⚠️ 以下所有内容总结都来自于 大语言模型的能力,如有错误,仅供参考,谨慎使用
🔴 请注意:千万不要用于严肃的学术场景,只能用于论文阅读前的初筛!
💗 如果您觉得我们的项目对您有帮助 ChatPaperFree ,还请您给我们一些鼓励!⭐️ HuggingFace免费体验
2025-04-04 更新
Prompting Forgetting: Unlearning in GANs via Textual Guidance
Authors:Piyush Nagasubramaniam, Neeraj Karamchandani, Chen Wu, Sencun Zhu
State-of-the-art generative models exhibit powerful image-generation capabilities, introducing various ethical and legal challenges to service providers hosting these models. Consequently, Content Removal Techniques (CRTs) have emerged as a growing area of research to control outputs without full-scale retraining. Recent work has explored the use of Machine Unlearning in generative models to address content removal. However, the focus of such research has been on diffusion models, and unlearning in Generative Adversarial Networks (GANs) has remained largely unexplored. We address this gap by proposing Text-to-Unlearn, a novel framework that selectively unlearns concepts from pre-trained GANs using only text prompts, enabling feature unlearning, identity unlearning, and fine-grained tasks like expression and multi-attribute removal in models trained on human faces. Leveraging natural language descriptions, our approach guides the unlearning process without requiring additional datasets or supervised fine-tuning, offering a scalable and efficient solution. To evaluate its effectiveness, we introduce an automatic unlearning assessment method adapted from state-of-the-art image-text alignment metrics, providing a comprehensive analysis of the unlearning methodology. To our knowledge, Text-to-Unlearn is the first cross-modal unlearning framework for GANs, representing a flexible and efficient advancement in managing generative model behavior.
当前先进的生成模型展现出强大的图像生成能力,给托管这些模型的服务提供商带来了各种伦理和法律挑战。因此,内容移除技术(CRTs)作为研究的一个不断增长领域出现,旨在控制输出而无需进行全面再训练。近期的研究探索了生成模型中机器遗忘的使用以解决内容移除问题。然而,此类研究的重点在扩散模型上,生成对抗网络(GANs)中的遗忘仍然未被充分探索。我们通过提出Text-to-Unlearn来解决这一问题,这是一个使用文本提示从预训练的GANs中选择性遗忘概念的新框架,可实现特征遗忘、身份遗忘和在人脸训练模型中的表情和多属性移除等精细任务。我们的方法利用自然语言描述来引导遗忘过程,无需额外的数据集或监督微调,提供可伸缩和高效的解决方案。为了评估其有效性,我们引入了一种自动遗忘评估方法,该方法改编自最先进的图像文本对齐指标,对遗忘方法进行全面分析。据我们所知,Text-to-Unlearn是第一个用于GANs的跨模式遗忘框架,代表着管理生成模型行为的一个灵活而高效的进步。
论文及项目相关链接
Summary
本文提出了一种名为Text-to-Unlearn的新型框架,该框架能够通过文本提示选择性地遗忘预训练的GAN模型中的概念,实现特征遗忘、身份遗忘以及表情和多属性移除等精细任务。该方法利用自然语言描述来引导遗忘过程,无需额外的数据集或监督微调,提供可伸缩和高效的解决方案。
Key Takeaways
- Text-to-Unlearn框架能够选择性地遗忘GAN模型中的特定概念。
- 框架支持特征遗忘、身份遗忘以及表情和多属性移除等精细任务。
- 该方法利用自然语言描述来引导遗忘过程。
- Text-to-Unlearn不需要额外的数据集或监督微调。
- 引入了一种自动遗忘评估方法,来自适应分析遗忘方法的有效性。
- Text-to-Unlearn是首个针对GANs的跨模态遗忘框架。
点此查看论文截图
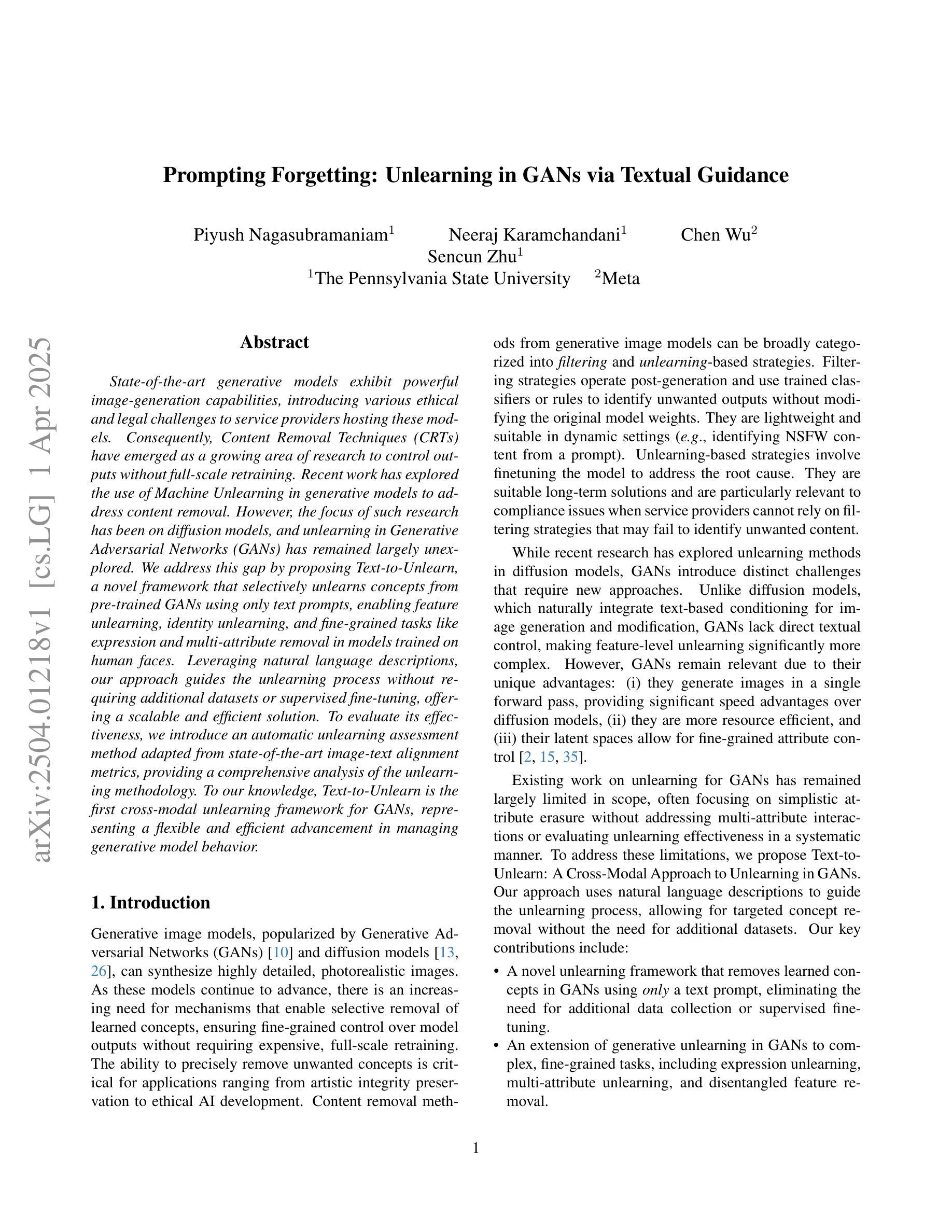
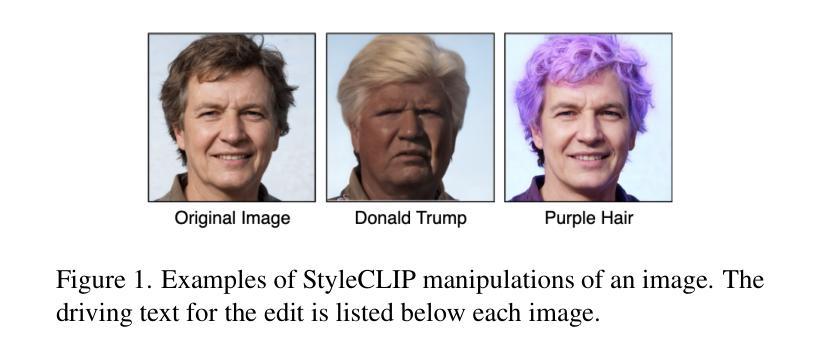
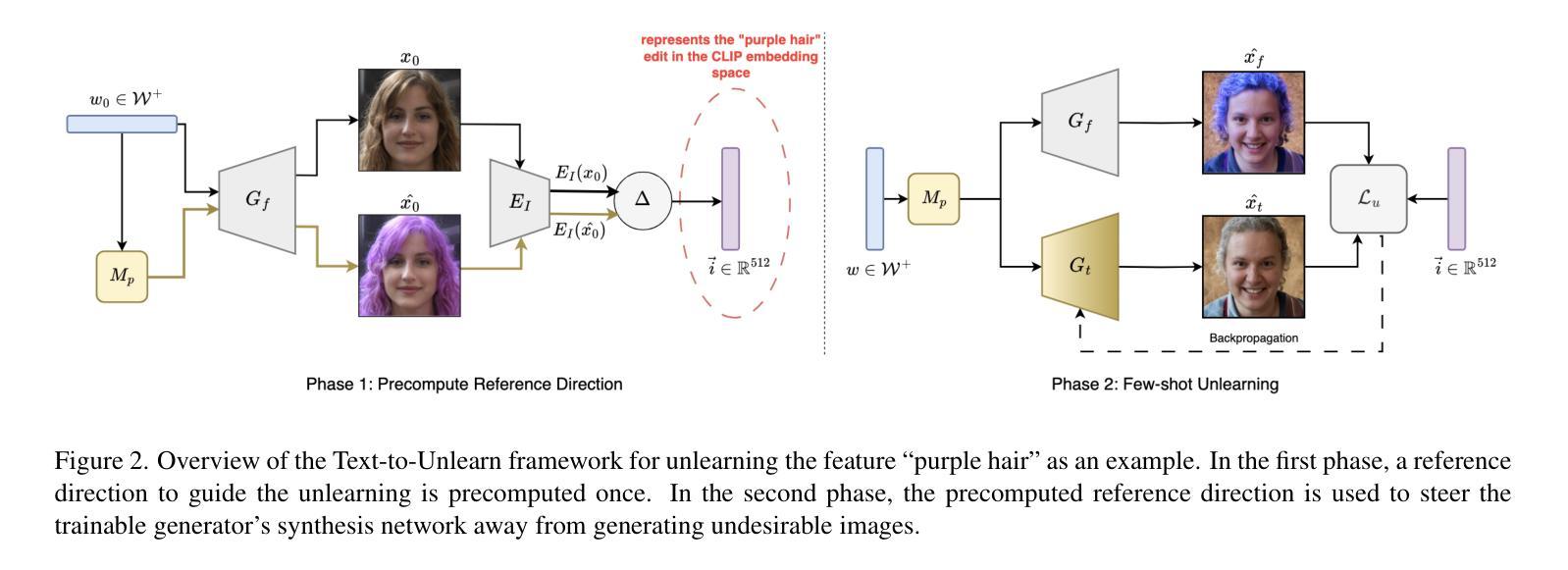
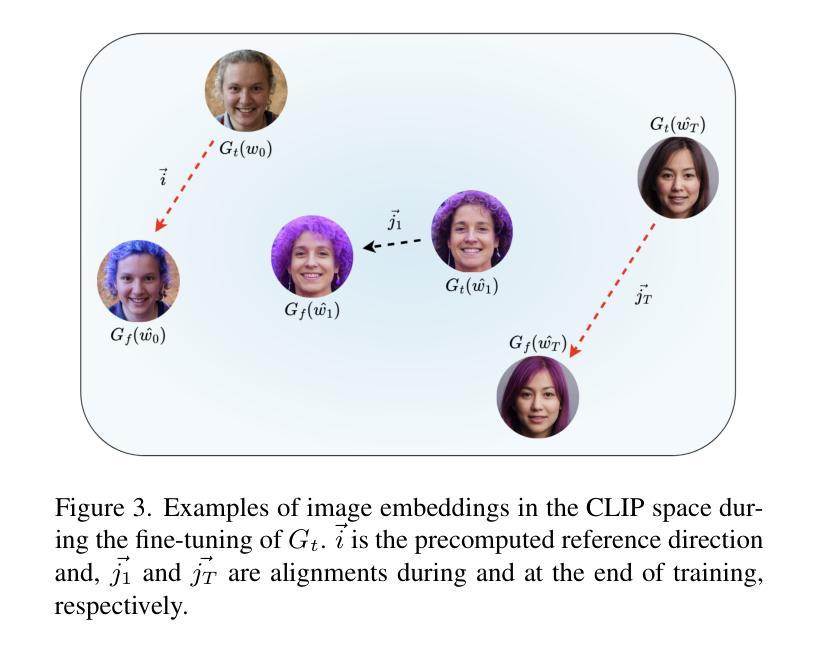
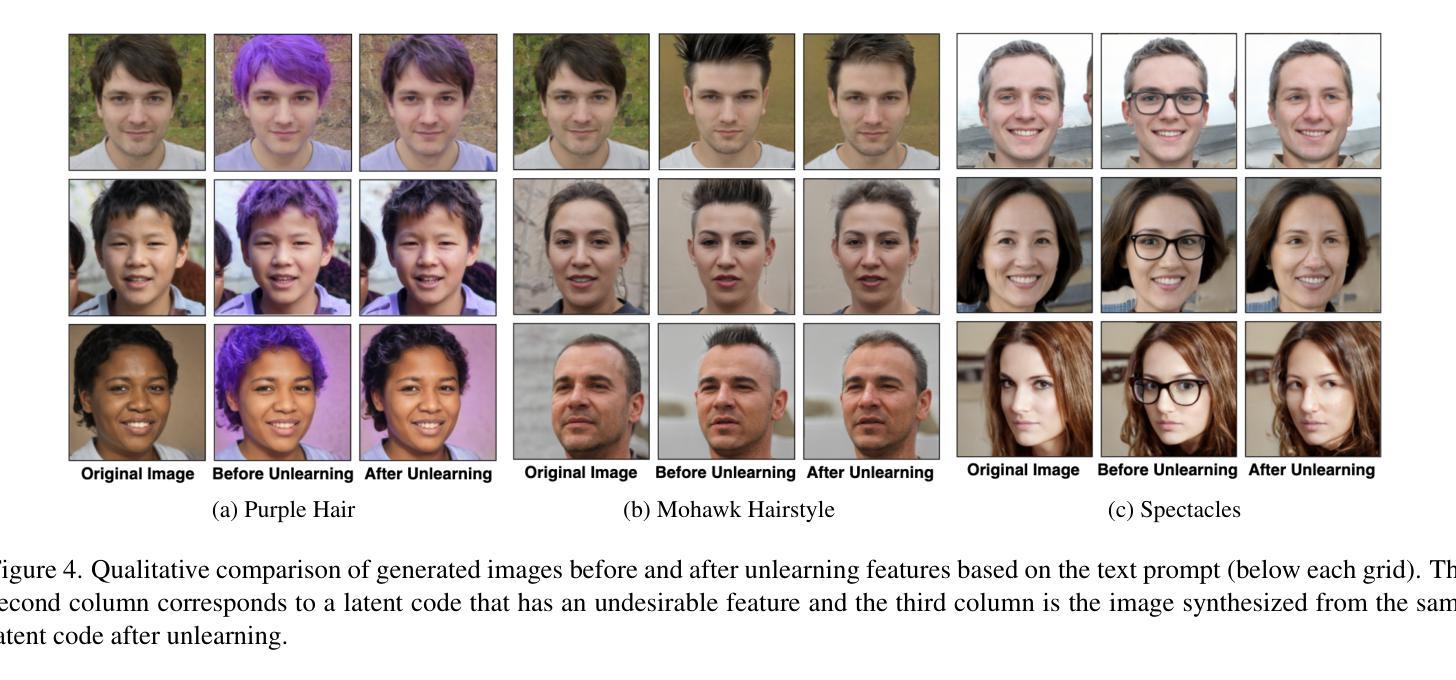
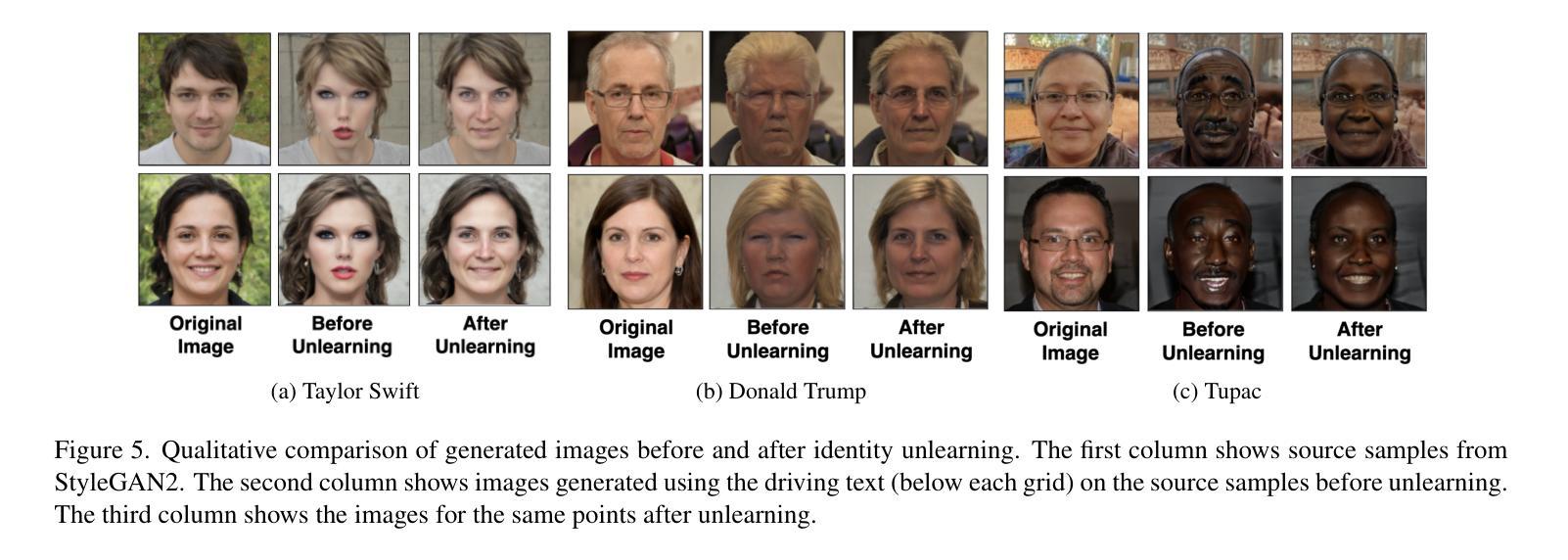
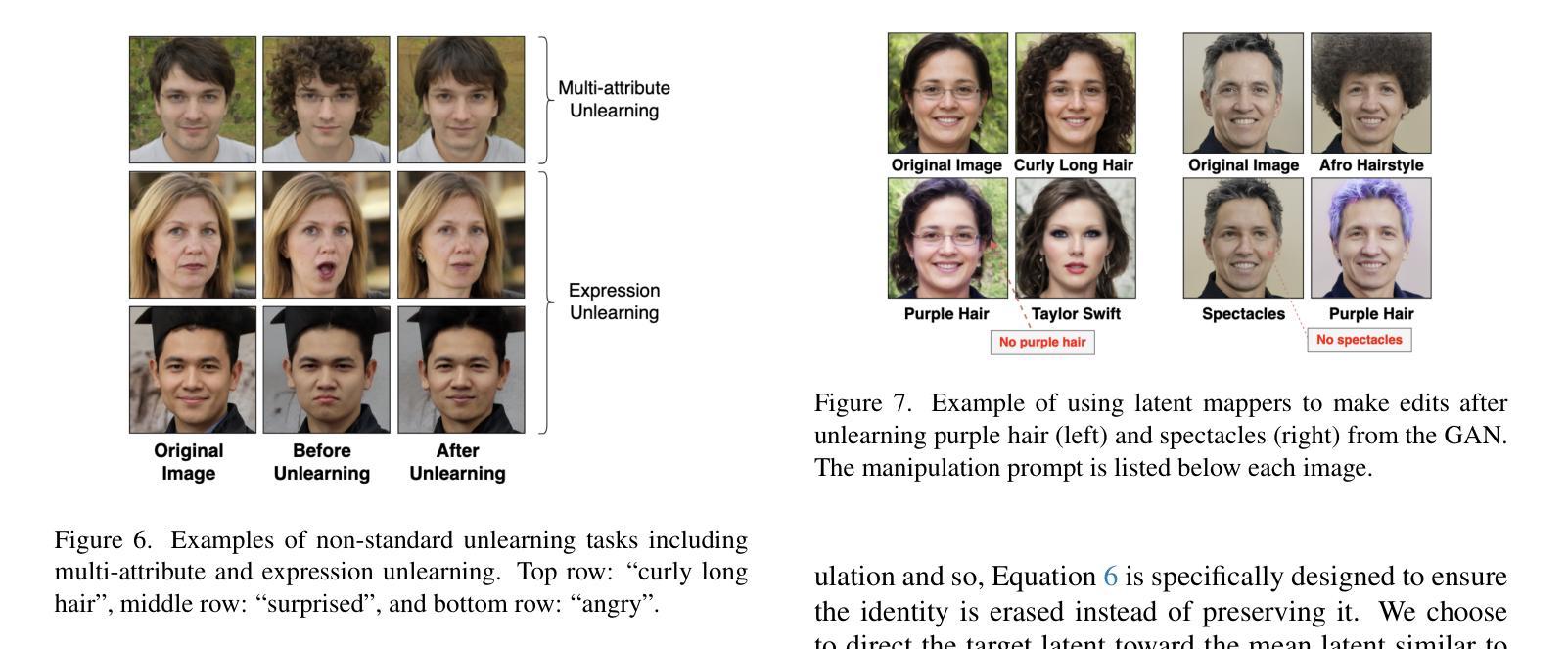
Generalization-aware Remote Sensing Change Detection via Domain-agnostic Learning
Authors:Qi Zang, Shuang Wang, Dong Zhao, Dou Quan, Yang Hu, Licheng Jiao
