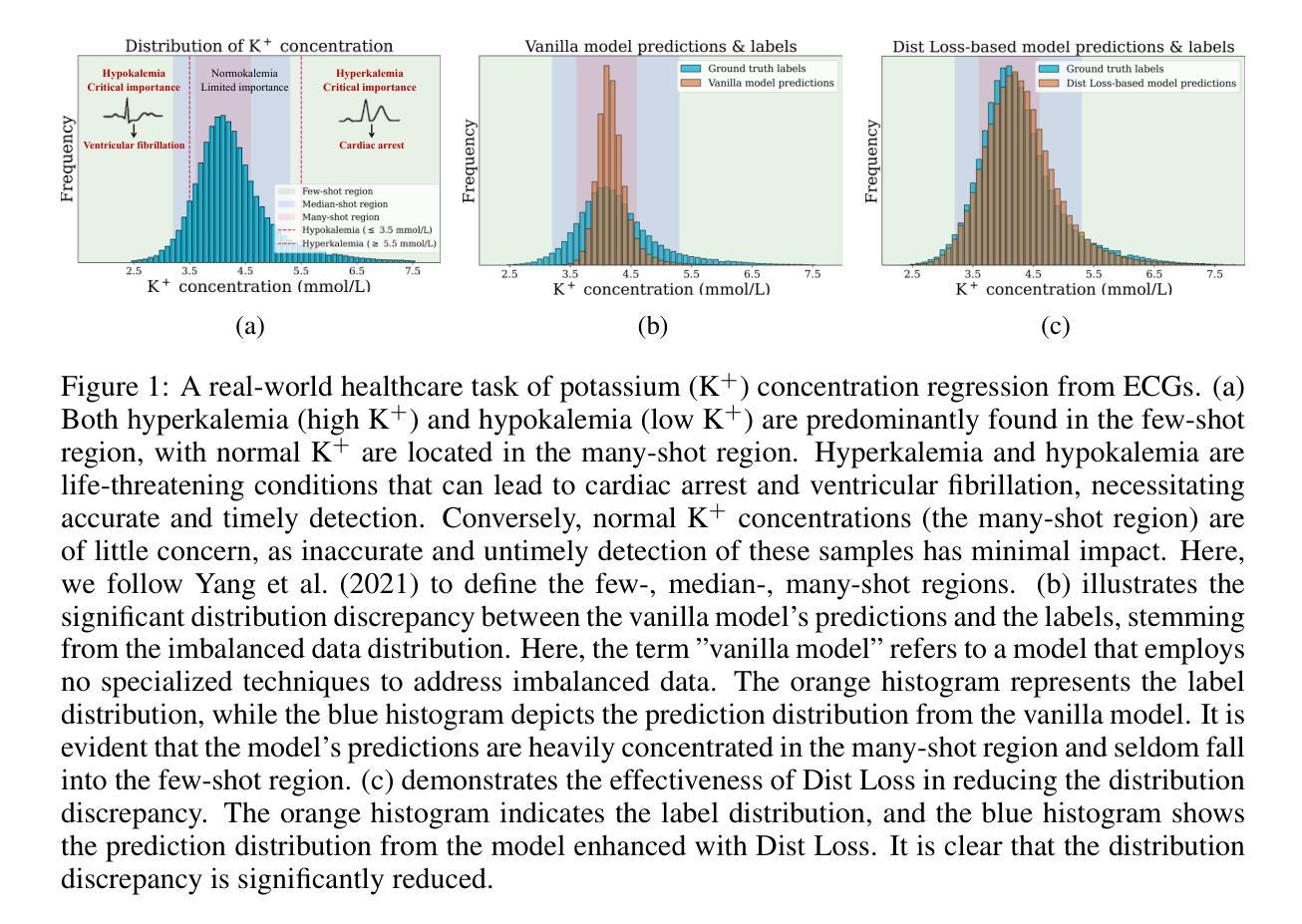
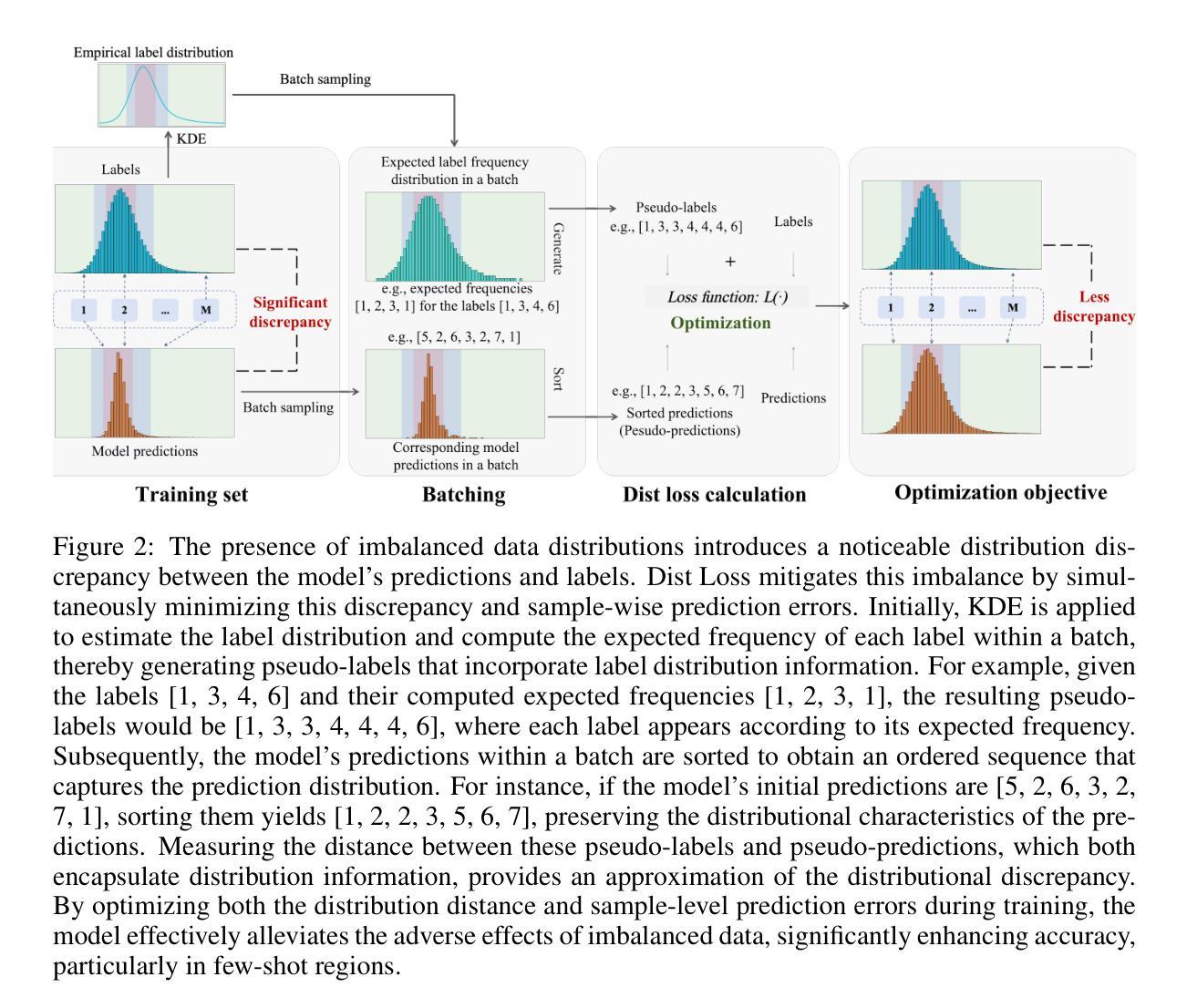
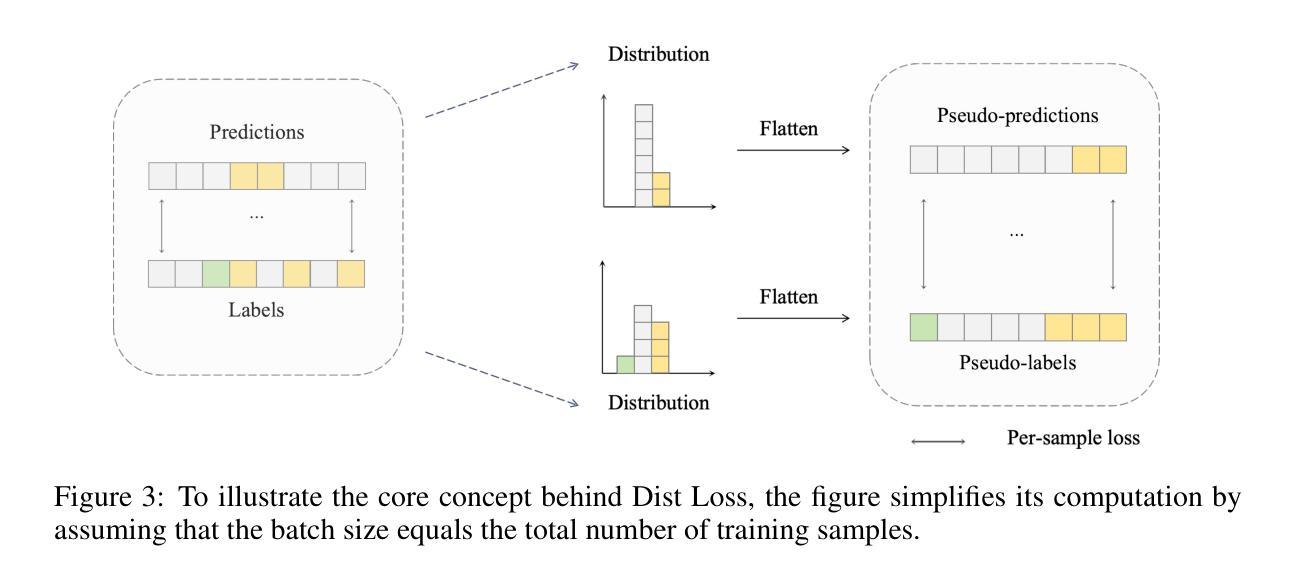
One Policy to Run Them All: an End-to-end Learning Approach to Multi-Embodiment Locomotion
Authors:Nico Bohlinger, Grzegorz Czechmanowski, Maciej Krupka, Piotr Kicki, Krzysztof Walas, Jan Peters, Davide Tateo
Deep Reinforcement Learning techniques are achieving state-of-the-art results in robust legged locomotion. While there exists a wide variety of legged platforms such as quadruped, humanoids, and hexapods, the field is still missing a single learning framework that can control all these different embodiments easily and effectively and possibly transfer, zero or few-shot, to unseen robot embodiments. We introduce URMA, the Unified Robot Morphology Architecture, to close this gap. Our framework brings the end-to-end Multi-Task Reinforcement Learning approach to the realm of legged robots, enabling the learned policy to control any type of robot morphology. The key idea of our method is to allow the network to learn an abstract locomotion controller that can be seamlessly shared between embodiments thanks to our morphology-agnostic encoders and decoders. This flexible architecture can be seen as a potential first step in building a foundation model for legged robot locomotion. Our experiments show that URMA can learn a locomotion policy on multiple embodiments that can be easily transferred to unseen robot platforms in simulation and the real world.
深度强化学习技术正在实现最新的稳健型腿足运动学研究成果。尽管存在多种腿足平台,如四足、人形和六足等,但该领域仍然缺乏一个单一的学习框架,能够轻松有效地控制所有这些不同的体现形式,并可能以零或少数几次转移的方式应用到未见过的机器人体现形式上。我们引入URMA,即统一机器人形态架构,以弥补这一空白。我们的框架将端到端多任务强化学习方法引入到腿足机器人的领域,使得学习到的策略能够控制任何类型的机器人形态。我们的方法的关键思想是让网络学习一个抽象的步态控制器,该控制器可以无缝地在各种形态之间共享,这得益于我们的形态无关编码器和解码器。这种灵活架构可以被视为构建腿足机器人运动基础模型的第一步。我们的实验表明,URMA可以在多种体现形式上学习步态策略,并且可以轻松地将其转移到仿真和实际世界中的未见过的机器人平台上。
论文及项目相关链接
Summary
深度强化学习在稳健的腿部运动方面取得了最新的成果。尽管存在多种腿部平台,如四足、人形和六足等,但领域里仍缺乏一个单一的学习框架,能够轻松有效地控制所有这些不同的体现形式,并可能零成本或少成本转移到看不见的机器人体现形式。我们推出URMA,即统一机器人形态架构,以弥补这一空白。我们的框架将终端到终端的多任务强化学习带到了腿部机器人领域,使学习到的策略能够控制任何类型的机器人形态。我们的方法的关键思想是让网络学习一个抽象的步态控制器,由于我们的形态无关编码器和解码器,该控制器可以在形态之间无缝共享。这一灵活架构可以看作是构建腿部机器人运动基础模型的一个潜在的第一步。实验表明,URMA可以在多种形态上学习步态策略,并能轻松地在仿真和真实环境中转移到未知的机器人平台上。
Key Takeaways
- 深度强化学习在腿部运动方面取得最新成果。
- 存在多种腿部平台,但缺乏一个统一的学习框架来控制它们。
- URMA(统一机器人形态架构)填补了这一空白。
- URMA框架采用端到端的多任务强化学习。
- 网络学习抽象的步态控制器,可在不同的机器人形态之间无缝共享。
- URMA的灵活架构是构建腿部机器人运动基础模型的初步尝试。
- 实验显示URMA能在多种形态上学习步态策略,并轻松转移到未知机器人平台。
点此查看论文截图
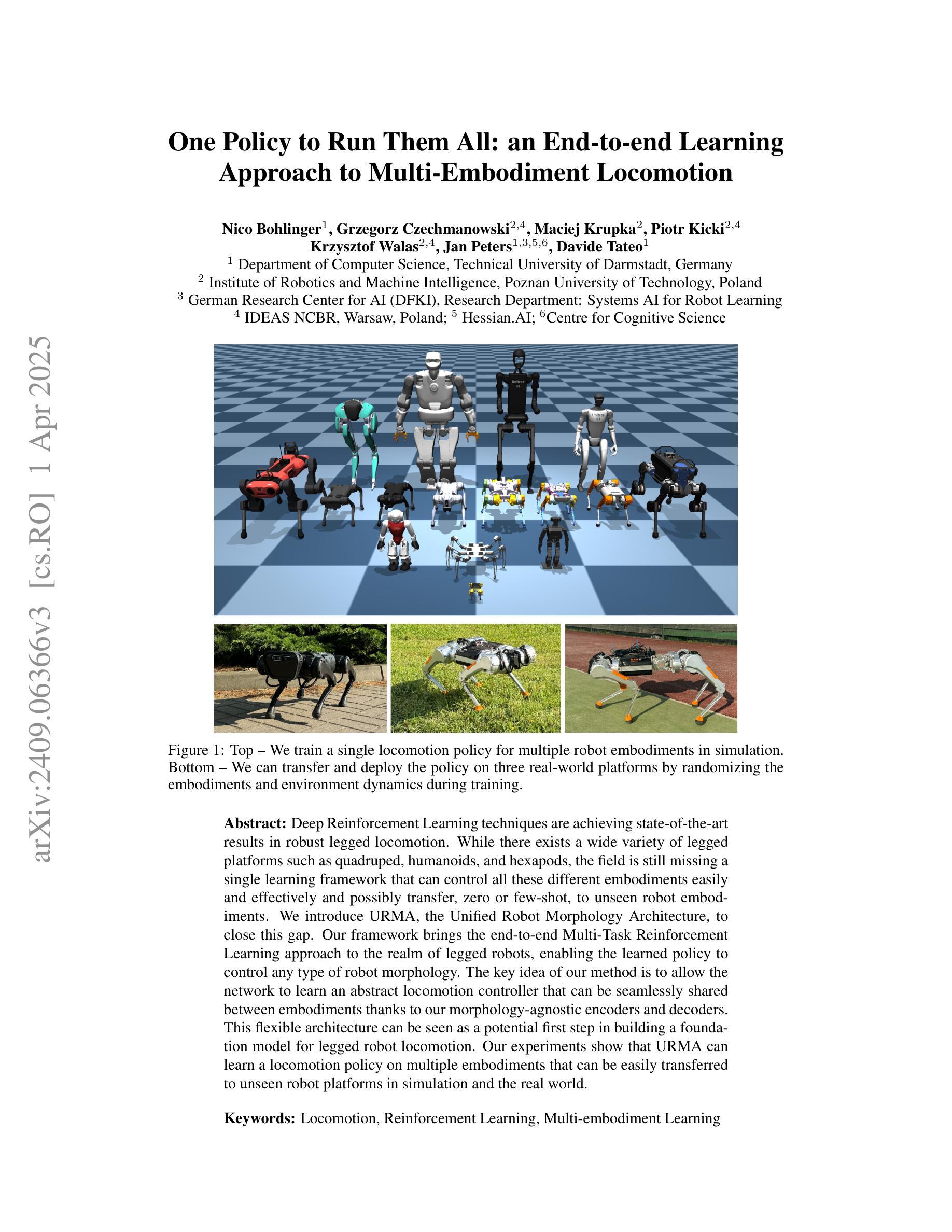
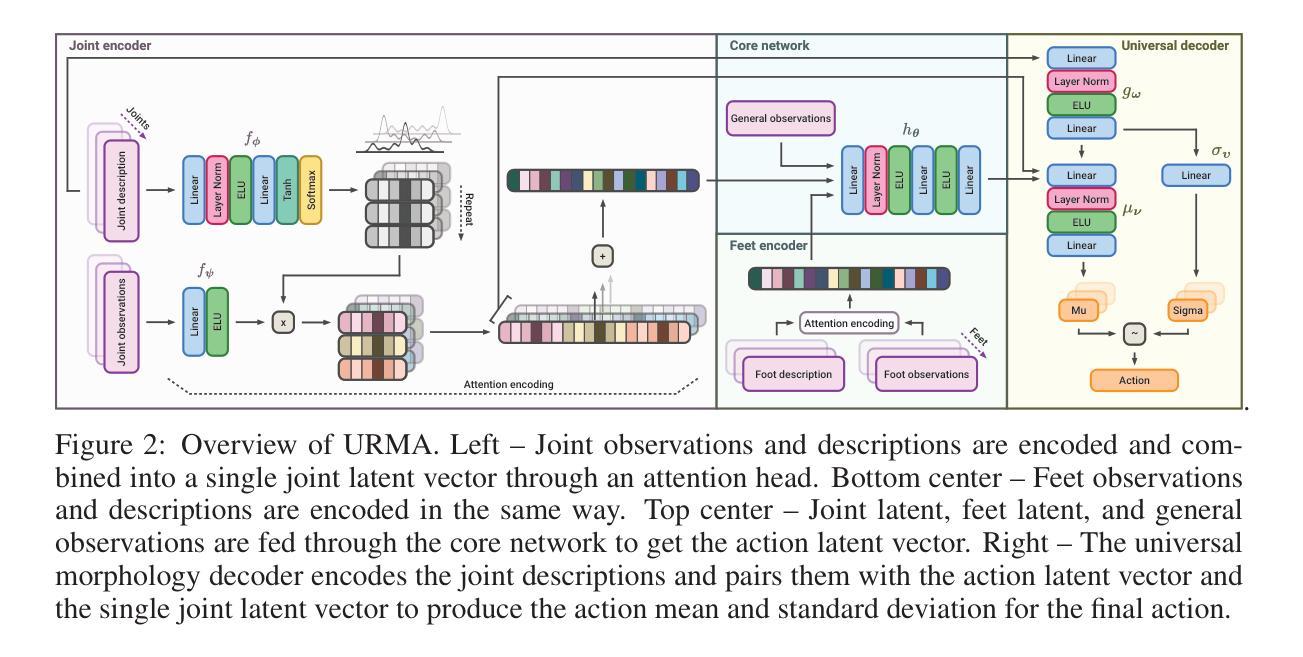
Open-FinLLMs: Open Multimodal Large Language Models for Financial Applications
Authors:Jimin Huang, Mengxi Xiao, Dong Li, Zihao Jiang, Yuzhe Yang, Yifei Zhang, Lingfei Qian, Yan Wang, Xueqing Peng, Yang Ren, Ruoyu Xiang, Zhengyu Chen, Xiao Zhang, Yueru He, Weiguang Han, Shunian Chen, Lihang Shen, Daniel Kim, Yangyang Yu, Yupeng Cao, Zhiyang Deng, Haohang Li, Duanyu Feng, Yongfu Dai, VijayaSai Somasundaram, Peng Lu, Guojun Xiong, Zhiwei Liu, Zheheng Luo, Zhiyuan Yao, Ruey-Ling Weng, Meikang Qiu, Kaleb E Smith, Honghai Yu, Yanzhao Lai, Min Peng, Jian-Yun Nie, Jordan W. Suchow, Xiao-Yang Liu, Benyou Wang, Alejandro Lopez-Lira, Qianqian Xie, Sophia Ananiadou, Junichi Tsujii
Financial LLMs hold promise for advancing financial tasks and domain-specific applications. However, they are limited by scarce corpora, weak multimodal capabilities, and narrow evaluations, making them less suited for real-world application. To address this, we introduce \textit{Open-FinLLMs}, the first open-source multimodal financial LLMs designed to handle diverse tasks across text, tabular, time-series, and chart data, excelling in zero-shot, few-shot, and fine-tuning settings. The suite includes FinLLaMA, pre-trained on a comprehensive 52-billion-token corpus; FinLLaMA-Instruct, fine-tuned with 573K financial instructions; and FinLLaVA, enhanced with 1.43M multimodal tuning pairs for strong cross-modal reasoning. We comprehensively evaluate Open-FinLLMs across 14 financial tasks, 30 datasets, and 4 multimodal tasks in zero-shot, few-shot, and supervised fine-tuning settings, introducing two new multimodal evaluation datasets. Our results show that Open-FinLLMs outperforms afvanced financial and general LLMs such as GPT-4, across financial NLP, decision-making, and multi-modal tasks, highlighting their potential to tackle real-world challenges. To foster innovation and collaboration across academia and industry, we release all codes (https://anonymous.4open.science/r/PIXIU2-0D70/B1D7/LICENSE) and models under OSI-approved licenses.
