⚠️ 以下所有内容总结都来自于 大语言模型的能力,如有错误,仅供参考,谨慎使用
🔴 请注意:千万不要用于严肃的学术场景,只能用于论文阅读前的初筛!
💗 如果您觉得我们的项目对您有帮助 ChatPaperFree ,还请您给我们一些鼓励!⭐️ HuggingFace免费体验
2025-04-04 更新
Follow Your Motion: A Generic Temporal Consistency Portrait Editing Framework with Trajectory Guidance
Authors:Haijie Yang, Zhenyu Zhang, Hao Tang, Jianjun Qian, Jian Yang
Pre-trained conditional diffusion models have demonstrated remarkable potential in image editing. However, they often face challenges with temporal consistency, particularly in the talking head domain, where continuous changes in facial expressions intensify the level of difficulty. These issues stem from the independent editing of individual images and the inherent loss of temporal continuity during the editing process. In this paper, we introduce Follow Your Motion (FYM), a generic framework for maintaining temporal consistency in portrait editing. Specifically, given portrait images rendered by a pre-trained 3D Gaussian Splatting model, we first develop a diffusion model that intuitively and inherently learns motion trajectory changes at different scales and pixel coordinates, from the first frame to each subsequent frame. This approach ensures that temporally inconsistent edited avatars inherit the motion information from the rendered avatars. Secondly, to maintain fine-grained expression temporal consistency in talking head editing, we propose a dynamic re-weighted attention mechanism. This mechanism assigns higher weight coefficients to landmark points in space and dynamically updates these weights based on landmark loss, achieving more consistent and refined facial expressions. Extensive experiments demonstrate that our method outperforms existing approaches in terms of temporal consistency and can be used to optimize and compensate for temporally inconsistent outputs in a range of applications, such as text-driven editing, relighting, and various other applications.
预训练条件扩散模型在图像编辑中表现出了显著潜力。然而,它们在时间连贯性方面常常面临挑战,特别是在说话人头部领域,面部表情的连续变化增加了难度。这些问题源于单独编辑个别图像以及在编辑过程中固有的时间连续性损失。在本文中,我们介绍了Follow Your Motion(FYM),这是一个维持肖像编辑时间连贯性的通用框架。具体来说,给定由预训练3D高斯平铺模型呈现的肖像图像,我们首先开发了一种扩散模型,该模型直观地、内在地学习从第一帧到每一后续帧在不同尺度和像素坐标上的运动轨迹变化。这种方法确保了时间上不一致的编辑化身继承了呈现化身的运动信息。其次,为了保持说话头部编辑中细微表情的时间连贯性,我们提出了一种动态加权注意力机制。该机制在空间地标点上分配较高的权重系数,并基于地标损失动态更新这些权重,实现更一致和精细的面部表情。大量实验表明,我们的方法在时间连贯性方面优于现有方法,并可用于优化和补偿各种应用中时间上不一致的输出,如文本驱动编辑、重新照明和其他各种应用。