Change detection has essential significance for the region’s development, in which pseudo-changes between bitemporal images induced by imaging environmental factors are key challenges. Existing transformation-based methods regard pseudo-changes as a kind of style shift and alleviate it by transforming bitemporal images into the same style using generative adversarial networks (GANs). However, their efforts are limited by two drawbacks: 1) Transformed images suffer from distortion that reduces feature discrimination. 2) Alignment hampers the model from learning domain-agnostic representations that degrades performance on scenes with domain shifts from the training data. Therefore, oriented from pseudo-changes caused by style differences, we present a generalizable domain-agnostic difference learning network (DonaNet). For the drawback 1), we argue for local-level statistics as style proxies to assist against domain shifts. For the drawback 2), DonaNet learns domain-agnostic representations by removing domain-specific style of encoded features and highlighting the class characteristics of objects. In the removal, we propose a domain difference removal module to reduce feature variance while preserving discriminative properties and propose its enhanced version to provide possibilities for eliminating more style by decorrelating the correlation between features. In the highlighting, we propose a cross-temporal generalization learning strategy to imitate latent domain shifts, thus enabling the model to extract feature representations more robust to shifts actively. Extensive experiments conducted on three public datasets demonstrate that DonaNet outperforms existing state-of-the-art methods with a smaller model size and is more robust to domain shift.
变化检测对区域发展具有重大意义,其中由成像环境因素引起的双时相图像之间的伪变化是关键挑战。现有的基于变换的方法将伪变化视为一种风格转变,并通过使用生成对抗网络(GAN)将双时相图像转换为相同风格来缓解这一问题。然而,他们的努力受限于两个缺点:1)变换后的图像会出现失真,降低特征辨别能力。2)对齐问题阻碍了模型学习领域无关的表示,降低了在场景域从训练数据中迁移时的性能。因此,针对由风格差异引起的伪变化,我们提出了一种通用的领域无关差异学习网络(DonaNet)。针对缺点一,我们主张使用局部级别的统计信息作为风格代理来帮助对抗领域迁移。针对缺点二,DonaNet通过学习去除编码特征的特定领域风格并突出对象的类特征来学习领域无关的表示。在去除过程中,我们提出了一个领域差异去除模块,以降低特征方差的同时保留判别属性,并提出了其增强版,通过解特征之间的相关性来消除更多风格的可能性。在突出过程中,我们提出了一种跨时间泛化学习策略来模拟潜在领域迁移,从而使模型能够更主动地提取对迁移更稳健的特征表示。在三个公共数据集上进行的广泛实验表明,DonaNet在较小的模型规模上优于现有最先进的方法,并且对领域迁移更具鲁棒性。
论文及项目相关链接
Summary
针对环境变化导致的伪变化问题,提出了一个通用的领域无关差异学习网络(DonaNet)。通过利用局部级别的统计信息作为风格代理来对抗领域偏移,同时学习领域无关的表示,通过去除特征中的特定风格并突出对象类别特征来解决现有方法的局限。DonaNet能有效去除风格并突出鉴别属性,实验结果证明了其在三个公开数据集上的优越性能。
Key Takeaways
- 伪变化是环境成像因素导致的双时态图像间的主要挑战之一。
- 现有方法通过将双时态图像转换为同一风格来解决伪变化问题,但存在图像失真和模型对齐问题。
- DonaNet通过利用局部级别的统计信息来对抗领域偏移,解决了上述问题。
- DonaNet通过学习去除特征中的特定风格并突出对象类别特征来学习领域无关的表示。
- DonaNet通过提出的领域差异去除模块减少了特征方差,同时保留了鉴别属性。
- DonaNet在模仿潜在领域偏移的策略上进行了创新,使其能够更稳健地提取特征表示。
点此查看论文截图
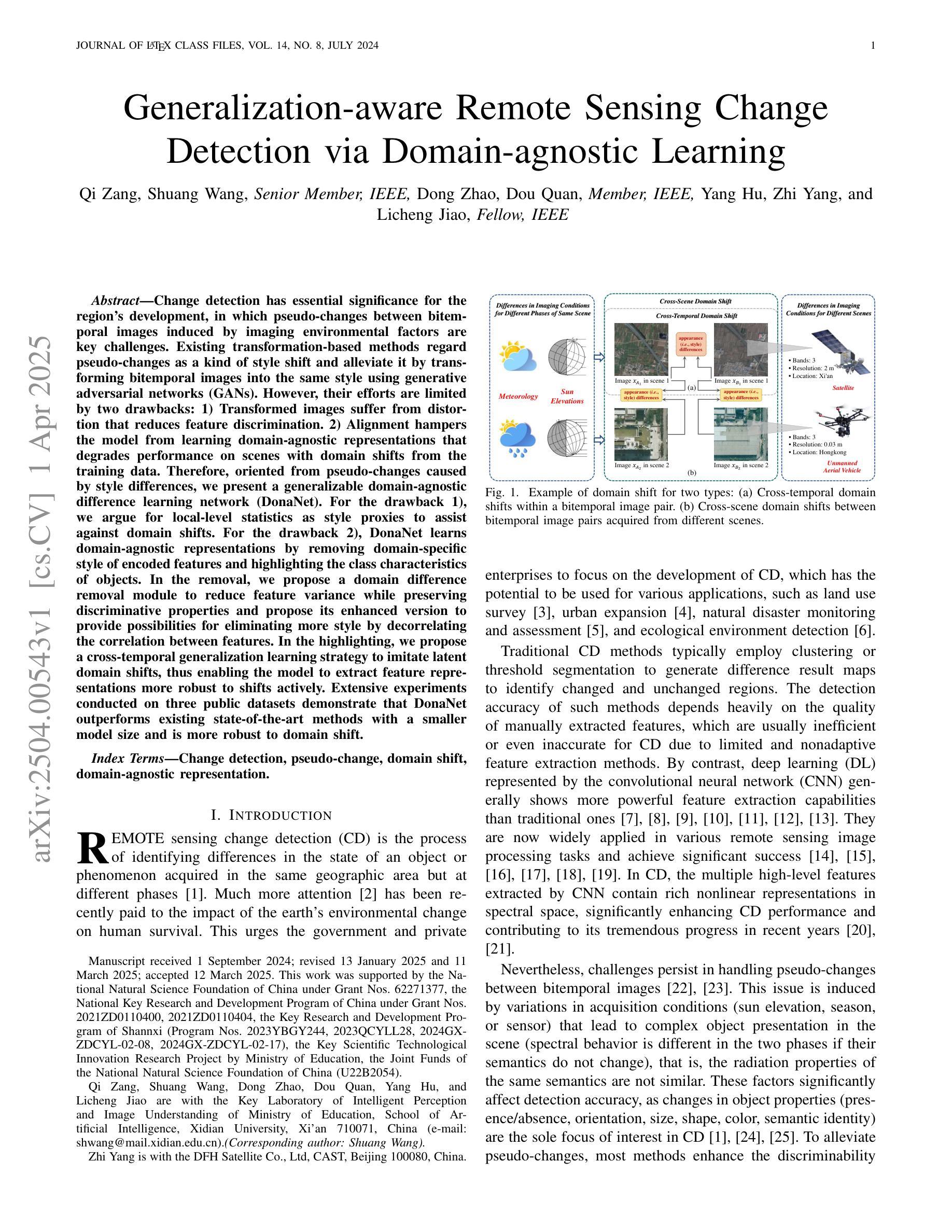
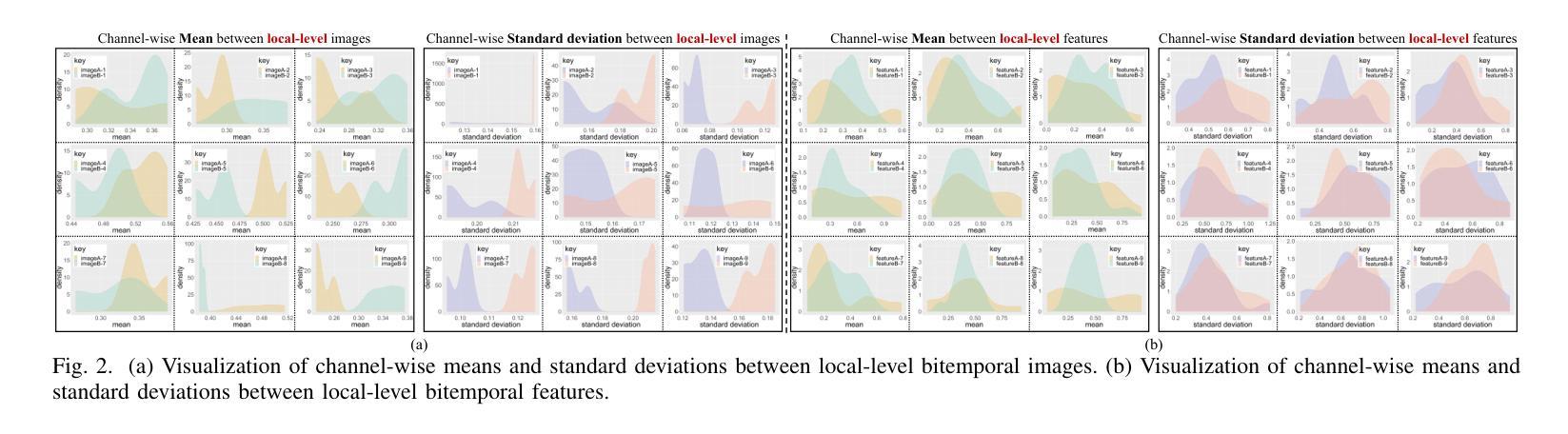
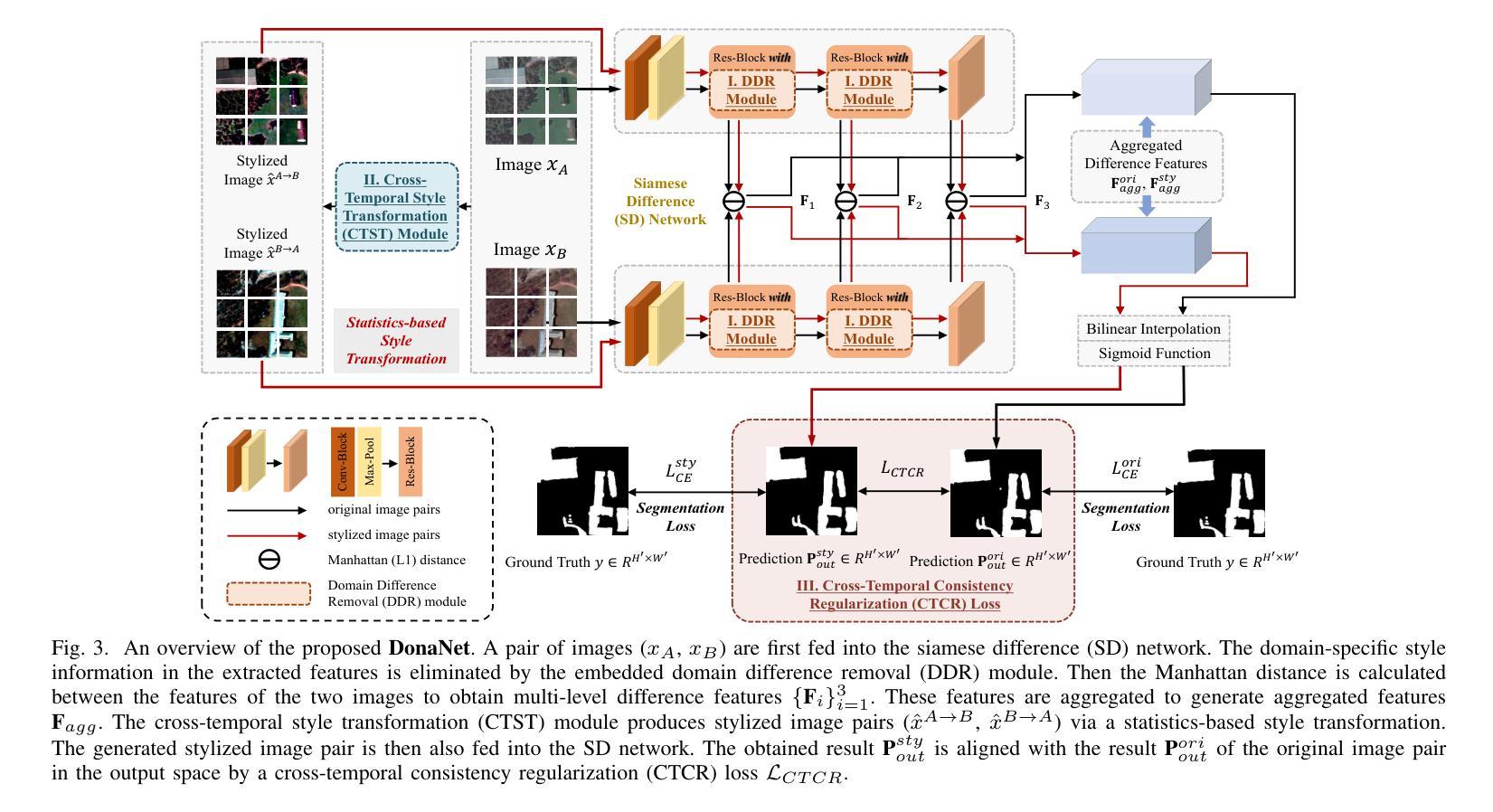
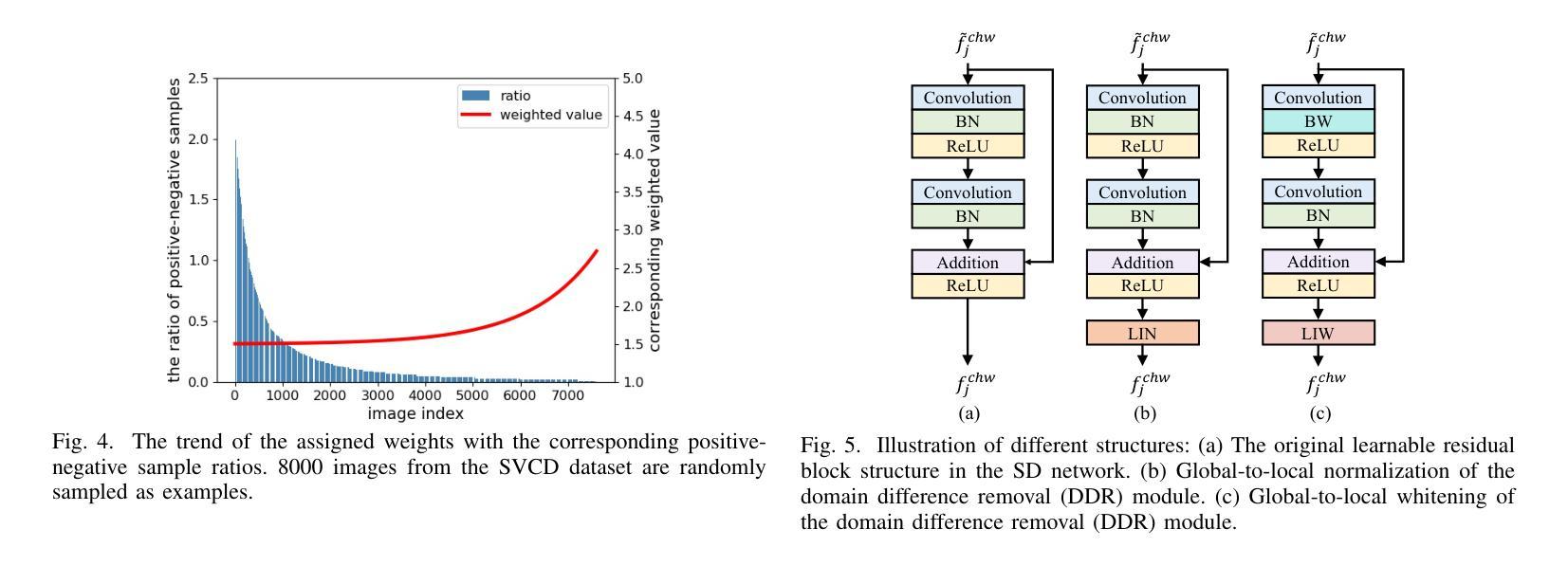
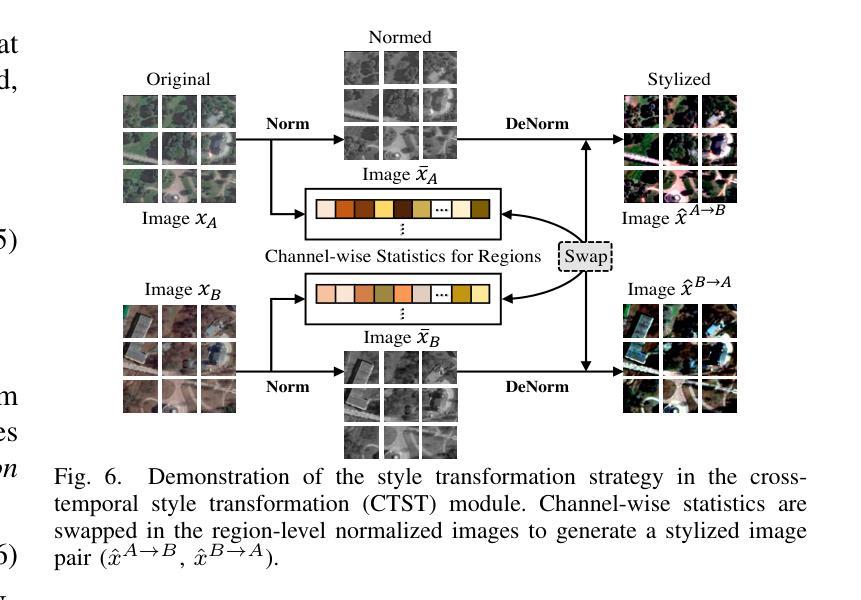
Exploring the Collaborative Advantage of Low-level Information on Generalizable AI-Generated Image Detection
Authors:Ziyin Zhou, Ke Sun, Zhongxi Chen, Xianming Lin, Yunpeng Luo, Ke Yan, Shouhong Ding, Xiaoshuai Sun
Existing state-of-the-art AI-Generated image detection methods mostly consider extracting low-level information from RGB images to help improve the generalization of AI-Generated image detection, such as noise patterns. However, these methods often consider only a single type of low-level information, which may lead to suboptimal generalization. Through empirical analysis, we have discovered a key insight: different low-level information often exhibits generalization capabilities for different types of forgeries. Furthermore, we found that simple fusion strategies are insufficient to leverage the detection advantages of each low-level and high-level information for various forgery types. Therefore, we propose the Adaptive Low-level Experts Injection (ALEI) framework. Our approach introduces Lora Experts, enabling the backbone network, which