⚠️ 以下所有内容总结都来自于 大语言模型的能力,如有错误,仅供参考,谨慎使用
🔴 请注意:千万不要用于严肃的学术场景,只能用于论文阅读前的初筛!
💗 如果您觉得我们的项目对您有帮助 ChatPaperFree ,还请您给我们一些鼓励!⭐️ HuggingFace免费体验
2025-04-05 更新
TailedCore: Few-Shot Sampling for Unsupervised Long-Tail Noisy Anomaly Detection
Authors:Yoon Gyo Jung, Jaewoo Park, Jaeho Yoon, Kuan-Chuan Peng, Wonchul Kim, Andrew Beng Jin Teoh, Octavia Camps
We aim to solve unsupervised anomaly detection in a practical challenging environment where the normal dataset is both contaminated with defective regions and its product class distribution is tailed but unknown. We observe that existing models suffer from tail-versus-noise trade-off where if a model is robust against pixel noise, then its performance deteriorates on tail class samples, and vice versa. To mitigate the issue, we handle the tail class and noise samples independently. To this end, we propose TailSampler, a novel class size predictor that estimates the class cardinality of samples based on a symmetric assumption on the class-wise distribution of embedding similarities. TailSampler can be utilized to sample the tail class samples exclusively, allowing to handle them separately. Based on these facets, we build a memory-based anomaly detection model TailedCore, whose memory both well captures tail class information and is noise-robust. We extensively validate the effectiveness of TailedCore on the unsupervised long-tail noisy anomaly detection setting, and show that TailedCore outperforms the state-of-the-art in most settings.
我们致力于在实际具有挑战性的环境中解决无监督异常检测问题,在这种环境中,正常数据集既受到缺陷区域的污染,其产品类别分布又存在尾部但未知。我们发现现有模型存在尾部与噪声之间的权衡问题,即模型如果对像素噪声具有很强的鲁棒性,那么它在尾部类别样本上的性能就会下降,反之亦然。为了解决这一问题,我们对尾部类别和噪声样本进行了独立处理。为此,我们提出了TailSampler,这是一种新型的类别大小预测器,它基于嵌入相似性在类别分布上的对称假设来估计样本的类别基数。TailSampler可用于仅对尾部类别样本进行采样,从而可以单独处理它们。基于这些方面,我们构建了一个基于内存的异常检测模型TailedCore,其内存能够很好地捕获尾部类别信息并且具有抗噪声性。我们在无监督的长尾噪声异常检测设置上进行了广泛的验证,结果表明TailedCore在大多数设置中都优于最新技术。
论文及项目相关链接
PDF Accepted to CVPR2025
Summary