论文及项目相关链接
PDF https://anonymous-hub1127.github.io/FYM.github.io/
Summary:
预训练条件扩散模型在图像编辑中展现出巨大潜力,但在动态头像等场景中存在时序不一致性问题。本文提出一种名为Follow Your Motion(FYM)的通用框架,用于在肖像编辑中保持时序一致性。通过利用预训练的3D高斯喷溅模型和扩散模型,FYM能够直觉地、内在地学习不同尺度和像素坐标的运动轨迹变化。同时,引入动态加权注意力机制,以维持精细的表情时序一致性。实验表明,该方法在时序一致性方面优于现有方法,并可应用于文本驱动编辑、重新照明等多种应用。
Key Takeaways:
- 预训练条件扩散模型在图像编辑中具有显著潜力,但在动态头像编辑中存在时序不一致性问题。
- Follow Your Motion(FYM)框架被提出以解决这一问题,通过维护肖像编辑中的时序一致性。
- FYM利用预训练的3D高斯喷溅模型和扩散模型来学习运动轨迹变化。
- 引入动态加权注意力机制以维持精细的表情时序一致性。
- FYM在时序一致性方面表现优越,优于现有方法。
- FYM可应用于多种应用,如文本驱动编辑、重新照明等。
点此查看论文截图
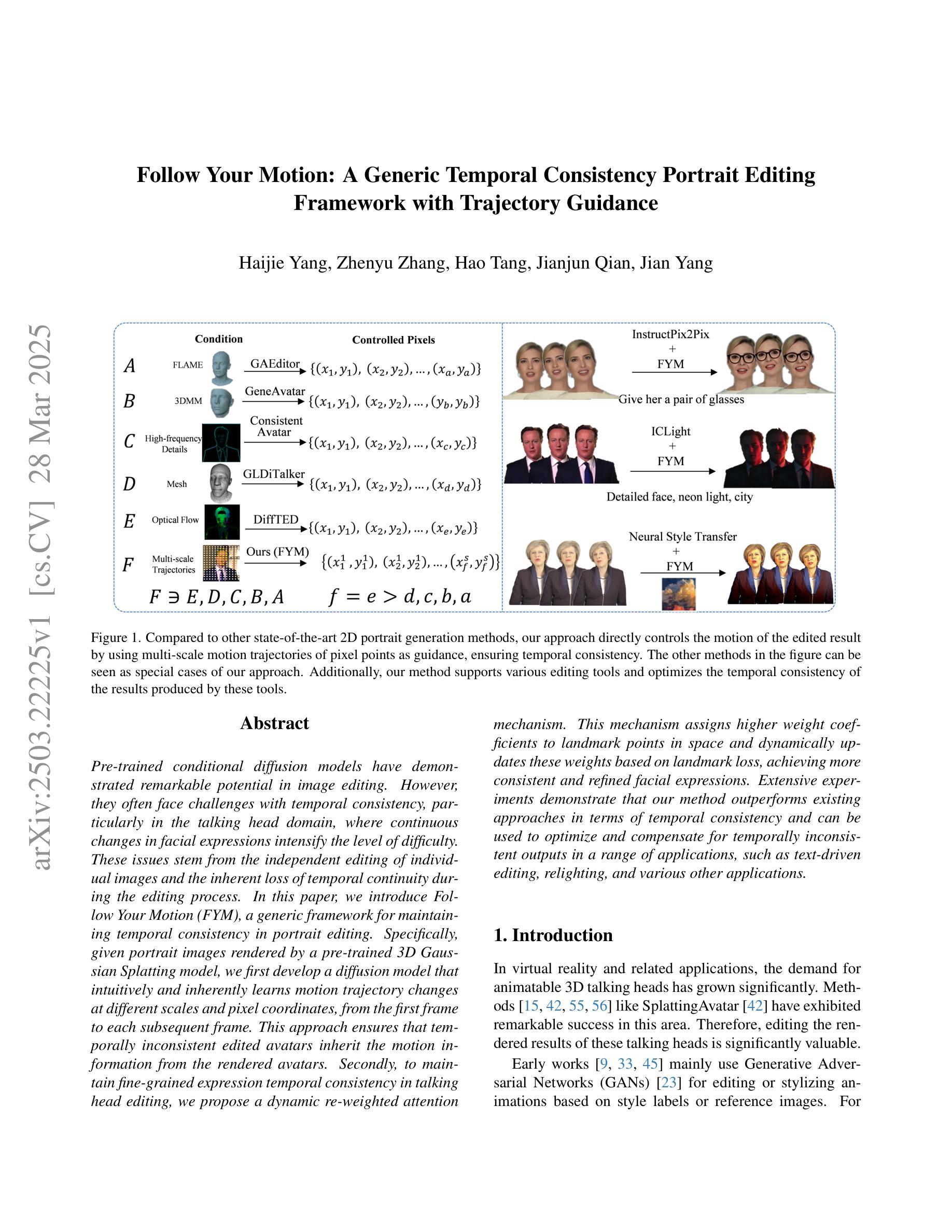
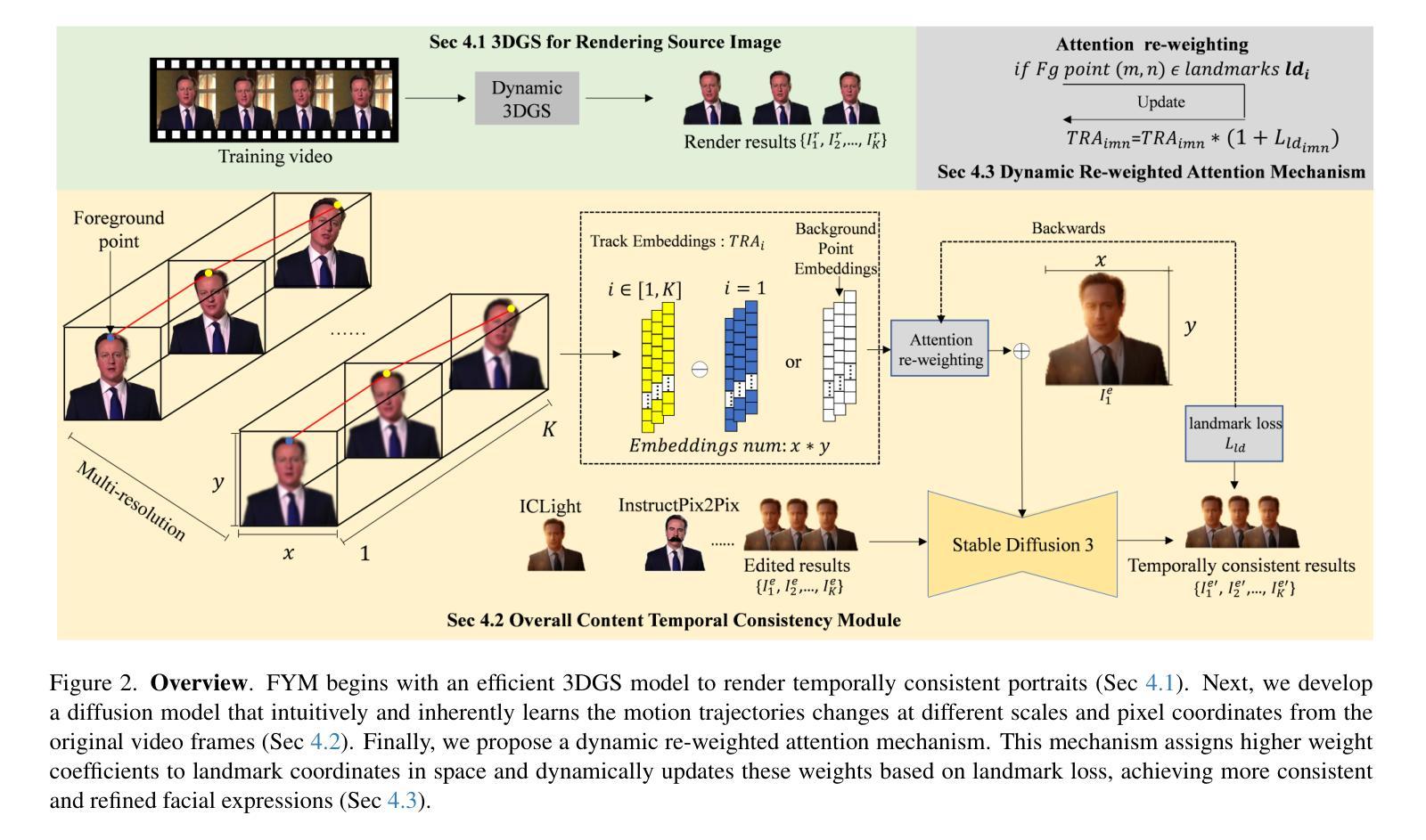
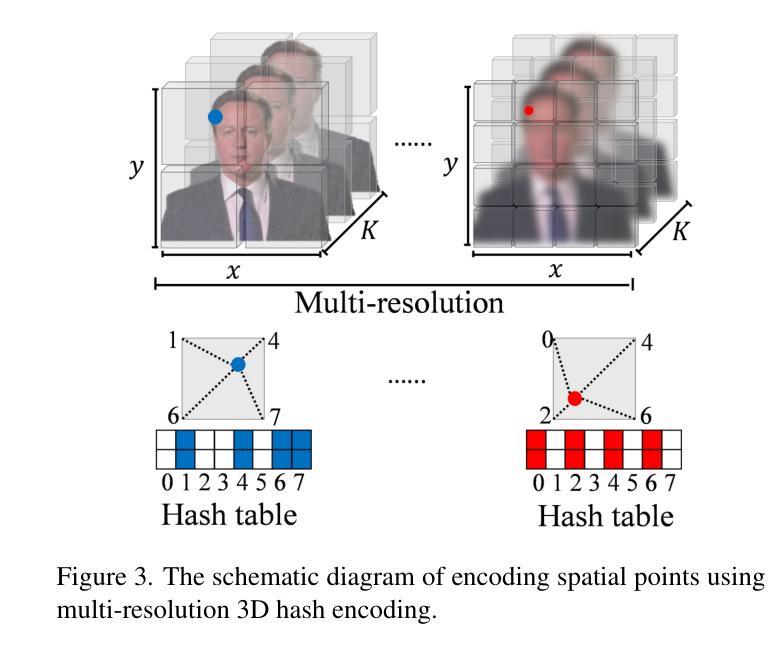
Audio-driven Gesture Generation via Deviation Feature in the Latent Space
Authors:Jiahui Chen, Yang Huan, Runhua Shi, Chanfan Ding, Xiaoqi Mo, Siyu Xiong, Yinong He
Gestures are essential for enhancing co-speech communication, offering visual emphasis and complementing verbal interactions. While prior work has concentrated on point-level motion or fully supervised data-driven methods, we focus on co-speech gestures, advocating for weakly supervised learning and pixel-level motion deviations. We introduce a weakly supervised framework that learns latent representation deviations, tailored for co-speech gesture video generation. Our approach employs a diffusion model to integrate latent motion features, enabling more precise and nuanced gesture representation. By leveraging weakly supervised deviations in latent space, we effectively generate hand gestures and mouth movements, crucial for realistic video production. Experiments show our method significantly improves video quality, surpassing current state-of-the-art techniques.
手势对于增强共语沟通、提供视觉重点和补充言语互动至关重要。虽然之前的工作主要集中在点级运动或完全监督的数据驱动方法上,但我们关注共语手势,提倡弱监督学习和像素级运动偏差。我们引入了一个弱监督框架,学习潜在表示偏差,专为共语手势视频生成而设计。我们的方法采用扩散模型来整合潜在运动特征,实现更精确和细微的手势表示。通过利用潜在空间中的弱监督偏差,我们可以有效地生成手势和口部动作,对于真实视频制作至关重要。实验表明,我们的方法显著提高了视频质量,超越了当前最先进的技术。
论文及项目相关链接
PDF 6 pages, 5 figures
Summary