is trained with high-level semantic RGB images, to accept and learn knowledge from different low-level information. We utilize a cross-attention method to adaptively fuse these features at intermediate layers. To prevent the backbone network from losing the modeling capabilities of different low-level features during the later stages of modeling, we developed a Low-level Information Adapter that interacts with the features extracted by the backbone network. Finally, we propose Dynamic Feature Selection, which dynamically selects the most suitable features for detecting the current image to maximize generalization detection capability. Extensive experiments demonstrate that our method, finetuned on only four categories of mainstream ProGAN data, performs excellently and achieves state-of-the-art results on multiple datasets containing unseen GAN and Diffusion methods.
现有最先进的AI生成图像检测的方法大多是从RGB图像中提取低级信息来帮助提高AI生成图像检测的泛化能力,例如噪声模式。然而,这些方法通常只考虑一种类型的低级信息,可能会导致次优的泛化效果。通过实证分析,我们发现了一个关键观点:不同的低级信息往往对不同类型的伪造表现出不同的泛化能力。此外,我们发现简单的融合策略不足以利用每种低级和高级信息对不同伪造类型的检测优势。因此,我们提出了自适应低级专家注入(ALEI)框架。我们的方法引入了Lora专家,使经过高级语义RGB图像训练的骨干网络能够接受和学习来自不同低级信息的知识。我们使用交叉注意方法来自适应地融合中间层的这些特征。为了防止骨干网络在建模后期失去对不同低级特征的建模能力,我们开发了一个低级信息适配器,它与骨干网络提取的特征进行交互。最后,我们提出了动态特征选择,它动态选择最适合检测当前图像的特征,以最大限度地提高泛化检测能力。大量实验表明,我们的方法仅在四种主流ProGAN数据上进行微调,即可在多数据集上取得出色的表现,达到业界领先水平,包括未见过的GAN和Diffusion方法。
论文及项目相关链接
Summary
现有AI生成图像检测的方法主要通过提取RGB图像的底层信息来提高检测泛化能力,如噪声模式。但这种方法往往只考虑单一类型的底层信息,可能导致泛化效果不佳。研究发现,不同底层信息对不同类型的伪造图像具有不同的泛化能力。为提高检测各种伪造类型的能力,提出了自适应底层专家注入(ALEI)框架。该框架引入Lora专家,使训练有高级语义RGB图像的背景网络能够接受并学习各种底层信息的知识。使用跨注意力方法在中间层自适应融合这些特征。为防止背景网络在后期建模中失去对底层特征的建模能力,开发了底层信息适配器,与背景网络提取的特征进行交互。最后,提出了动态特征选择,能够动态选择最适合检测当前图像的特征,以最大化泛化检测能力。实验表明,该方法在多个包含未见过的GAN和扩散方法的数据集上表现优异,达到最新技术水平。
Key Takeaways
- 当前AI生成图像检测主要依赖RGB图像的底层信息来提高泛化能力,但存在局限性。
- 不同底层信息对不同类型的伪造图像具有不同的泛化能力。
- 提出了ALEI框架,通过引入Lora专家结合底层和高层次信息。
- 使用跨注意力方法在中间层融合特征。
- 开发了底层信息适配器,保持对底层特征的建模能力。
- 动态特征选择能最大化泛化检测能力。
- 在多个数据集上实现优异性能,达到最新技术水平。
点此查看论文截图
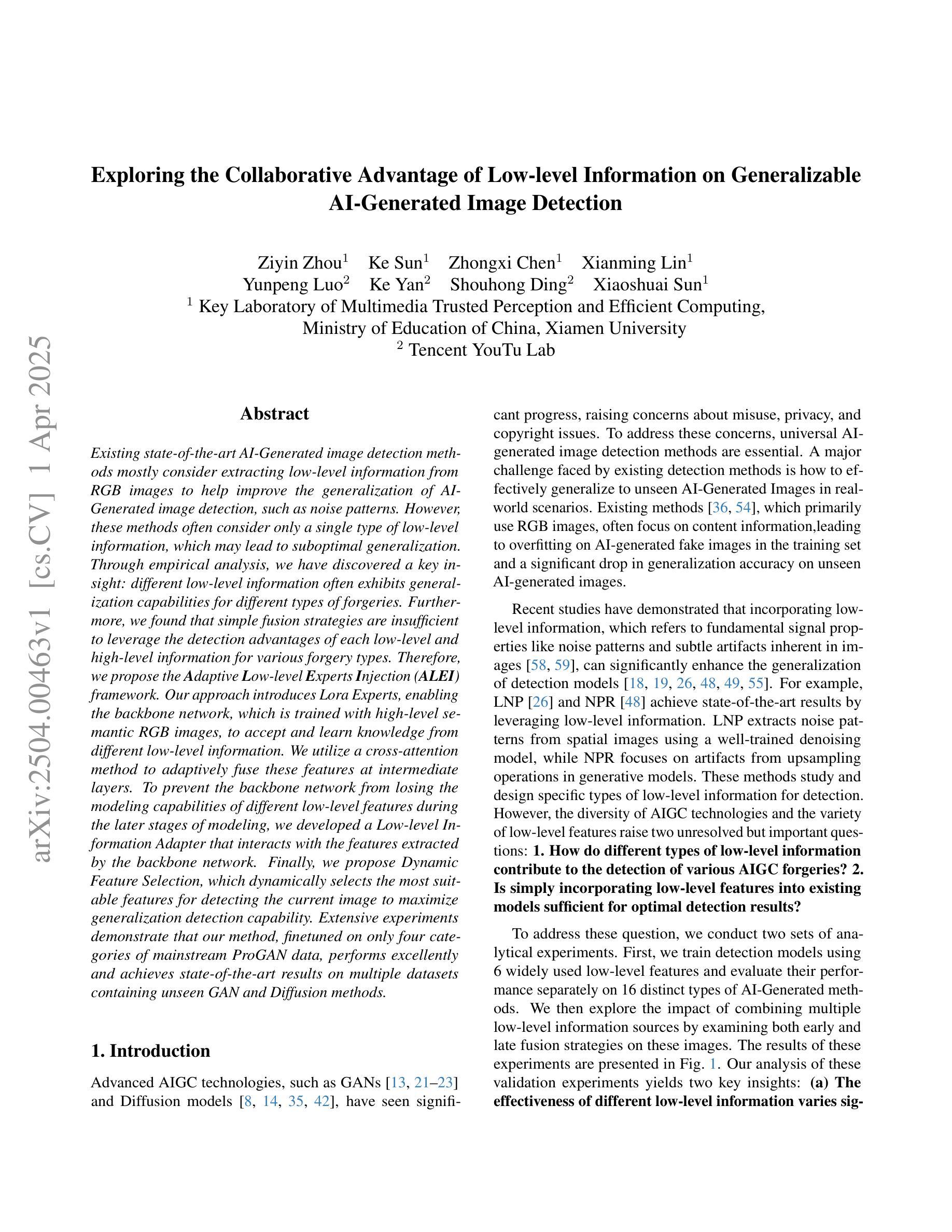
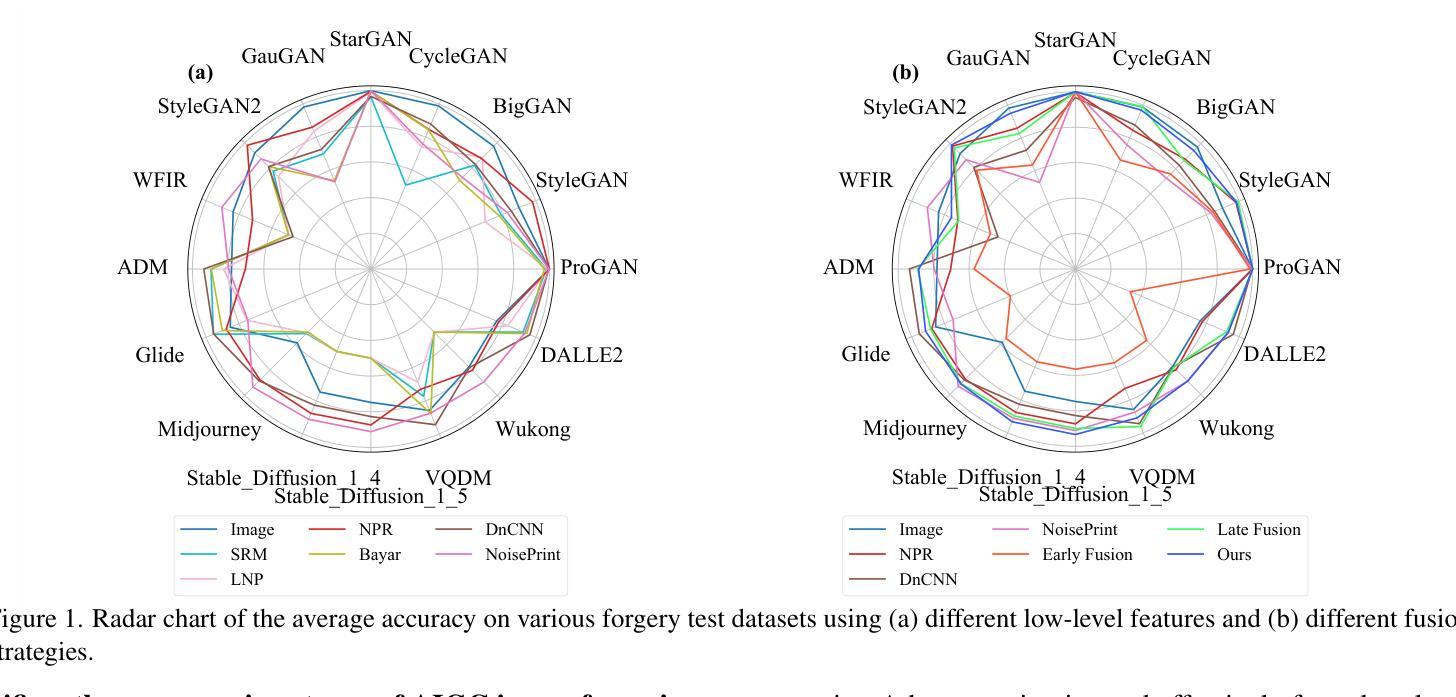
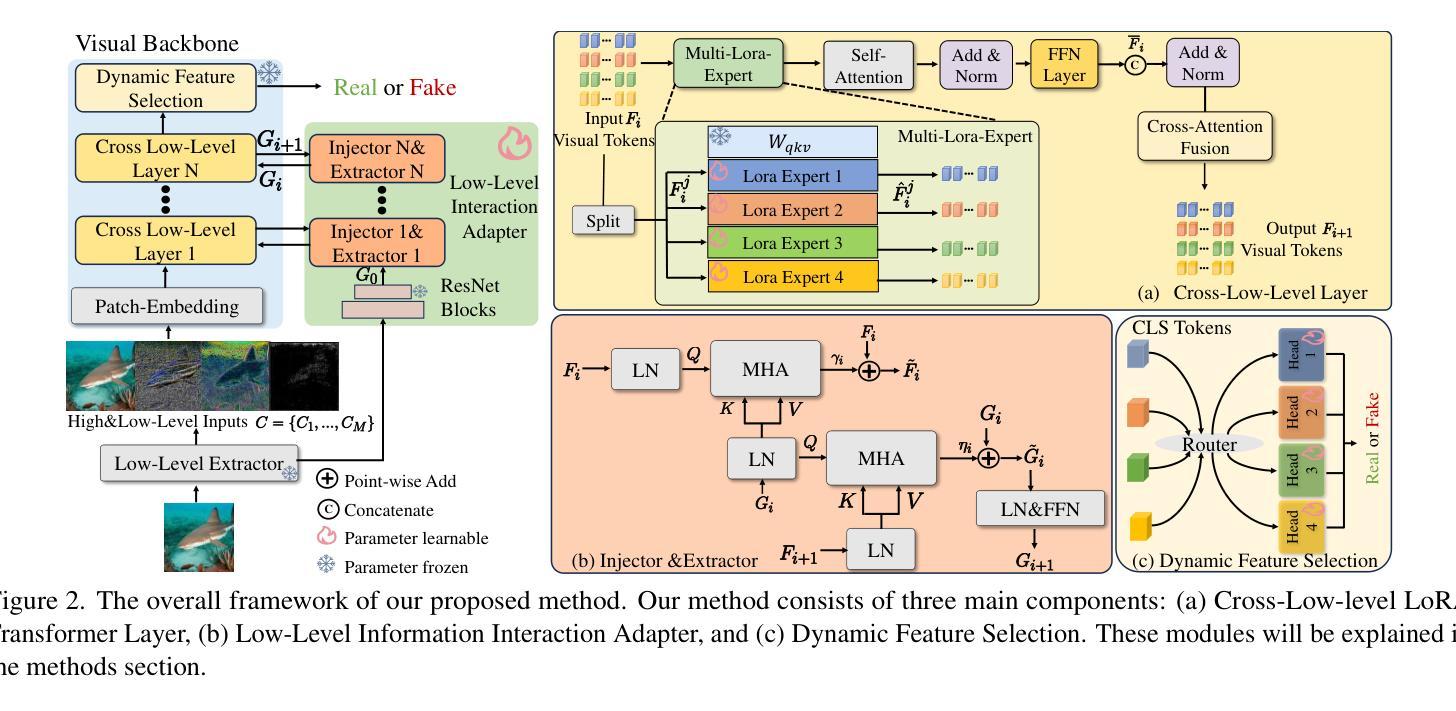
Style Quantization for Data-Efficient GAN Training
Authors:Jian Wang, Xin Lan, Jizhe Zhou, Yuxin Tian, Jiancheng Lv
Under limited data setting, GANs often struggle to navigate and effectively exploit the input latent space. Consequently, images generated from adjacent variables in a sparse input latent space may exhibit significant discrepancies in realism, leading to suboptimal consistency regularization (CR) outcomes. To address this, we propose \textit{SQ-GAN}, a novel approach that enhances CR by introducing a style space quantization scheme. This method transforms the sparse, continuous input latent space into a compact, structured discrete proxy space, allowing each element to correspond to a specific real data point, thereby improving CR performance. Instead of direct quantization, we first map the input latent variables into a less entangled ``style’’ space and apply quantization using a learnable codebook. This enables each quantized code to control distinct factors of variation. Additionally, we optimize the optimal transport distance to align the codebook codes with features extracted from the training data by a foundation model, embedding external knowledge into the codebook and establishing a semantically rich vocabulary that properly describes the training dataset. Extensive experiments demonstrate significant improvements in both discriminator robustness and generation quality with our method.