本文旨在解决在实际挑战环境下无监督异常检测问题,其中正常数据集含有缺陷区域且其产品类别分布长尾未知。现有模型面临尾部与噪声之间的权衡问题。为解决此问题,本文独立处理尾部类别和噪声样本。为此,提出了TailSampler,一种基于嵌入相似性对称假设的新型类别大小预测器,可估算样本类别基数。TailSampler可用于单独采样尾部类别样本。基于此,构建了一个基于内存的异常检测模型TailedCore,其内存能够很好地捕捉尾部类别信息且对噪声具有鲁棒性。在无人监督的长期尾部噪声异常检测设置中验证了TailedCore的有效性,并显示其在大多数设置中优于现有技术。
Key Takeaways
- 解决实际挑战环境下的无监督异常检测问题,其中数据集存在缺陷和未知的长尾产品类别分布。
- 现有模型面临尾部类别与噪声之间的权衡问题。
- 提出TailSampler,一种新型类别大小预测器,可估算样本类别基数,用于独立处理尾部类别样本。
- 基于TailSampler构建TailedCore模型,该模型在捕捉尾部类别信息和噪声鲁棒性方面表现出色。
- TailedCore通过单独处理尾部类别和噪声样本,提高了异常检测性能。
- 在长期尾部噪声异常检测设置的无人监督环境中验证了TailedCore的有效性。
点此查看论文截图
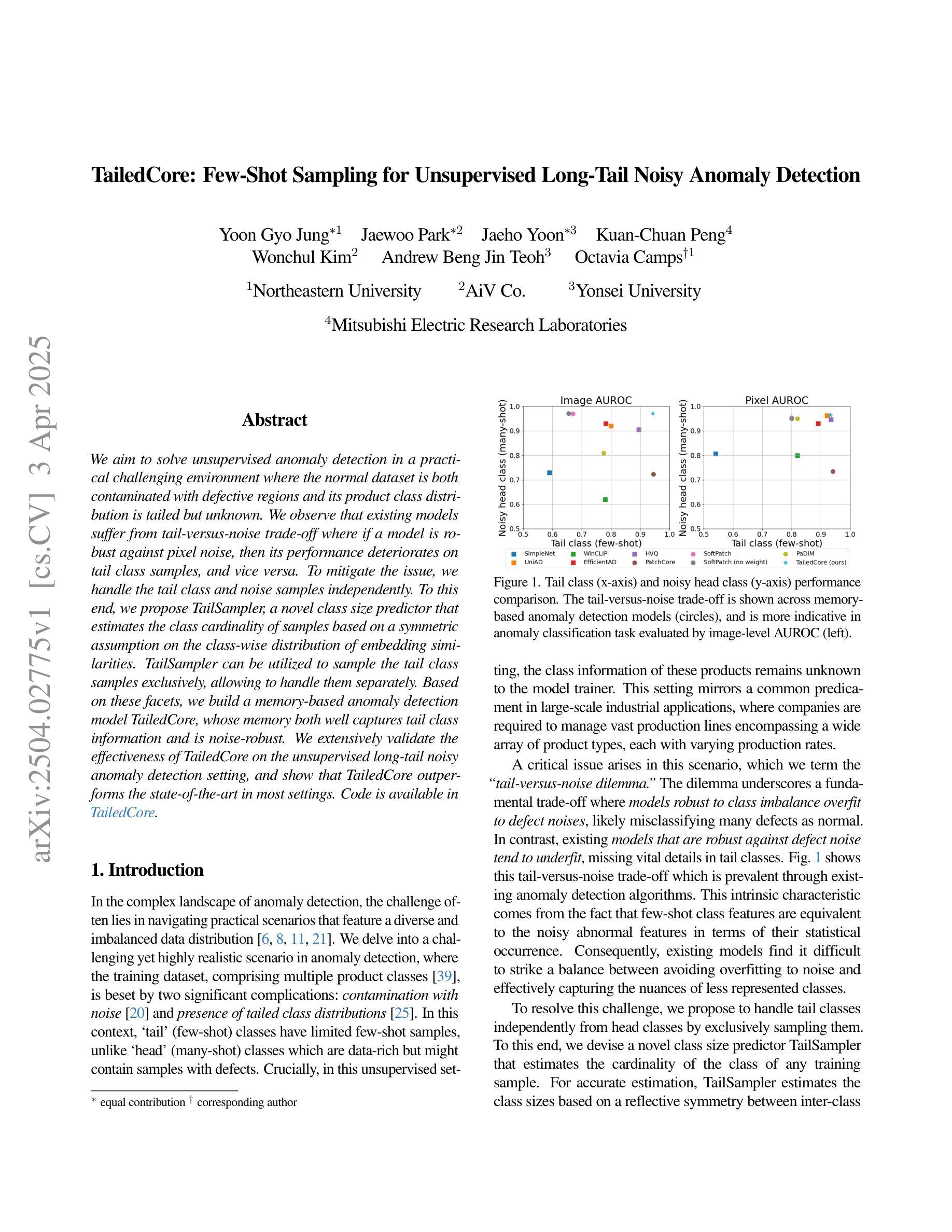
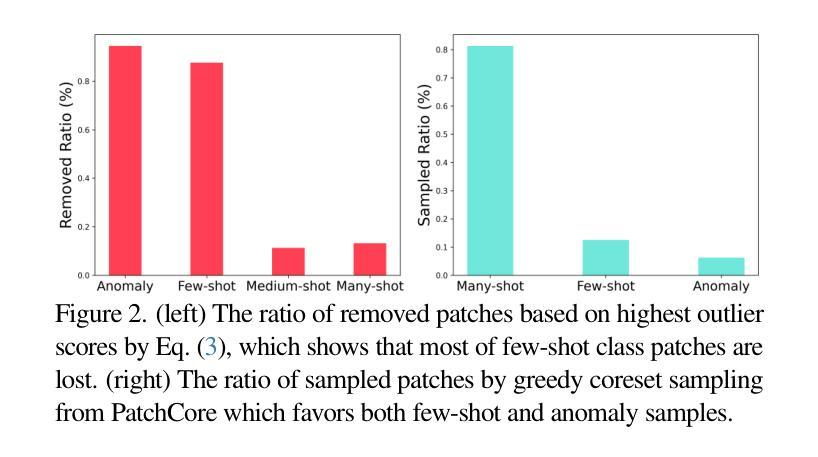
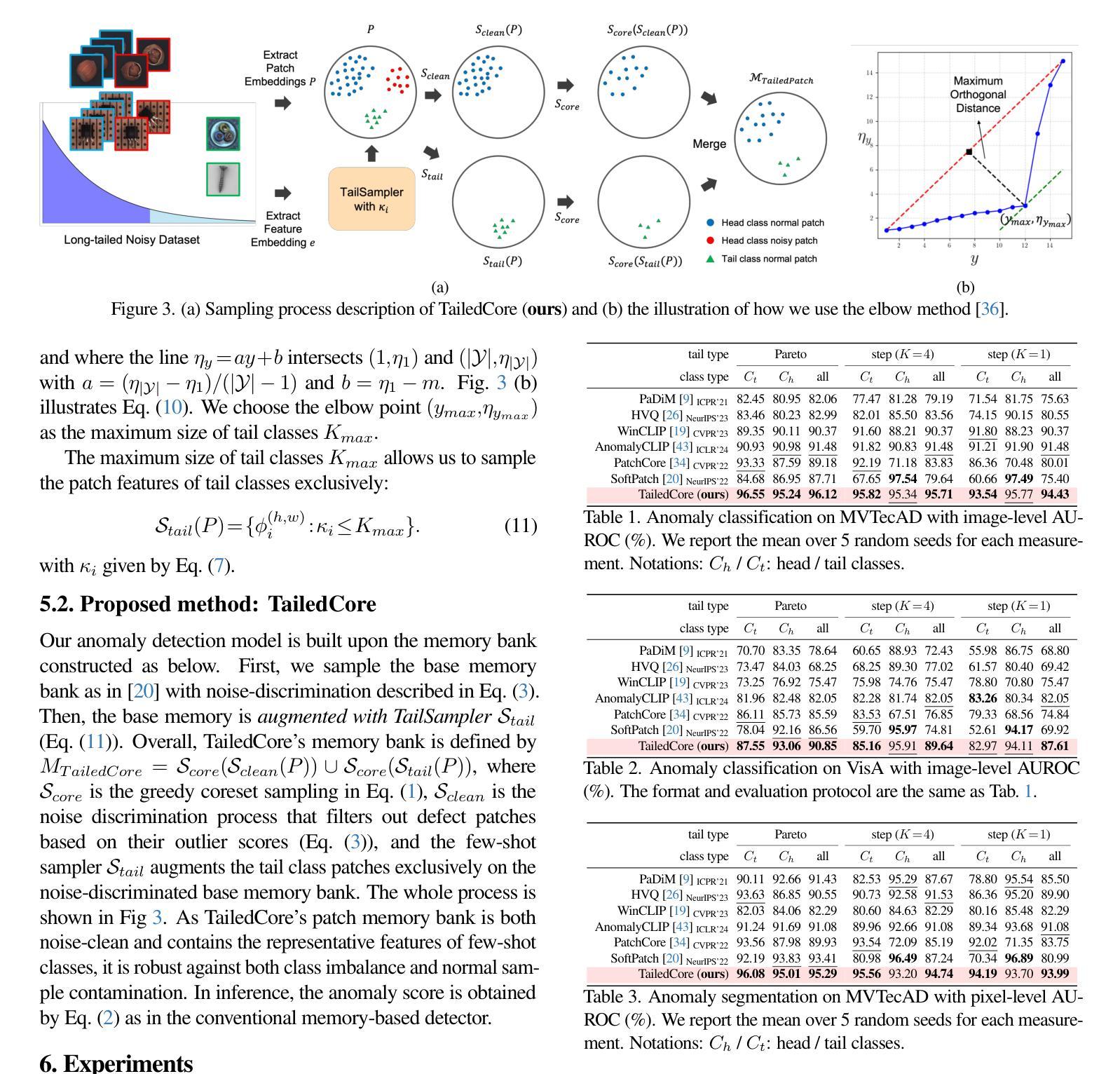
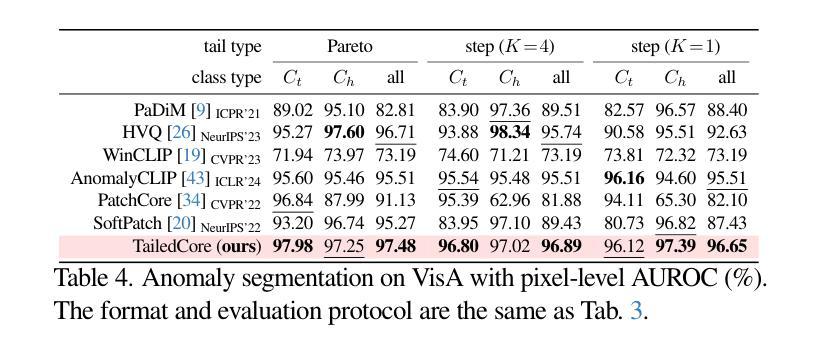
BOOST: Bootstrapping Strategy-Driven Reasoning Programs for Program-Guided Fact-Checking
Authors:Qisheng Hu, Quanyu Long, Wenya Wang
Program-guided reasoning has shown promise in complex claim fact-checking by decomposing claims into function calls and executing reasoning programs. However, prior work primarily relies on few-shot in-context learning (ICL) with ad-hoc demonstrations, which limit program diversity and require manual design with substantial domain knowledge. Fundamentally, the underlying principles of effective reasoning program generation still remain underexplored, making it challenging to construct effective demonstrations. To address this, we propose BOOST, a bootstrapping-based framework for few-shot reasoning program generation. BOOST explicitly integrates claim decomposition and information-gathering strategies as structural guidance for program generation, iteratively refining bootstrapped demonstrations in a strategy-driven and data-centric manner without human intervention. This enables a seamless transition from zero-shot to few-shot strategic program-guided learning, enhancing interpretability and effectiveness. Experimental results show that BOOST outperforms prior few-shot baselines in both zero-shot and few-shot settings for complex claim verification.