本文探讨了点级动作的传统研究焦点是口语辅助手势,多采用全监督数据驱动方法,而本文提出利用弱监督学习和像素级运动偏差的框架来增强对口语手势的捕捉。引入的弱监督框架学习潜在表示偏差,适用于口语手势视频生成。通过扩散模型整合潜在运动特征,实现更精确的手势表达。实验证明,该方法显著提高了视频质量。
Key Takeaways
- 手势在协同口语交流中起到关键作用,提供视觉强调并补充口头互动。
- 现有研究多关注点级运动或全监督数据驱动的方法,本文侧重于口语手势的研究。
- 提出使用弱监督学习和像素级运动偏差的方法来处理口语手势视频生成。
- 引入的框架可以学习潜在表示的偏差,这有助于提高手势和口型动作的准确性。
- 通过扩散模型整合潜在运动特征,增强手势表达的精确性和细腻性。
- 利用弱监督的潜在空间偏差生成手部和口部动作,对于生成逼真的视频至关重要。
点此查看论文截图
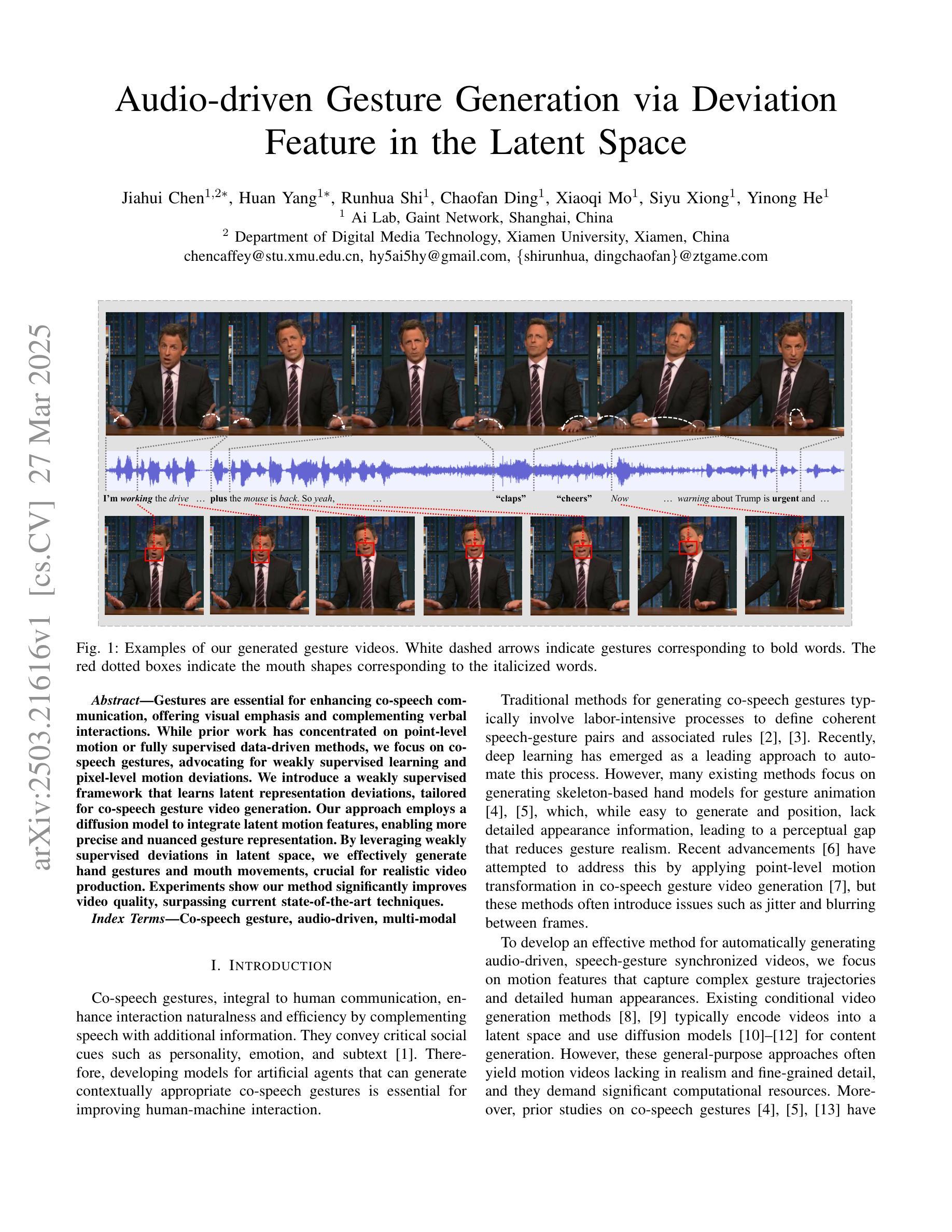
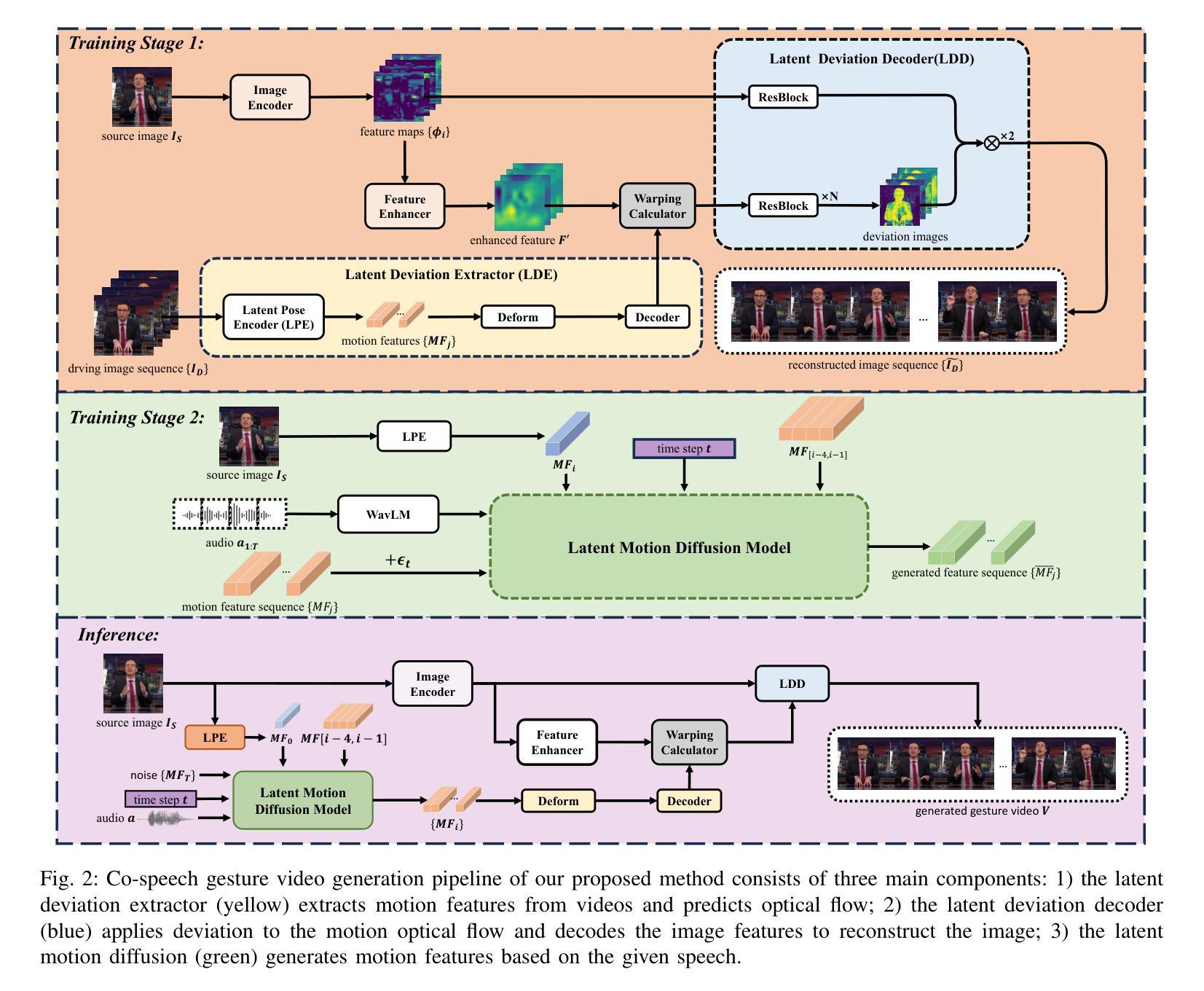
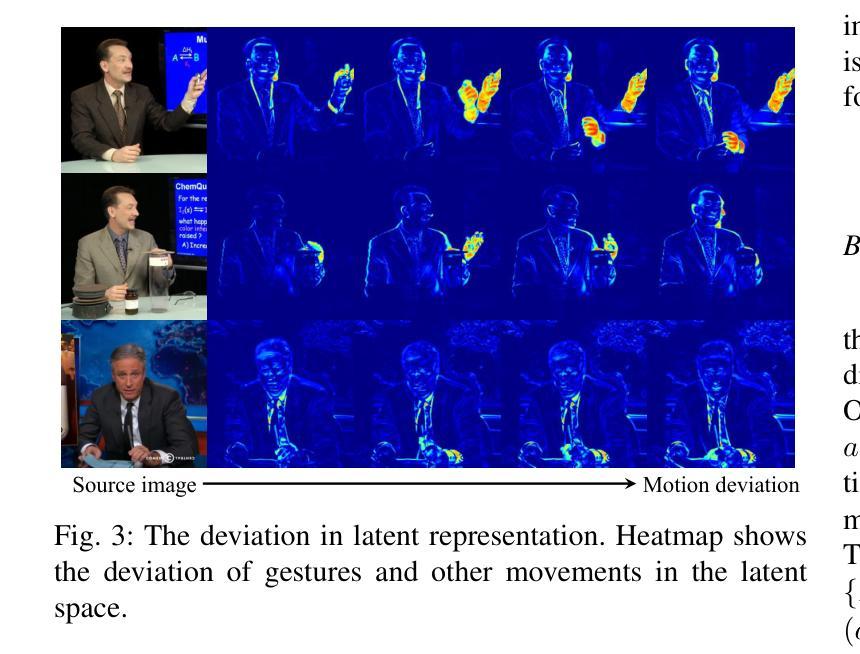
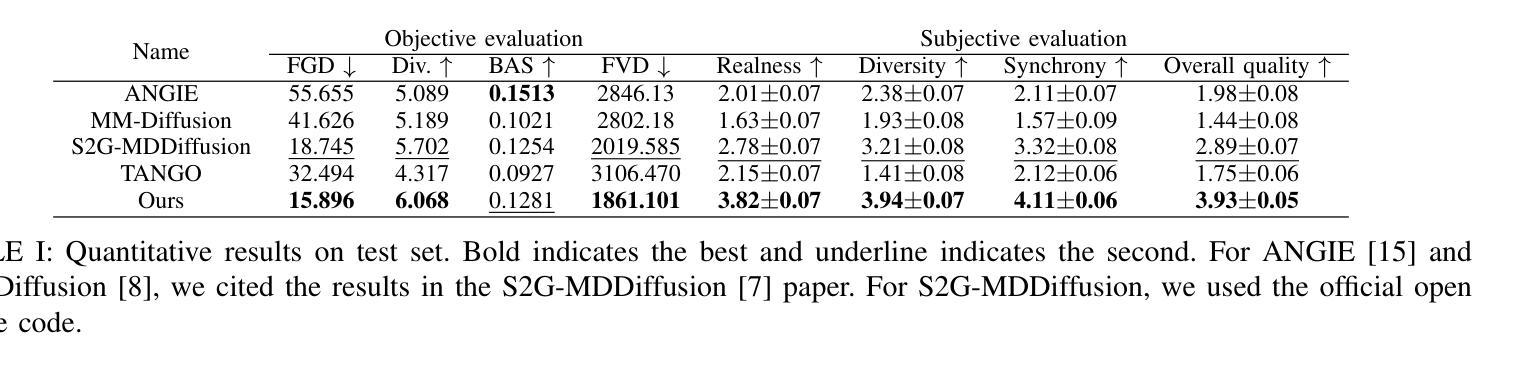
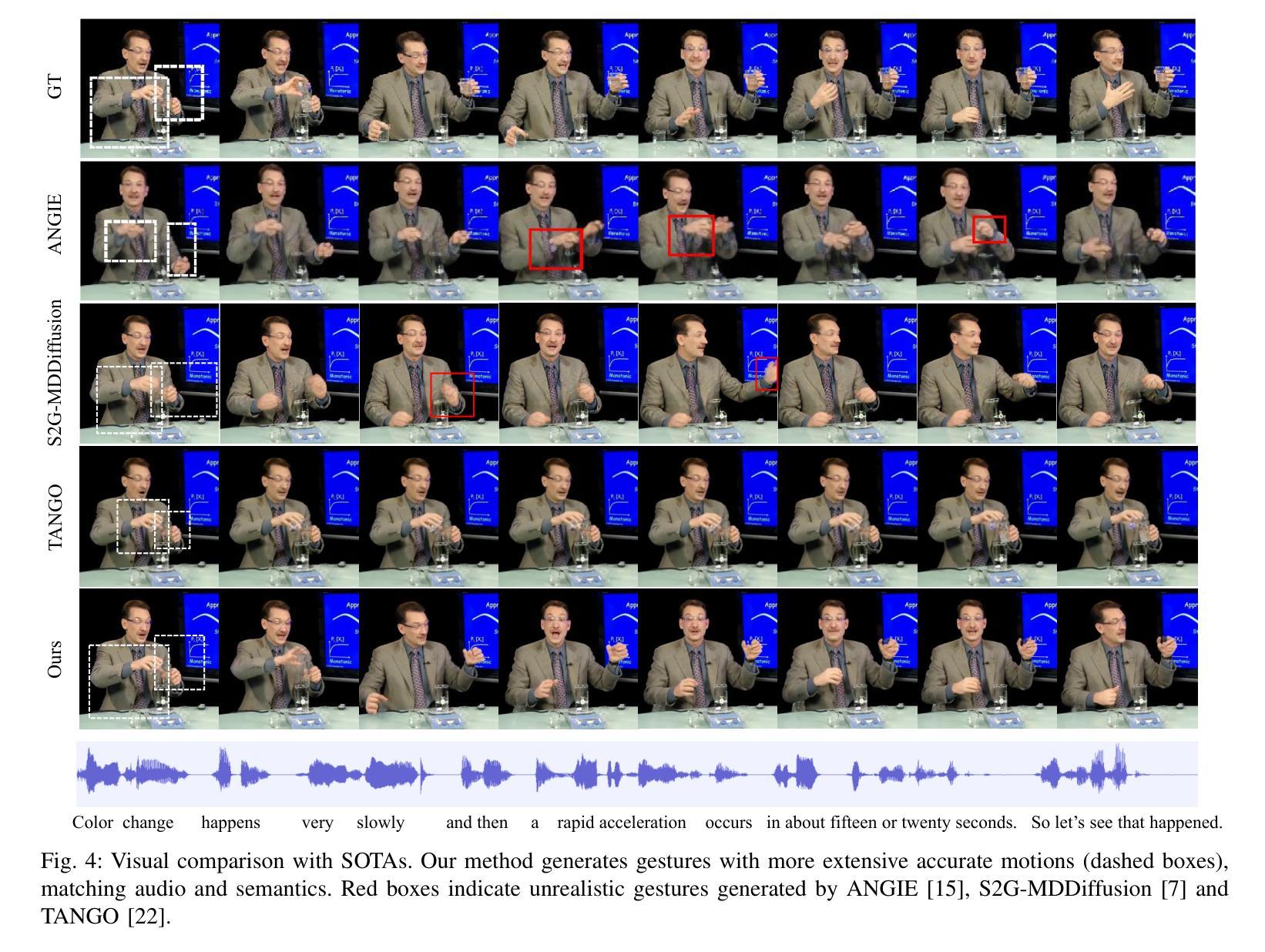
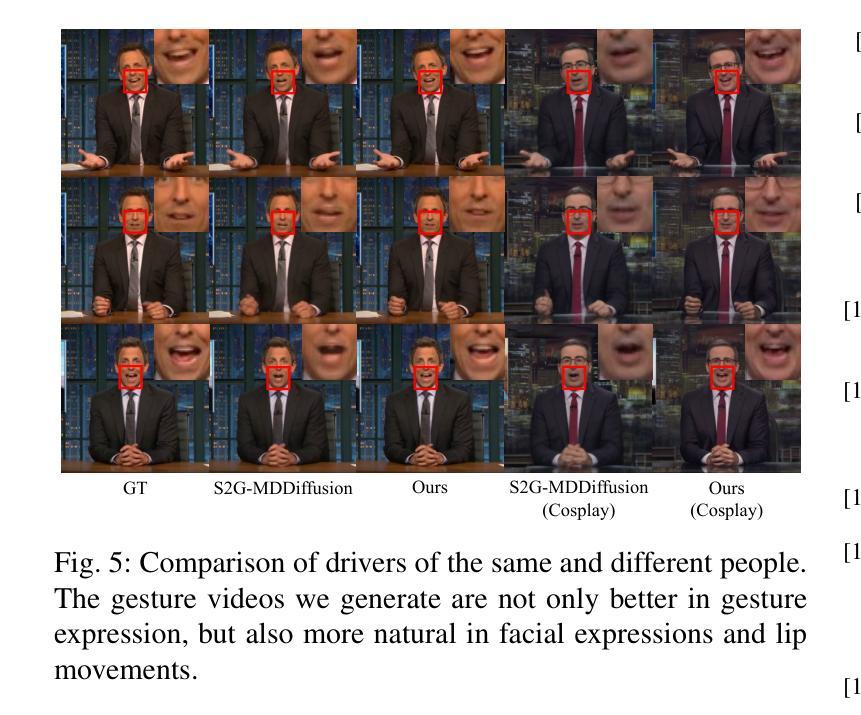

Perceptually Accurate 3D Talking Head Generation: New Definitions, Speech-Mesh Representation, and Evaluation Metrics
Authors:Lee Chae-Yeon, Oh Hyun-Bin, Han EunGi, Kim Sung-Bin, Suekyeong Nam, Tae-Hyun Oh
Recent advancements in speech-driven 3D talking head generation have made significant progress in lip synchronization. However, existing models still struggle to capture the perceptual alignment between varying speech characteristics and corresponding lip movements. In this work, we claim that three criteria – Temporal Synchronization, Lip Readability, and Expressiveness – are crucial for achieving perceptually accurate lip movements. Motivated by our hypothesis that a desirable representation space exists to meet these three criteria, we introduce a speech-mesh synchronized representation that captures intricate correspondences between speech signals and 3D face meshes. We found that our learned representation exhibits desirable characteristics, and we plug it into existing models as a perceptual loss to better align lip movements to the given speech. In addition, we utilize this representation as a perceptual metric and introduce two other physically grounded lip synchronization metrics to assess how well the generated 3D talking heads align with these three criteria. Experiments show that training 3D talking head generation models with our perceptual loss significantly improve all three aspects of perceptually accurate lip synchronization. Codes and datasets are available at https://perceptual-3d-talking-head.github.io/.