在有限数据设置下,生成对抗网络(GANs)往往难以导航并有效地利用输入潜在空间。因此,从稀疏输入潜在空间中的相邻变量生成的图像在逼真度方面可能存在显著差异,从而导致次优的一致性正则化(CR)结果。为了解决这一问题,我们提出了SQ-GAN这一新方法,它通过引入风格空间量化方案来增强CR。该方法将稀疏、连续的输入潜在空间转换为紧凑、结构化的离散代理空间,使每个元素都能对应一个特定的真实数据点,从而提高CR性能。我们不是直接进行量化,而是首先将输入潜在变量映射到一个不那么纠缠的“风格”空间,并使用可学习的代码本进行量化。这使得每个量化的代码能够控制不同的变化因素。此外,我们优化了最佳传输距离,将代码本中的代码与基础模型从训练数据中提取的特征进行对齐,将外部知识嵌入到代码本中,建立了一个语义丰富的词汇表,适当地描述了训练数据集。大量实验证明,我们的方法在判别器鲁棒性和生成质量方面都有显著提高。
论文及项目相关链接
Summary
在有限数据环境下,生成对抗网络(GANs)在输入潜在空间的导航和有效探索方面常面临挑战。针对这一问题,本文提出了SQ-GAN,通过引入风格空间量化方案,增强一致性正则化(CR)的性能。该方法将稀疏的连续输入潜在空间转化为紧凑的结构化离散代理空间,使每个元素对应一个真实数据点,从而提高CR性能。实验表明,该方法在判别器鲁棒性和生成质量方面均有显著提高。
Key Takeaways
- 在有限数据环境下,GANs在导航和有效探索输入潜在空间时面临挑战。
- 提出的SQ-GAN通过风格空间量化方案增强一致性正则化(CR)性能。
- SQ-GAN将稀疏的连续输入潜在空间转化为紧凑的结构化离散代理空间。
- 该方法使每个量化代码对应一个真实数据点,提高CR性能。
- 采用学习代码本进行量化,使每个量化代码控制不同的变量因素。
- 通过优化传输距离,将代码本代码与基础模型提取的特征对齐,嵌入外部知识,建立丰富的语义词汇表。
点此查看论文截图
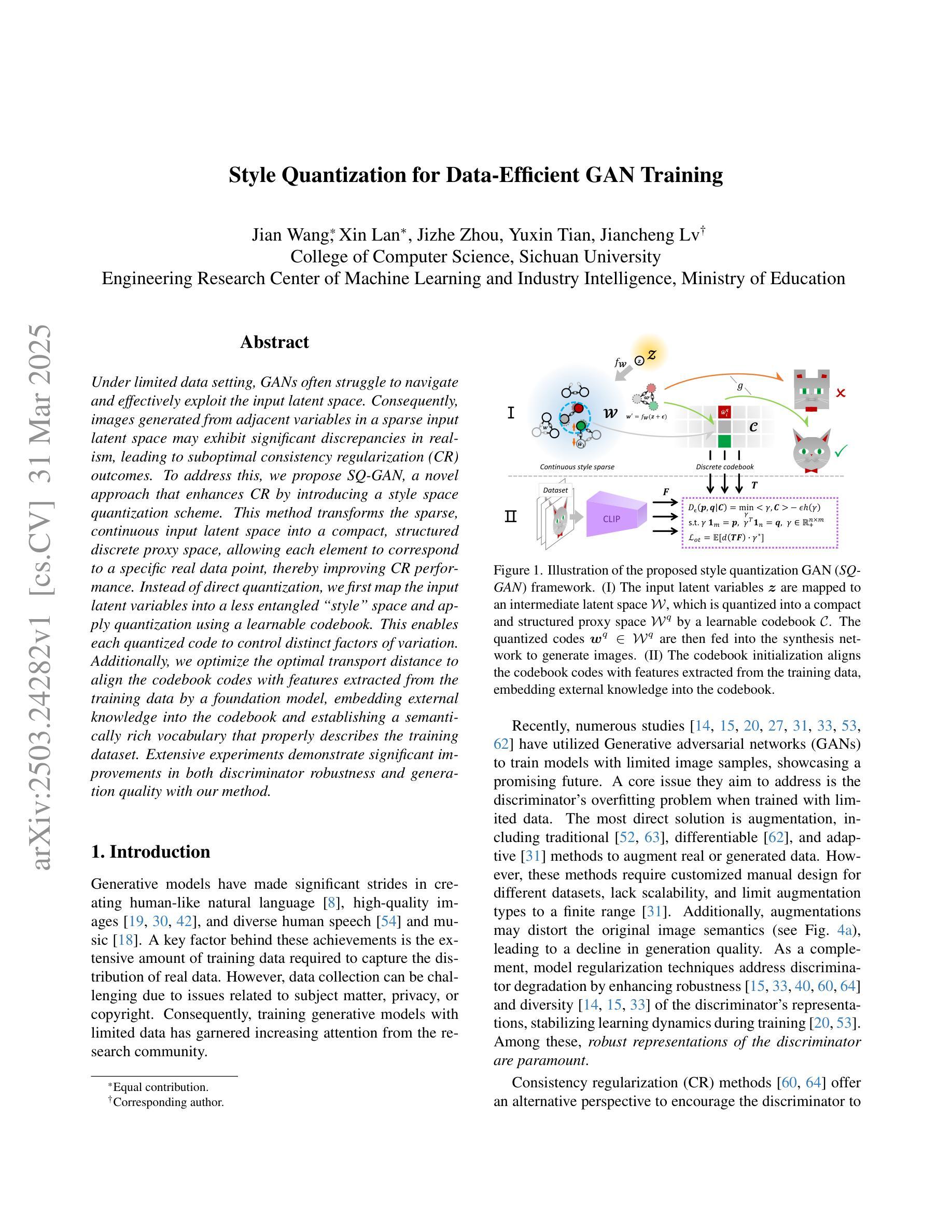
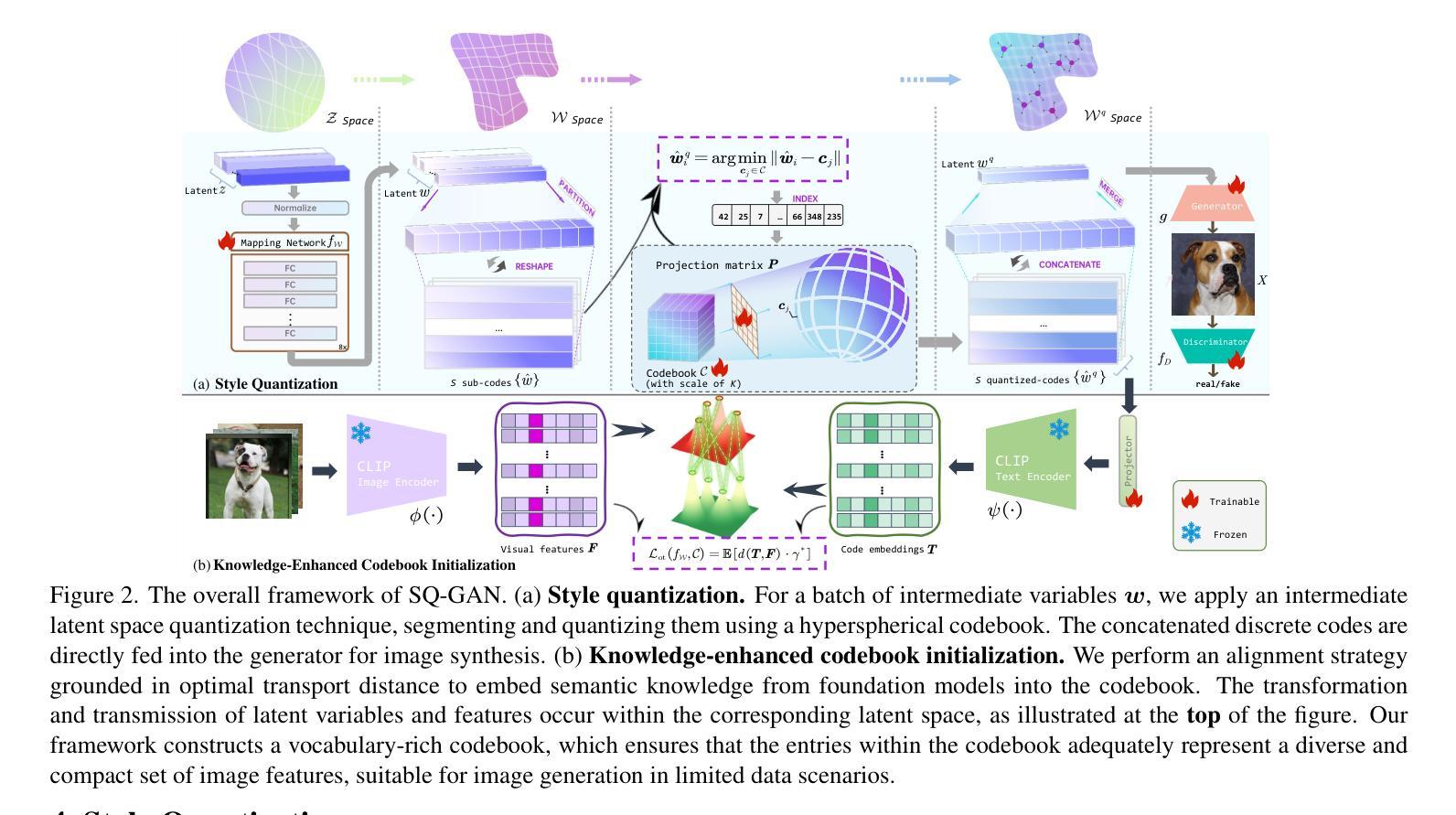
Deterministic Medical Image Translation via High-fidelity Brownian Bridges
Authors:Qisheng He, Nicholas Summerfield, Peiyong Wang, Carri Glide-Hurst, Ming Dong
Recent studies have shown that diffusion models produce superior synthetic images when compared to Generative Adversarial Networks (GANs). However, their outputs are often non-deterministic and lack high fidelity to the ground truth due to the inherent randomness. In this paper, we propose a novel High-fidelity Brownian bridge model (HiFi-BBrg) for deterministic medical image translations. Our model comprises two distinct yet mutually beneficial mappings: a generation mapping and a reconstruction mapping. The Brownian bridge training process is guided by the fidelity loss and adversarial training in the reconstruction mapping. This ensures that translated images can be accurately reversed to their original forms, thereby achieving consistent translations with high fidelity to the ground truth. Our extensive experiments on multiple datasets show HiFi-BBrg outperforms state-of-the-art methods in multi-modal image translation and multi-image super-resolution.