金融LLM(大型预训练语言模型)在推进金融任务和特定领域应用方面展现出巨大的潜力。然而,它们受到有限语料库、弱多模态能力和狭窄评估范围的限制,使得它们不太适合实际应用。为了解决这个问题,我们推出了”Open-FinLLMs”,这是首个开源的多模态金融LLM,旨在处理文本、表格、时间序列和图表数据等多样任务,并在零样本、少样本和微调场景中表现出色。该套件包括在全面的52亿令牌语料库上预训练的FinLLaMA、使用573K金融指令进行微调的FinLLaMA-Instruct以及通过143万对多模态调优增强功能的FinLLaVA,以实现强大的跨模态推理。我们全面评估了Open-FinLLMs在14个金融任务、30个数据集和4个多模态任务中的表现,包括零样本、少样本和监督微调场景,并引入了两个新的多模态评估数据集。我们的结果表明,Open-FinLLMs在金融NLP、决策制定和多模态任务方面优于先进的金融和通用LLM,如GPT-4,凸显了它们解决现实挑战的潜力。为了促进学术界和产业界的创新和合作,我们发布了所有代码(https://anonymous.4open.science/r/PIXIU2-0D70/B1D7/LICENSE)和模型,并遵循OSI批准许可证。
论文及项目相关链接
PDF 33 pages, 13 figures
Summary
金融LLM在推进金融任务和特定领域应用方面有很大潜力,但受限于稀缺语料库、弱多模态能力和狭窄评估范围,不太适合现实世界应用。为解决这一问题,我们推出Open-FinLLMs,首个开源多模态金融LLM,可处理文本、表格、时间序列和图表数据的多样化任务,在零样本、少样本和微调设置中都表现出色。包括基于52亿令牌语料库的预训练FinLLaMA、以57.3万条金融指令精细调教的FinLLaMA-Instruct,以及通过143万对多模态调优增强能力的FinLLaVA。我们全面评估了Open-FinLLMs在14个金融任务、30个数据集和4个多模态任务中的表现,并引入两个新的多模态评估数据集。结果表明,Open-FinLLMs在金融NLP、决策和多模态任务方面优于GPT-4等先进金融和通用LLM,展现出解决现实挑战的巨大潜力。我们发布所有代码和模型,以促进学术界和产业界的创新和合作。
Key Takeaways
- 金融LLM具有推进金融任务和领域特定应用的潜力。
- 当前金融LLM受限于稀缺语料库、弱多模态能力和狭窄评估。
- Open-FinLLMs旨在解决这些问题,具备处理多样化任务的能力。
- Open-FinLLMs包括预训练的FinLLaMA、精细调教的FinLLaMA-Instruct和增强多模态推理的FinLLaVA。
- 在多个金融任务和多模态任务中,Open-FinLLMs表现出超越先进金融和通用LLM的潜力。
- Open-FinLLMs的推出旨在促进学术界和产业界的合作与创新。
点此查看论文截图
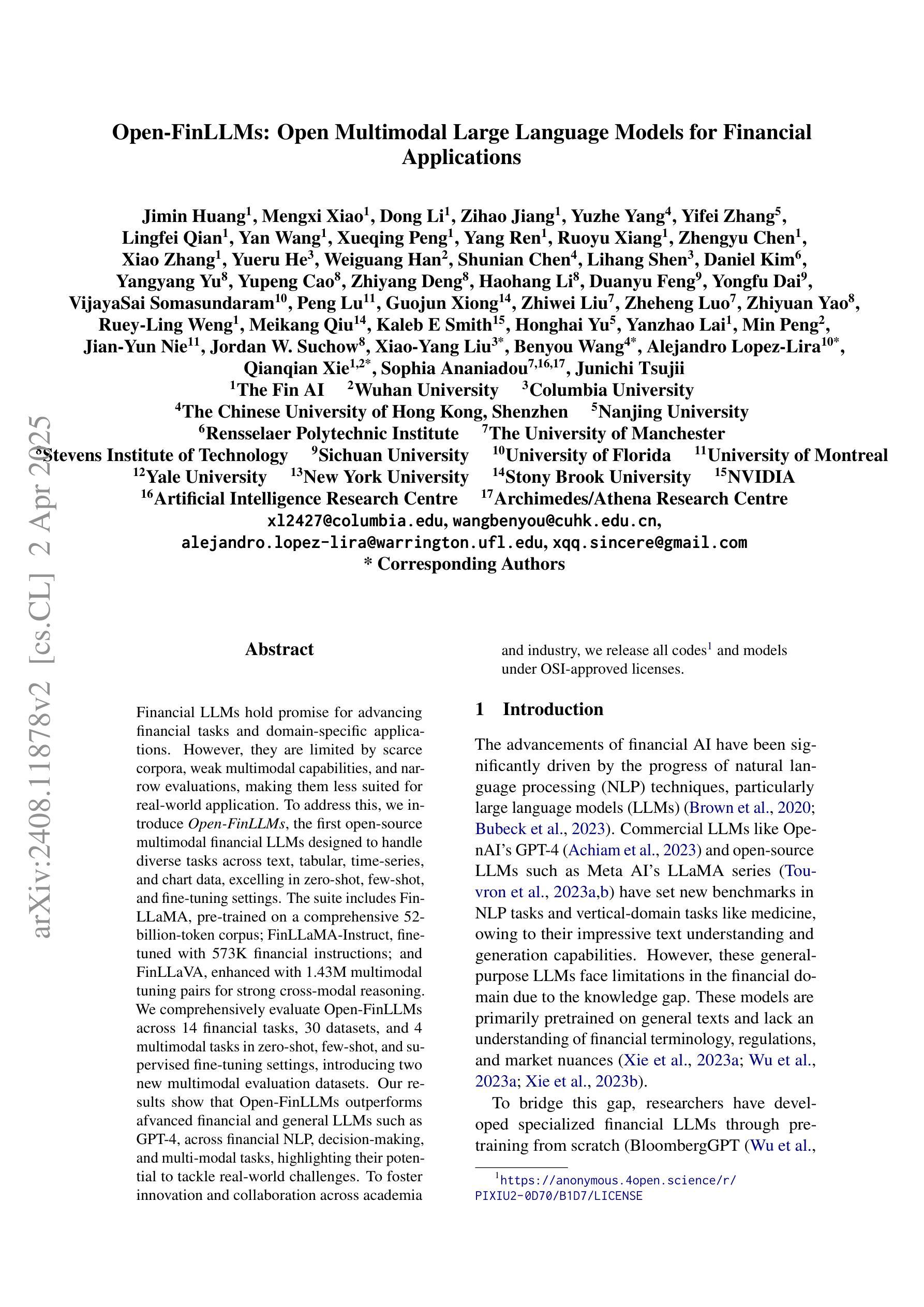
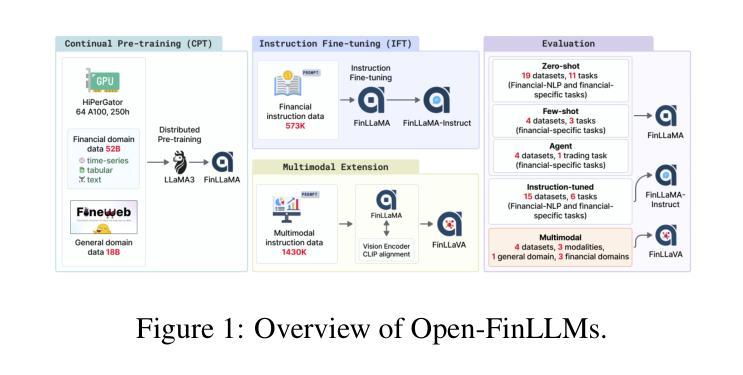
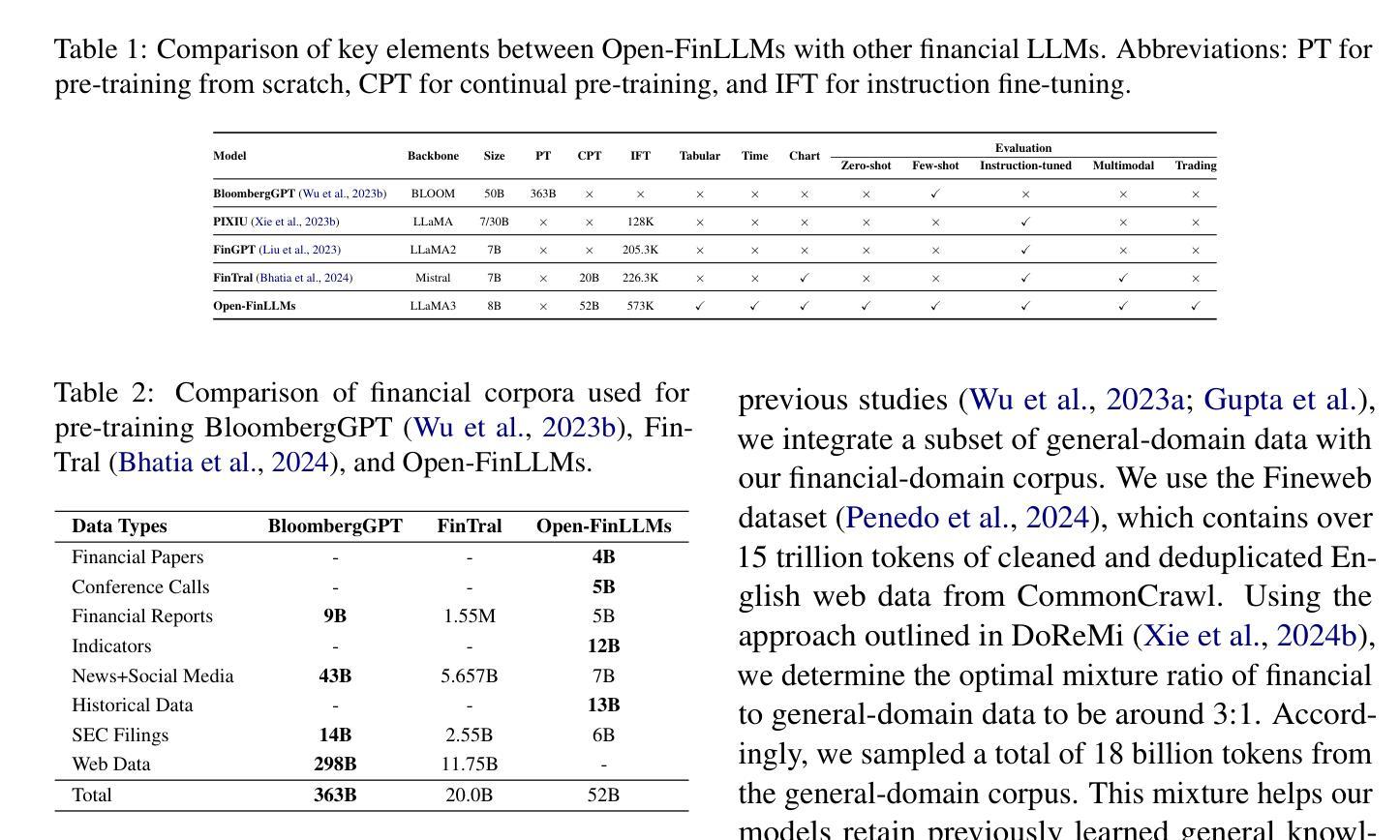
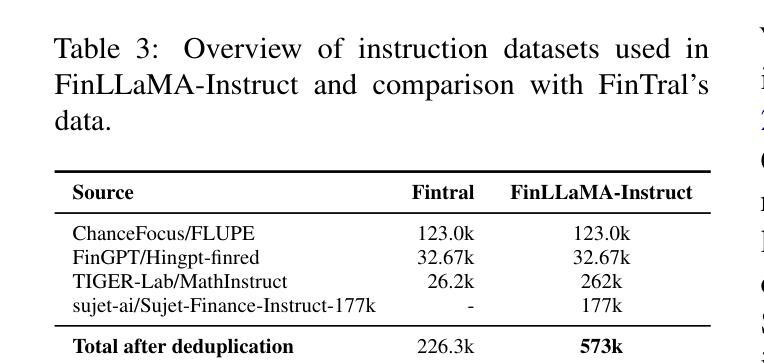
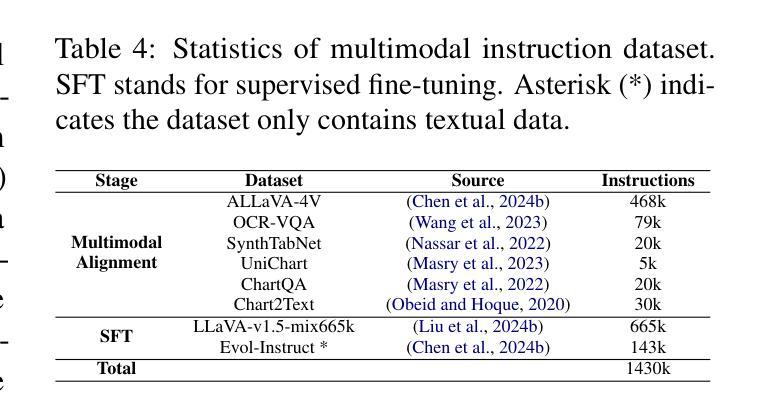
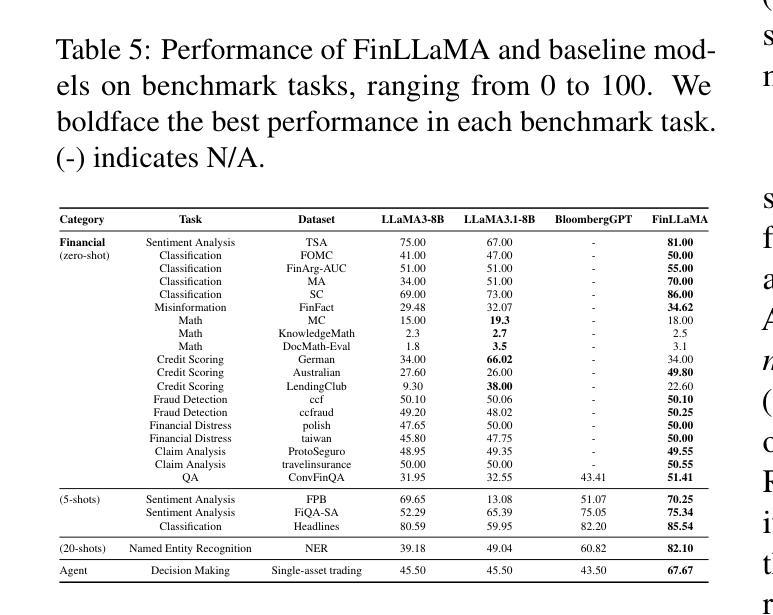
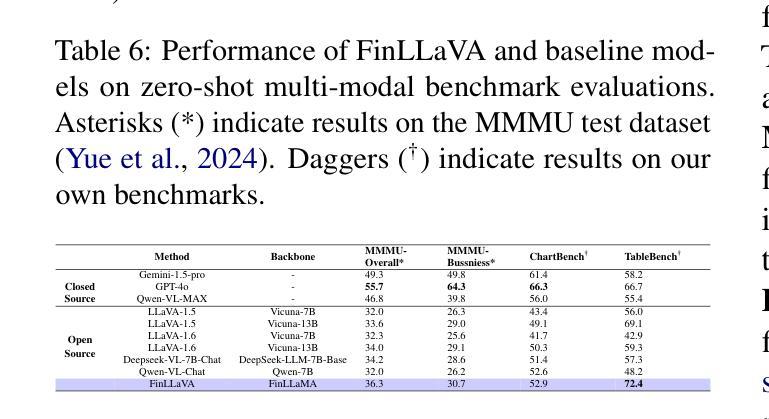
Non-Determinism of “Deterministic” LLM Settings
Authors:Berk Atil, Sarp Aykent, Alexa Chittams, Lisheng Fu, Rebecca J. Passonneau, Evan Radcliffe, Guru Rajan Rajagopal, Adam Sloan, Tomasz Tudrej, Ferhan Ture, Zhe Wu, Lixinyu Xu, Breck Baldwin
LLM (large language model) practitioners commonly notice that outputs can vary for the same inputs under settings expected to be deterministic. Yet the questions of how pervasive this is, and with what impact on results, have not to our knowledge been systematically investigated. We investigate non-determinism in five LLMs configured to be deterministic when applied to eight common tasks in across 10 runs, in both zero-shot and few-shot settings. We see accuracy variations up to 15% across naturally occurring runs with a gap of best possible performance to worst possible performance up to 70%. In fact, none of the LLMs consistently delivers repeatable accuracy across all tasks, much less identical output strings. Sharing preliminary results with insiders has revealed that non-determinism perhaps essential to the efficient use of compute resources via co-mingled data in input buffers so this issue is not going away anytime soon. To better quantify our observations, we introduce metrics focused on quantifying determinism, TARr@N for the total agreement rate at N runs over raw output, and TARa@N for total agreement rate of parsed-out answers. Our code and data are publicly available at https://github.com/breckbaldwin/llm-stability.