近期语音驱动的3D说话人头部生成技术的进展在嘴唇同步方面取得了重大突破。然而,现有模型仍然难以捕捉不同语音特征与相应嘴唇运动之间的感知对齐。在这项工作中,我们认为三个标准——时间同步、嘴唇可读性和表现力——对于实现感知上准确的嘴唇运动至关重要。受存在一个合适的表示空间来满足这三个标准的假设的启发,我们引入了一种语音网格同步表示,该表示捕捉了语音信号和3D面部网格之间的精细对应关系。我们发现我们学到的表示具有理想的特性,我们将其插入现有模型作为感知损失,以更好地将嘴唇运动与给定语音对齐。此外,我们利用这种表示作为感知度量,并引入其他两个基于物理的嘴唇同步度量来评估生成的3D说话人的头部与这三个标准的对齐程度。实验表明,使用我们的感知损失训练3D说话人头部生成模型,可以显著提高感知嘴唇同步的三个方面的准确性。相关代码和数据集可在https://perceptual-3d-talking-head.github.io/获得。
论文及项目相关链接
PDF CVPR 2025. Project page: https://perceptual-3d-talking-head.github.io/
Summary
本文探讨了近期语音驱动的三维谈话头生成技术的进展,特别是在唇同步方面的成果。文章指出,要实现感知准确的唇动,需满足三个标准:时间同步、唇可读性和表达力。为此,文章引入了一种语音网格同步表示法,能够捕捉语音信号和三维面部网格之间的精细对应关系。通过将此表示法插入现有模型中作为感知损失,可以更好地对齐唇动与语音。此外,文章还利用此表示法作为感知指标,并引入另外两个基于物理的唇同步指标,以评估生成的三维谈话头是否满足这三个标准。实验表明,使用本文的感知损失训练三维谈话头生成模型,可显著提高唇同步的感知准确性。
Key Takeaways
- 近期语音驱动的三维谈话头生成技术在唇同步方面取得显著进展。
- 三个标准对实现感知准确的唇动至关重要:时间同步、唇可读性和表达力。
- 引入了一种语音网格同步表示法,以捕捉语音信号和三维面部网格之间的对应关系。
- 通过将感知损失插入现有模型中,可更好地对齐唇动与语音。
- 利用感知指标和其他两个基于物理的唇同步指标来评估三维谈话头的质量。
- 使用感知损失训练的模型在唇同步的感知准确性上表现出显著提高。
点此查看论文截图
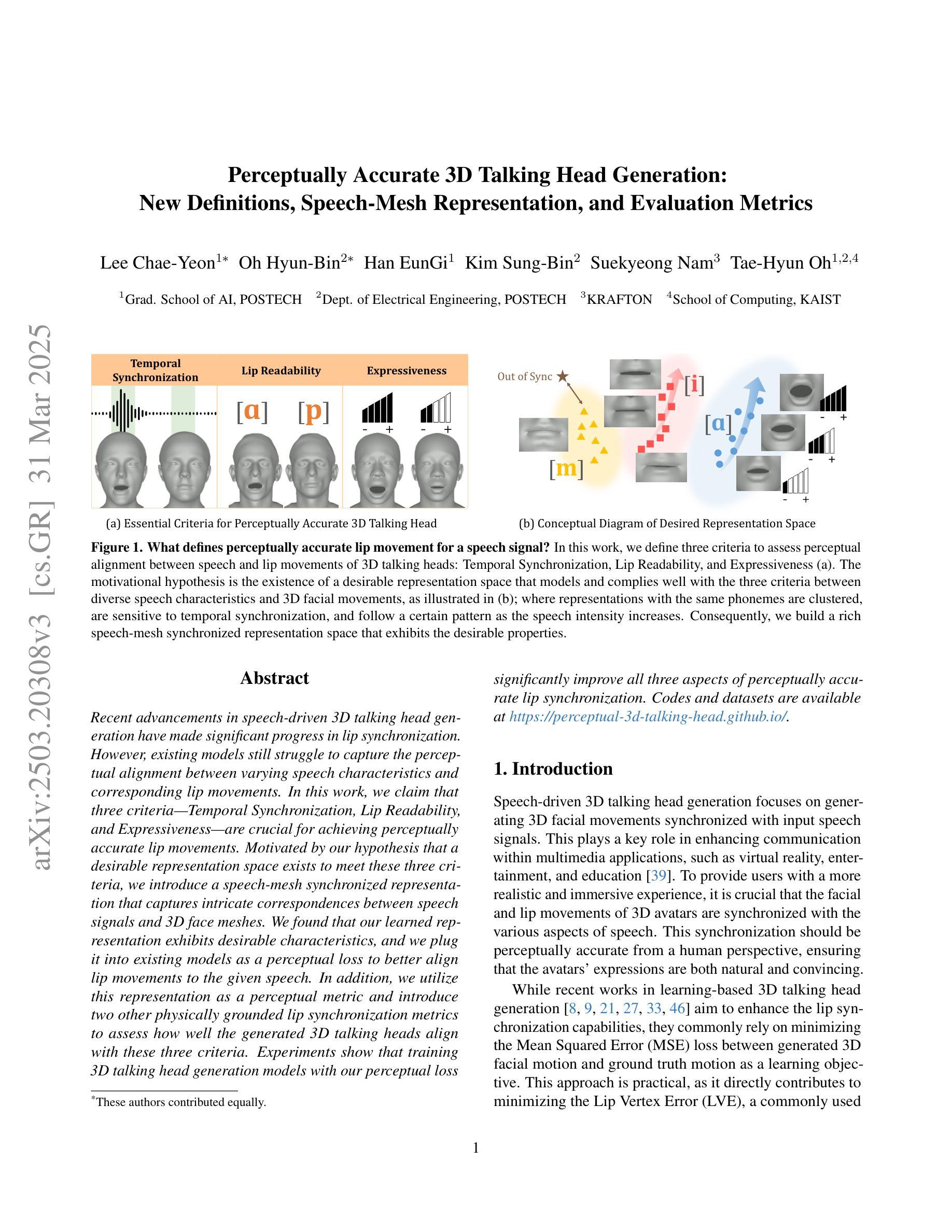
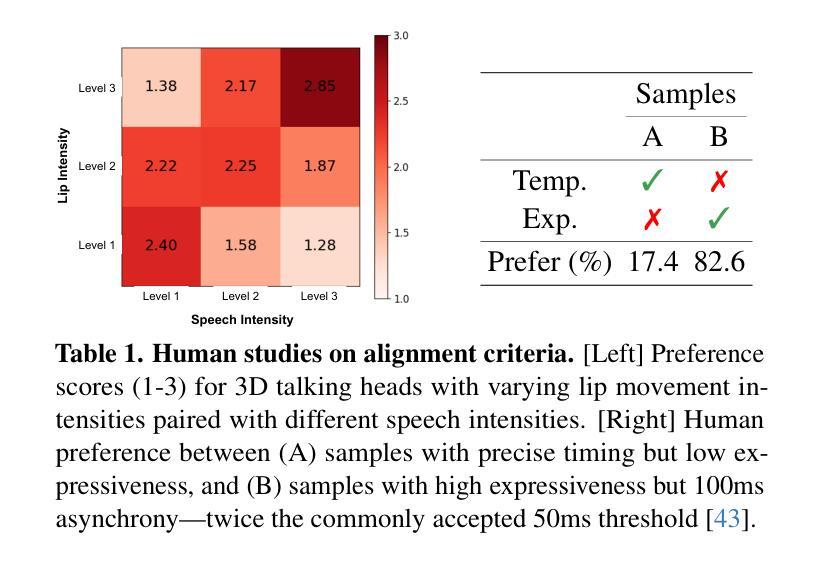
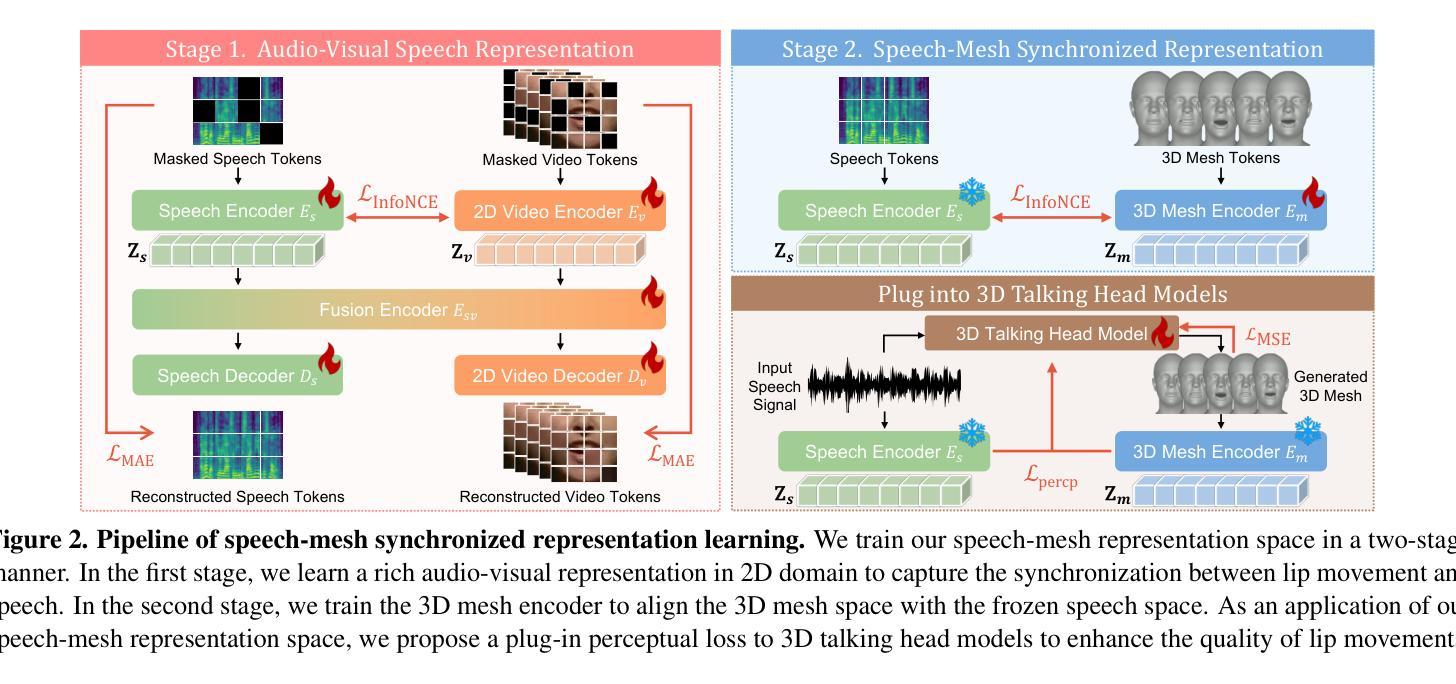
MagicDistillation: Weak-to-Strong Video Distillation for Large-Scale Few-Step Synthesis
Authors:Shitong Shao, Hongwei Yi, Hanzhong Guo, Tian Ye, Daquan Zhou, Michael Lingelbach, Zhiqiang Xu, Zeke Xie