最近的研究表明,与生成对抗网络(GANs)相比,扩散模型在生成合成图像方面表现更优越。然而,由于其内在的随机性,它们的输出通常是非确定的,并且对真实情况的保真度不高。在本文中,我们提出了一种用于确定性医学图像翻译的新型高保真布朗桥模型(HiFi-BBrg)。我们的模型包括两个独特而相互有益的映射:生成映射和重建映射。布朗桥训练过程由重建映射中的保真度损失和对抗性训练引导。这确保了翻译后的图像可以准确地恢复到其原始形式,从而实现与真实情况高度一致的翻译。我们在多个数据集上的广泛实验表明,HiFi-BBrg在跨模态图像翻译和多图像超分辨率方面优于最先进的方法。
论文及项目相关链接
Summary:最近研究表明扩散模型在生成合成图像方面优于生成对抗网络(GANs),但其输出通常具有非确定性且对真实数据缺乏高保真度。本文提出了一种新型的高保真布朗桥模型(HiFi-BBrg)用于确定性医学图像转换。该模型包含两个独特且相互促进的映射:生成映射和重建映射。布朗桥训练过程由重建映射中的保真损失和对抗训练引导,确保翻译后的图像可以准确还原为原始形式,从而实现高保真度的一致翻译。在多个数据集上的实验表明,HiFi-BBrg在多模态图像转换和多图像超分辨率方面优于现有先进技术。
Key Takeaways:
- 扩散模型在生成合成图像方面表现出优越性,但存在非确定性和对真实数据缺乏高保真度的问题。
- 论文提出了一种新型的高保真布朗桥模型(HiFi-BBrg)用于医学图像转换。
- HiFi-BBrg模型包含两个关键映射:生成映射和重建映射,这两个映射相互促进。
- 布朗桥训练过程通过重建映射中的保真损失和对抗训练来引导,确保图像翻译的准确性。
- HiFi-BBrg能够实现高保真度的一致翻译,克服现有技术的不足。
- 在多个数据集上的实验表明,HiFi-BBrg在多模态图像转换和多图像超分辨率方面优于现有技术。
点此查看论文截图
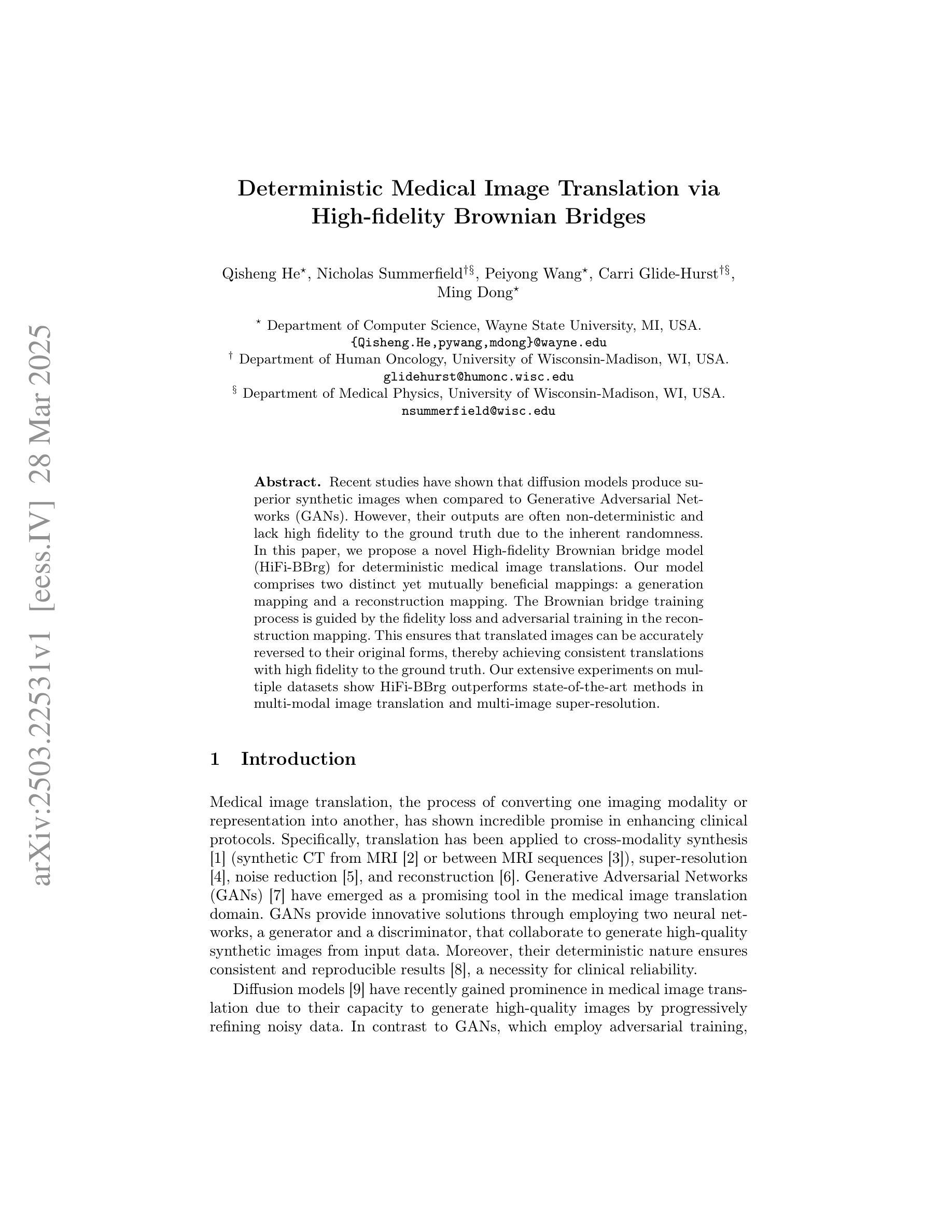
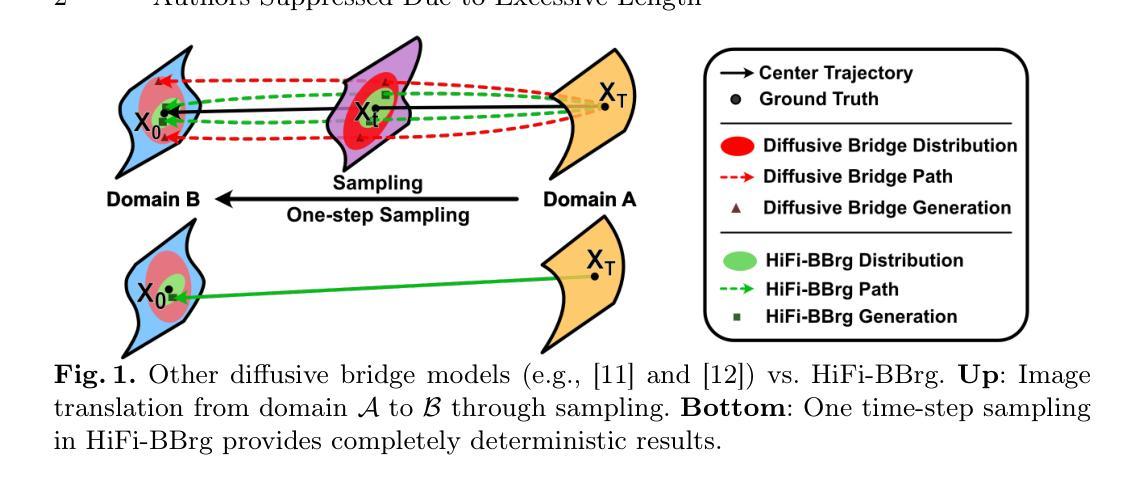
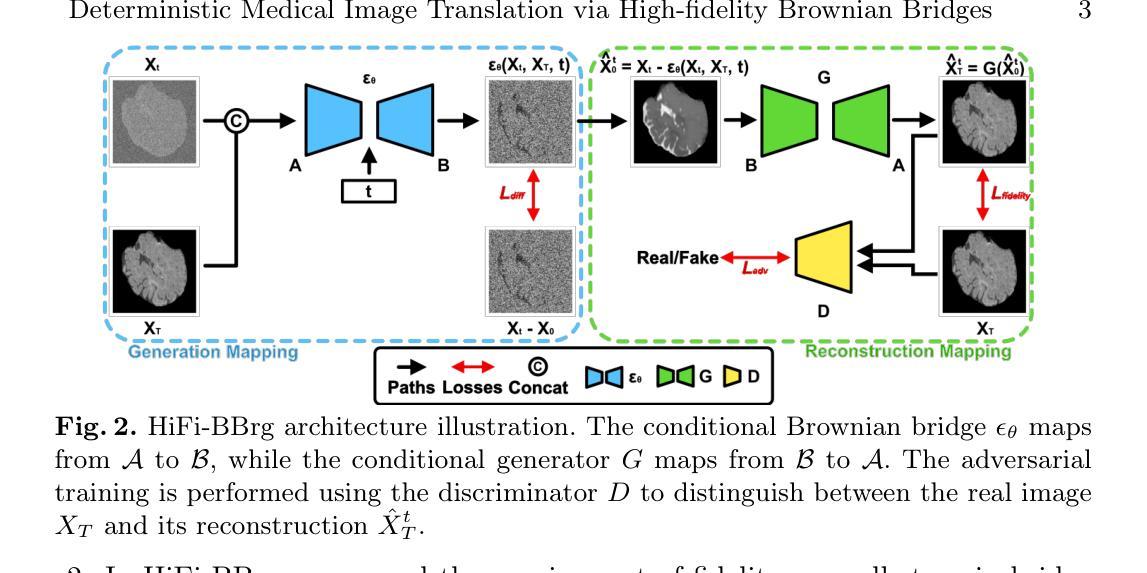
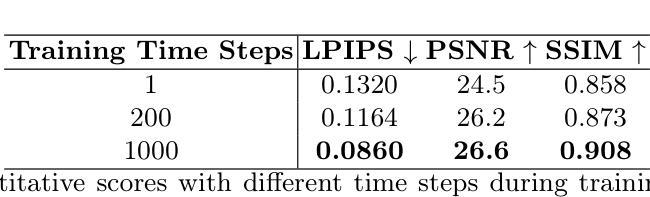
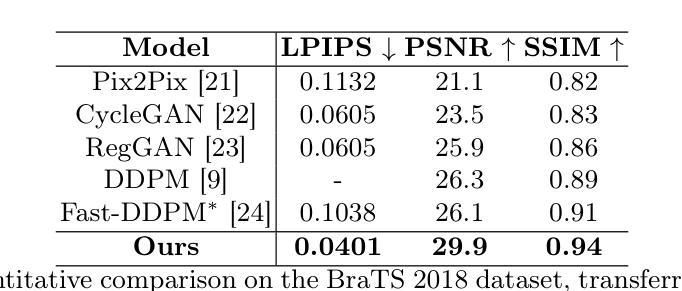
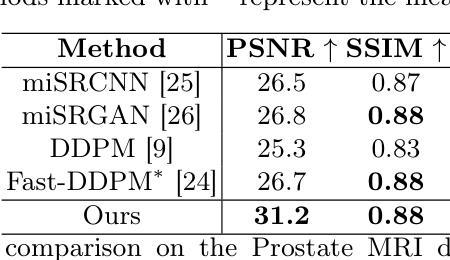
Att-Adapter: A Robust and Precise Domain-Specific Multi-Attributes T2I Diffusion Adapter via Conditional Variational Autoencoder
Authors:Wonwoong Cho, Yan-Ying Chen, Matthew Klenk, David I. Inouye, Yanxia Zhang
Text-to-Image (T2I) Diffusion Models have achieved remarkable performance in generating high quality images. However, enabling precise control of continuous attributes, especially multiple attributes simultaneously, in a new domain (e.g., numeric values like eye openness or car width) with text-only guidance remains a significant challenge. To address this, we introduce the Attribute (Att) Adapter, a novel plug-and-play module designed to enable fine-grained, multi-attributes control in pretrained diffusion models. Our approach learns a single control adapter from a set of sample images that can be unpaired and contain multiple visual attributes. The Att-Adapter leverages the decoupled cross attention module to naturally harmonize the multiple domain attributes with text conditioning. We further introduce Conditional Variational Autoencoder (CVAE) to the Att-Adapter to mitigate overfitting, matching the diverse nature of the visual world. Evaluations on two public datasets show that Att-Adapter outperforms all LoRA-based baselines in controlling continuous attributes. Additionally, our method enables a broader control range and also improves disentanglement across multiple attributes, surpassing StyleGAN-based techniques. Notably, Att-Adapter is flexible, requiring no paired synthetic data for training, and is easily scalable to multiple attributes within a single model.
文本到图像(T2I)扩散模型在生成高质量图像方面取得了显著的成绩。然而,在全新领域实现连续属性的精确控制,尤其是同时控制多个属性(例如,像眼睛睁开程度或汽车宽度这样的数值)仅通过文本指导仍然是一个巨大的挑战。为了解决这个问题,我们引入了属性(Att)适配器,这是一种新型即插即用模块,旨在在预训练的扩散模型中实现精细的多属性控制。我们的方法从一组样本图像中学习单个控制适配器,这些图像可以是未配对的,并包含多个视觉属性。Att-Adapter利用解耦交叉注意力模块,自然地协调多个域属性与文本条件。我们还将条件变分自动编码器(CVAE)引入到Att-Adapter中,以减轻过拟合问题,适应视觉世界的多样性。在两个公共数据集上的评估表明,Att-Adapter在控制连续属性方面优于所有基于LoRA的基线。此外,我们的方法扩大了控制范围,并改善了多个属性之间的解纠缠,超越了基于StyleGAN的技术。值得注意的是,Att-Adapter非常灵活,无需配对合成数据进行训练,并且很容易在单个模型内扩展到多个属性。