大型语言模型(LLM)的实践者通常注意到,在预期为确定性设置的条件下,相同输入的输出了可能会发生变动。然而,至于这种现象的普遍性以及对结果的影响程度,据我们了解尚未有系统性的研究。我们在五个被配置为确定性的LLM上进行了调查,这些LLM在零样本和少样本设置下应用于八个常见任务,并进行了十次运行。我们看到自然运行之间的准确率变动高达15%,最佳可能性能与最差可能性能之间的差距高达70%。事实上,没有任何一个LLM在所有任务上都能提供一致的准确率,更别提输出完全相同的字符串了。与业内专家的初步结果分享显示,非确定性对于通过输入缓冲区中的混合数据实现计算资源的有效利用可能是至关重要的,因此这一问题在短期内不会得到解决。为了更好地量化我们的观察结果,我们引入了专注于量化确定性的指标,包括TARr@N(N次运行中原始输出的总协议率)和TARa@N(解析出的答案的总协议率)。我们的代码和数据可在https://github.com/breckbaldwin/llm-stability上公开访问。
论文及项目相关链接
Summary
大型语言模型(LLM)在实践中表现出非确定性行为,对相同输入的输出在不同运行设置下会有变化。本文对此进行了系统调查,在五个LLM模型中对八个常见任务进行十次运行测试,发现在零样本和少样本设置下,准确度变化高达15%,最佳与最差性能之间的差距最大可达70%。共享初步结果表明,非确定性对于有效利用计算资源可能是必要的。本文引入了专注于量化确定性的指标TARr@N和TARa@N,分别用于衡量原始输出和解析答案的共识程度。
Key Takeaways
- LLM模型在实践中表现出非确定性行为,即相同输入在不同运行设置下会产生不同的输出。
- 系统调查了五个LLM模型在八个常见任务中的非确定性表现,发现准确度变化大,最佳与最差性能差距显著。
- LLM模型在所有任务上无法保持一致的准确性,且很少产生完全相同的输出字符串。
- 非确定性对于计算资源的有效利用可能是必要的,可能与输入缓冲区中的混合数据有关。
- 引入TARr@N和TARa@N指标,用于量化LLM模型的确定性评估。
- 该研究的代码和数据已公开可用。
- 此问题(非确定性)在短期内不会得到解决。
点此查看论文截图
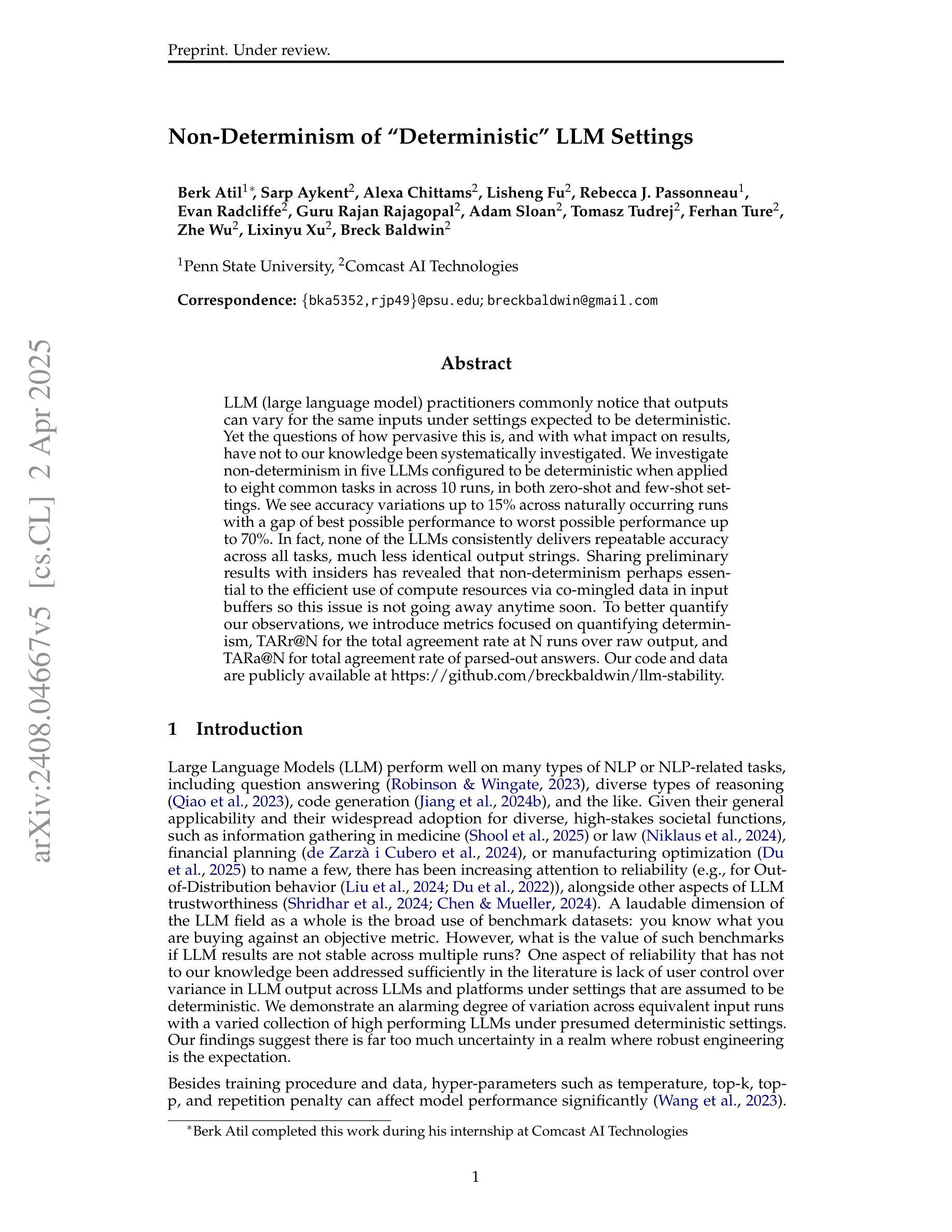
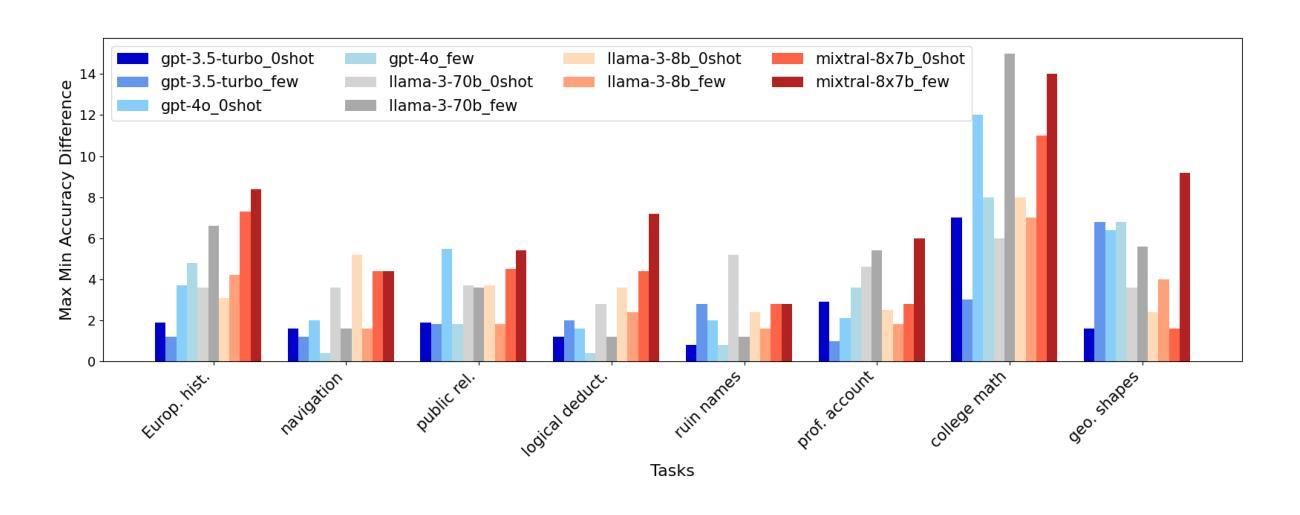
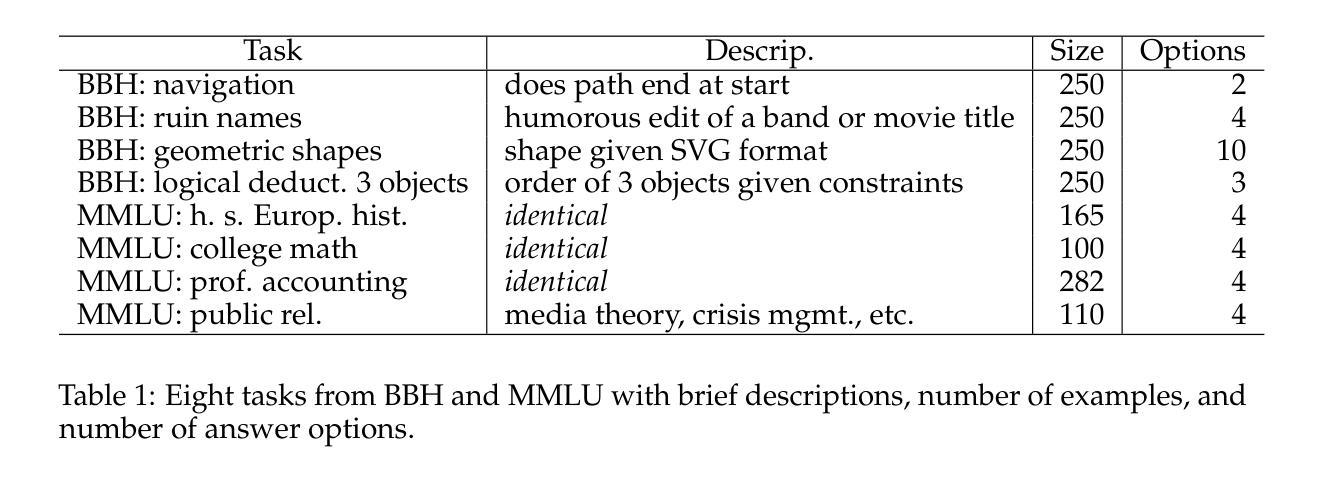
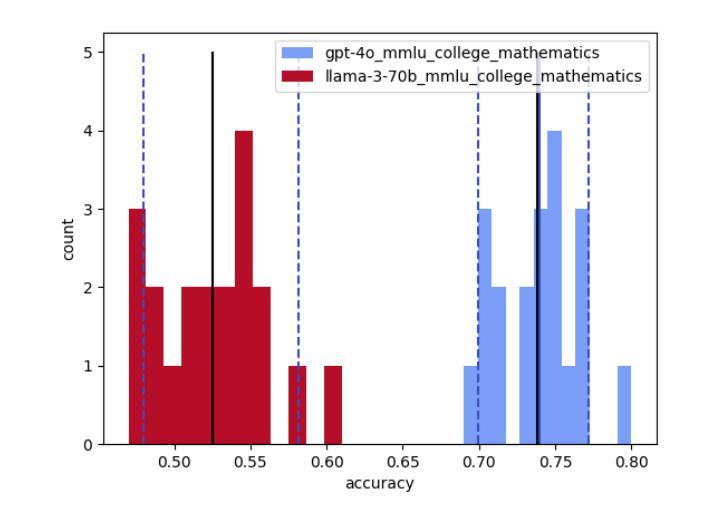
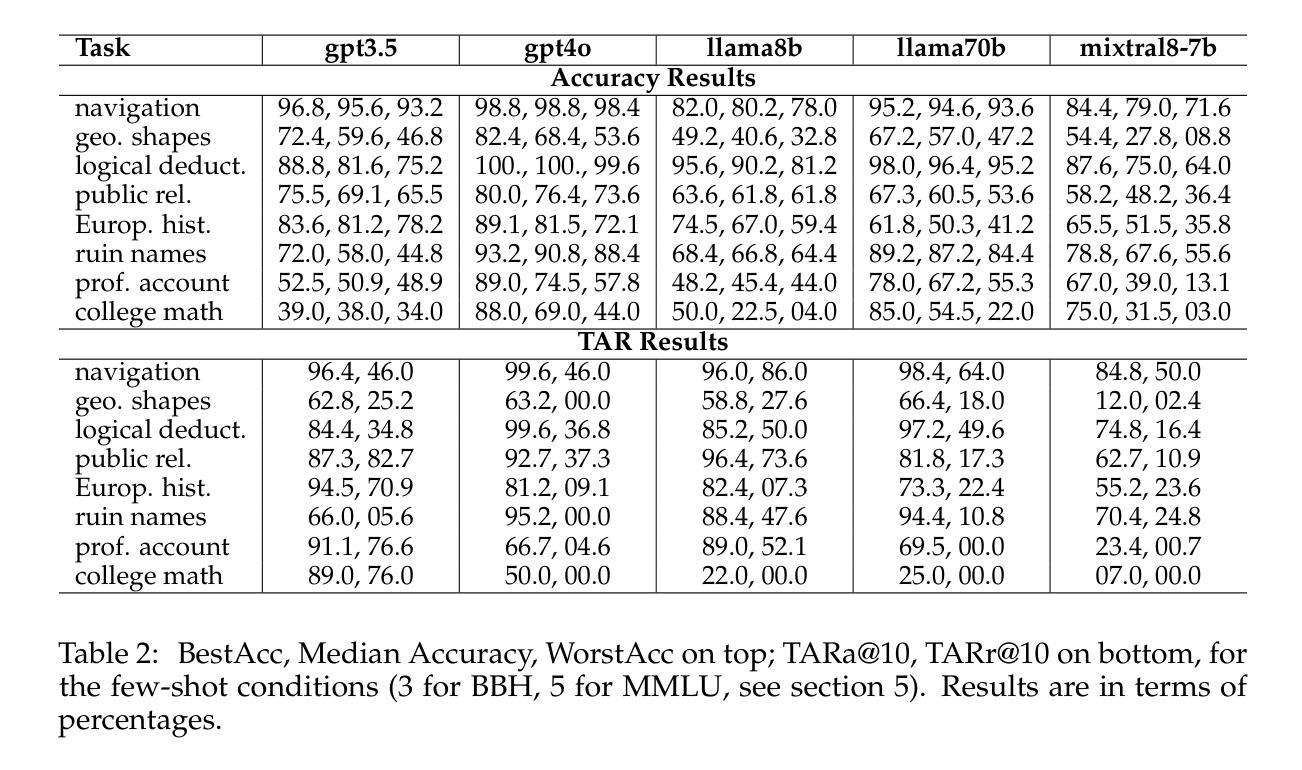
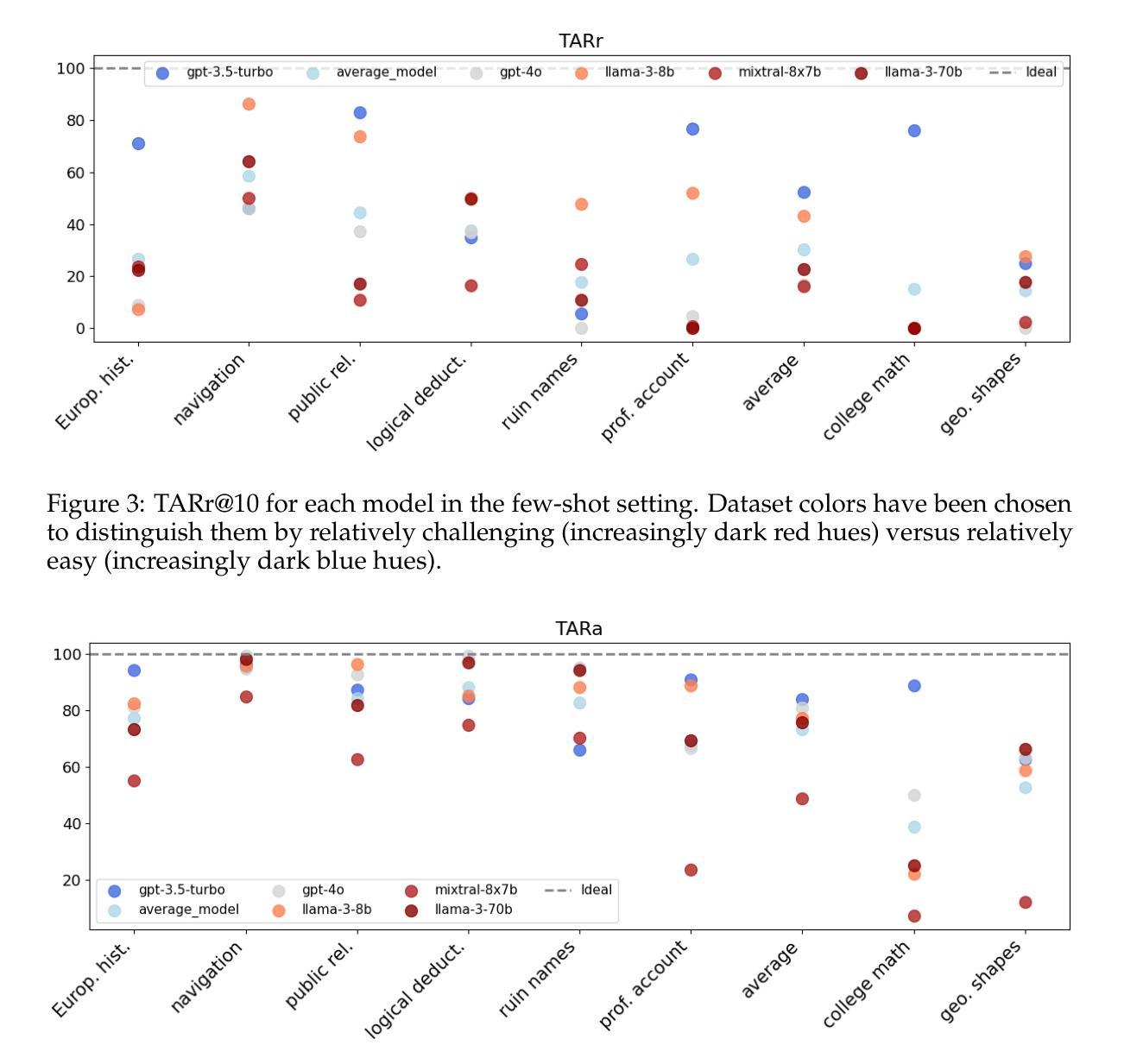
FsPONER: Few-shot Prompt Optimization for Named Entity Recognition in Domain-specific Scenarios
Authors:Yongjian Tang, Rakebul Hasan, Thomas Runkler
Large Language Models (LLMs) have provided a new pathway for Named Entity Recognition (NER) tasks. Compared with fine-tuning, LLM-powered prompting methods avoid the need for training, conserve substantial computational resources, and rely on minimal annotated data. Previous studies have achieved comparable performance to fully supervised BERT-based fine-tuning approaches on general NER benchmarks. However, none of the previous approaches has investigated the efficiency of LLM-based few-shot learning in domain-specific scenarios. To address this gap, we introduce FsPONER, a novel approach for optimizing few-shot prompts, and evaluate its performance on domain-specific NER datasets, with a focus on industrial manufacturing and maintenance, while using multiple LLMs – GPT-4-32K, GPT-3.5-Turbo, LLaMA 2-chat, and Vicuna. FsPONER consists of three few-shot selection methods based on random sampling, TF-IDF vectors, and a combination of both. We compare these methods with a general-purpose GPT-NER method as the number of few-shot examples increases and evaluate their optimal NER performance against fine-tuned BERT and LLaMA 2-chat. In the considered real-world scenarios with data scarcity, FsPONER with TF-IDF surpasses fine-tuned models by approximately 10% in F1 score.