程序引导推理通过将声明分解为函数调用并执行推理程序,在复杂的声明查证中显示出良好的前景。然而,早期的工作主要依赖于基于上下文的学习(ICL)少数样本演示,这限制了程序的多样性并需要大量手动设计知识和经验的投入。从根本上讲,有效推理程序生成的基本原理尚未得到充分研究,这增加了构建有效演示的挑战性。为了解决这一问题,我们提出了基于BOOST策略的少数样本推理程序生成框架。BOOST明确地将声明分解和信息收集策略整合为程序生成的结构性指导,以策略驱动和数据为中心的方式无人工干预地迭代优化自举演示。这实现了从零样本到少数样本战略程序引导学习的无缝过渡,提高了可解释性和有效性。实验结果表明,在零样本和少数样本场景下,BOOST对复杂的声明验证任务都超过了之前的少数样本基线模型。
论文及项目相关链接
PDF 18 pages, 5 figures
Summary
本文探讨了基于程序的推理在复杂声明核查中的应用前景。然而,先前的工作主要依赖于少数场景下的上下文学习(ICL),这种方式限制了程序多样性并需要大量领域知识来设计。针对这一问题,本文提出了基于助推技术的少数场景推理程序生成框架BOOST。BOOST通过整合声明分解和信息收集策略作为程序生成的引导结构,并以策略驱动和数据中心的方式进行迭代改进示范程序,提高了程序引导学习的效果。实验结果显示,BOOST在零场景和少数场景下的复杂声明验证中均优于先前的少数场景基线方法。
Key Takeaways
- 程序引导推理在复杂声明核查中展现潜力。
- 先前的工作主要依赖少数场景下的上下文学习(ICL),限制了程序多样性并需要大量领域知识。
- BOOST框架通过整合声明分解和信息收集策略来引导程序生成。
- BOOST通过迭代改进示范程序,实现了从零场景到少数场景的程序引导学习的无缝过渡。
- BOOST提高了程序引导学习的解释性和有效性。
- 实验结果显示,BOOST在复杂声明验证方面优于先前的少数场景基线方法。
点此查看论文截图
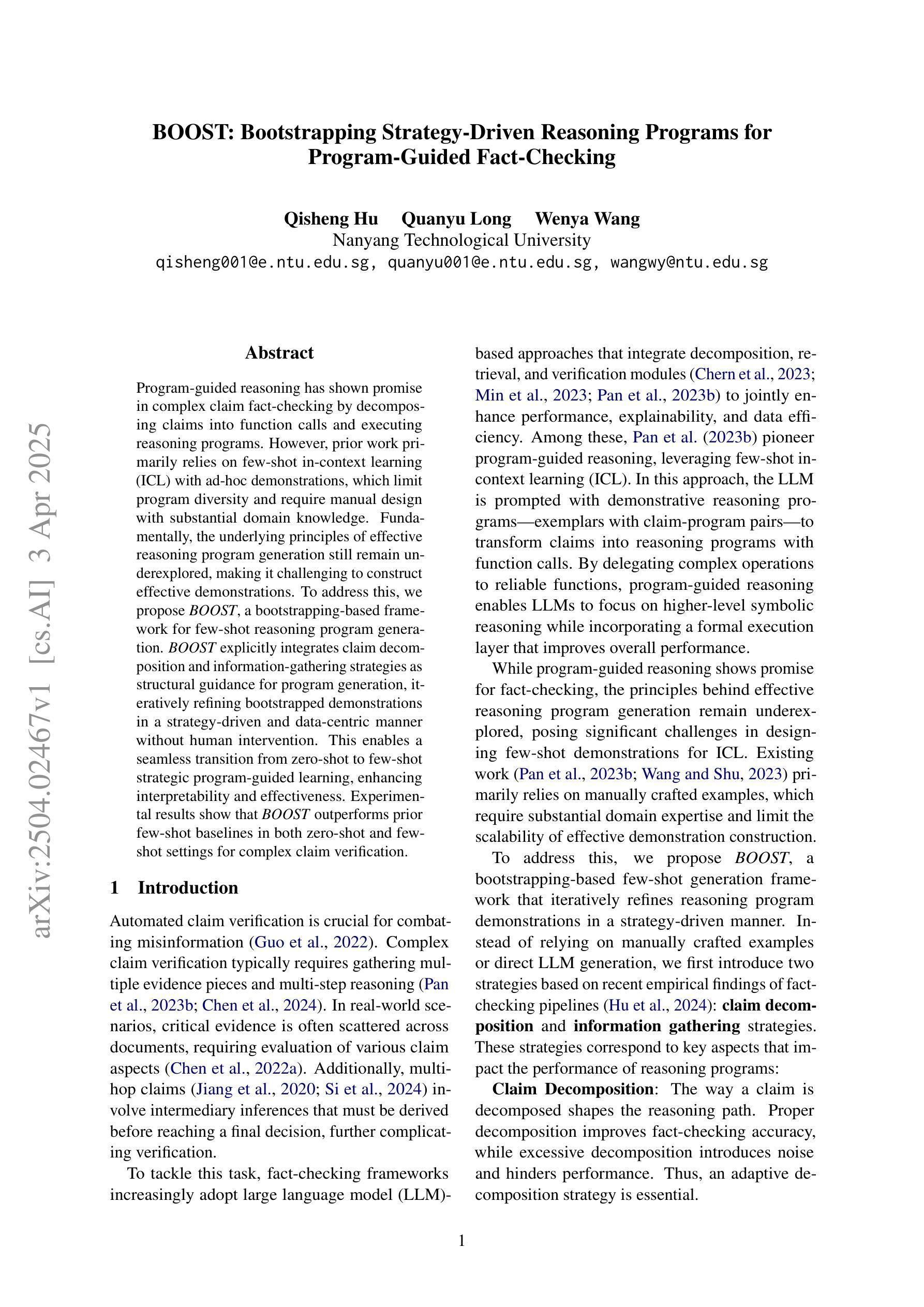
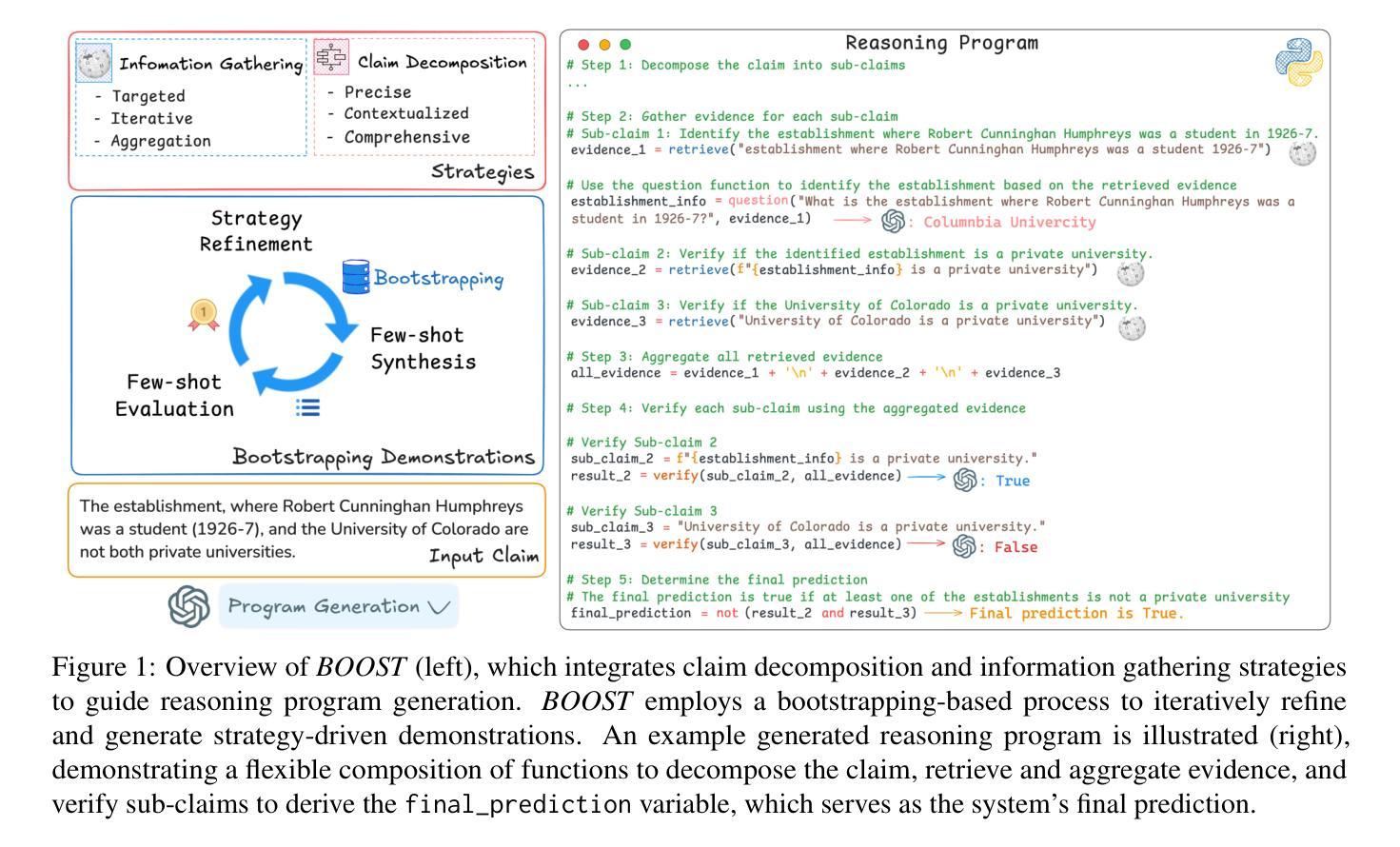
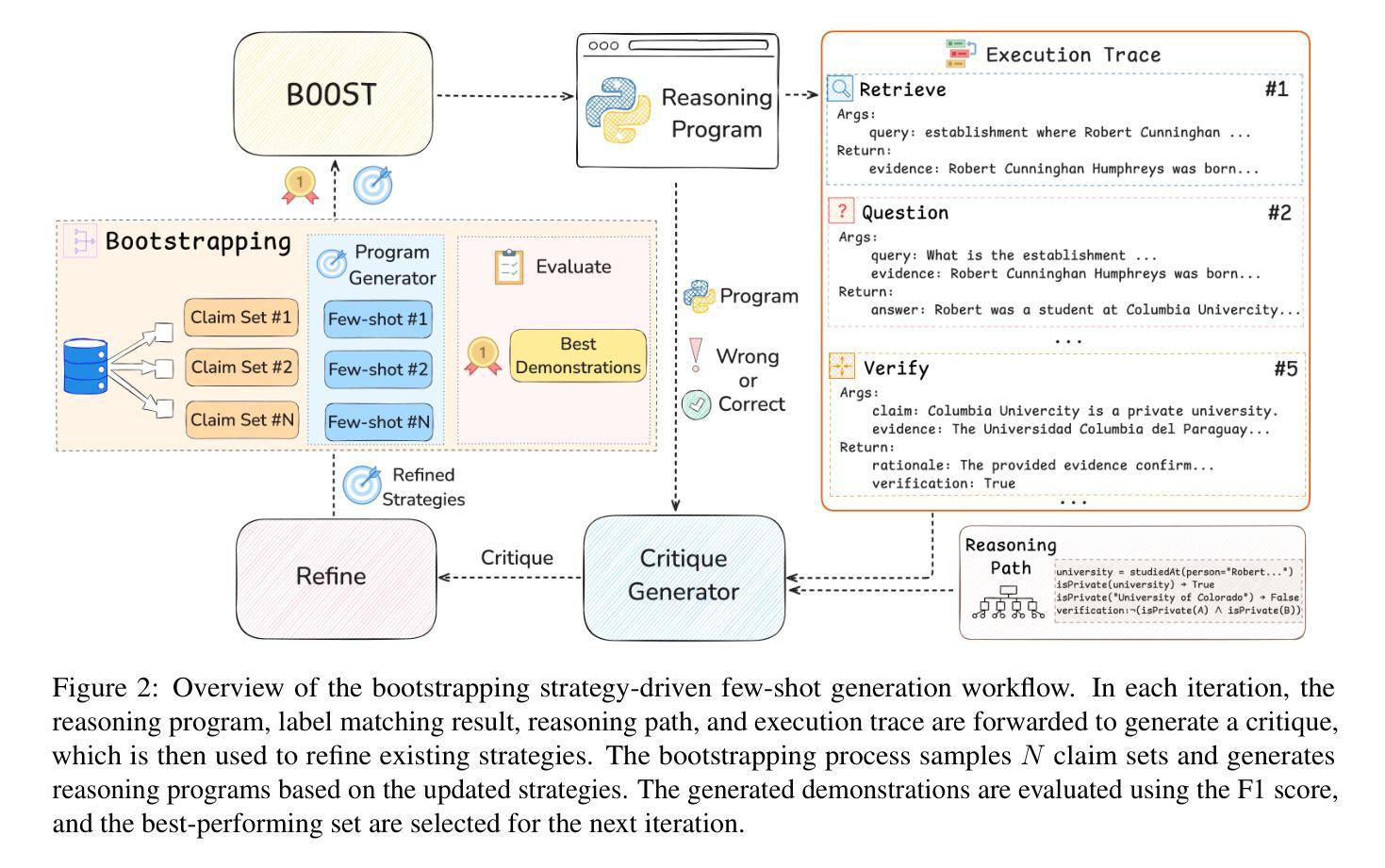
Taylor Series-Inspired Local Structure Fitting Network for Few-shot Point Cloud Semantic Segmentation
Authors:Changshuo Wang, Shuting He, Xiang Fang, Meiqing Wu, Siew-Kei Lam, Prayag Tiwari
Few-shot point cloud semantic segmentation aims to accurately segment “unseen” new categories in point cloud scenes using limited labeled data. However, pretraining-based methods not only introduce excessive time overhead but also overlook the local structure representation among irregular point clouds. To address these issues, we propose a pretraining-free local structure fitting network for few-shot point cloud semantic segmentation, named TaylorSeg. Specifically, inspired by Taylor series, we treat the local structure representation of irregular point clouds as a polynomial fitting problem and propose a novel local structure fitting convolution, called TaylorConv. This convolution learns the low-order basic information and high-order refined information of point clouds from explicit encoding of local geometric structures. Then, using TaylorConv as the basic component, we construct two variants of TaylorSeg: a non-parametric TaylorSeg-NN and a parametric TaylorSeg-PN. The former can achieve performance comparable to existing parametric models without pretraining. For the latter, we equip it with an Adaptive Push-Pull (APP) module to mitigate the feature distribution differences between the query set and the support set. Extensive experiments validate the effectiveness of the proposed method. Notably, under the 2-way 1-shot setting, TaylorSeg-PN achieves improvements of +2.28% and +4.37% mIoU on the S3DIS and ScanNet datasets respectively, compared to the previous state-of-the-art methods. Our code is available at https://github.com/changshuowang/TaylorSeg.