论文及项目相关链接
Summary
文本到图像(T2I)扩散模型已生成高质量图像方面取得了显著成效。然而,使用纯文本指导在新的领域(如眼睛睁开程度或汽车宽度等数值)实现连续属性的精确控制,尤其是同时控制多个属性,仍然是一个重大挑战。为了解决这个问题,我们引入了属性适配器(Att-Adapter),这是一种新型即插即用模块,旨在在预训练的扩散模型中实现精细粒度的多属性控制。我们的方法从一组未配对的包含多个视觉属性的样本图像中学习单个控制适配器。Att-Adapter利用解耦交叉注意力模块,自然地协调文本条件与多个域属性。我们还将条件变分自编码器(CVAE)引入到Att-Adapter中,以减轻过拟合问题,适应视觉世界的多样性。在公开数据集上的评估显示,Att-Adapter在控制连续属性方面优于所有基于LoRA的方法。此外,我们的方法扩大了控制范围,并改善了多个属性之间的解纠缠,超越了StyleGAN技术。值得注意的是,Att-Adapter非常灵活,无需配对合成数据进行训练,且可以轻松扩展到单个模型内的多个属性。
Key Takeaways
- T2I扩散模型在生成高质量图像方面表现出显著性能。
- 实现新领域中多个属性的精确控制是一大挑战。
- 属性适配器(Att-Adapter)是一种新型模块,旨在解决这一挑战,实现精细粒度的多属性控制。
- Att-Adapter能从未配对的样本图像中学习。
- 利用解耦交叉注意力模块和条件变分自编码器(CVAE)实现更自然的属性协调与适应视觉世界的多样性。
- Att-Adapter在控制连续属性方面优于基于LoRA的方法,并能扩大控制范围,改善多个属性间的解纠缠。
点此查看论文截图
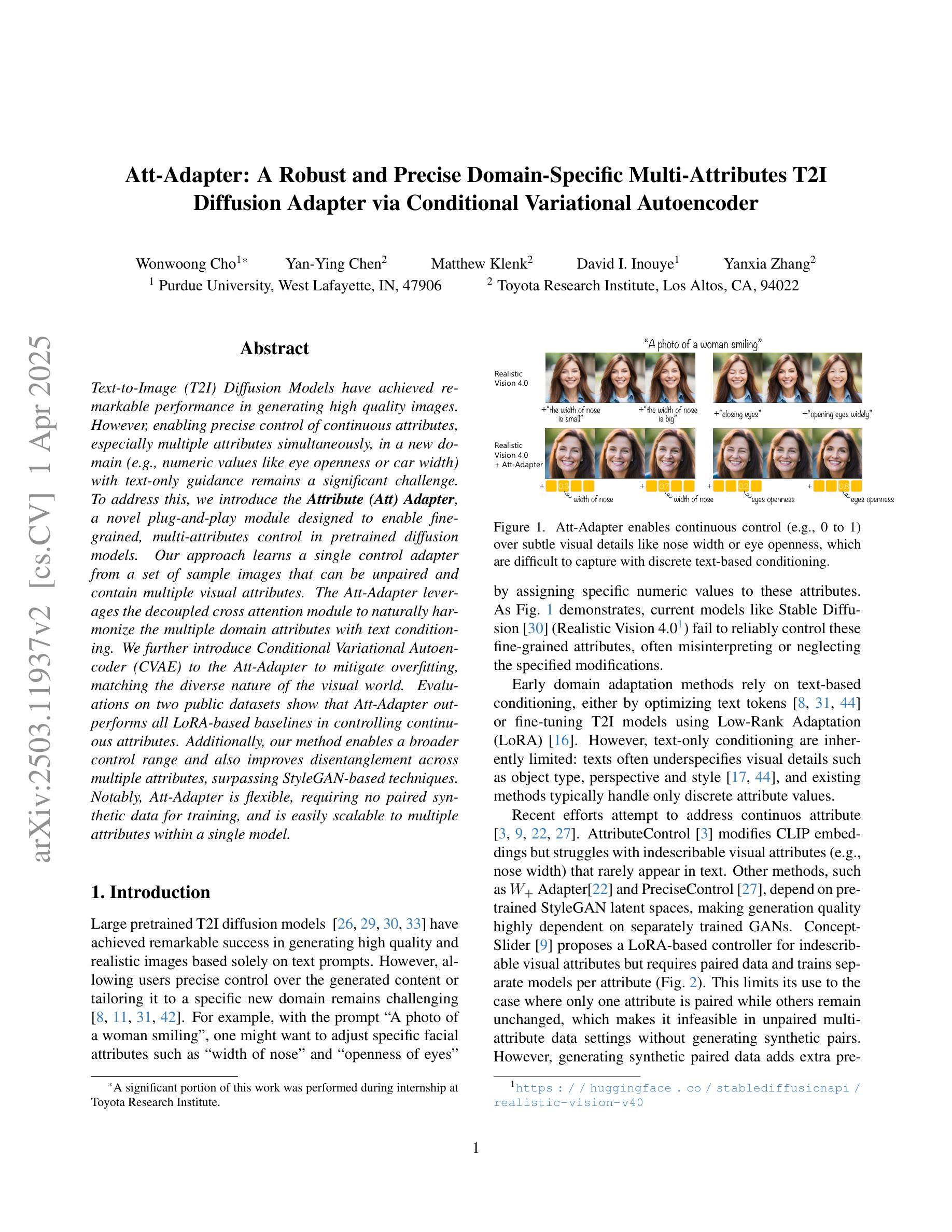
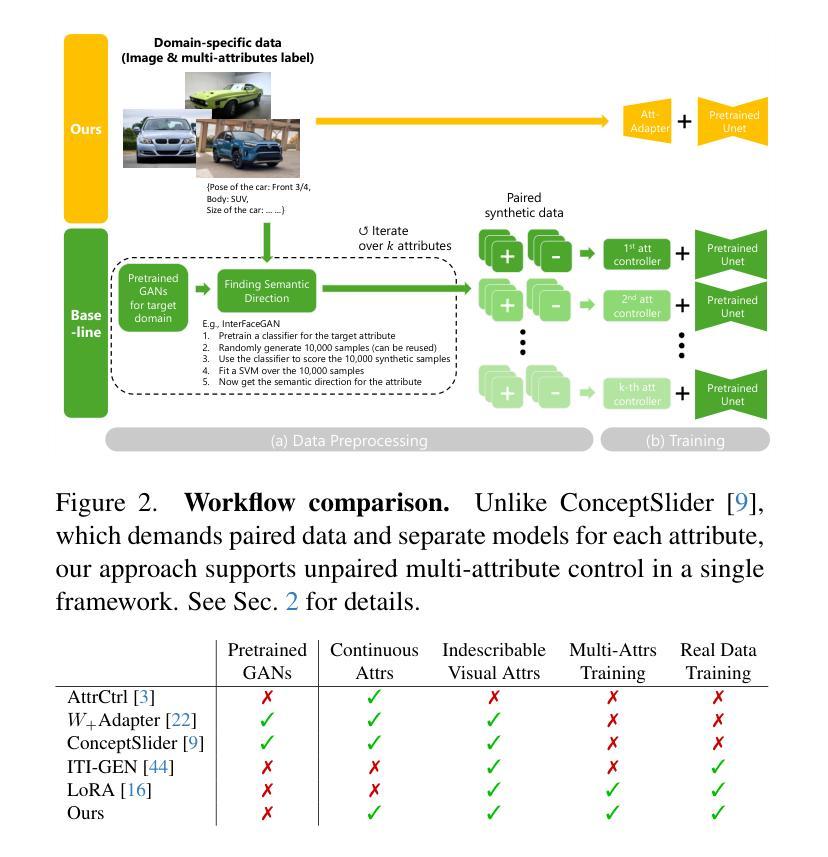
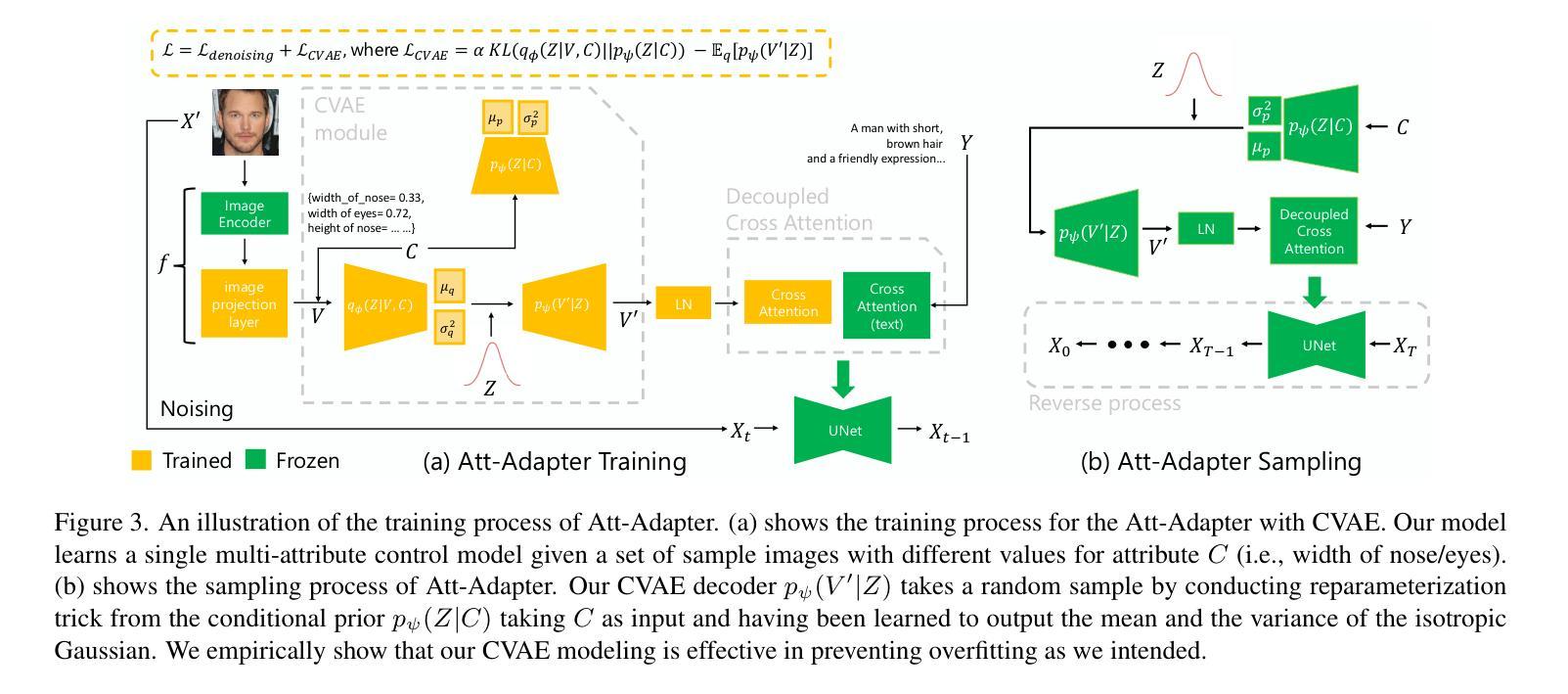
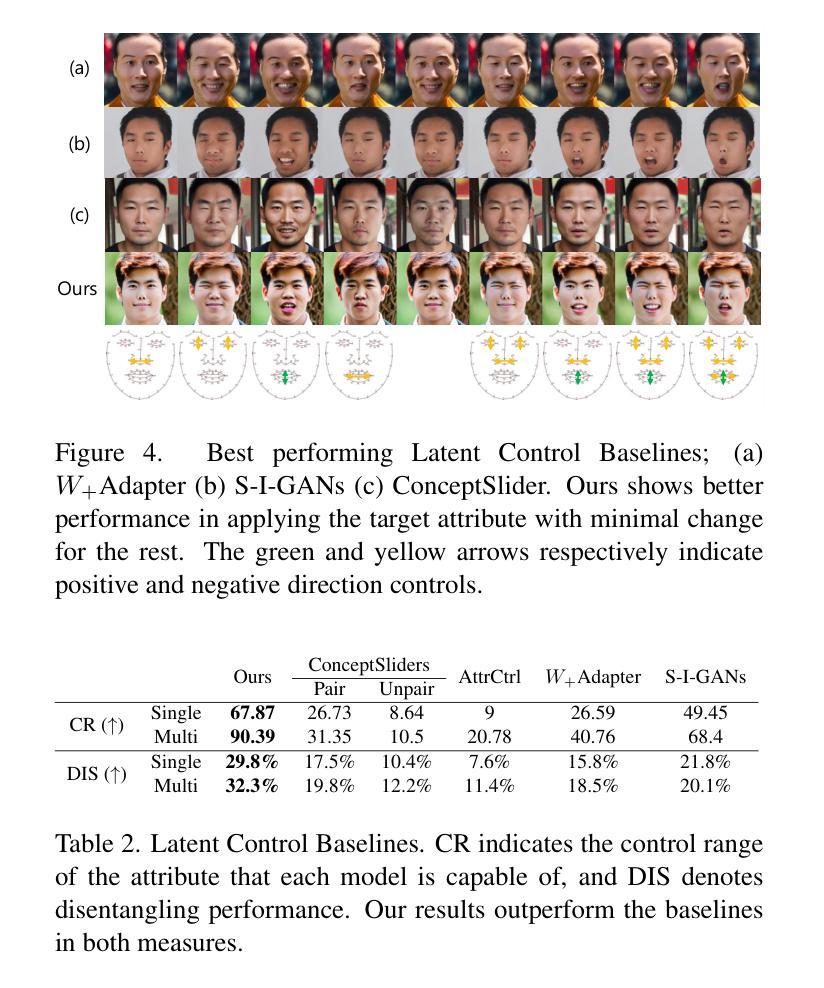
Synthetic Prior for Few-Shot Drivable Head Avatar Inversion
Authors:Wojciech Zielonka, Stephan J. Garbin, Alexandros Lattas, George Kopanas, Paulo Gotardo, Thabo Beeler, Justus Thies, Timo Bolkart
We present SynShot, a novel method for the few-shot inversion of a drivable head avatar based on a synthetic prior. We tackle three major challenges. First, training a controllable 3D generative network requires a large number of diverse sequences, for which pairs of images and high-quality tracked meshes are not always available. Second, the use of real data is strictly regulated (e.g., under the General Data Protection Regulation, which mandates frequent deletion of models and data to accommodate a situation when a participant’s consent is withdrawn). Synthetic data, free from these constraints, is an appealing alternative. Third, state-of-the-art monocular avatar models struggle to generalize to new views and expressions, lacking a strong prior and often overfitting to a specific viewpoint distribution. Inspired by machine learning models trained solely on synthetic data, we propose a method that learns a prior model from a large dataset of synthetic heads with diverse identities, expressions, and viewpoints. With few input images, SynShot fine-tunes the pretrained synthetic prior to bridge the domain gap, modeling a photorealistic head avatar that generalizes to novel expressions and viewpoints. We model the head avatar using 3D Gaussian splatting and a convolutional encoder-decoder that outputs Gaussian parameters in UV texture space. To account for the different modeling complexities over parts of the head (e.g., skin vs hair), we embed the prior with explicit control for upsampling the number of per-part primitives. Compared to SOTA monocular and GAN-based methods, SynShot significantly improves novel view and expression synthesis.