大型语言模型(LLM)为命名实体识别(NER)任务提供了新的途径。与微调相比,LLM驱动的提示方法无需训练,可以节省大量计算资源,并依赖极少量的注释数据。之前的研究已在通用NER基准测试上实现了与完全监督的BERT微调方法相当的性能。然而,以前的任何方法都没有研究LLM在特定领域的少样本学习中的效率。为了弥补这一空白,我们引入了FsPONER,这是一种优化少样本提示的新方法,并评估其在特定领域的NER数据集上的性能,重点关注工业制造和维护领域,同时使用多个LLM——GPT-4-32K、GPT-3.5 Turbo、LLaMA 2聊天和Vicuna。FsPONER包括三种基于随机采样、TF-IDF向量和两者组合的少样本选择方法。我们随着少样本示例数量的增加,将这些方法与通用GPT-NER方法进行比较,并评估其最佳NER性能与微调BERT和LLaMA 2聊天的性能。在数据稀缺的现实世界场景中,使用TF-IDF的FsPONER在F1分数上超越了微调模型约10%。
论文及项目相关链接
PDF accepted in the main track at the 27th European Conference on Artificial Intelligence (ECAI-2024)
Summary
LLMs在命名实体识别(NER)任务中展现出巨大潜力,采用提示方法而无需训练,节省了计算资源,并依赖少量标注数据。本文介绍了一种新型的基于LLM的少样本学习方法FsPONER,并重点对工业制造和维护领域的特定数据集进行评估。FsPONER包含三种基于随机采样、TF-IDF向量和两者结合的少样本选择方法,在某些场景下表现优异,尤其是在数据稀缺的现实中超越微调BERT模型约10%的F1分数。
Key Takeaways
- LLMs为NER任务提供了新的途径,采用提示方法无需训练,节省计算资源。
- FsPONER是一种新型的基于LLM的少样本学习方法,用于优化NER任务的性能。
- FsPONER在特定领域数据集(如工业制造和维护)上表现出优异性能。
- FsPONER包含三种少样本选择方法:随机采样、TF-IDF向量和两者的组合。
- 在某些场景下,FsPONER超越微调BERT模型约10%的F1分数。
- 使用多个LLMs(如GPT-4-32K、GPT-3.5-Turbo、LLaMA 2-chat和Vicuna)进行评估,显示LLMs在NER任务中的潜力。
点此查看论文截图
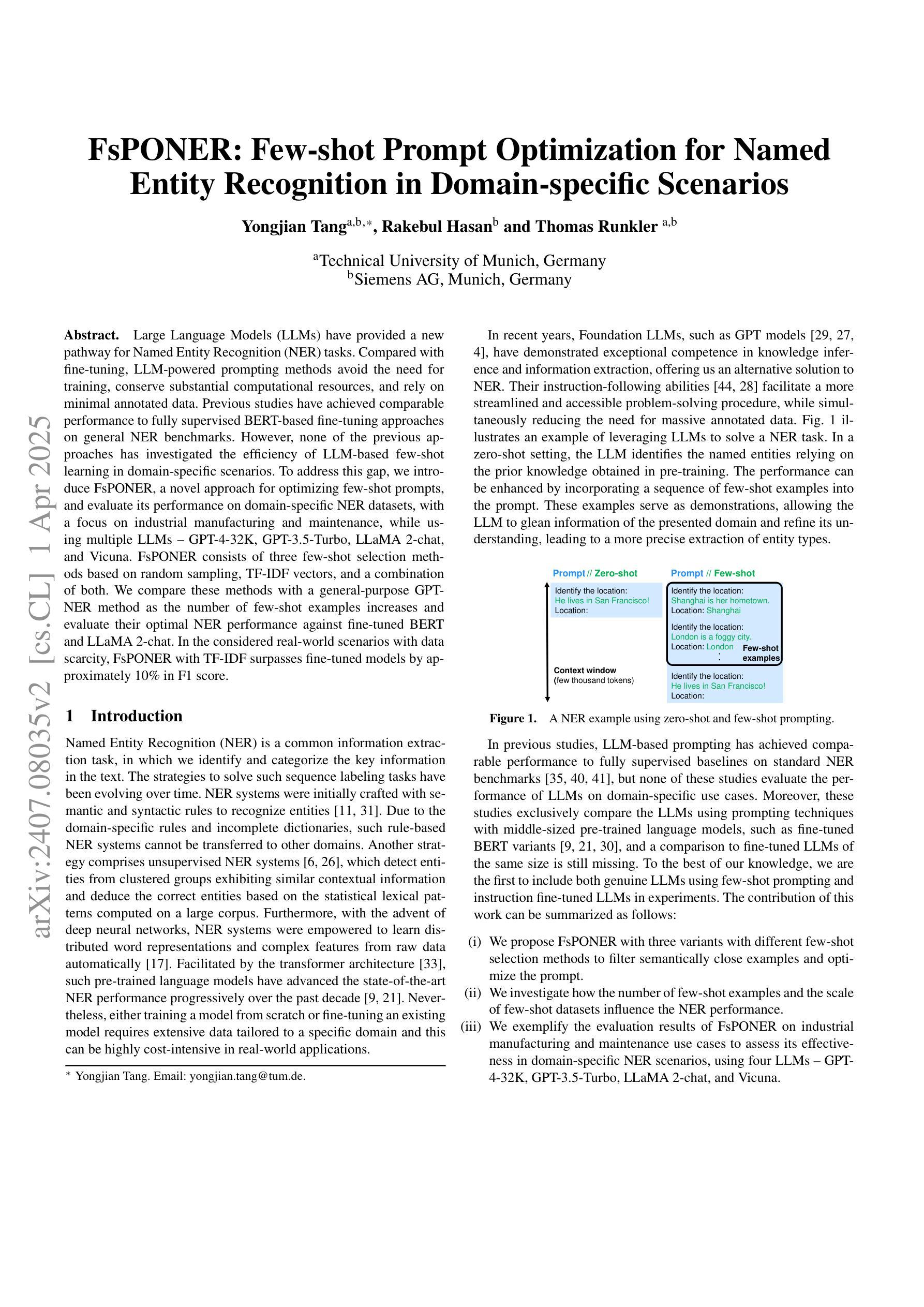
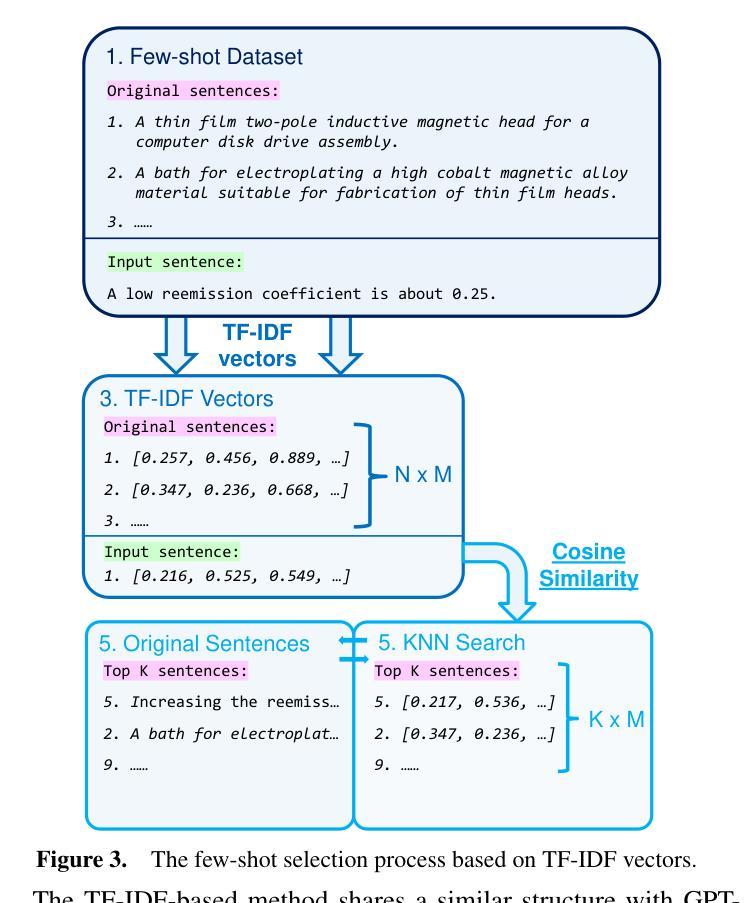
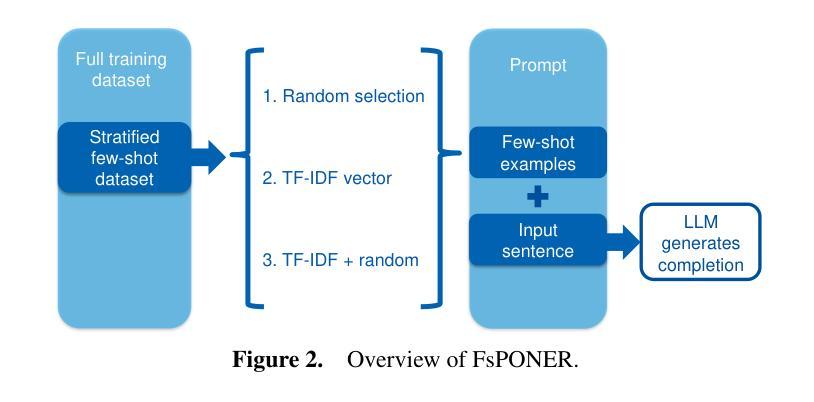
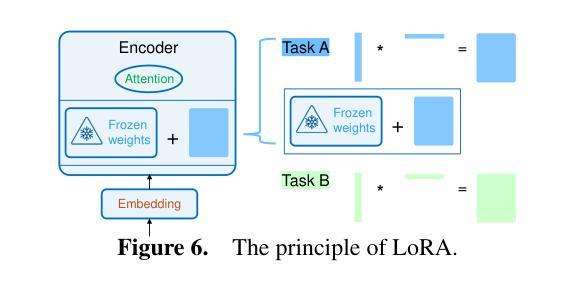
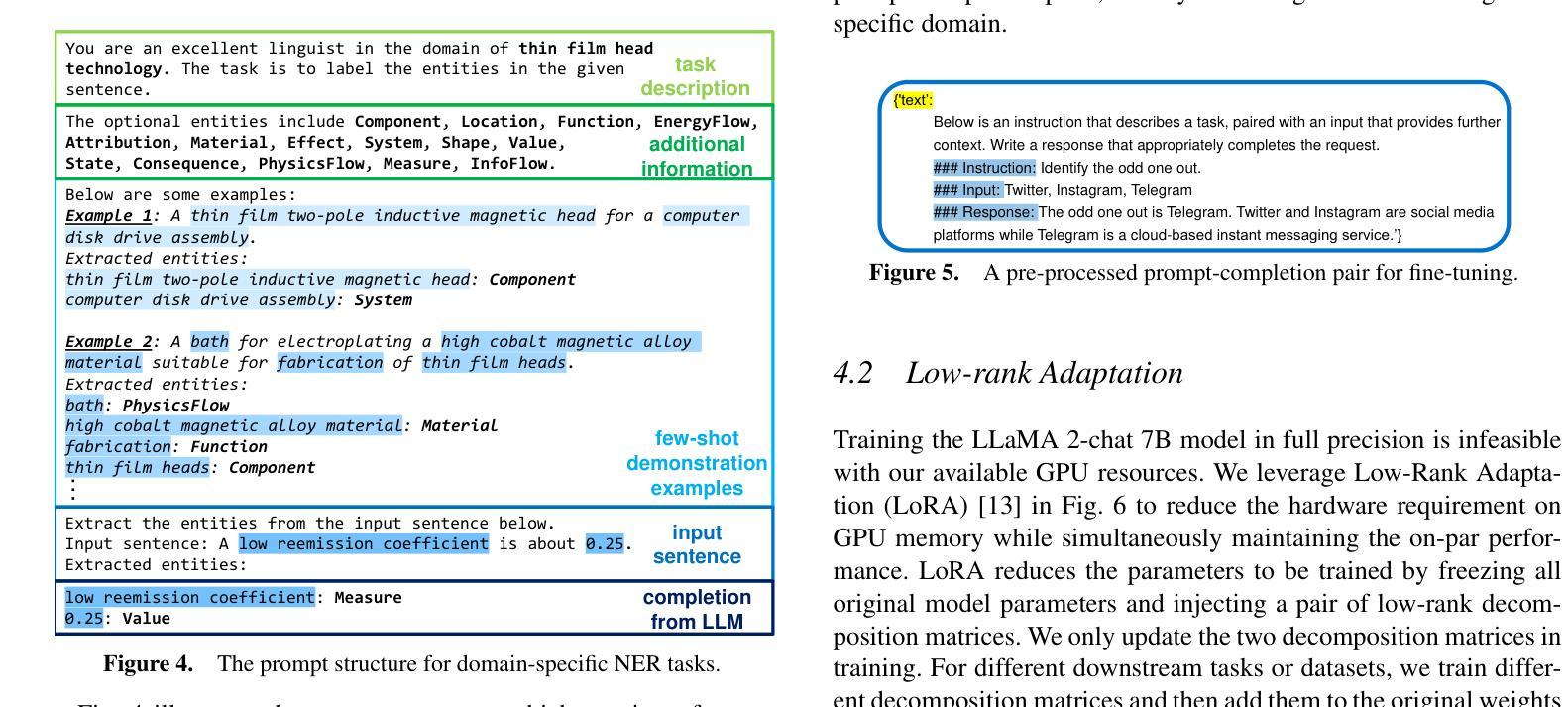
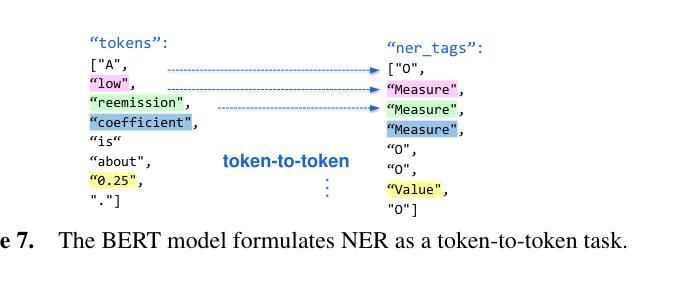
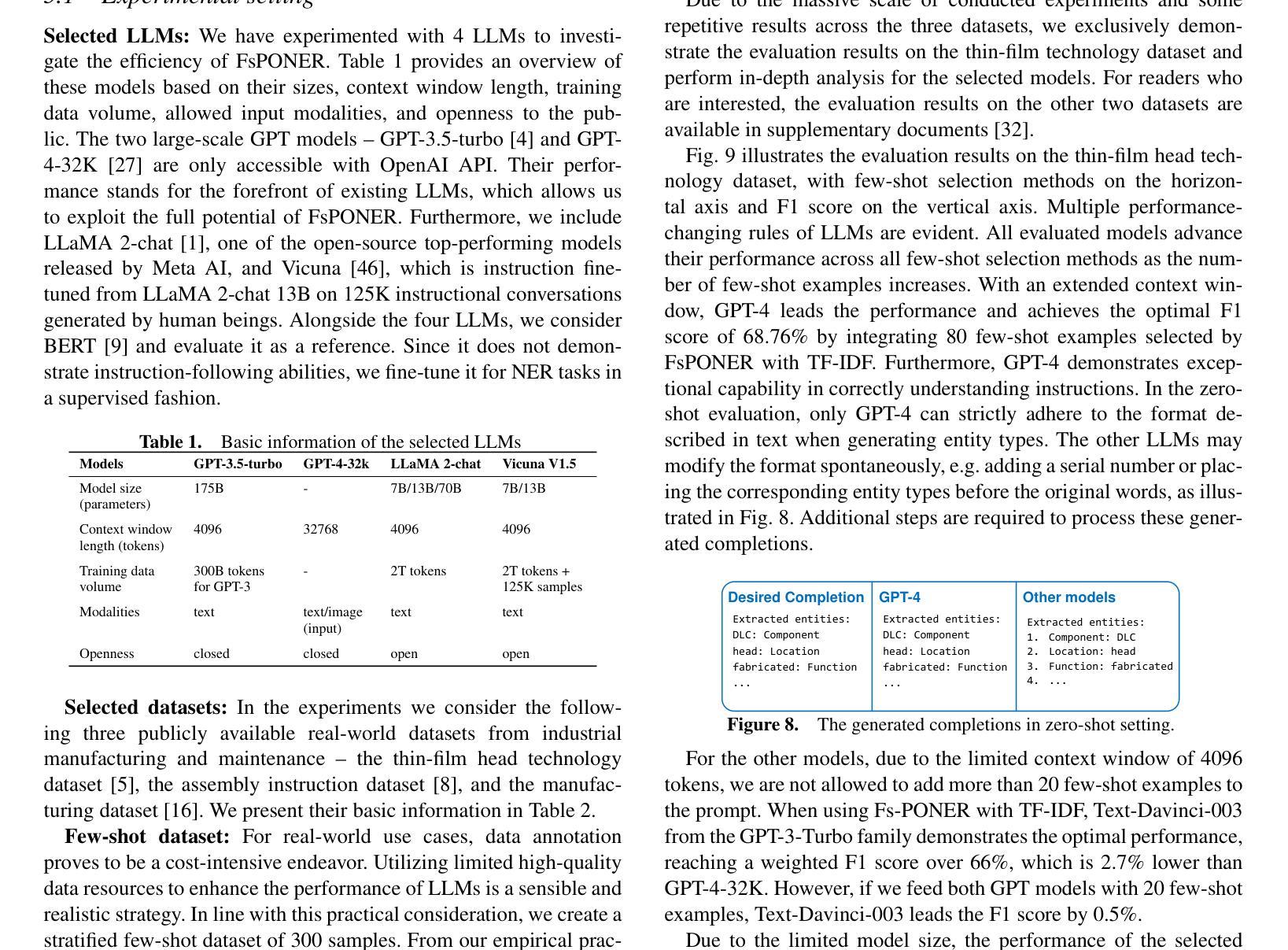
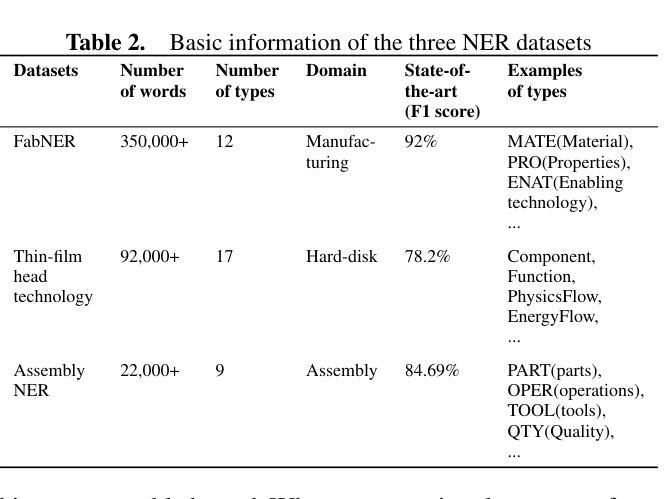
Induction Heads as an Essential Mechanism for Pattern Matching in In-context Learning
Authors:Joy Crosbie, Ekaterina Shutova
Large language models (LLMs) have shown a remarkable ability to learn and perform complex tasks through in-context learning (ICL). However, a comprehensive understanding of its internal mechanisms is still lacking. This paper explores the role of induction heads in a few-shot ICL setting. We analyse two state-of-the-art models, Llama-3-8B and InternLM2-20B on abstract pattern recognition and NLP tasks. Our results show that even a minimal ablation of induction heads leads to ICL performance decreases of up to ~32% for abstract pattern recognition tasks, bringing the performance close to random. For NLP tasks, this ablation substantially decreases the model’s ability to benefit from examples, bringing few-shot ICL performance close to that of zero-shot prompts. We further use attention knockout to disable specific induction patterns, and present fine-grained evidence for the role that the induction mechanism plays in ICL.
大型语言模型(LLM)显示出通过上下文学习(ICL)学习和执行复杂任务的能力。然而,对其内部机制的全面理解仍然缺乏。本文探讨了归纳头在少量上下文学习设置中的角色。我们分析了两种最先进的模型,即Llama-3-8B和InternLM2-20B在抽象模式识别和NLP任务上的表现。我们的结果表明,即使是最小的归纳头消融也会导致抽象模式识别任务的上下文学习性能下降高达约32%,将性能降低到接近随机水平。对于NLP任务,这种消融大大降低了模型从例子中受益的能力,使得少量的上下文学习性能接近于零样本提示。我们进一步使用注意力消除法来禁用特定的归纳模式,并为归纳机制在上下文学习中的作用提供精细的证据。