少量点云语义分割旨在利用有限的标记数据准确分割点云场景中的“未见”新类别。然而,基于预训练的方法不仅引入了过多的时间开销,而且忽略了不规则点云之间的局部结构表示。为了解决这些问题,我们提出了一种用于少量点云语义分割的无预训练局部结构拟合网络,名为TaylorSeg。具体地说,受到泰勒级数(Taylor series)的启发,我们将不规则点云的局部结构表示视为多项式拟合问题,并提出了一种新的局部结构拟合卷积,称为TaylorConv。这种卷积通过显式编码局部几何结构来学习点云的低阶基本信息和高阶精细信息。然后,以TaylorConv为基本组件,我们构建了TaylorSeg的两个变体:非参数的TaylorSeg-NN和参数的TaylorSeg-PN。前者可以在没有预训练的情况下实现与现有参数模型相当的性能。对于后者,我们为其配备了一个自适应推拉(APP)模块,以减轻查询集和支持集之间的特征分布差异。大量实验验证了所提出方法的有效性。值得注意的是,在2路1炮的设置下,TaylorSeg-PN在S3DIS和ScanNet数据集上的mIoU分别提高了+2.28%和+4.37%,与之前的最新方法相比。我们的代码位于https://github.com/changshuowang/TaylorSeg。
论文及项目相关链接
Summary
针对少样本点云语义分割任务,我们提出了一个无需预训练的局部结构拟合网络——TaylorSeg。它采用泰勒级数作为灵感来源,将不规则点云的局部结构表示作为多项式拟合问题,并创新性地提出了泰勒卷积(TaylorConv)。该卷积能从局部几何结构的显式编码中学习点云的低阶基础信息和高阶精细信息。使用TaylorConv作为基础组件,我们构建了非参数的TaylorSeg-NN和参数化的TaylorSeg-PN两种变体。前者无需预训练即可达到与现有参数模型相当的性能,而后者配备了自适应推拉(APP)模块以减小查询集和支持集之间的特征分布差异。实验证明,该方法有效。在2路1射击设置下,TaylorSeg-PN在S3DIS和ScanNet数据集上的mIoU分别提高了+2.28%和+4.37%。
Key Takeaways