我们提出了一种名为SynShot的新方法,用于基于合成先验的少数镜头驱动头部化身倒置。我们解决了三个主要挑战。首先,训练可控的3D生成网络需要大量的不同序列,而这些序列的图像和高质量跟踪网格并不总是可用。其次,真实数据的使用受到严格监管(例如,根据《通用数据保护条例》,当参与者同意撤回时,需要频繁删除模型和数删除模型和数据据)。不受这些约束的合成数据是一个吸引人的选择。第三,最先进的单目化身模型难以推广到新的视角和表情,缺乏强大的先验知识,并且经常过度适应特定的视角分布。受仅使用合成数据训练的机器学习模型的启发,我们提出了一种方法,该方法从包含不同身份、表情和视角的大量合成头部数据中学习先验模型。凭借少数输入图像,SynShot微调了预训练的合成先验,以弥合领域之间的差距,建模一个对新型表情和视角通用的逼真头部化身。我们使用3D高斯贴图技术和卷积编码器-解码器对头部化身进行建模,该编码器-解码器输出UV纹理空间的高斯参数。为了考虑头部各部分的建模复杂性(例如皮肤和头发),我们通过嵌入先验来明确控制每个部分的原始数量。与最先进的单目和基于GAN的方法相比,SynShot在新型视角和表情合成方面有了显着提高。
论文及项目相关链接
PDF Accepted to CVPR25 Website: https://zielon.github.io/synshot/
Summary
本文提出了SynShot方法,这是一种基于合成先验的少数镜头即可驱动头部化身反演技术的新方法。该方法解决了三大挑战:缺乏多样序列图像与高质量追踪网格的训练数据、真实数据使用受限以及当前单眼化身模型在新视角和表情下的泛化能力弱。通过从大量合成头部数据中学习先验模型,SynShot只需少量输入图像即可微调预训练的合成先验,以缩小领域差距,并建立一个能泛化到新表情和视角的光照头部化身。该方法采用三维高斯贴片技术和卷积编码器-解码器建模头部化身,输出UV纹理空间的高斯参数。为了处理头部不同部分的建模复杂性(如皮肤和头发),该方法具有对每个部分原始数量的上采样进行显式控制的能力。相较于当前单眼和基于GAN的方法,SynShot极大地提升了新型视角和表情的合成效果。
Key Takeaways
一、SynShot是一种基于合成先验的少数镜头即可驱动头部化身反演技术的新方法。
二、该方法解决了训练3D生成网络所面临的三大挑战:缺乏多样序列图像与高质量追踪网格的训练数据、真实数据使用受限以及单眼化身模型在新视角和表情下的泛化难题。
三、通过从合成头部数据中学习先验模型,SynShot能够利用少量输入图像微调预训练模型,生成泛化性强的光照头部化身。
四、采用三维高斯贴片技术和卷积编码器-解码器进行头部化身建模,提升新型视角和表情的合成效果。
点此查看论文截图
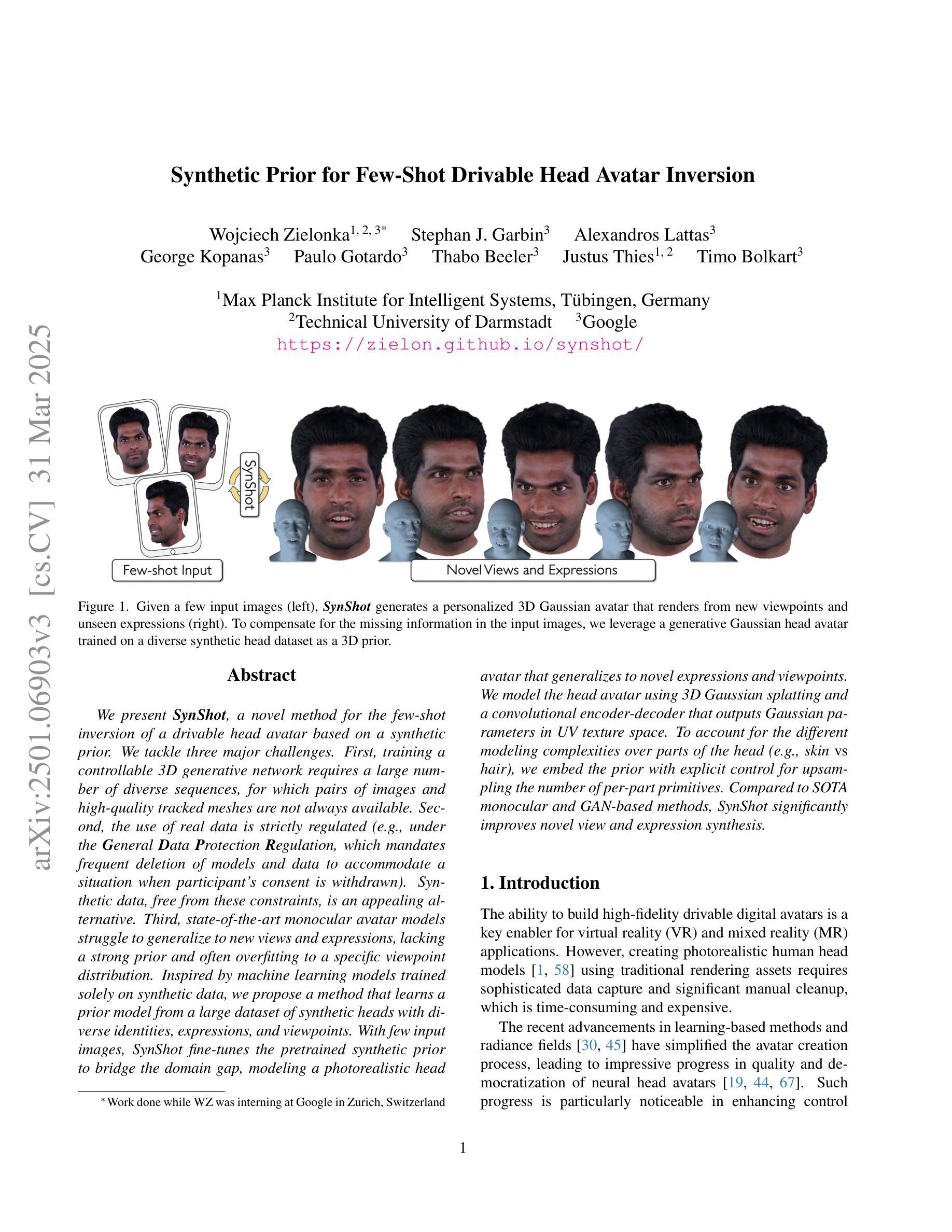
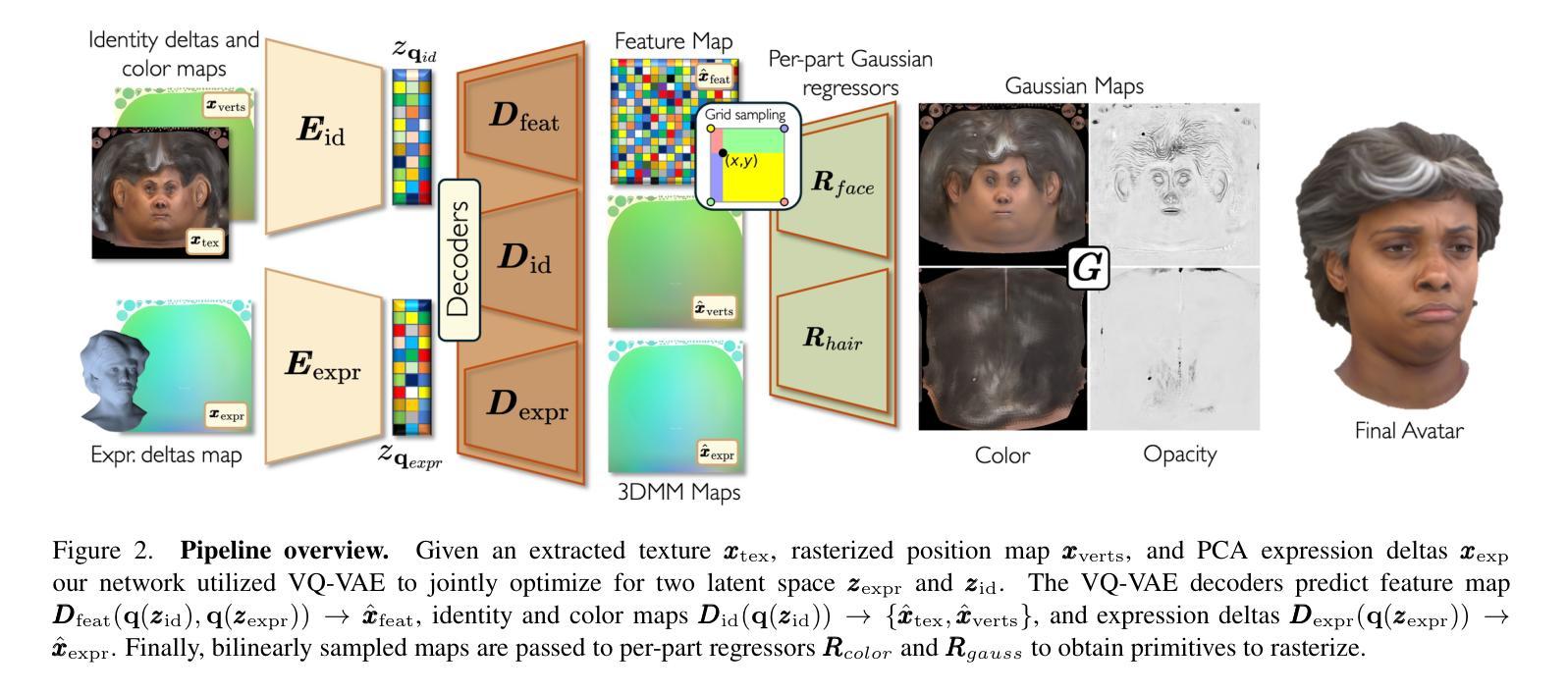
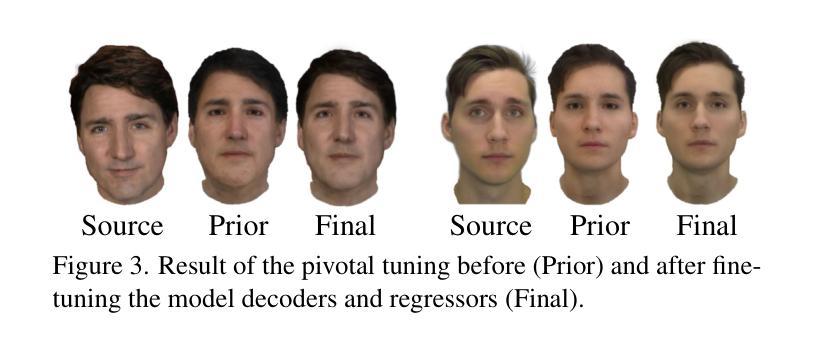
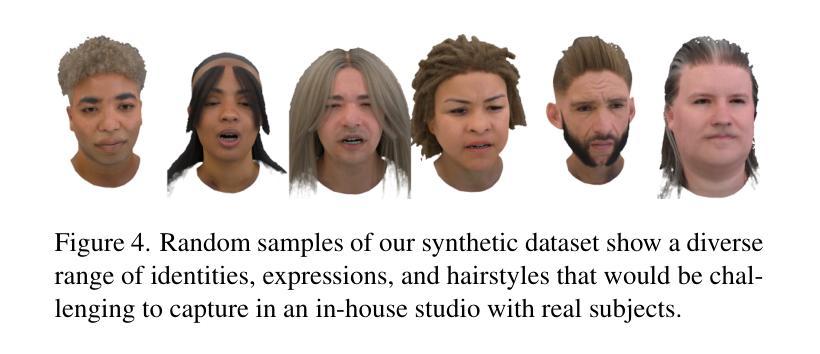
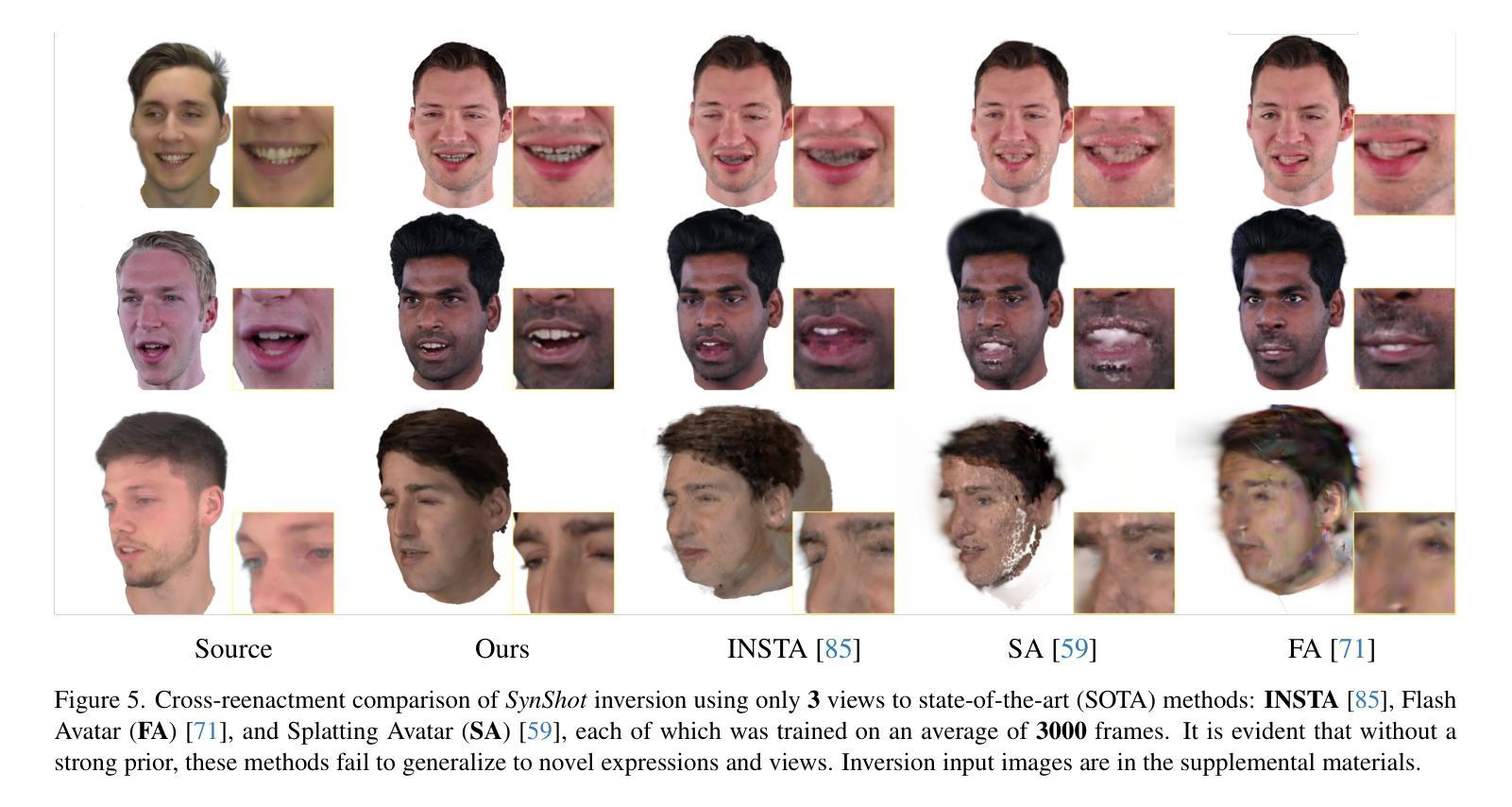
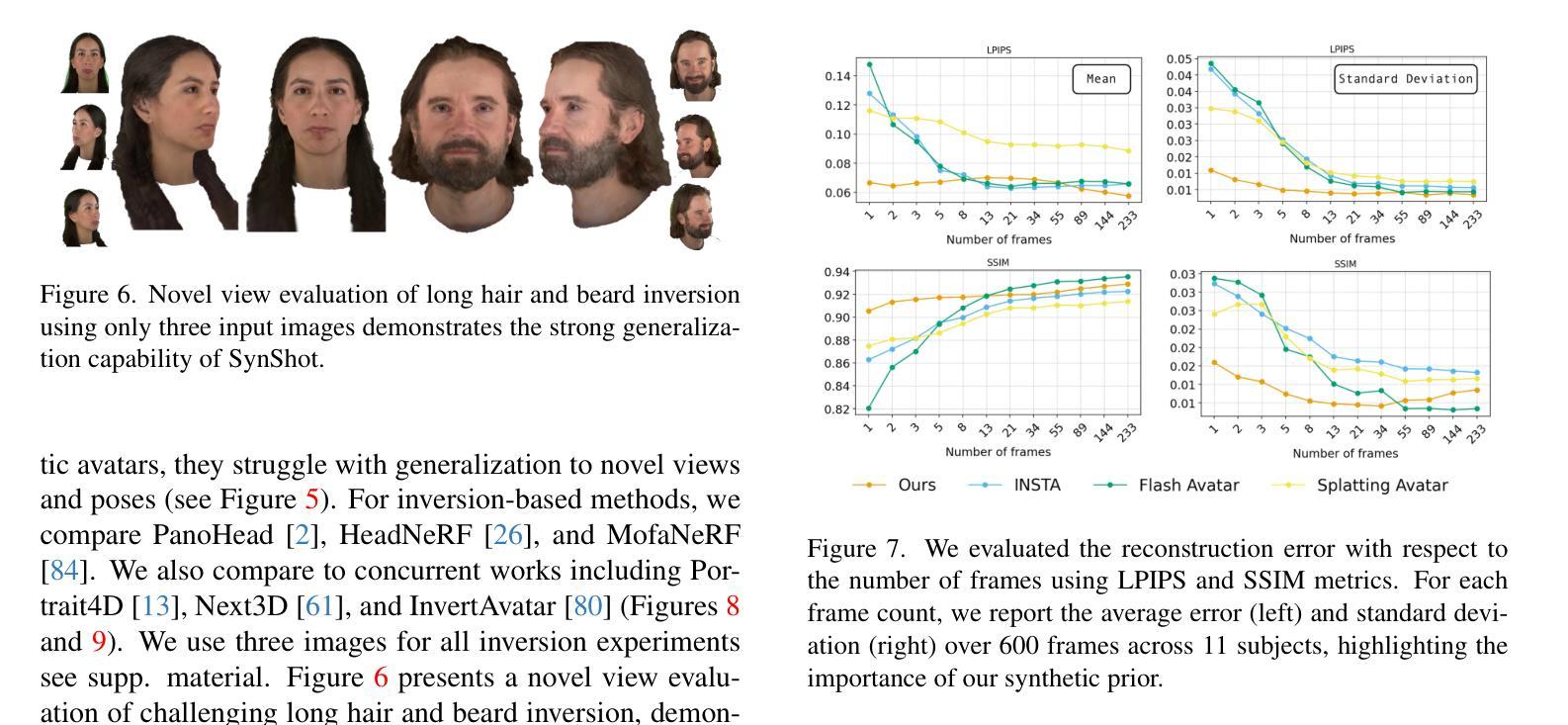
Singular Value Scaling: Efficient Generative Model Compression via Pruned Weights Refinement
Authors:Hyeonjin Kim, Jaejun Yoo
While pruning methods effectively maintain model performance without extra training costs, they often focus solely on preserving crucial connections, overlooking the impact of pruned weights on subsequent fine-tuning or distillation, leading to inefficiencies. Moreover, most compression techniques for generative models have been developed primarily for GANs, tailored to specific architectures like StyleGAN, and research into compressing Diffusion models has just begun. Even more, these methods are often applicable only to GANs or Diffusion models, highlighting the need for approaches that work across both model types. In this paper, we introduce Singular Value Scaling (SVS), a versatile technique for refining pruned weights, applicable to both model types. Our analysis reveals that pruned weights often exhibit dominant singular vectors, hindering fine-tuning efficiency and leading to suboptimal performance compared to random initialization. Our method enhances weight initialization by minimizing the disparities between singular values of pruned weights, thereby improving the fine-tuning process. This approach not only guides the compressed model toward superior solutions but also significantly speeds up fine-tuning. Extensive experiments on StyleGAN2, StyleGAN3 and DDPM demonstrate that SVS improves compression performance across model types without additional training costs. Our code is available at: https://github.com/LAIT-CVLab/Singular-Value-Scaling.