论文及项目相关链接
PDF 9 pages, 7 figures; Code link added
Summary
大型语言模型(LLMs)通过上下文学习(ICL)展现出了令人瞩目的学习和执行复杂任务的能力,但其内部机制仍缺乏全面理解。本文在少样本ICL环境中,探讨了归纳头的作用。我们对两种最新模型Llama-3-8B和InternLM2-20B在抽象模式识别和NLP任务上进行了分析。结果表明,即使进行少量的归纳头消融也会导致ICL性能下降约至原来的最大达约达32%,严重影响抽象模式识别任务的性能。对于NLP任务,消融使模型几乎丧失从示例中受益的能力,使少样本ICL性能接近零样本提示性能。我们进一步使用注意力剔除技术来禁用特定的归纳模式,并为归纳机制在ICL中的角色提供了精细的证据。
Key Takeaways
- 大型语言模型(LLMs)通过上下文学习(ICL)表现出强大的学习和执行任务的能力。
- 归纳头在LLMs的ICL中起到关键作用。
- 对归纳头进行消融会导致LLMs在抽象模式识别任务上的性能下降高达约32%。
- 对于NLP任务,消融归纳头会使模型丧失从示例中学习的能力,使少样本ICL性能接近零样本提示性能。
- 通过注意力剔除技术,可以禁用特定的归纳模式。
- 论文为归纳机制在ICL中的角色提供了精细的证据。
点此查看论文截图
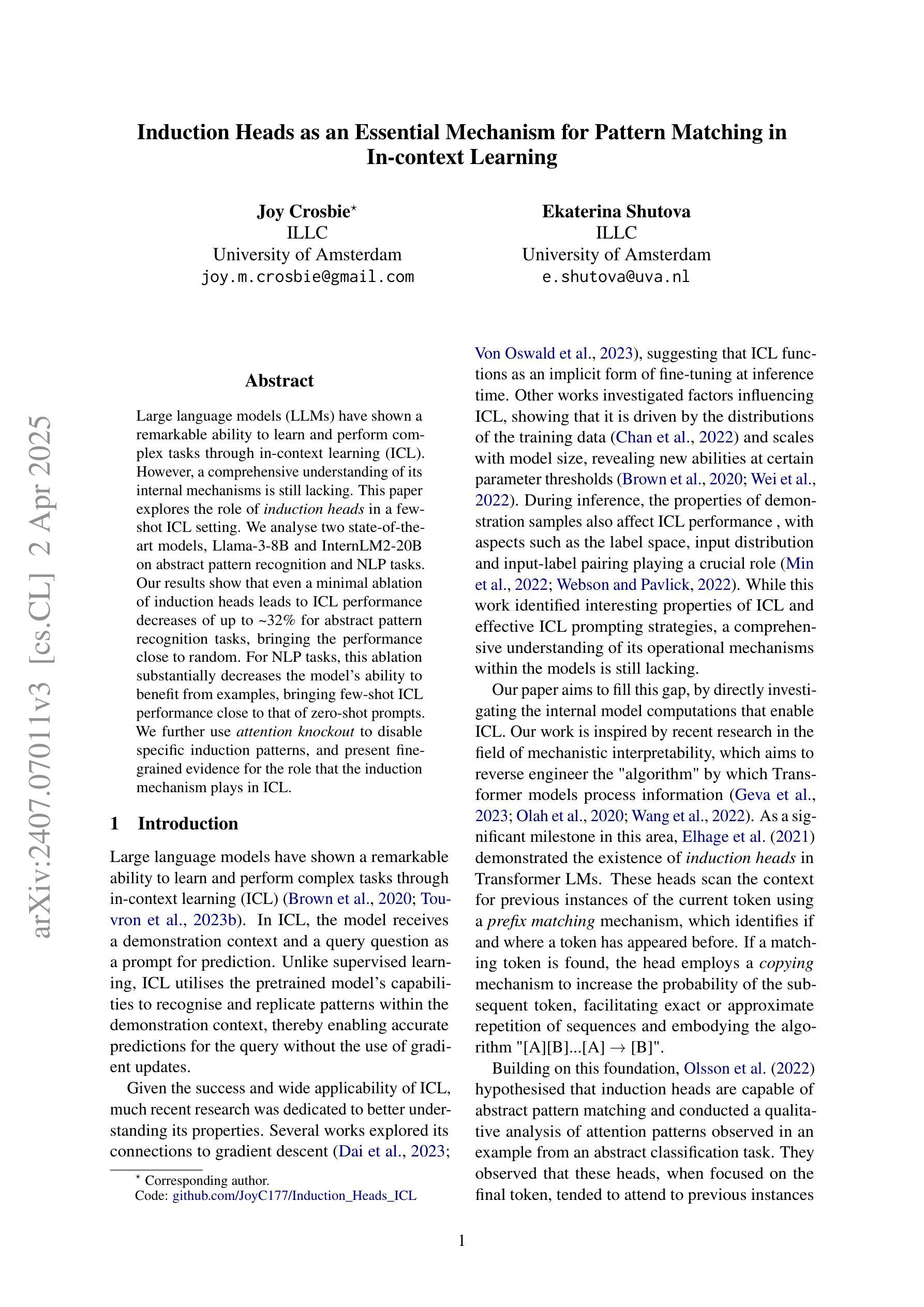
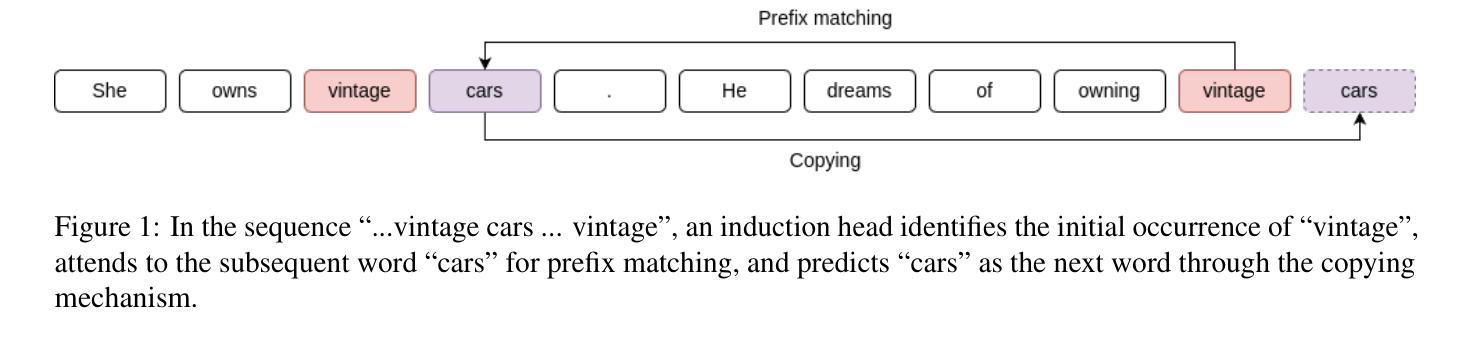
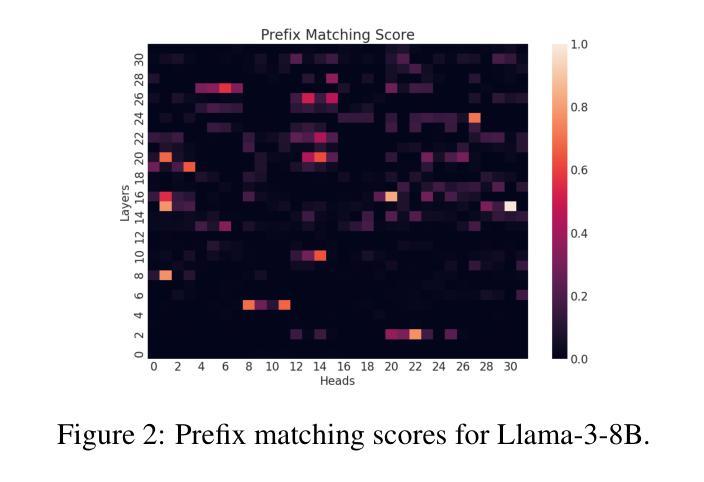
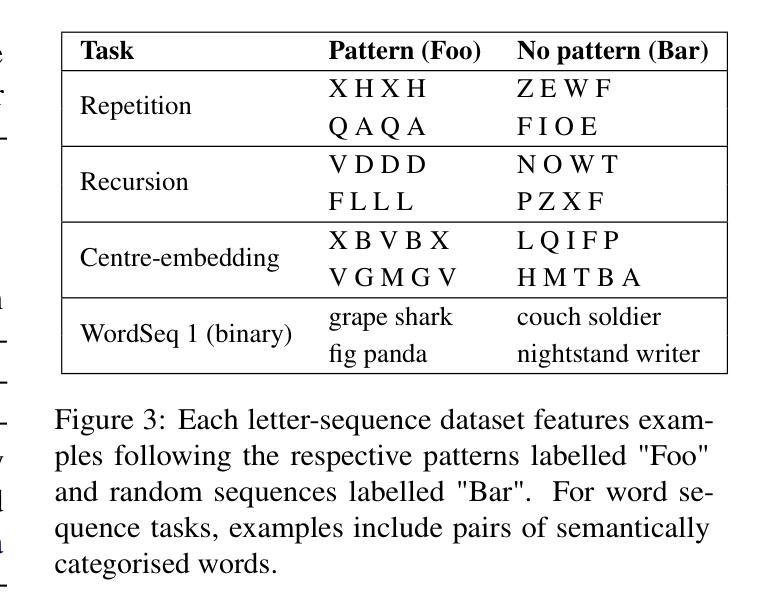
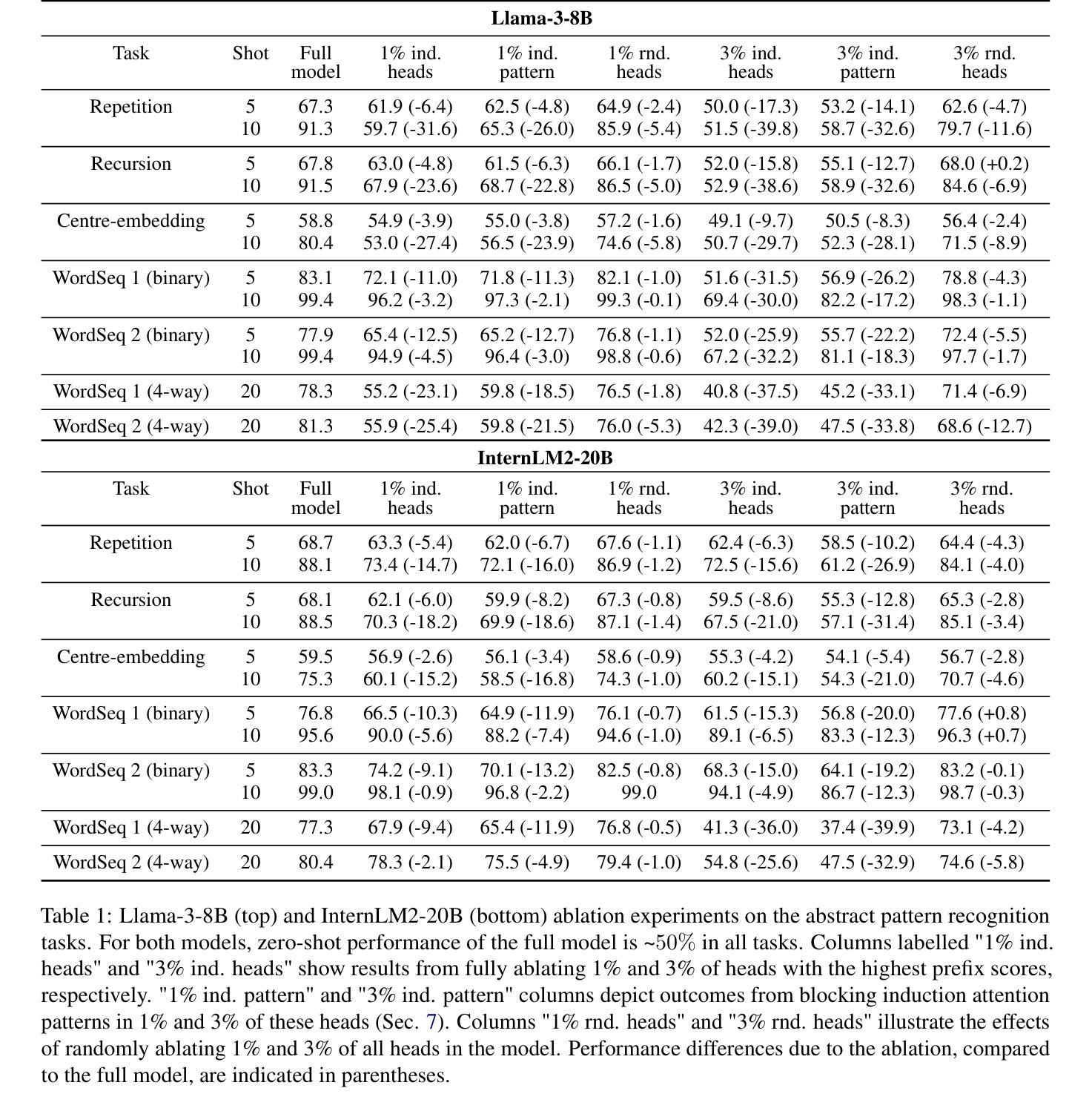
CoMM: A Coherent Interleaved Image-Text Dataset for Multimodal Understanding and Generation
Authors:Wei Chen, Lin Li, Yongqi Yang, Bin Wen, Fan Yang, Tingting Gao, Yu Wu, Long Chen
Interleaved image-text generation has emerged as a crucial multimodal task, aiming at creating sequences of interleaved visual and textual content given a query. Despite notable advancements in recent multimodal large language models (MLLMs), generating integrated image-text sequences that exhibit narrative coherence and entity and style consistency remains challenging due to poor training data quality. To address this gap, we introduce CoMM, a high-quality Coherent interleaved image-text MultiModal dataset designed to enhance the coherence, consistency, and alignment of generated multimodal content. Initially, CoMM harnesses raw data from diverse sources, focusing on instructional content and visual storytelling, establishing a foundation for coherent and consistent content. To further refine the data quality, we devise a multi-perspective filter strategy that leverages advanced pre-trained models to ensure the development of sentences, consistency of inserted images, and semantic alignment between them. Various quality evaluation metrics are designed to prove the high quality of the filtered dataset. Meanwhile, extensive few-shot experiments on various downstream tasks demonstrate CoMM’s effectiveness in significantly enhancing the in-context learning capabilities of MLLMs. Moreover, we propose four new tasks to evaluate MLLMs’ interleaved generation abilities, supported by a comprehensive evaluation framework. We believe CoMM opens a new avenue for advanced MLLMs with superior multimodal in-context learning and understanding ability.