- Few-shot point cloud semantic segmentation旨在利用有限的标记数据准确分割点云场景中的“未见”新类别。
- 提出的TaylorSeg网络无需预训练,专注于处理不规则点云的局部结构表示。
- 泰勒卷积(TaylorConv)作为核心组件,用于学习点云的局部几何结构信息。
- TaylorSeg包括非参数的TaylorSeg-NN和参数化的TaylorSeg-PN两种变体。
- TaylorSeg-PN配备了自适应推拉(APP)模块以减小查询集和支持集之间的特征分布差异。
- 实验结果显示,TaylorSeg在少样本点云语义分割任务中取得了显著效果。
- 在特定设置下,TaylorSeg-PN相较于现有方法提高了mIoU,表现优异。
点此查看论文截图
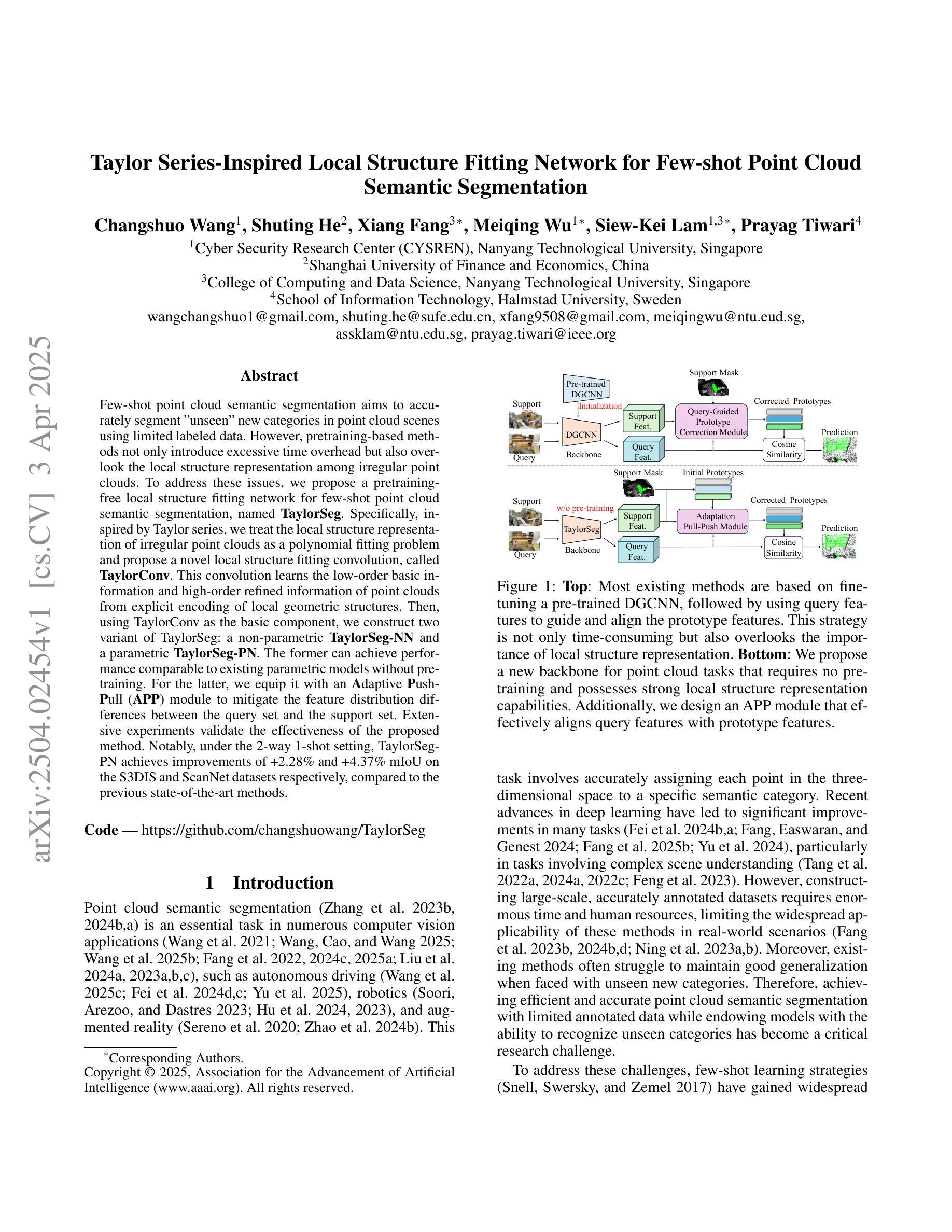
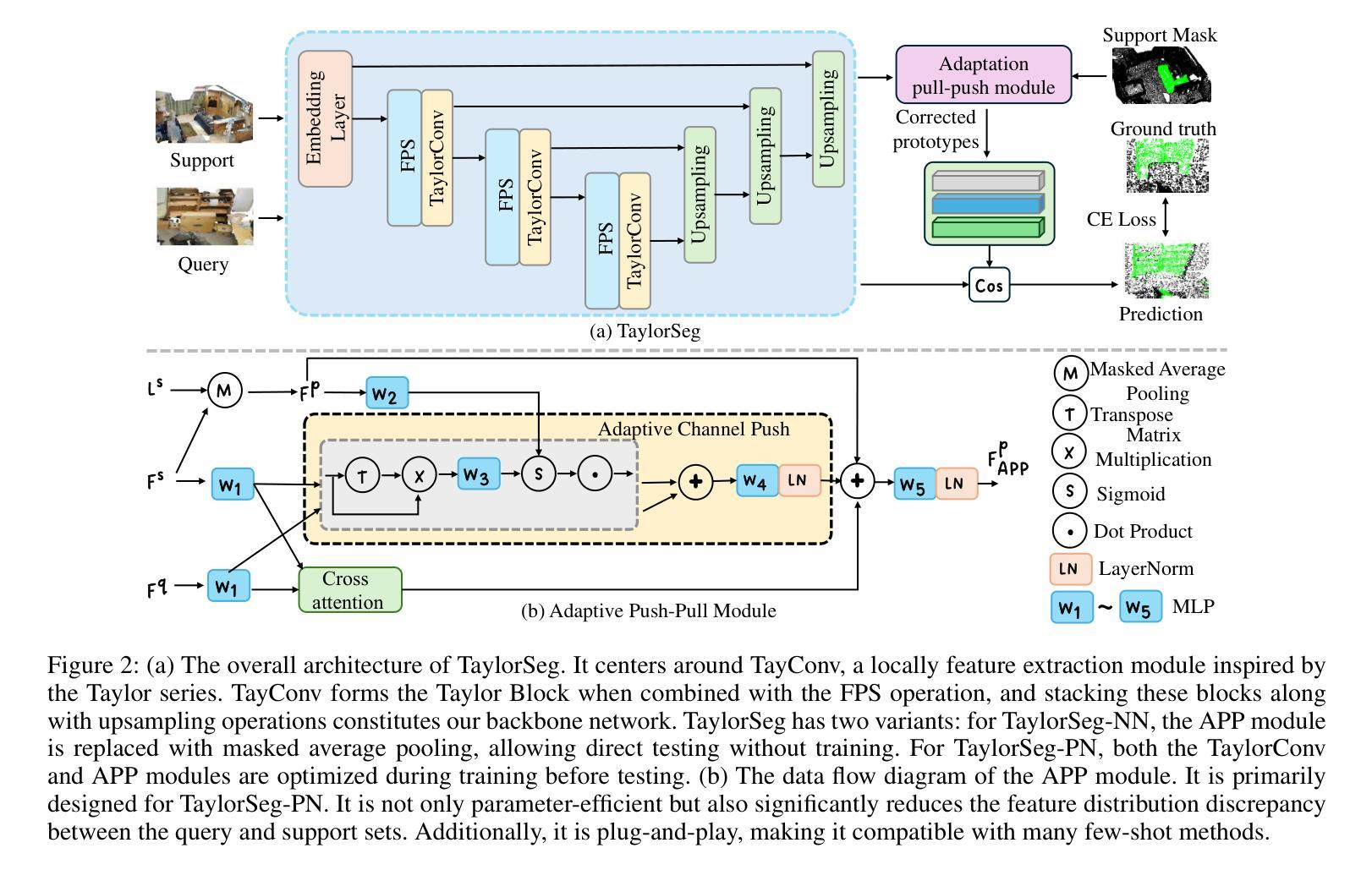
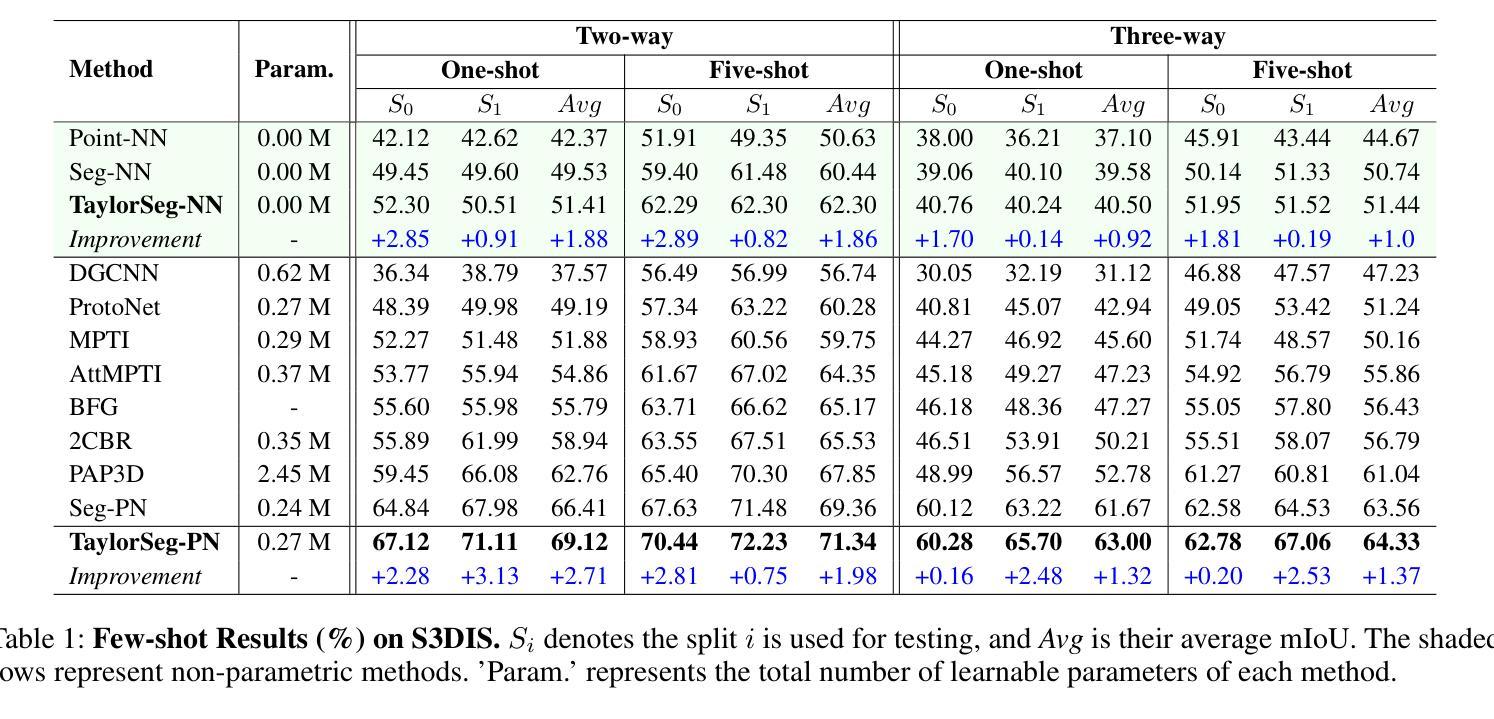
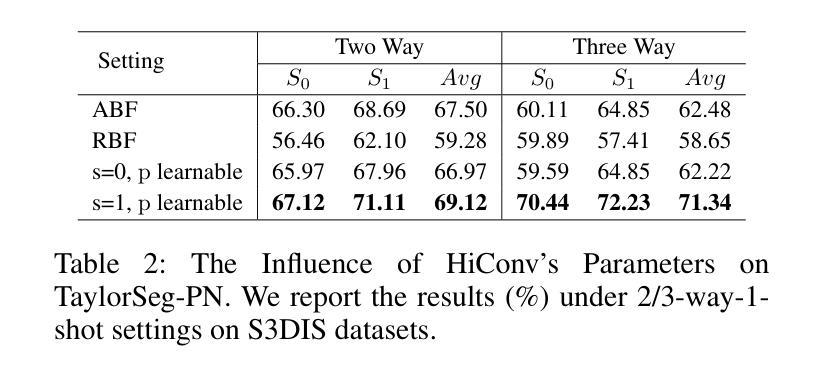
Towards Interpretable Soft Prompts
Authors:Oam Patel, Jason Wang, Nikhil Shivakumar Nayak, Suraj Srinivas, Himabindu Lakkaraju
Soft prompts have been popularized as a cheap and easy way to improve task-specific LLM performance beyond few-shot prompts. Despite their origin as an automated prompting method, however, soft prompts and other trainable prompts remain a black-box method with no immediately interpretable connections to prompting. We create a novel theoretical framework for