虽然剪枝方法可以有效地保持模型性能而无需额外的训练成本,但它们通常只专注于保留关键连接,而忽视了剪枝权重对后续微调或蒸馏的影响,从而导致效率低下。此外,针对生成模型的压缩技术主要是为生成对抗网络(GANs)开发的,专为特定的架构(如StyleGAN)定制,而对Diffusion模型的压缩研究才刚刚开始。而且,这些方法往往仅适用于GANs或Diffusion模型,这突显了对在这两种模型类型中都适用的方法的需求。在本文中,我们介绍了奇异值缩放(Singular Value Scaling, SVS),这是一种精炼剪枝权重的通用技术,适用于这两种模型类型。我们的分析表明,剪枝权重通常表现出主要的奇异向量,阻碍微调效率,导致与随机初始化相比性能不佳。我们的方法通过最小化剪枝权重的奇异值之间的差异来增强权重初始化,从而改进微调过程。这种方法不仅引导压缩模型达到更优的解决方案,而且还大大加快了微调速度。在StyleGAN2、StyleGAN3和DDPM上的广泛实验表明,SVS提高了各种模型的压缩性能,且无需额外的训练成本。我们的代码可在以下网址找到:https://github.com/LAIT-CVlab/Singular-Value-Scaling。
论文及项目相关链接
PDF Accepted to AAAI 2025
Summary
本文介绍了一种名为奇异值缩放(SVS)的技术,该技术旨在优化修剪权重,并适用于生成模型中的GAN和扩散模型。分析表明,修剪的权重往往表现出显著的奇异向量,影响微调效率并导致性能下降。SVS技术通过最小化修剪权重的奇异值差异,改进权重初始化,从而提高微调过程效率,引导压缩模型达到更好的解决方案,同时加快微调速度。实验证明,SVS技术在StyleGAN2、StyleGAN3和DDPM上均能提高压缩性能,且无需额外的训练成本。
Key Takeaways
- 修剪方法虽能有效维持模型性能并节省训练成本,但往往只关注保留关键连接,忽视了修剪权重对后续微调或蒸馏的影响,导致效率不高。
- 大多数生成模型的压缩技术主要针对GANs和特定的架构(如StyleGAN)开发,对扩散模型的压缩研究才刚刚开始。
- 目前的方法往往只适用于GANs或扩散模型,需要开发适用于两种模型类型的方法。
- 本文提出了奇异值缩放(SVS)技术,这是一种优化修剪权重的通用方法,适用于GANs和扩散模型。
- 分析显示,修剪的权重具有显著的奇异向量,这会影响微调效率并导致性能下降。
- SVS技术通过最小化修剪权重的奇异值差异,改进了权重的初始化,从而提高了微调过程的效率。
点此查看论文截图
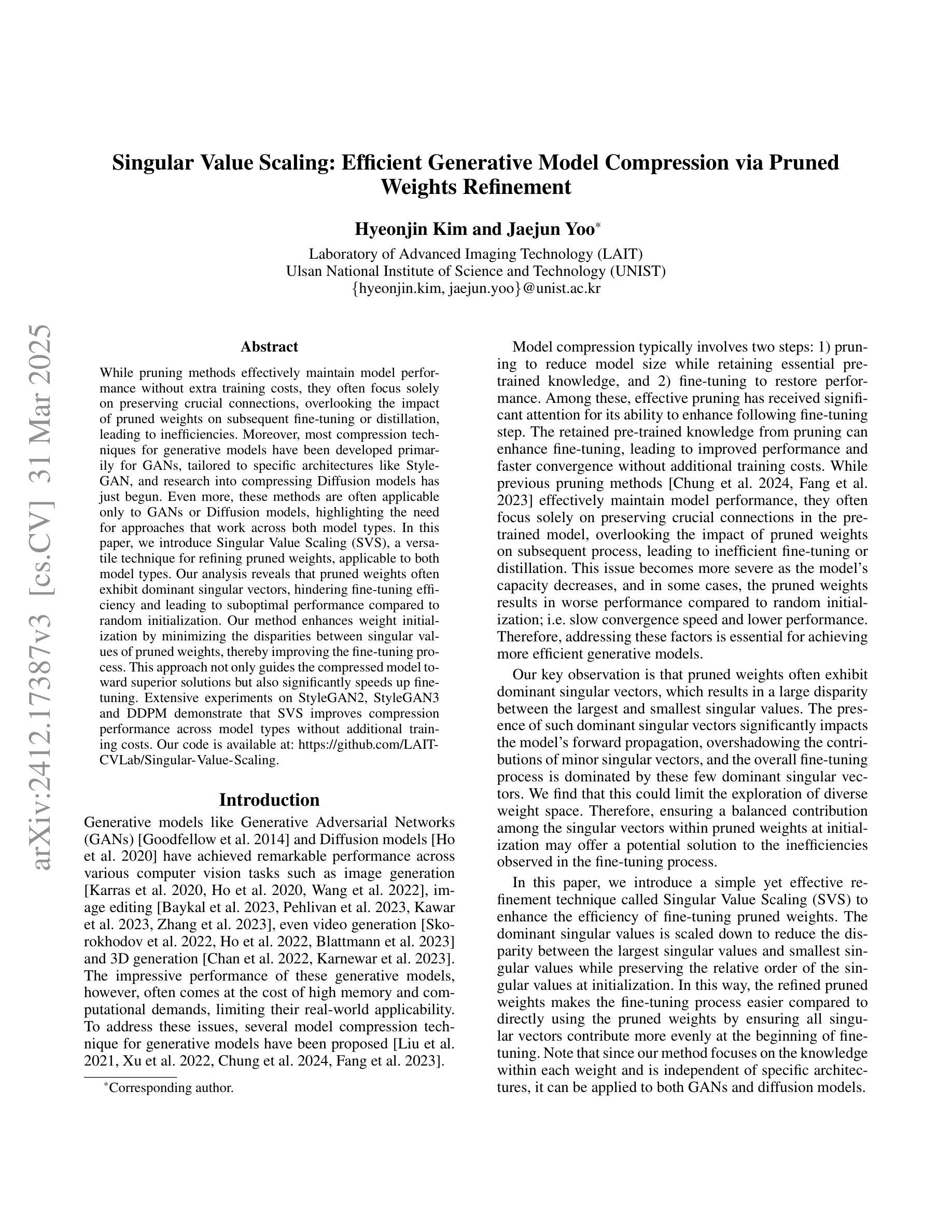
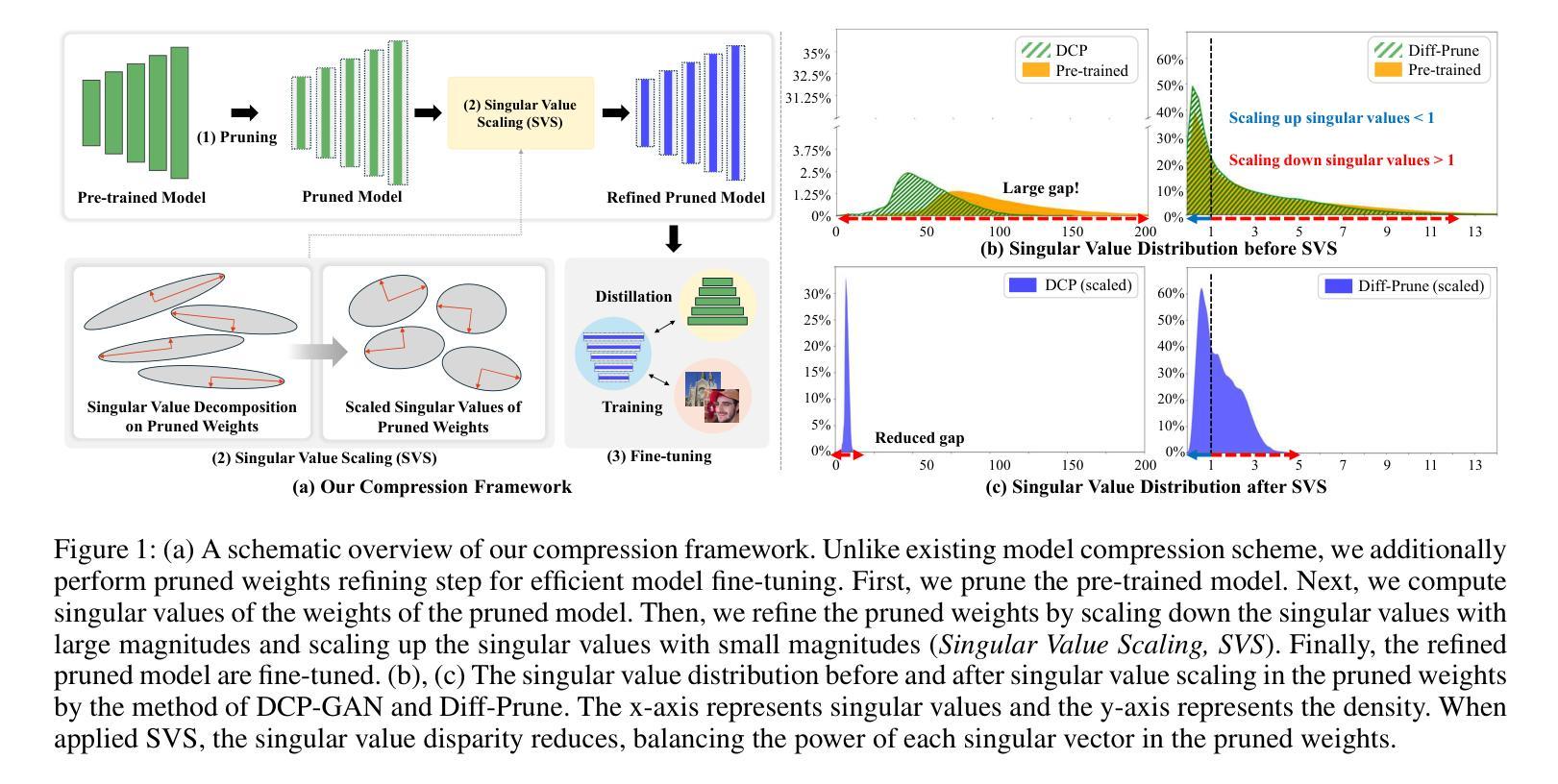
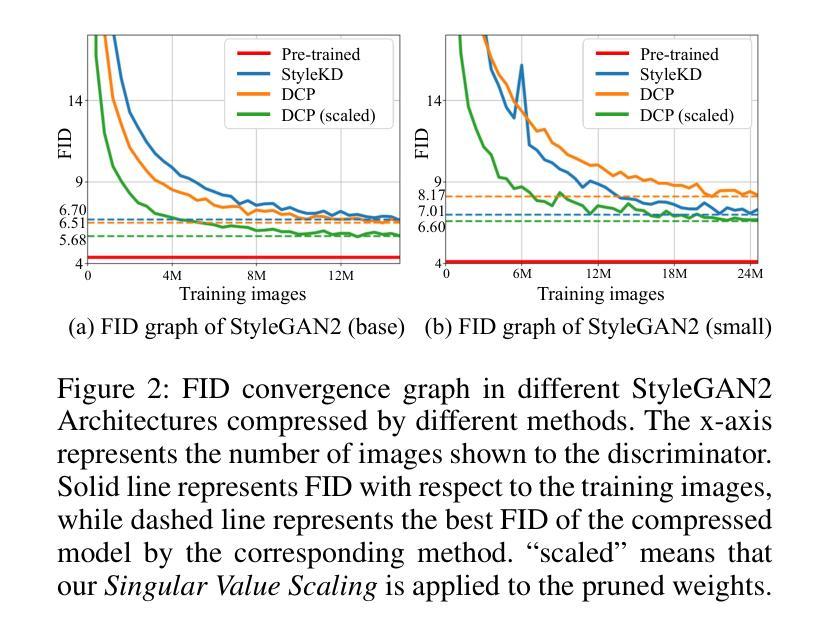
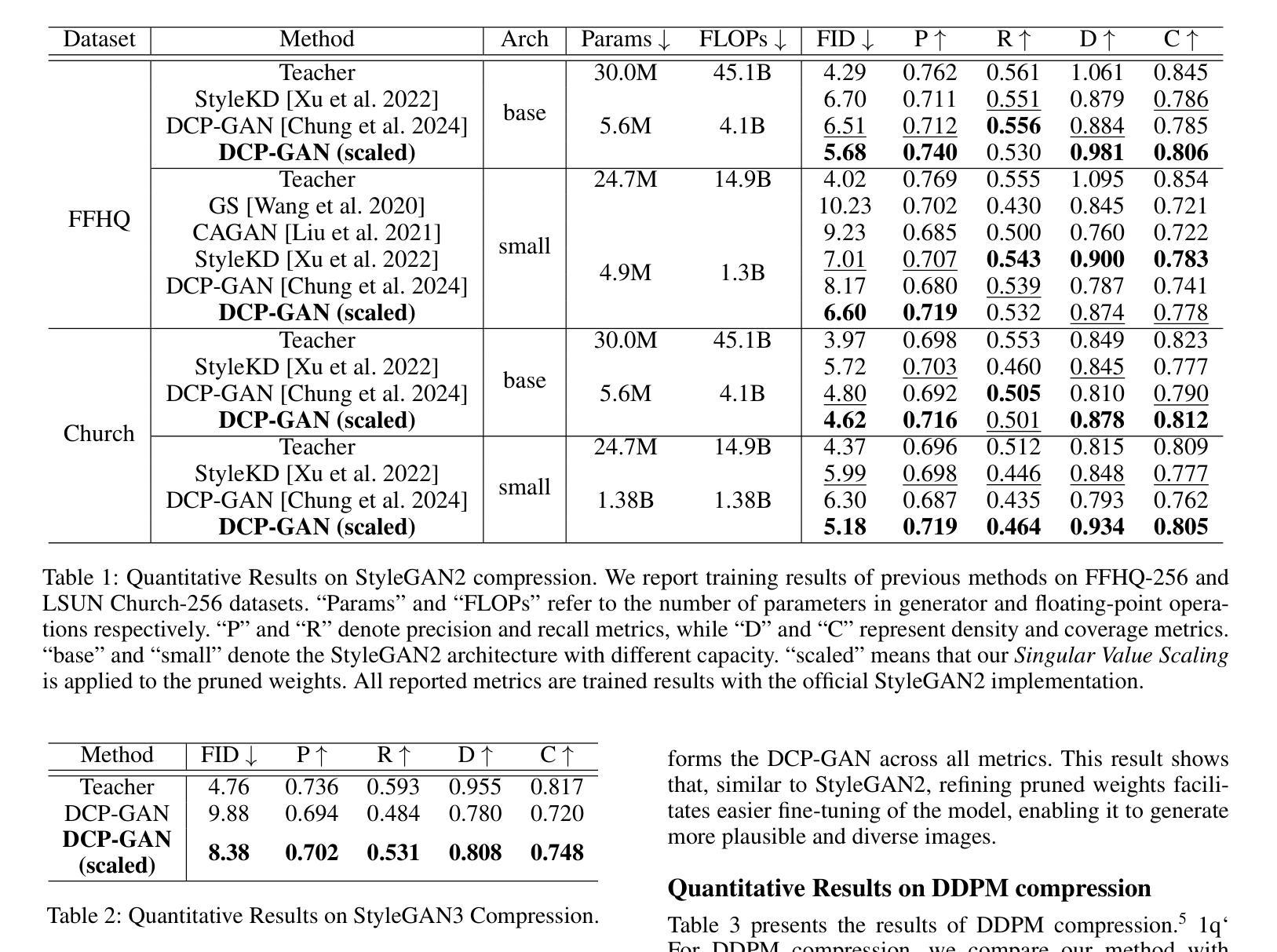
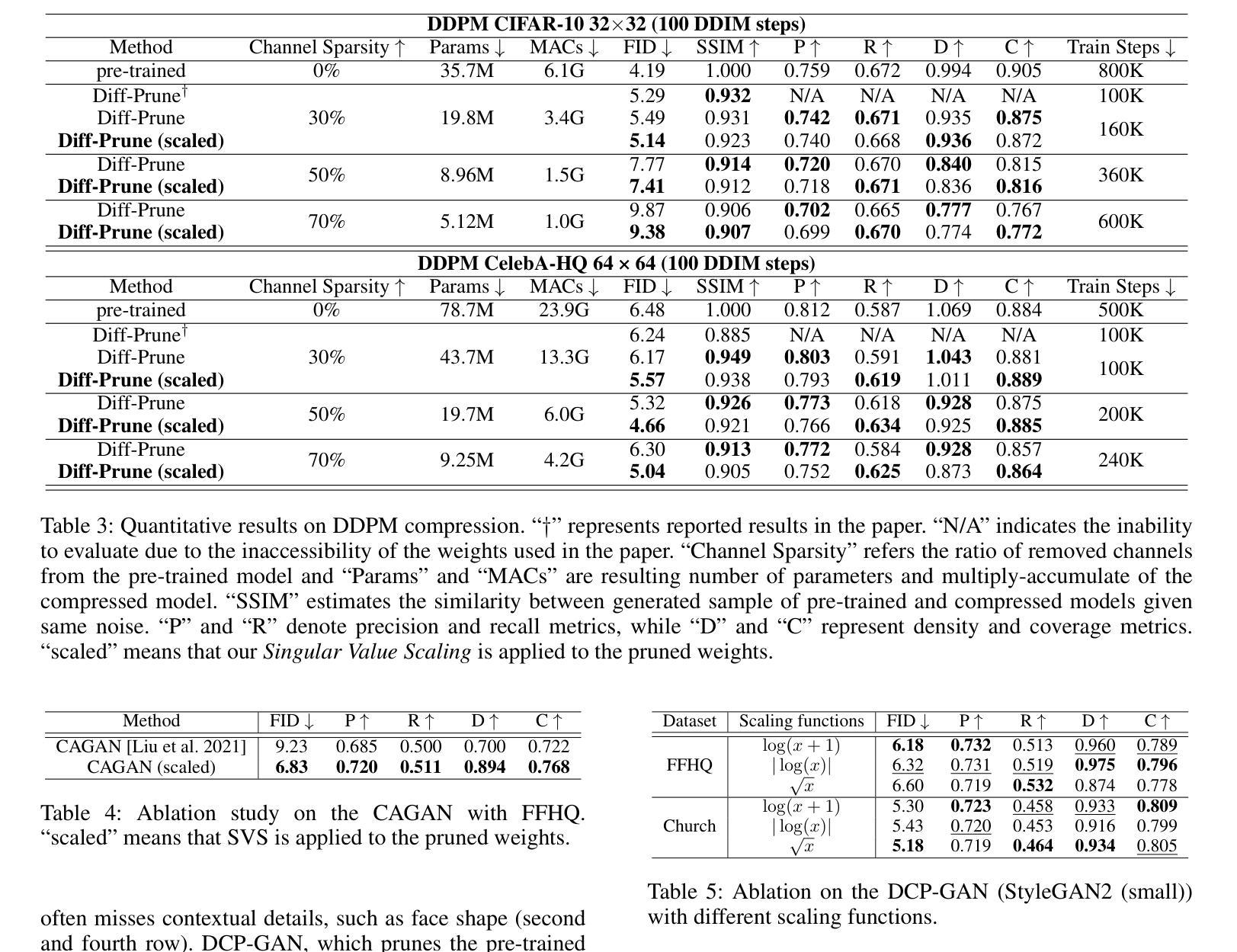