Recently, open-source video diffusion models (VDMs), such as WanX, Magic141 and HunyuanVideo, have been scaled to over 10 billion parameters. These large-scale VDMs have demonstrated significant improvements over smaller-scale VDMs across multiple dimensions, including enhanced visual quality and more natural motion dynamics. However, these models face two major limitations: (1) High inference overhead: Large-scale VDMs require approximately 10 minutes to synthesize a 28-step video on a single H100 GPU. (2) Limited in portrait video synthesis: Models like WanX-I2V and HunyuanVideo-I2V often produce unnatural facial expressions and movements in portrait videos. To address these challenges, we propose MagicDistillation, a novel framework designed to reduce inference overhead while ensuring the generalization of VDMs for portrait video synthesis. Specifically, we primarily use sufficiently high-quality talking video to fine-tune Magic141, which is dedicated to portrait video synthesis. We then employ LoRA to effectively and efficiently fine-tune the fake DiT within the step distillation framework known as distribution matching distillation (DMD). Following this, we apply weak-to-strong (W2S) distribution matching and minimize the discrepancy between the fake data distribution and the ground truth distribution, thereby improving the visual fidelity and motion dynamics of the synthesized videos. Experimental results on portrait video synthesis demonstrate the effectiveness of MagicDistillation, as our method surpasses Euler, LCM, and DMD baselines in both FID/FVD metrics and VBench. Moreover, MagicDistillation, requiring only 4 steps, also outperforms WanX-I2V (14B) and HunyuanVideo-I2V (13B) on visualization and VBench. Our project page is https://magicdistillation.github.io/MagicDistillation/.