交互式图像文本生成已经成为一项关键的多模态任务,其目标是根据查询生成交替出现的视觉和文本内容序列。尽管最近的多模态大型语言模型(MLLM)取得了显著的进展,但生成具有叙事连贯性和实体及风格一致性的集成图像文本序列仍然是一个挑战,主要是由于训练数据质量差。为了解决这一差距,我们引入了CoMM,这是一个高质量的多模态连贯交互式图像文本数据集,旨在提高生成的多模态内容的一致性、连贯性和对齐性。
论文及项目相关链接
PDF 22 pages, Accepted by CVPR 2025
Summary
本文介绍了一个名为CoMM的高质量图像文本多媒体数据集,该数据集用于提高生成多媒体内容的连贯性、一致性和对齐性。通过从各种资源中获取原始数据,并专注于指令内容和视觉叙事,建立连贯和一致内容的基础。采用多视角过滤策略,利用先进的预训练模型确保句子发展、插入图像的一致性和语义对齐。经过质量评估证明,过滤后的数据集质量高。此外,在各种下游任务上的少量样本实验证明了CoMM在提高多媒体大型语言模型的上下文学习能力方面的有效性。同时,提出了四个新任务来评估多媒体大型语言模型的交错生成能力,并由综合评估框架支持。
Key Takeaways
- CoMM是一个高质量的图像文本多媒体数据集,旨在提高生成多媒体内容的连贯性、一致性和对齐性。
- 该数据集从各种资源获取原始数据,专注于指令内容和视觉叙事。
- 采用多视角过滤策略,利用先进的预训练模型进行质量提升。
- 过滤后的数据集经过质量评估证明表现优异。
- 在下游任务上的少量样本实验证明了CoMM的有效性。
- 提出了四个新任务来评估多媒体大型语言模型的交错生成能力。
点此查看论文截图
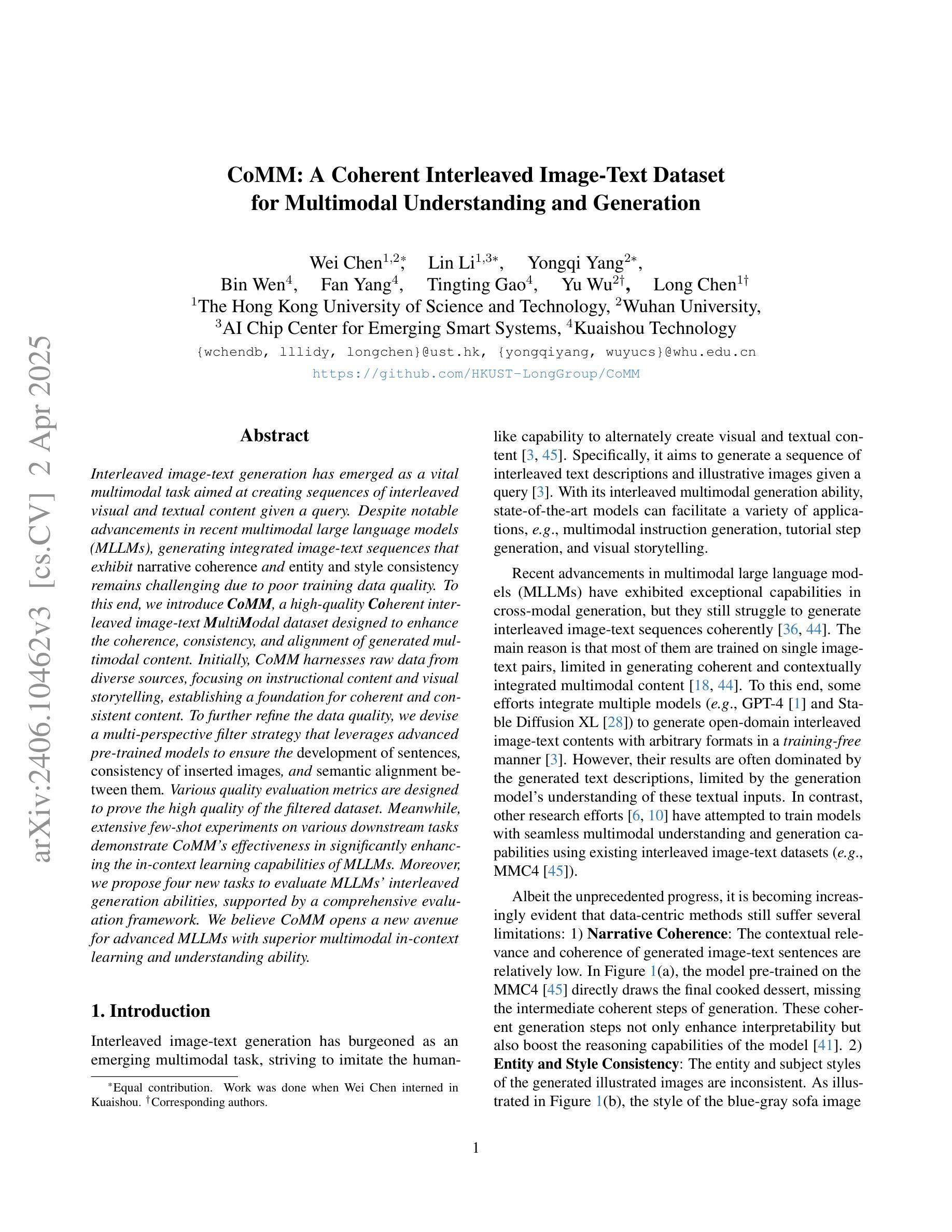
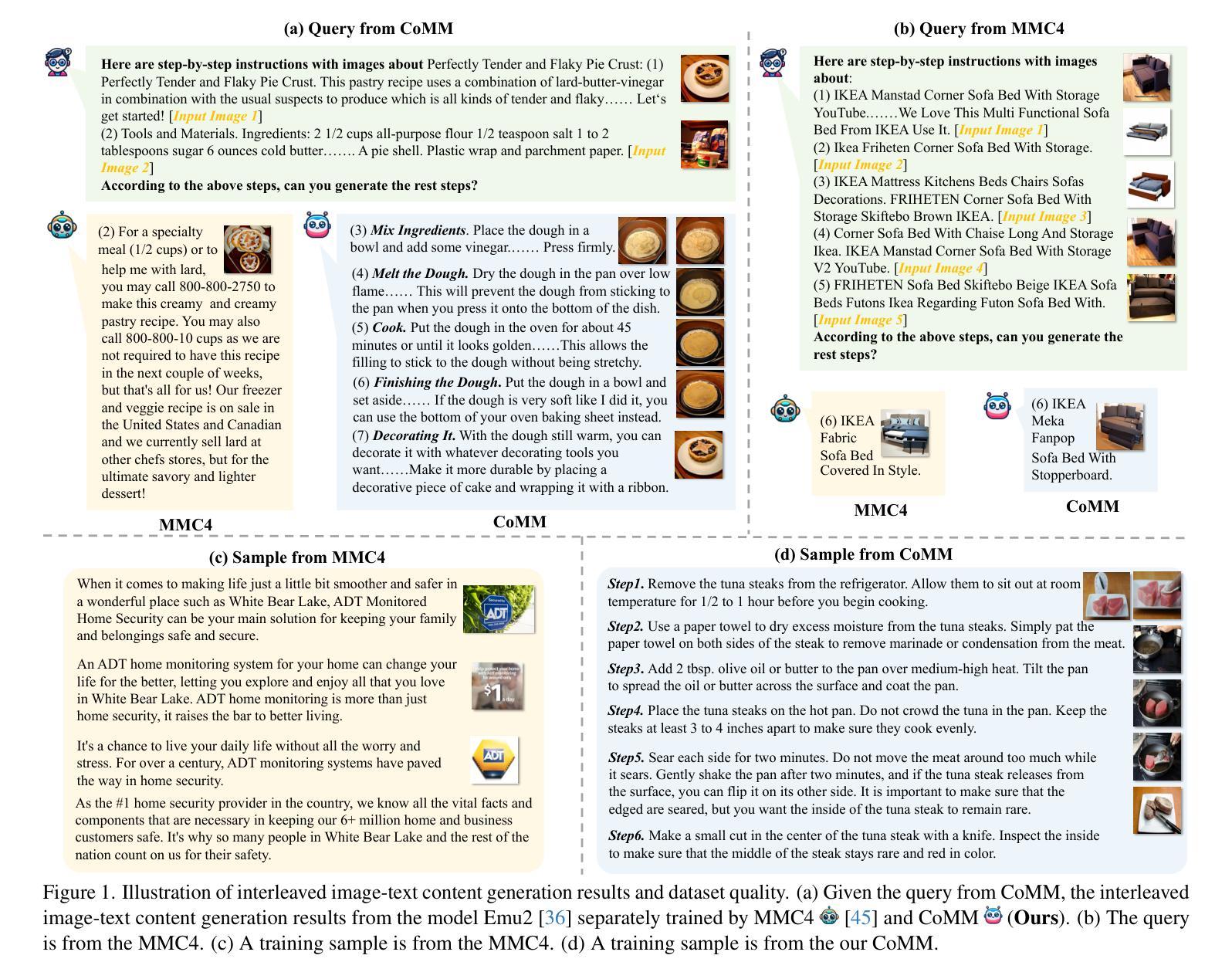
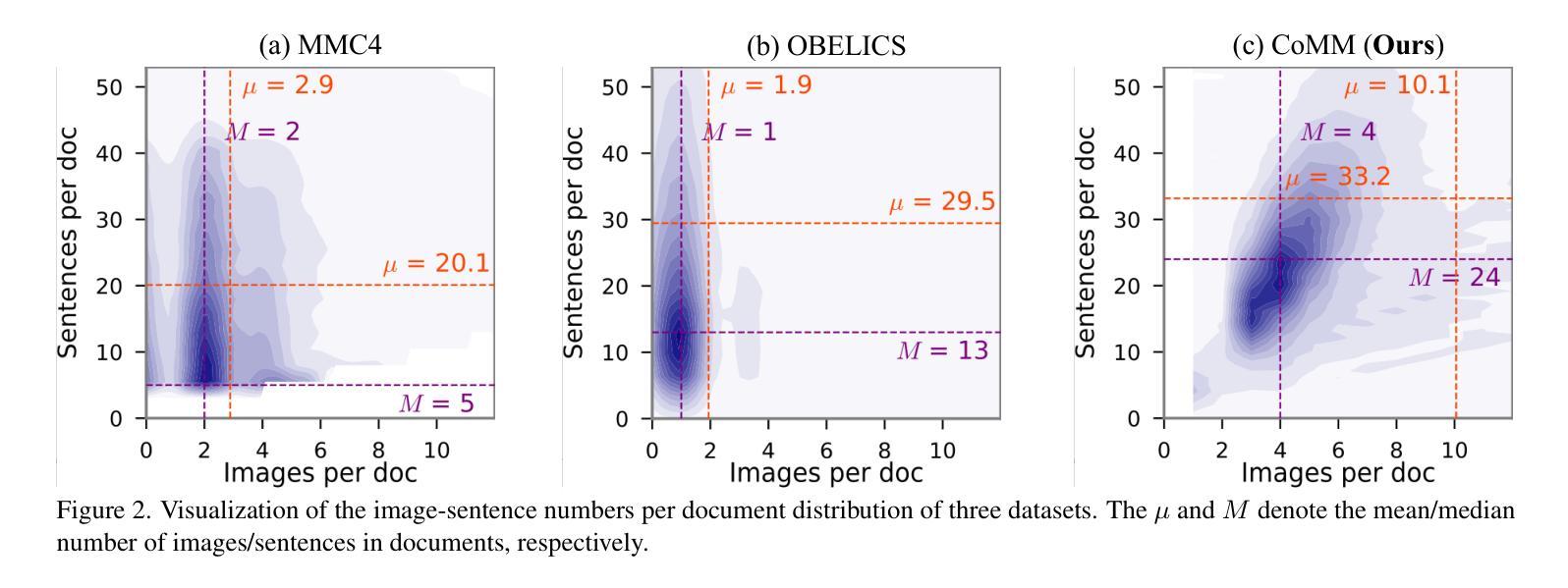
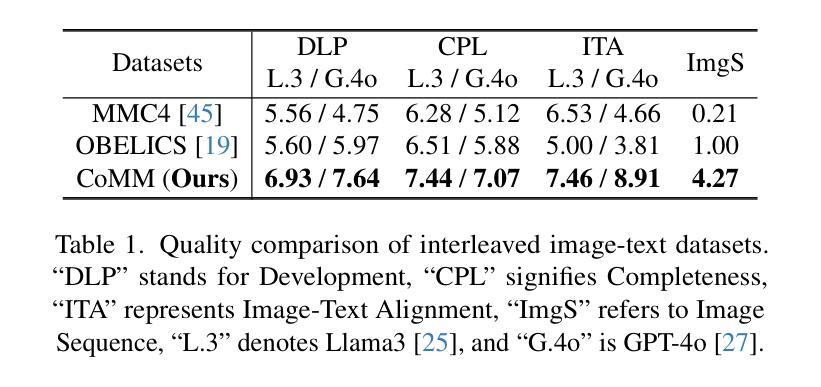
Consistency-Guided Asynchronous Contrastive Tuning for Few-Shot Class-Incremental Tuning of Foundation Models
Authors:Shuvendu Roy, Elham Dolatabadi, Arash Afkanpour, Ali Etemad
We propose Consistency-guided Asynchronous Contrastive Tuning (CoACT), a novel method for continuously tuning foundation models to learn new classes in few-shot settings. CoACT consists of three key components:(i) asynchronous contrastive tuning, which learns new classes by including LoRA modules in the pre-trained encoder while enforcing consistency between two asynchronous encoders; (ii) controlled fine-tuning, which facilitates effective tuning of a subset of the foundation model; and (iii) consistency-guided incremental tuning, which enforces additional regularization during later sessions to reduce forgetting of the learned classes. We evaluate our proposed solution on Few-Shot Class-Incremental Learning (FSCIL) as well as a new and more challenging setup called Few-Shot Class-Incremental Tuning (FSCIT), which facilitates the continual tuning of vision foundation models to learn new classes with only a few samples per class. Unlike traditional FSCIL, FSCIT does not require a large in-distribution base session for initial fully supervised training prior to the incremental few-shot sessions. We conduct extensive evaluations across 16 diverse datasets, demonstrating the effectiveness of CoACT in both FSCIL and FSCIT setups. CoACT outperforms existing methods by up to 5.02% in FSCIL and up to 12.51% in FSCIT for individual datasets, with an average improvement of 2.47%. Furthermore, CoACT exhibits reduced forgetting and enhanced robustness in low-shot experiments. Detailed ablation and sensitivity studies highlight the contribution of each component of CoACT. We make our code publicly available at https://github.com/ShuvenduRoy/CoACT-FSCIL.