最近,开源视频扩散模型(VDMs),如WanX、Magic141和HunyuanVideo,已经被扩展到超过10亿参数。这些大规模VDMs在多个维度上相对于小规模VDMs表现出了显著改进,包括增强的视觉质量和更自然的运动动态。然而,这些模型面临两大局限:(1)推理开销高:大规模VDMs在单个H100 GPU上合成一个28步的视频大约需要10分钟。(2)肖像视频合成受限:像WanX-I2V和HunyuanVideo-I2V这样的模型在肖像视频中经常产生不自然的面部表情和动作。为了解决这些挑战,我们提出了MagicDistillation,这是一个新颖框架,旨在降低推理开销,同时确保VDM在肖像视频合成的泛化能力。具体来说,我们主要使用高质量讲话视频对Magic141进行微调,该模型专注于肖像视频合成。然后,我们采用LoRA在所谓的分布匹配蒸馏(DMD)的步骤蒸馏框架内有效地对假DiT进行微调。接下来,我们应用弱到强(W2S)分布匹配,并最小化假数据分布与真实数据分布之间的差异,从而提高合成视频的视觉逼真度和运动动态。关于肖像视频合成的实验结果证明了MagicDistillation的有效性,我们的方法在FID/FVD指标和VBench上都超越了Euler、LCM和DMD基线。此外,MagicDistillation仅需要4步,还在可视化和VBench方面超越了WanX-I2V(14B)和HunyuanVideo-I2V(13B)。我们的项目页面是https://magicdistillation.github.io/MagicDistillation/。
论文及项目相关链接
摘要
基于开源视频扩散模型的MagicDistillation框架旨在解决大规模视频扩散模型面临的高推理开销和肖像视频合成受限的问题。通过精细调整Magic141模型并使用LoRA技术有效调整假数据的分布匹配蒸馏(DMD)步骤内的DiT,框架能够减少推理时间并提高肖像视频合成的泛化能力。实验结果证明MagicDistillation在视觉效果和动态效果方面均超越了Euler、LCM和DMD基准测试,并在可视化效果方面超越了WanX-I2V和HunyuanVideo-I2V。该项目页面位于[https://magicdistillation.github.io/MagicDistillation/] 。
关键见解:
1. 大型开源视频扩散模型(如WanX,Magic141和HunyuanVideo)已被扩展到超过十亿个参数,这些模型在视觉质量和自然运动动态方面表现出显著改进。但存在两个主要局限性:高推理开销和肖像视频合成的局限性。
2. MagicDistillation框架旨在解决这些问题,通过减少推理时间并提高大规模视频扩散模型在肖像视频合成方面的泛化能力。
3. 该框架主要通过使用高质量对话视频对Magic141进行微调,专注于肖像视频合成。
4. 使用LoRA有效地微调假数据的分布匹配蒸馏(DMD)步骤内的DiT。
5. 通过应用弱到强(W2S)分布匹配,最小化假数据分布与地面真实分布之间的差异,从而提高合成视频的视觉保真度和运动动态。
6. 实验结果表明,MagicDistillation在肖像视频合成方面优于Euler、LCM和DMD基准测试,并在可视化效果和VBench上超越了WanX-I2V和HunyuanVideo-I2V。
7. 项目页面提供了有关MagicDistillation的更多详细信息。
点此查看论文截图
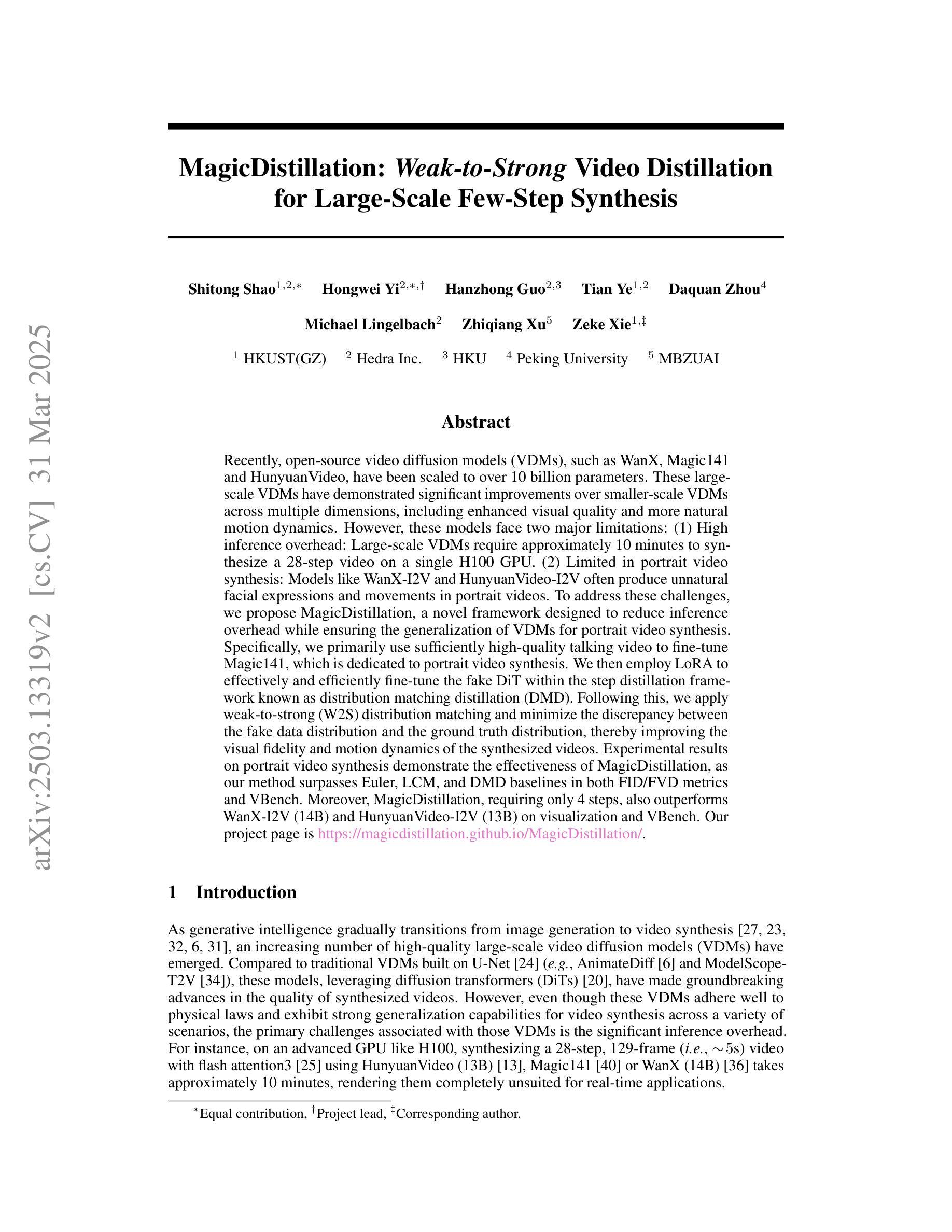
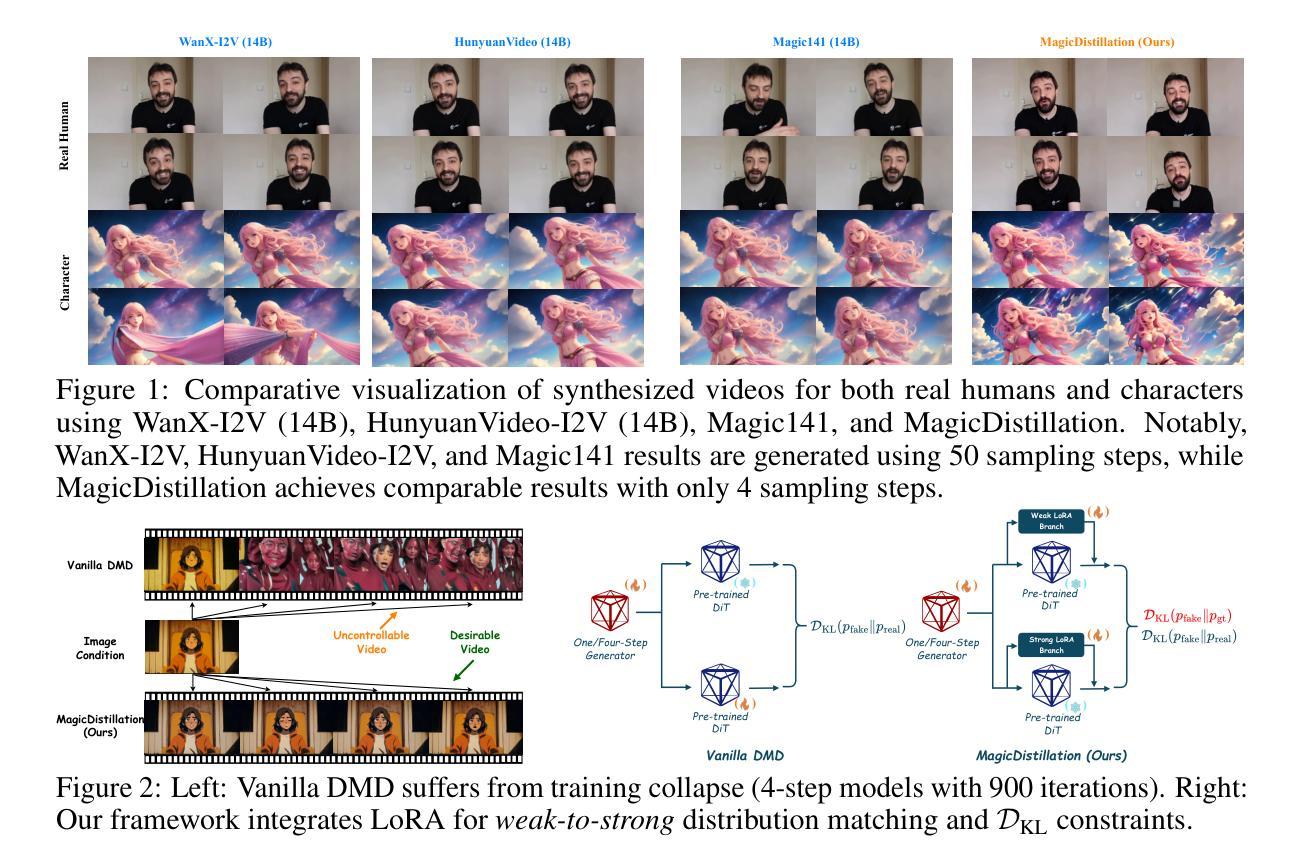
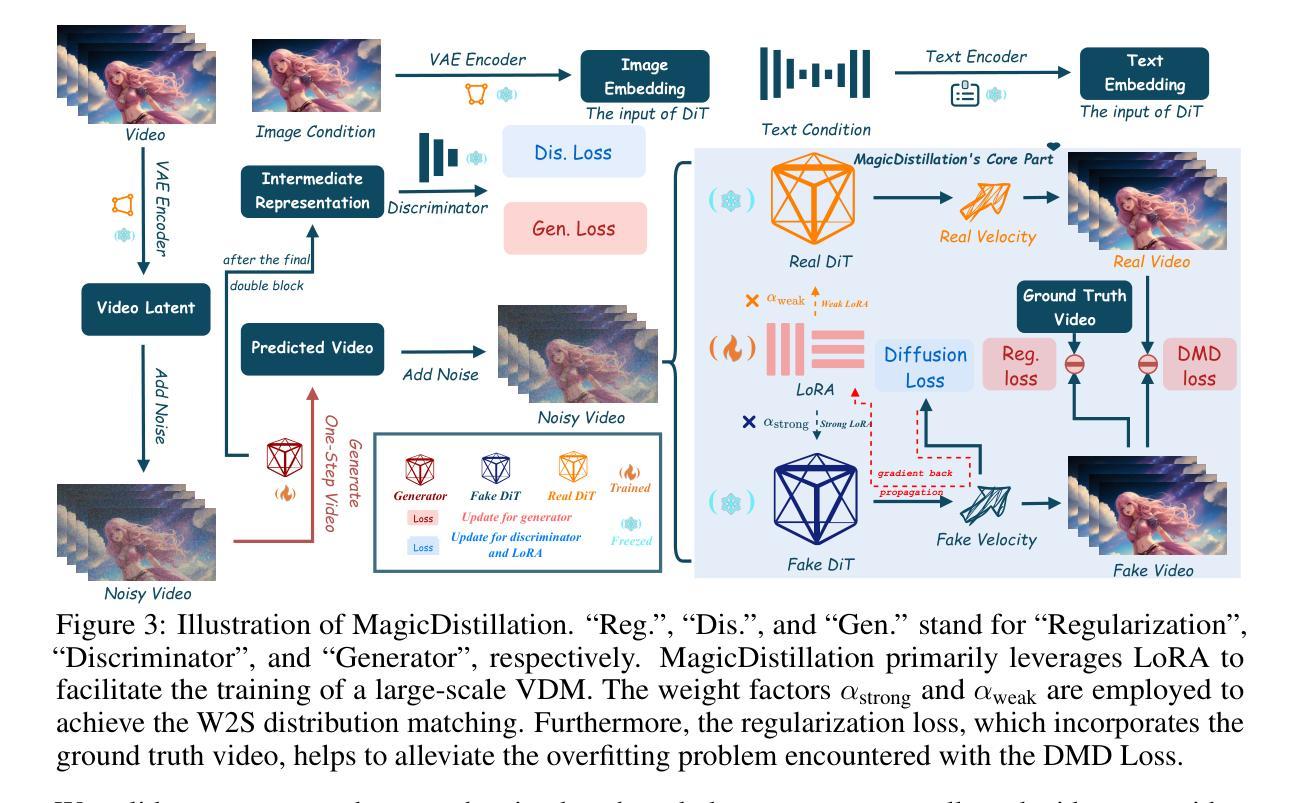
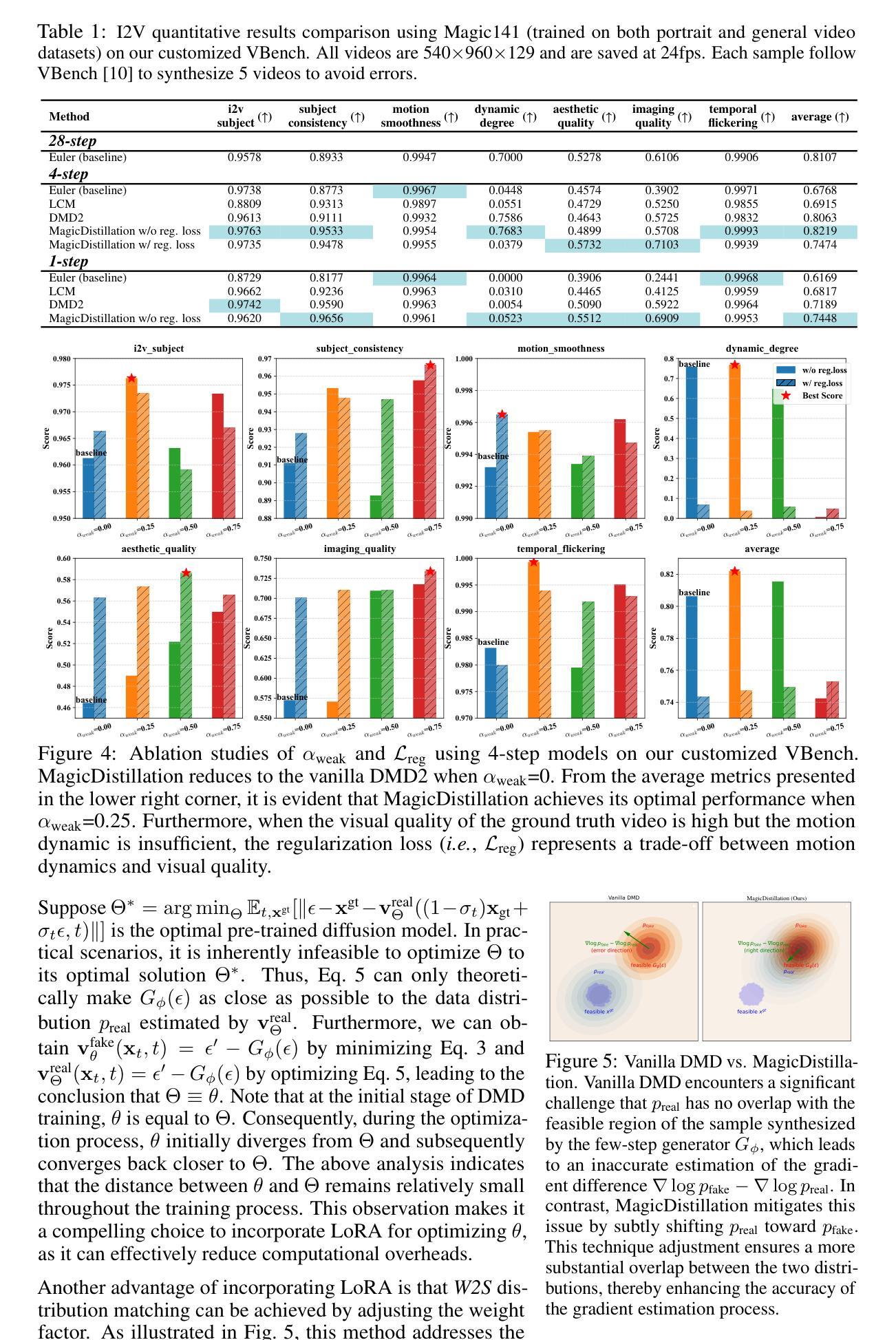
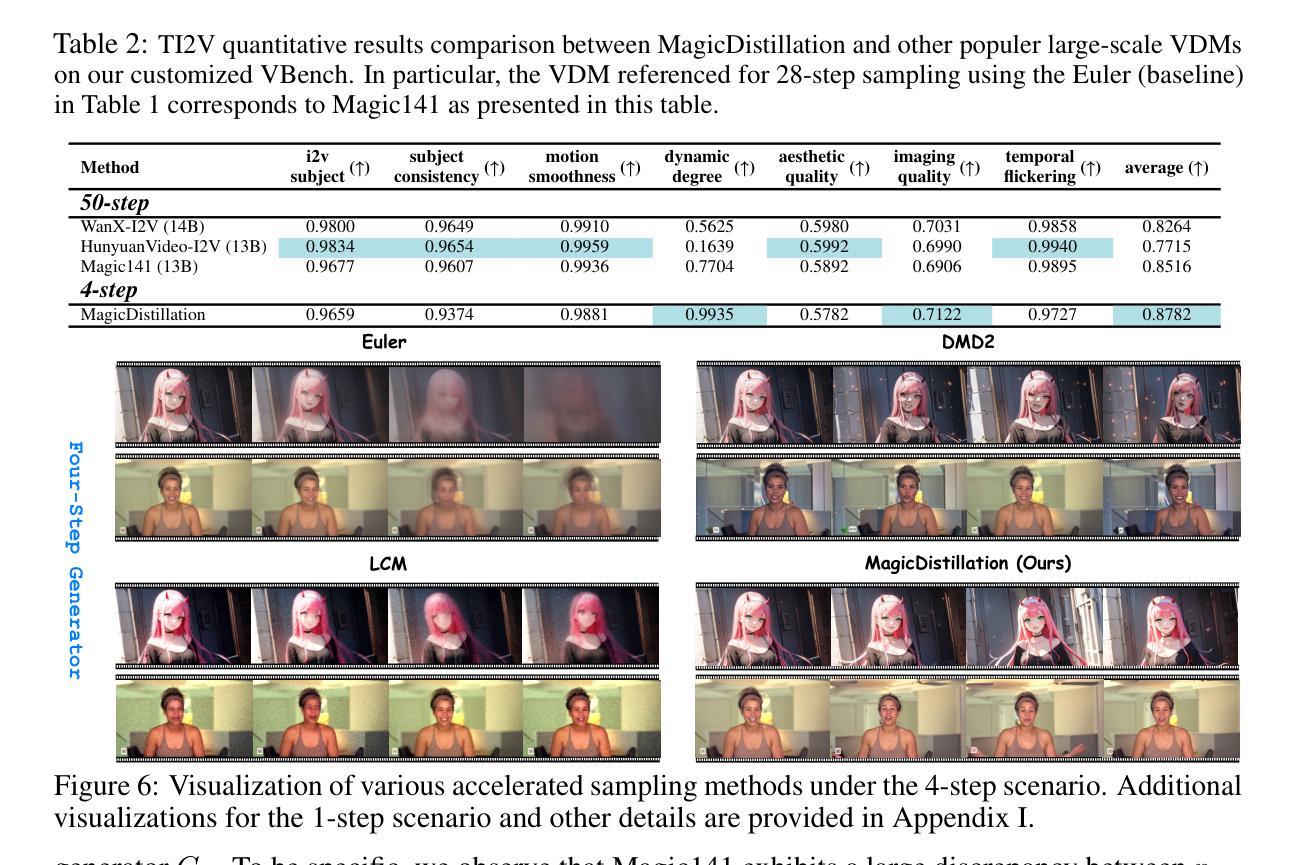
GMTalker: Gaussian Mixture-based Audio-Driven Emotional Talking Video Portraits
Authors:Yibo Xia, Lizhen Wang, Xiang Deng, Xiaoyan Luo, Yunhong Wang, Yebin Liu
Synthesizing high-fidelity and emotion-controllable talking video portraits, with audio-lip sync, vivid expressions, realistic head poses, and eye blinks, has been an important and challenging task in recent years. Most existing methods suffer in achieving personalized and precise emotion control, smooth transitions between different emotion states, and the generation of diverse motions. To tackle these challenges, we present GMTalker, a Gaussian mixture-based emotional talking portraits generation framework. Specifically, we propose a Gaussian mixture-based expression generator that can construct a continuous and disentangled latent space, achieving more flexible emotion manipulation. Furthermore, we introduce a normalizing flow-based motion generator pretrained on a large dataset with a wide-range motion to generate diverse head poses, blinks, and eyeball movements. Finally, we propose a personalized emotion-guided head generator with an emotion mapping network that can