我们提出了一种新的方法,名为一致性引导异步对比调优(CoACT),用于在少量样本设置下连续调整基础模型以学习新类别。CoACT包含三个关键组件:(i) 异步对比调优,通过在预训练编码器中包含LoRA模块,同时强制两个异步编码器之间的一致性,来学习新类别;(ii) 受控微调,便于有效地调整基础模型的一个子集;(iii) 一致性引导增量调优,在后续会话期间强制执行额外的正则化,以减少已学习类别的遗忘。我们在少量类别增量学习(FSCIL)以及称为少量类别增量调整(FSCIT)的新颖且更具挑战性的设置上评估了我们提出的解决方案。FSCIT促进了视觉基础模型在只有每个类别几个样本的情况下不断学习新类别。与传统的FSCIL不同,FSCIT不需要在增量少量会话之前进行大规模内部基础会话的初始完全监督训练。我们在16个不同的数据集上进行了广泛评估,证明了CoACT在FSCIL和FSCIT设置中的有效性。在FSCIL中,CoACT将现有方法的性能提高了高达5.02%,在FSCIT中提高了高达12.51%,针对单个数据集的平均改进为2.47%。此外,CoACT在低样本实验中表现出减少遗忘和增强稳健性的特点。详细的消融和敏感性研究突出了CoACT每个组件的贡献。我们在https://github.com/ShuvenduRoy/CoACT-FSCIL 公开了我们的代码。
论文及项目相关链接
PDF Accepted in Transactions on Machine Learning Research (TMLR)
摘要
本研究提出一种名为Consistency-guided Asynchronous Contrastive Tuning(CoACT)的新方法,用于在少量样本情况下连续调整基础模型以学习新类别。CoACT包含三个关键组件:(i)异步对比调整,通过包含LoRA模块在预训练编码器中学习新类别,同时强制两个异步编码器之间的一致性;(ii)受控微调,促进基础模型子集的有效调整;(iii)一致性引导增量调整,在后续会话期间强制执行附加正则化,以减少已学习类别的遗忘。本研究在少量类别增量学习(FSCIL)以及称为少量类别增量调整(FSCIT)的新挑战设置上评估了所提方法的有效性,后者促进了视觉基础模型连续调整以学习新类别,并且每类仅使用少量样本。与传统FSCIL不同,FSCIT不需要大量内部基本会话进行初始完全监督训练,再进行增量少量会话。在16个不同数据集上的广泛评估表明,CoACT在FSCIL和FSCIT设置中均表现出卓越效果。在FSCIL中,CoACT较现有方法最多高出5.02%,在FSCIT中最多高出12.51%。总体而言,CoACT减少了遗忘并在低样本实验中表现出增强的稳健性。详细的消融和敏感性研究突出了CoACT每个组件的贡献。我们的代码已公开发布在https://github.com/ShuvenduRoy/CoACT-FSCIL。
要点掌握
- CoACT是一种用于在少量样本情况下连续调整基础模型学习新类别的新方法。
- CoACT包含三个关键组件:异步对比调整、受控微调、一致性引导增量调整。
- CoACT在FSCIL和FSCIT两种设置上进行了评估,并表现出卓越的效果。
- CoACT在FSCIL和FSCIT中都较现有方法有显著提高,平均提高了2.47%。
- CoACT减少了遗忘并在低样本实验中表现出增强的稳健性。
- 公开的代码地址是https://github.com/ShuvenduRoy/CoACT-FSCIL。
点此查看论文截图
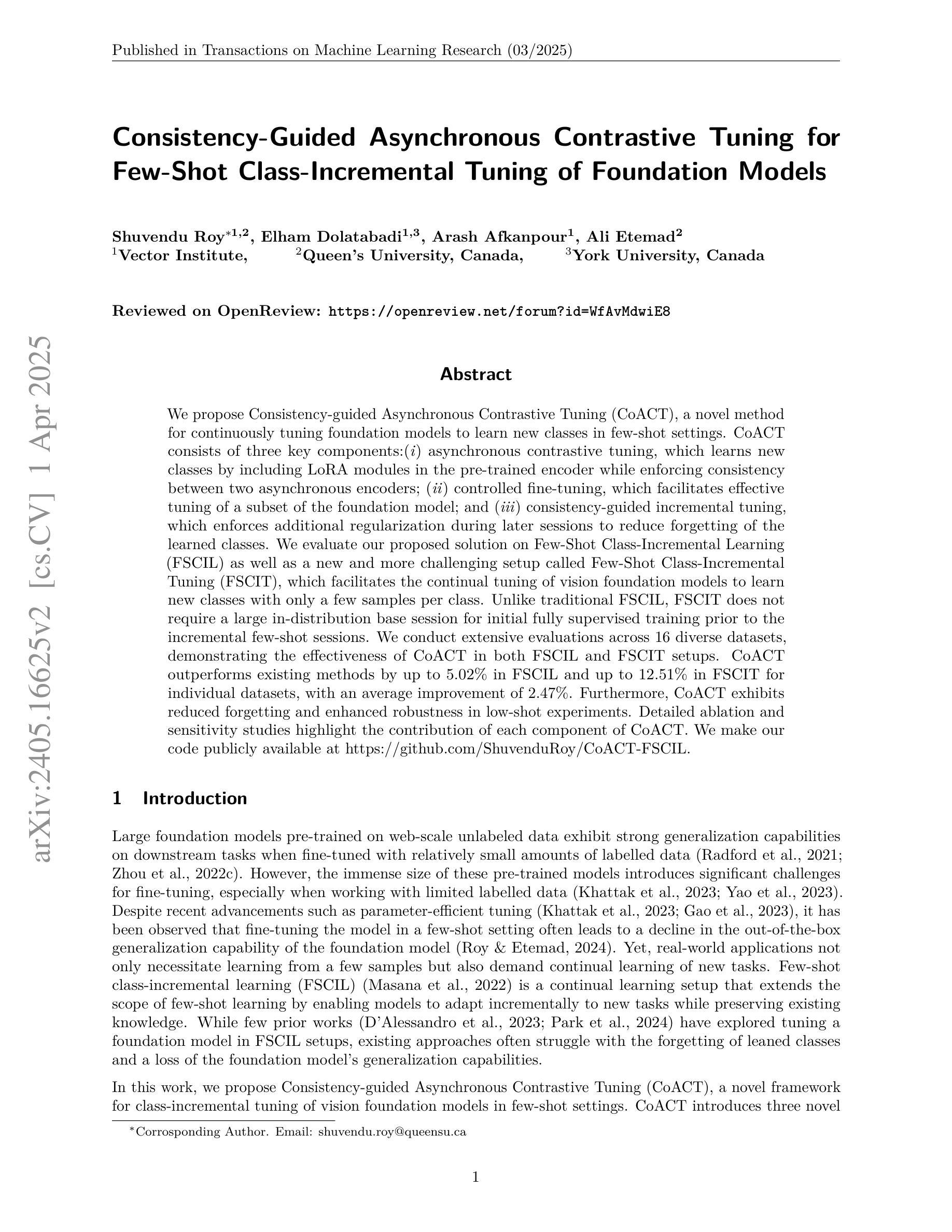
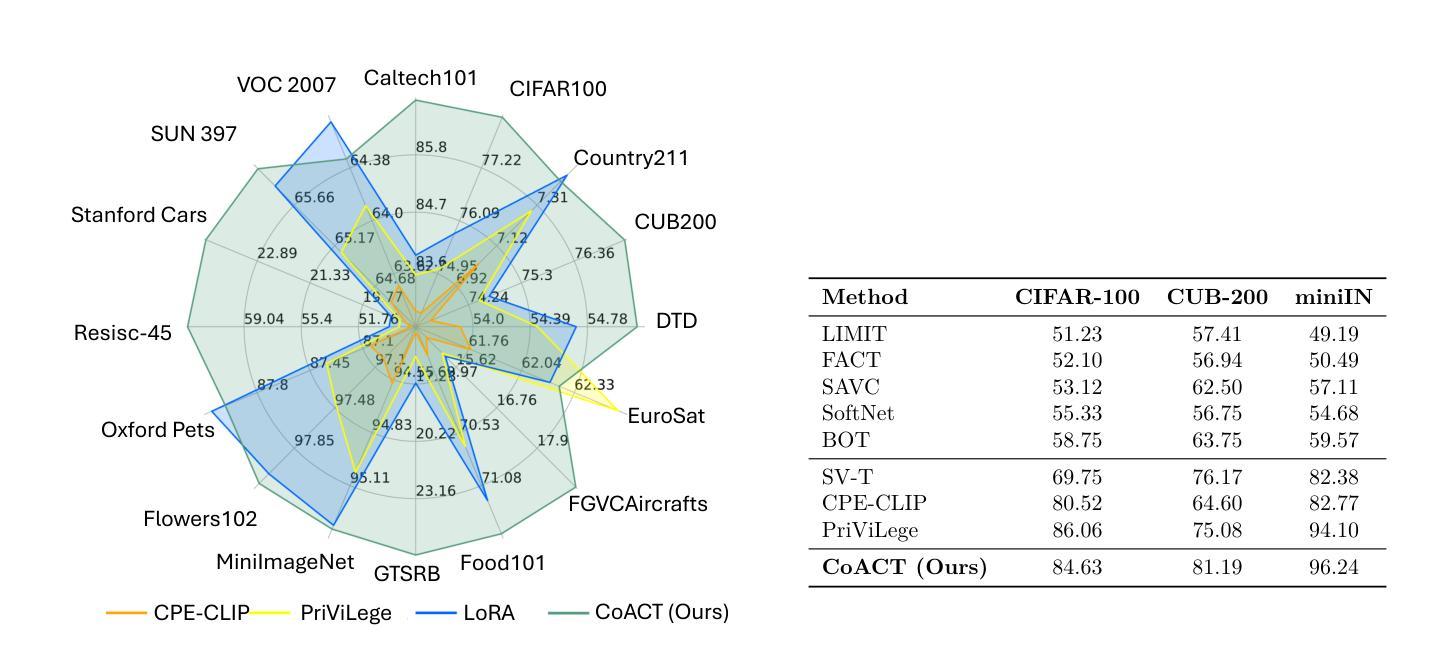
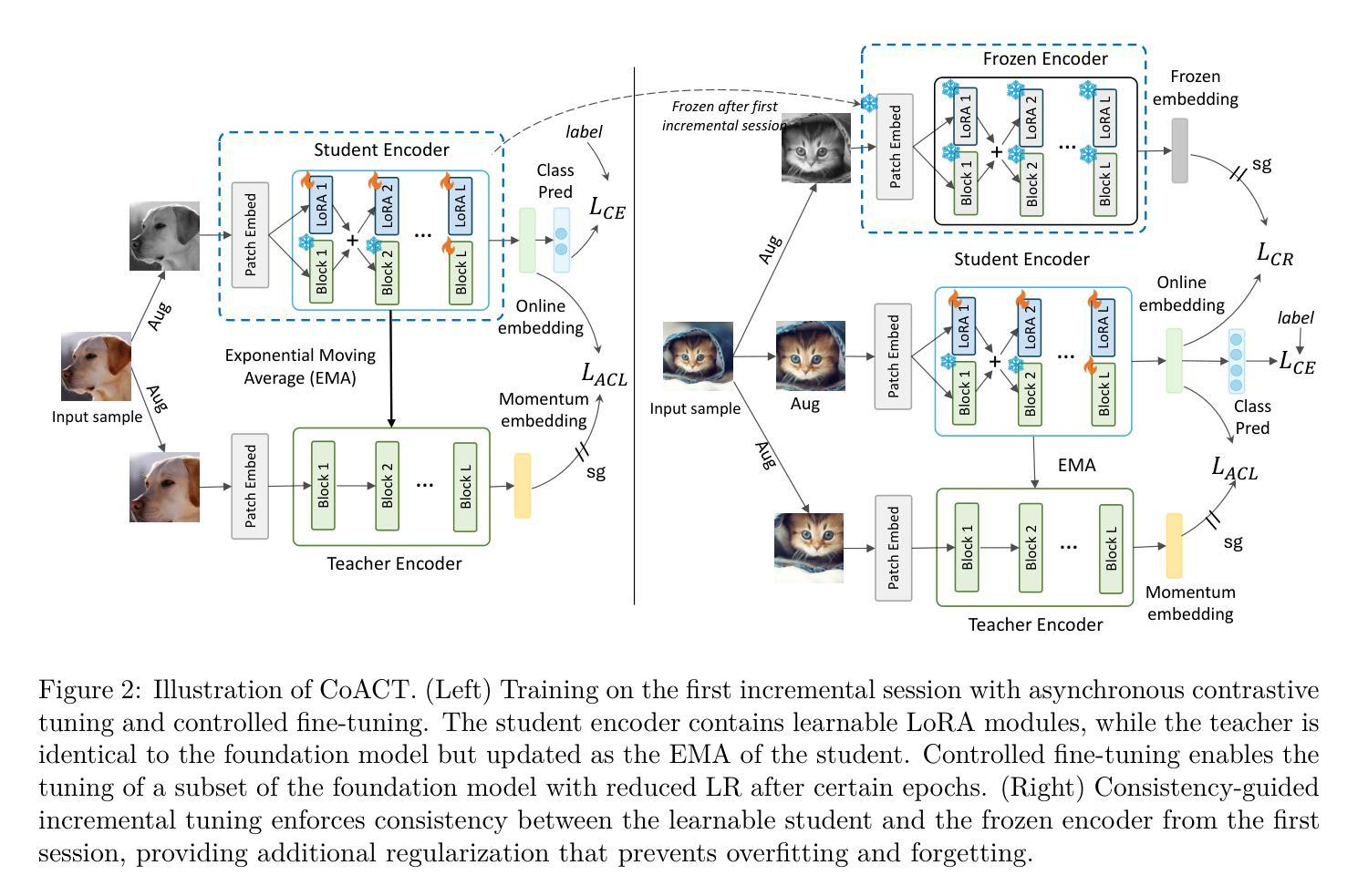
VL-ICL Bench: The Devil in the Details of Multimodal In-Context Learning
Authors:Yongshuo Zong, Ondrej Bohdal, Timothy Hospedales
Large language models (LLMs) famously exhibit emergent in-context learning (ICL) – the ability to rapidly adapt to new tasks using few-shot examples provided as a prompt, without updating the model’s weights. Built on top of LLMs, vision large language models (VLLMs) have advanced significantly in areas such as recognition, reasoning, and grounding. However, investigations into \emph{multimodal ICL} have predominantly focused on few-shot visual question answering (VQA), and image captioning, which we will show neither exploit the strengths of ICL, nor test its limitations. The broader capabilities and limitations of multimodal ICL remain under-explored. In this study, we introduce a comprehensive benchmark VL-ICL Bench for multimodal in-context learning, encompassing a broad spectrum of tasks that involve both images and text as inputs and outputs, and different types of challenges, from {perception to reasoning and long context length}. We evaluate the abilities of state-of-the-art VLLMs against this benchmark suite, revealing their diverse strengths and weaknesses, and showing that even the most advanced models, such as GPT-4, find the tasks challenging. By highlighting a range of new ICL tasks, and the associated strengths and limitations of existing models, we hope that our dataset will inspire future work on enhancing the in-context learning capabilities of VLLMs, as well as inspire new applications that leverage VLLM ICL. The code and dataset are available at https://github.com/ys-zong/VL-ICL.
大型语言模型(LLM)展现出一种称为情境涌现学习(ICL)的能力,即使用少量示例快速适应新任务,而无需更新模型权重。建立在大型语言模型之上的视觉大型语言模型(VLLM)在识别、推理和接地等领域取得了显著进展。然而,对多模态ICL的研究主要集中在视觉问答和图像描述生成等方面,这些领域并没有充分利用ICL的优势,也没有测试其局限性。多模态ICL的更广泛的能力和局限性尚未被充分探索。在这项研究中,我们引入了全面的基准测试VL-ICL Bench,用于多模态情境学习,涵盖了一系列任务,这些任务涉及图像和文本作为输入和输出,以及从感知到推理和长语境长度的不同类型挑战。我们用这个基准测试套件评估了最先进的VLLM的能力,揭示了它们的各种优势和劣势,并表明即使是最先进的模型,如GPT-4,也会发现这些任务具有挑战性。通过突出一系列新的ICL任务以及现有模型的相关优势和局限性,我们希望我们的数据集将激发未来对增强VLLM情境学习能力的研究,并激发利用VLLM ICL的新应用。代码和数据集可在https://github.com/ys-zong/VL-ICL访问。
论文及项目相关链接
PDF ICLR 2025
Summary
大型语言模型展现出新兴的在情境内学习(ICL)能力,能够通过少量示例迅速适应新任务。在此基础上,视觉大型语言模型(VLLMs)在识别、推理和接地等领域取得了显著进展。然而,多模态ICL的探究主要集中在少数视觉问答和图像描述上,这并未充分利用ICL的优势,也没有测试其局限性。本研究引入了一个全面的多模态情境内学习基准VL-ICL Bench,涵盖了一系列涉及图像和文本作为输入和输出的任务,以及从感知到推理和长语境长度的不同类型挑战。我们评估了最先进VLLMs的能力,揭示了它们的各种优势和劣势,并显示即使是最先进的模型,如GPT-4,也会发现这些任务具有挑战性。
Key Takeaways
- 大型语言模型展现出情境内学习能力,能通过少量示例迅速适应新任务。
- 视觉大型语言模型在识别、推理和接地等领域取得显著进展。
- 当前多模态ICL研究主要集中在视觉问答和图像描述上,未能充分利用ICL的优势和测试其局限性。
- 引入了一个全面的多模态情境内学习基准VL-ICL Bench,涵盖广泛的任务类型,包括图像和文本作为输入和输出,涉及不同类型的挑战。
- 评估了最先进VLLMs的能力,揭示了其优势和劣势。
- 现有最先进的模型,如GPT-4,在面对这些新任务时仍会面临挑战。
点此查看论文截图
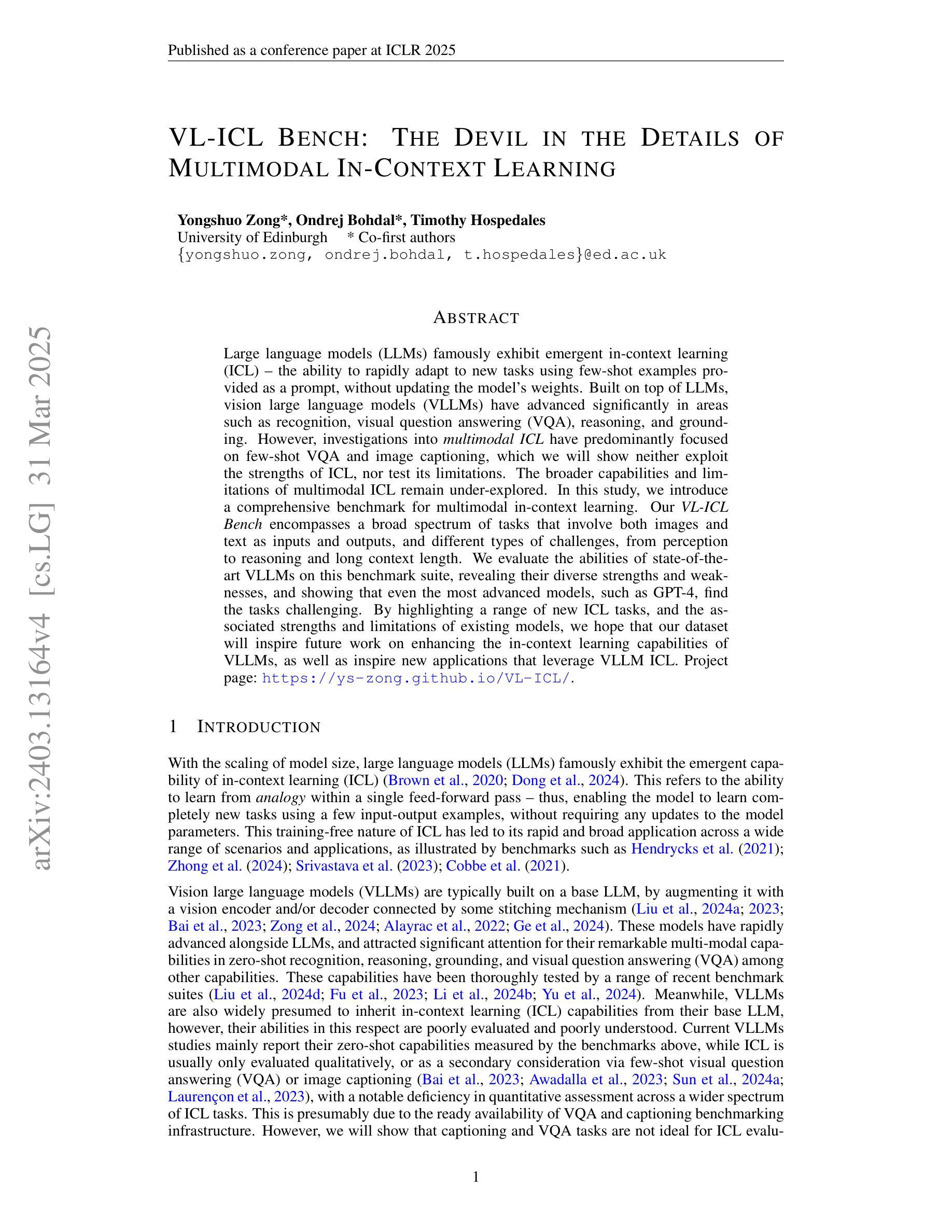
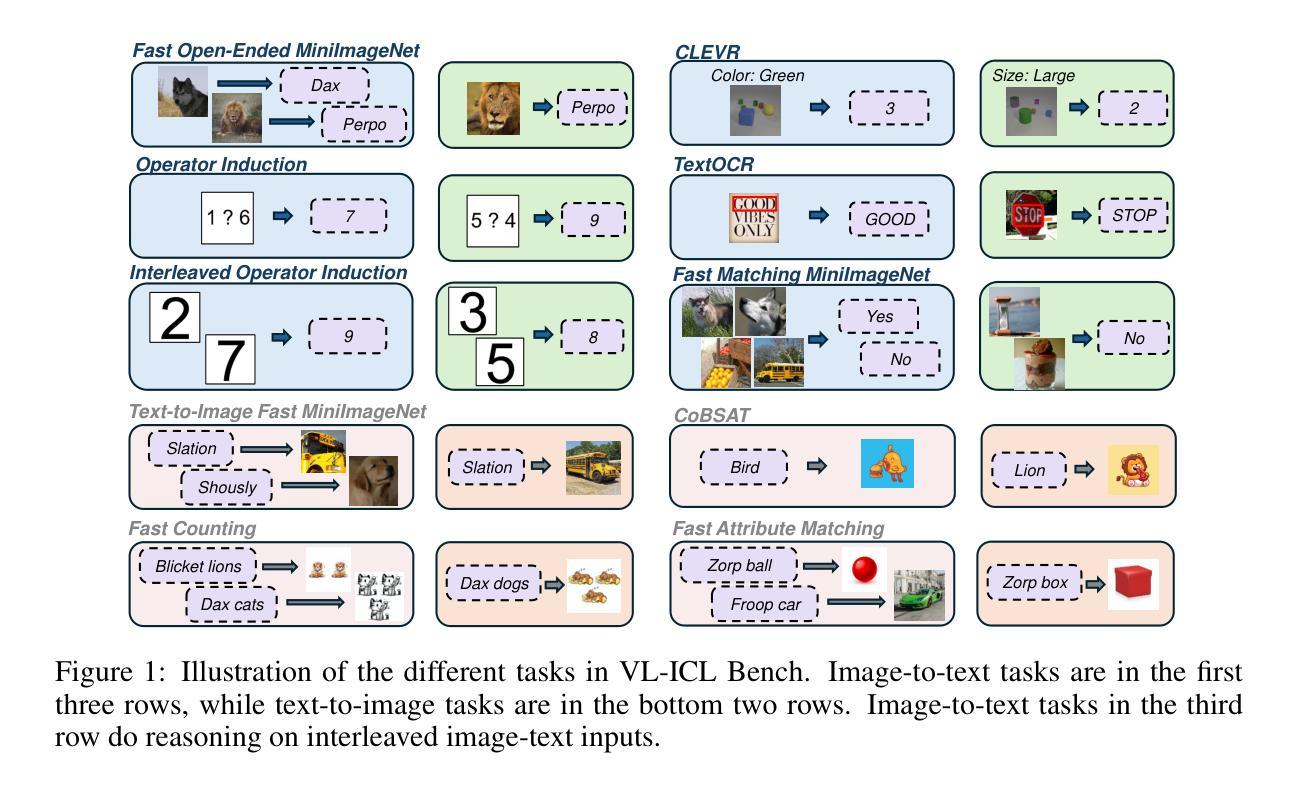
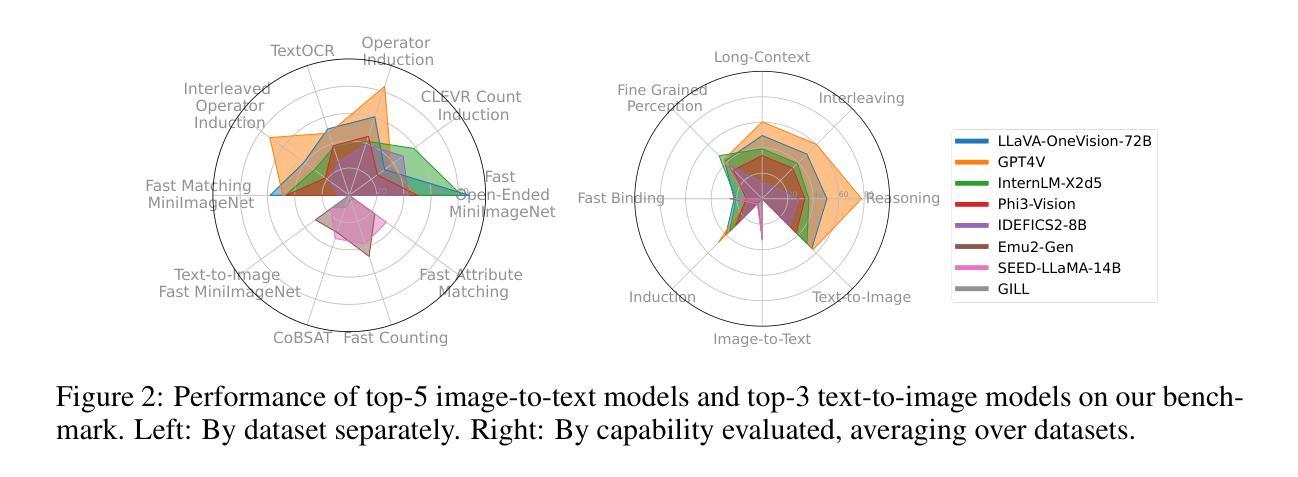
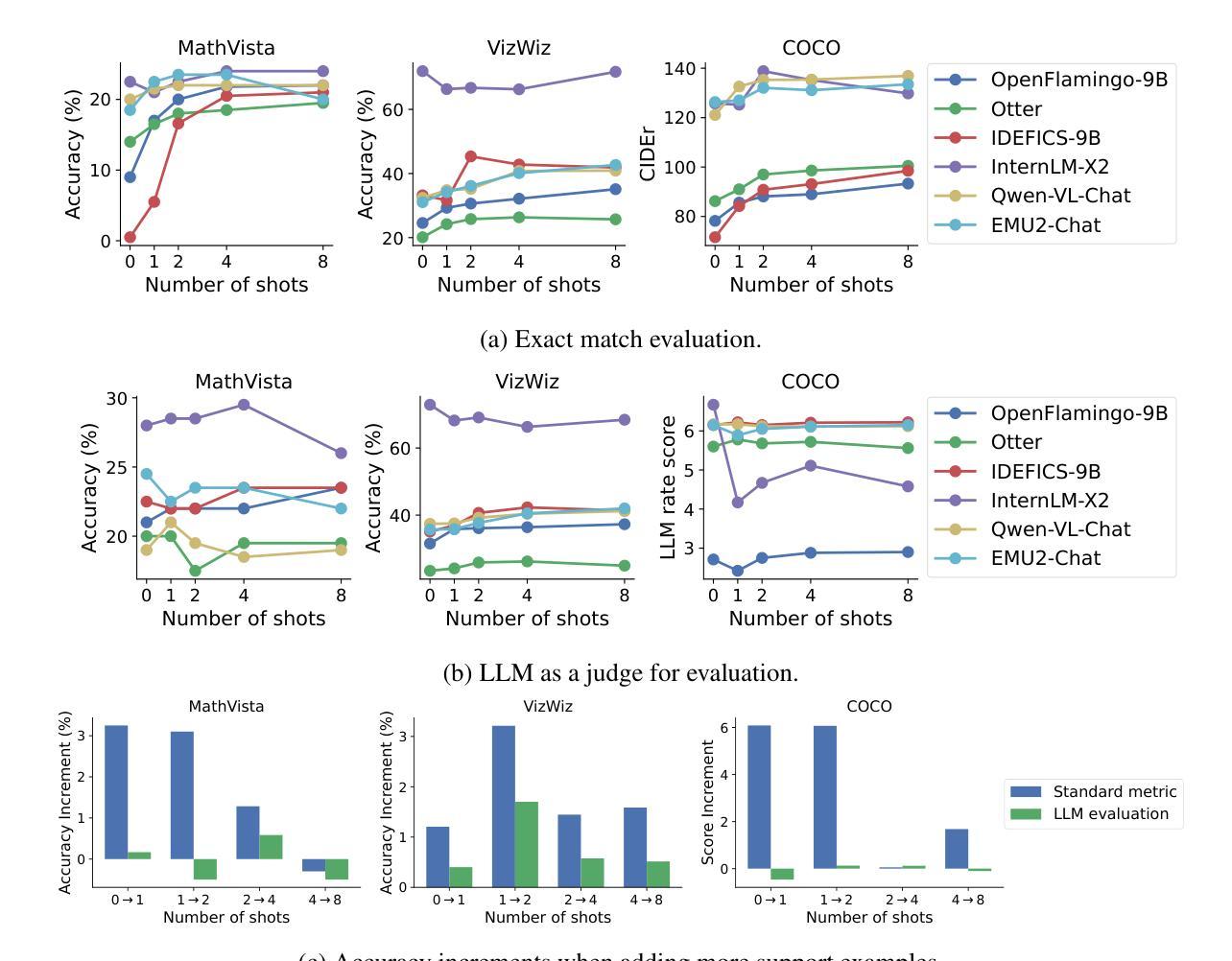
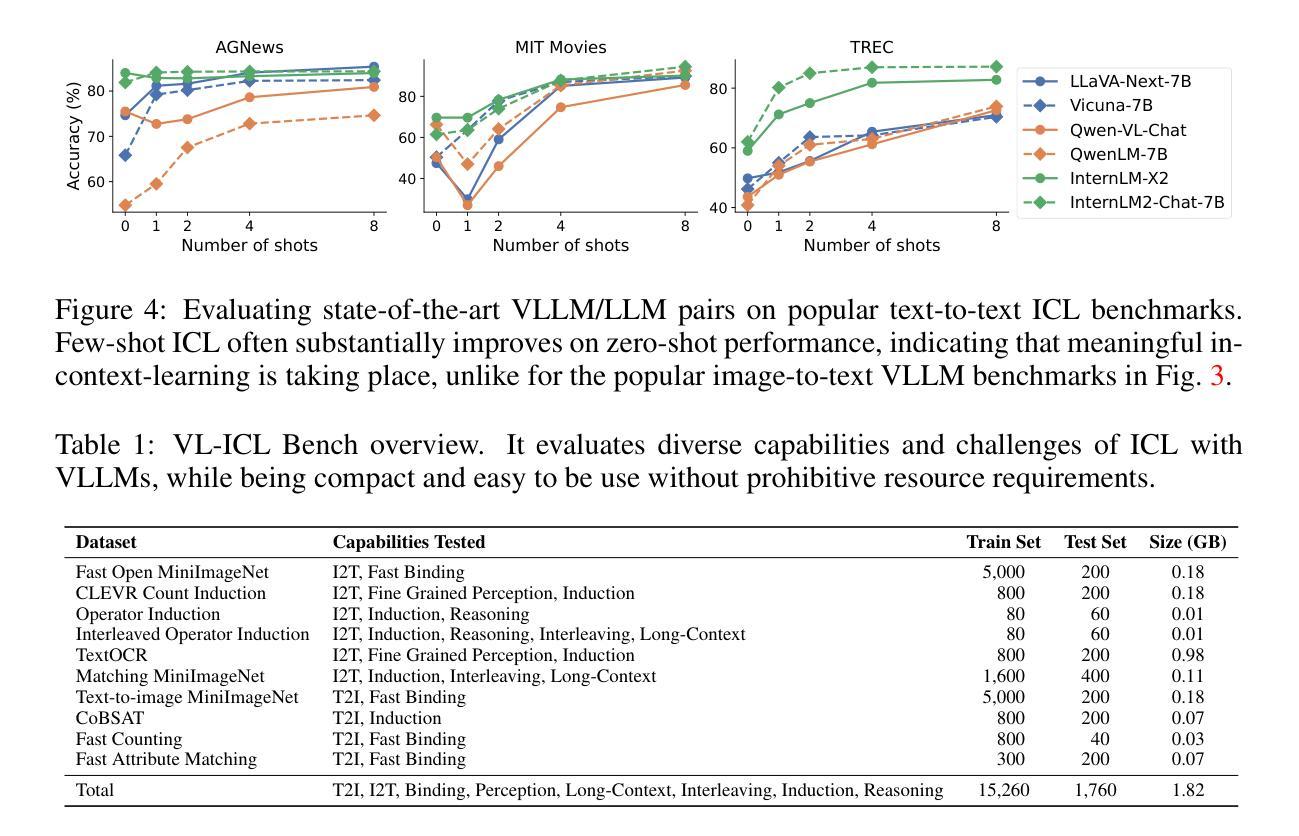