synthesize high-fidelity and faithful emotional video portraits. Both quantitative and qualitative experiments demonstrate our method outperforms previous methods in image quality, photo-realism, emotion accuracy, and motion diversity.
近年来,合成高保真和情绪可控的说话视频肖像已经成为一项重要且具有挑战性的任务,这些视频需要具有音频-嘴唇同步、生动的表情、真实的头部姿势和眨眼等特征。现有的大多数方法在实现对个人化精准情绪控制、不同情绪状态间流畅过渡以及生成多样动作方面存在困难。为了应对这些挑战,我们提出了GMTalker,这是一个基于高斯混合的情绪化说话肖像生成框架。具体来说,我们提出了一个基于高斯混合的表情生成器,能够构建连续且解耦的潜在空间,实现更灵活的情绪操控。此外,我们还引入了一个基于标准化流技术的动作生成器,该生成器在包含广泛动作的大型数据集上进行预训练,以生成多样化的头部姿势、眨眼以及眼球运动。最后,我们提出了一个个性化的情绪引导头部生成器,包括情绪映射网络,能够合成高保真和真实的情绪视频肖像。定量和定性实验均表明,我们的方法在图像质量、逼真度、情绪准确性和动作多样性方面优于以前的方法。
论文及项目相关链接
PDF Project page: https://bob35buaa.github.io/GMTalker. This work has been submitted to the IEEE journal for possible publication
Summary
高保真、情感可控的语音视频肖像合成是一项重要且具有挑战性的任务,包括音频与唇部的同步、生动的表情、真实的头部姿势和眨眼等。针对个性化、精确情感控制、不同情感状态间的平滑过渡以及多样动作生成等挑战,提出GMTalker框架,基于高斯混合的情感谈话肖像生成方法。构建连续且解耦的潜在空间,实现更灵活的情感操控;引入基于正规流技术的动作生成器,在大型数据集上预训练,生成多样化的头部姿势、眨眼和眼球运动;提出个性化情感引导头部生成器,带有情感映射网络,能合成高保真和真实的情感视频肖像。实验证明该方法在图像质量、逼真度、情感准确性和动作多样性上优于先前的方法。
Key Takeaways
- GMTalker是一个基于高斯混合的情感谈话肖像生成框架,旨在解决高保真、情感可控的语音视频肖像合成中的挑战。
- 该框架提出了一个基于高斯混合的表达生成器,构建了连续且解耦的潜在空间,实现更灵活的情感操控。
- 引入正规流技术,用于生成多样化的头部姿势、眨眼和眼球运动。
- 提出了个性化情感引导头部生成器,带有情感映射网络,确保合成的视频肖像具有真实感和高保真度。
- 该方法在图像质量、逼真度、情感准确性和动作多样性上表现出优越性。
- 实验结果证明了GMTalker的有效性,其性能优于先前的方法。
点此查看论文截图
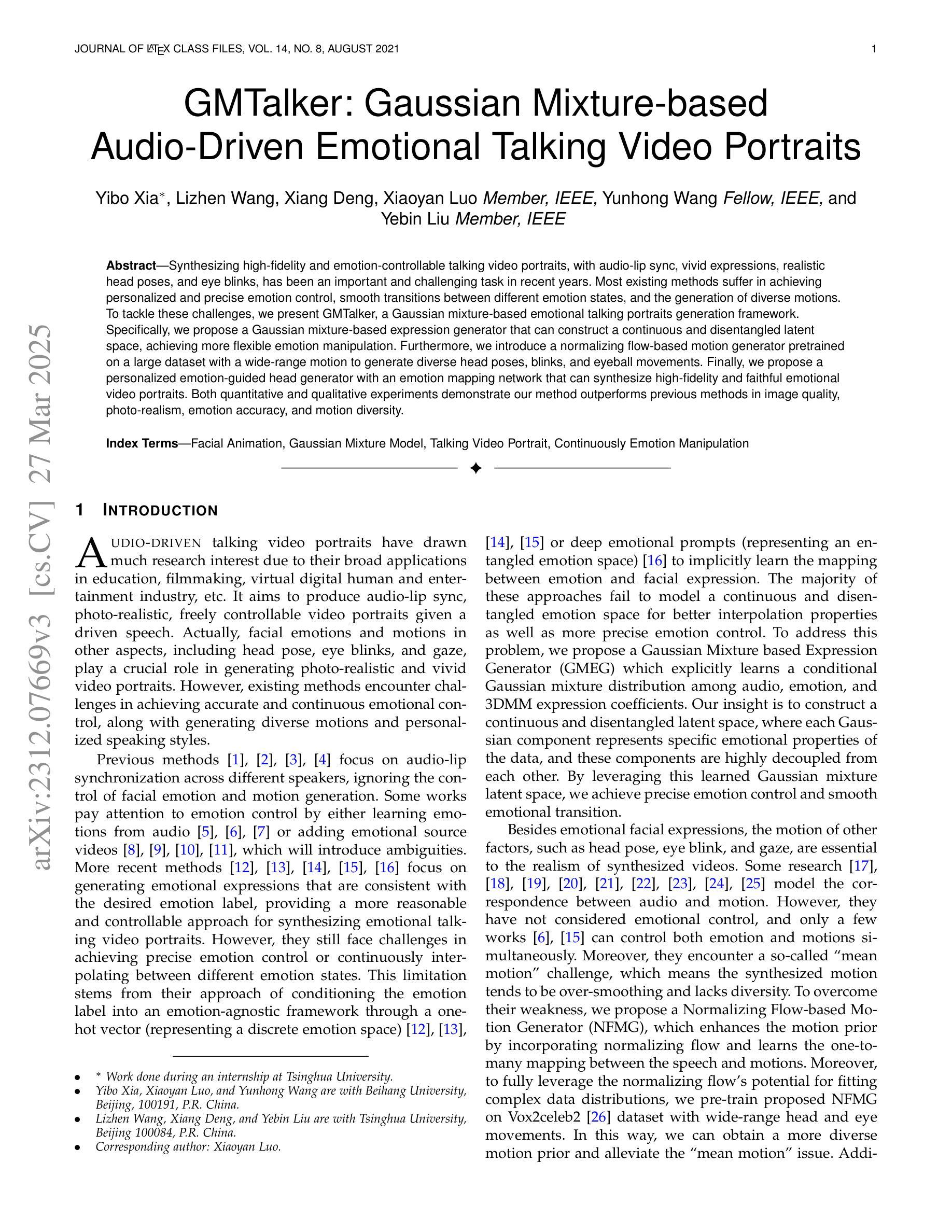
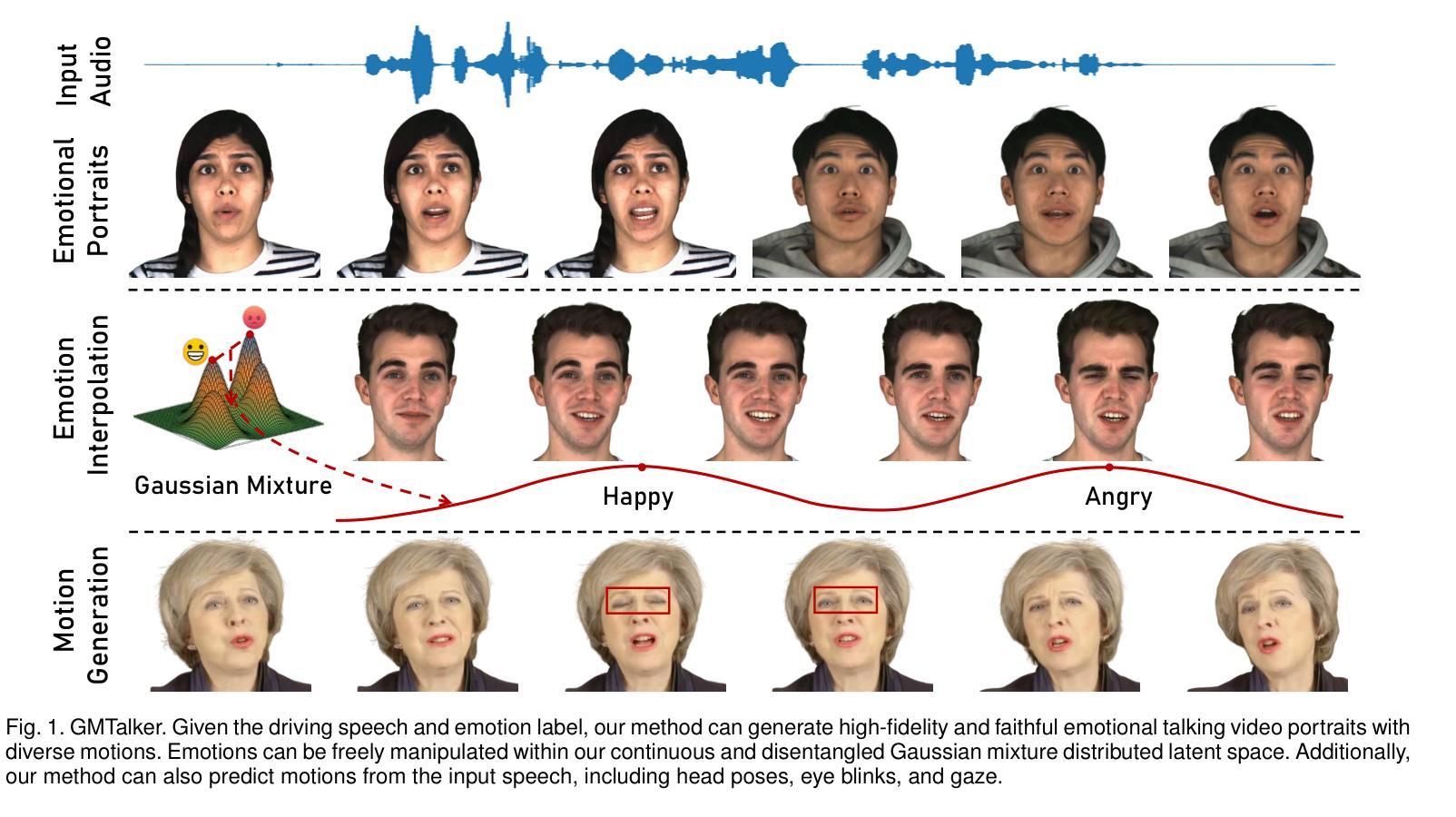
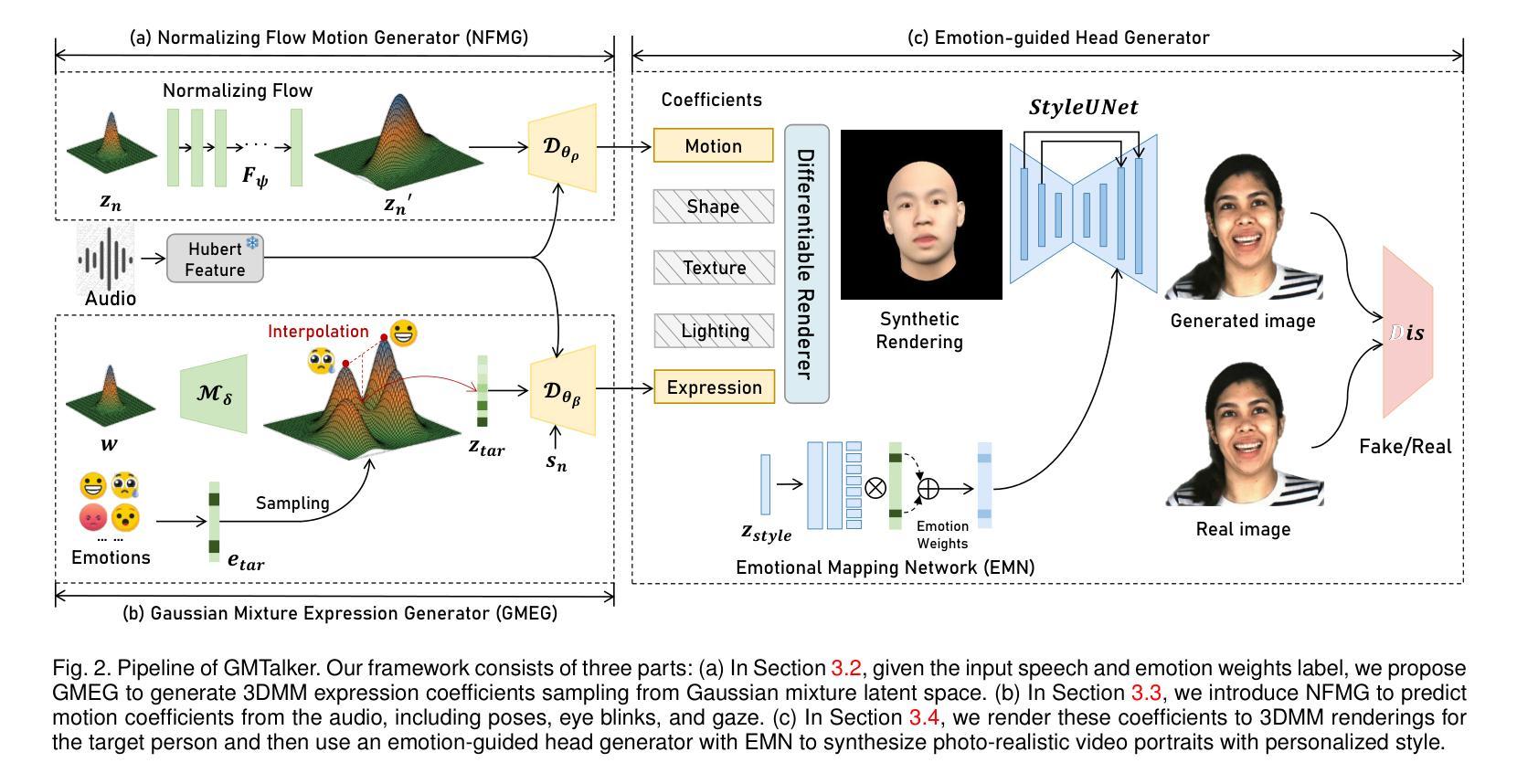
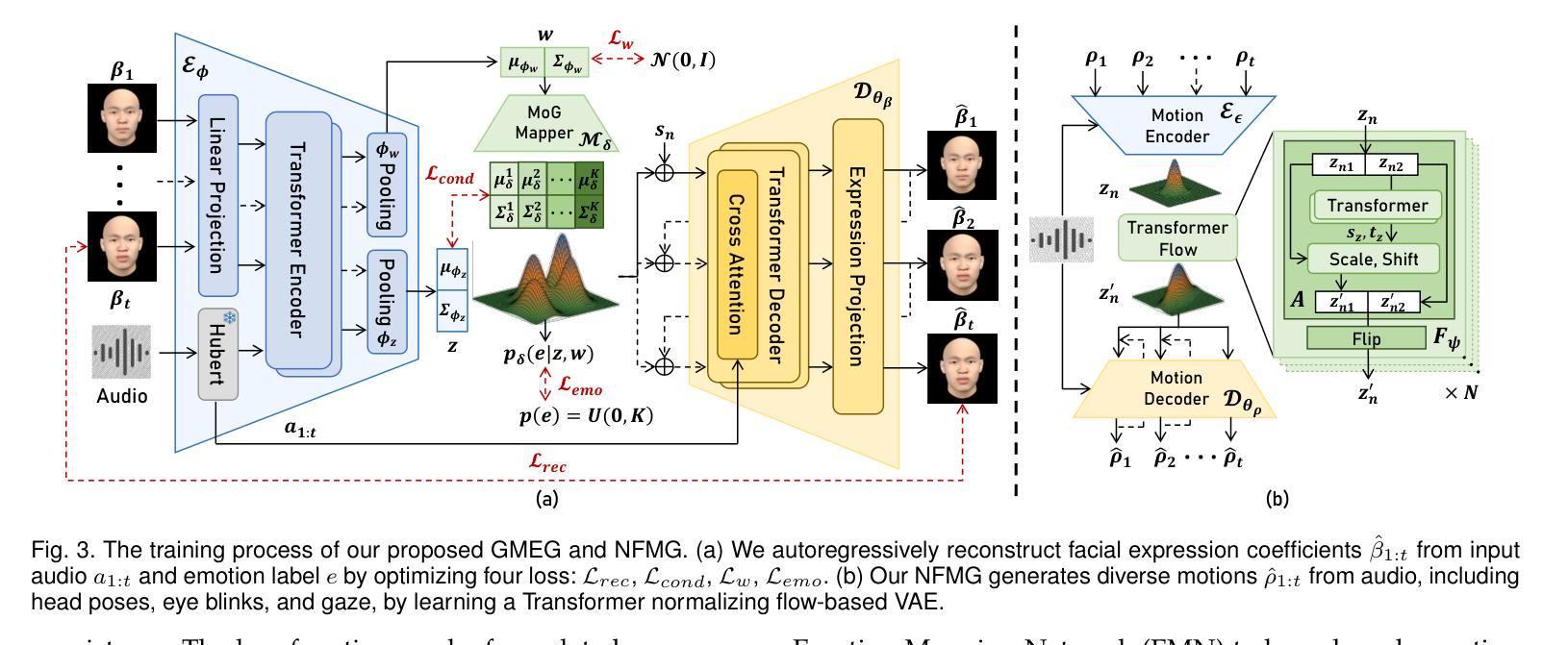