evaluating the interpretability of trainable prompts based on two desiderata: faithfulness and scrutability. We find that existing methods do not naturally satisfy our proposed interpretability criterion. Instead, our framework inspires a new direction of trainable prompting methods that explicitly optimizes for interpretability. To this end, we formulate and test new interpretability-oriented objective functions for two state-of-the-art prompt tuners: Hard Prompts Made Easy (PEZ) and RLPrompt. Our experiments with GPT-2 demonstrate a fundamental trade-off between interpretability and the task-performance of the trainable prompt, explicating the hardness of the soft prompt interpretability problem and revealing odd behavior that arises when one optimizes for an interpretability proxy.
软提示作为一种低成本、易操作的方法来提升特定任务的LLM性能,在超出少数几种场景提示下受到广泛欢迎。然而,尽管其起源于一种自动化提示方法,软提示和其他可训练的提示仍然是黑箱方法,与提示之间没有直接的、可解释的关联。我们基于两个期望(忠实性和可审查性)为评估可训练提示的可解释性创建了一个新型的理论框架。我们发现现有方法并不能自然满足我们提出的可解释性标准。相反,我们的框架为可训练提示方法提供了新的方向,该方法旨在明确优化可解释性。为此,我们为两种先进的提示调整器制定了新的面向可解释性的目标函数,并对它们进行了测试:易操作硬提示(PEZ)和RLPrompt。我们在GPT-2上的实验展示了可训练提示的可解释性与任务性能之间的基本权衡,揭示了软提示的可解释性问题的复杂性,并揭示了当优化一个可解释性的代理时出现的异常行为。
论文及项目相关链接
PDF 9 pages, 8 figures
Summary
本文介绍了软提示作为提高任务特定大型语言模型性能的一种廉价且简单的方法,但其作为自动化提示方法存在黑箱问题,缺乏与提示的直接联系。因此,作者提出了一个评估可训练提示解释性的新理论框架,包括忠实度和审查性两个要素。实验表明,现有方法无法满足作者提出的解释性标准,新框架启发了一种新的可训练提示方法,该方法明确优化了解释性。同时作者提出了面向两种先进提示调整器的新解释性目标函数,并通过GPT-2的实验验证了软提示解释性问题的基本权衡,展示了优化解释性代理时的异常行为。
Key Takeaways
- 软提示作为一种提高任务特定大型语言模型性能的方法受到欢迎,但其存在黑箱问题。
- 作者提出了一个评估可训练提示解释性的新理论框架,包括忠实度和审查性两个要素。
- 现有方法无法满足作者提出的解释性标准,需要新的方向来优化可训练提示方法。
- 作者提出了面向两种先进提示调整器的新解释性目标函数。
- 实验表明软提示存在解释性问题,优化解释性时存在权衡。
- 软提示的异常行为在优化解释性代理时更为明显。
点此查看论文截图
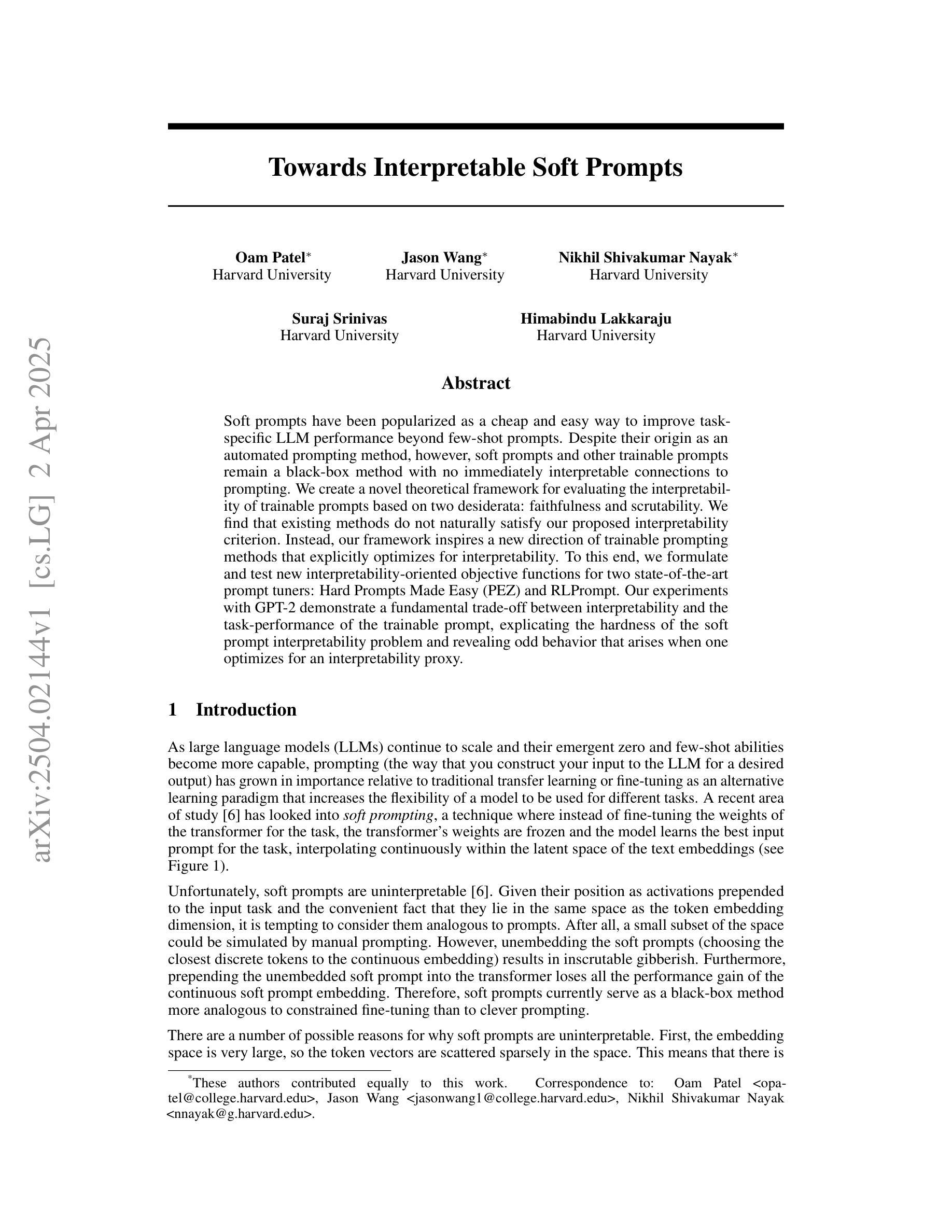
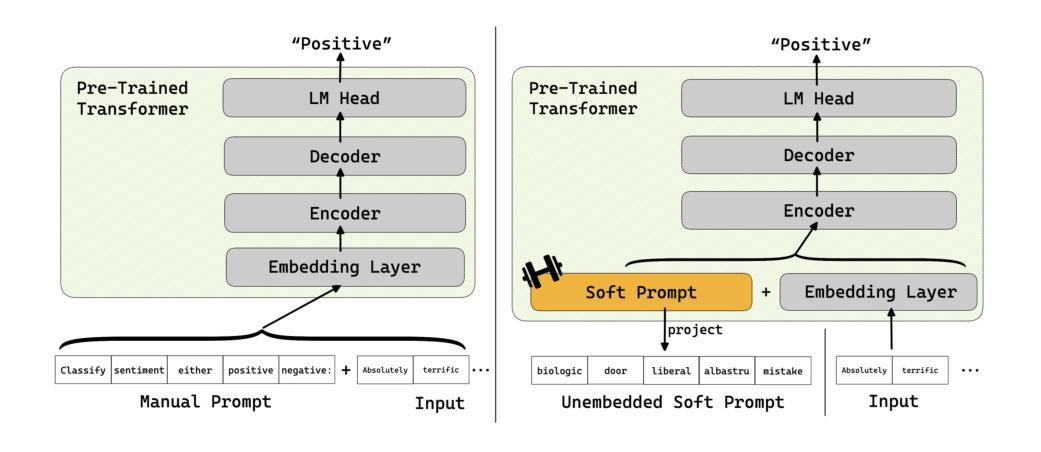
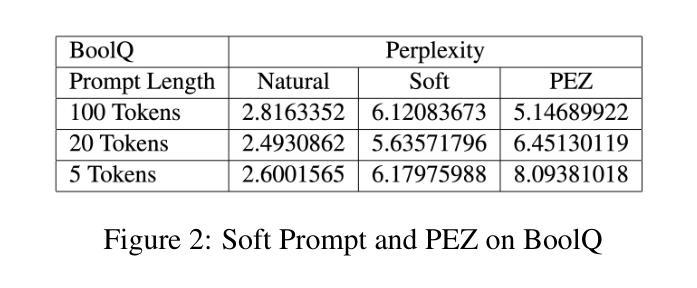
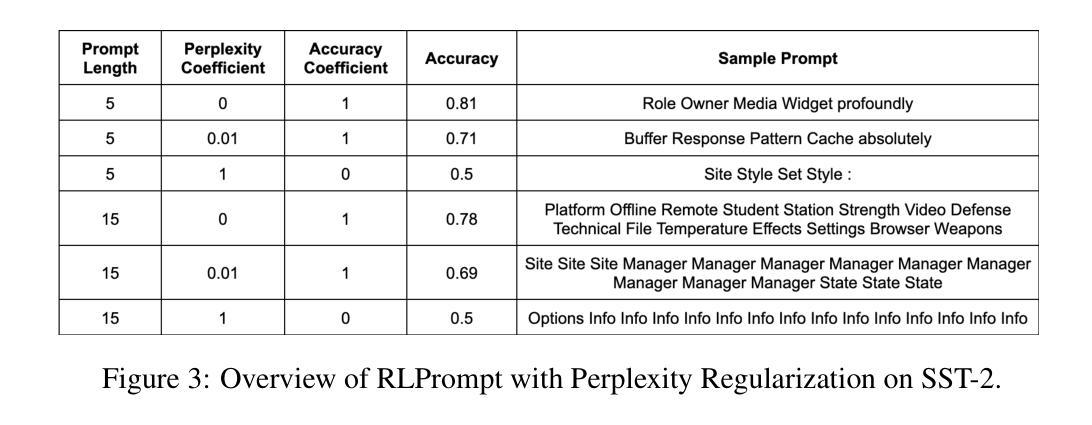

MageSQL: Enhancing In-context Learning for Text-to-SQL Applications with Large Language Models
Authors:Chen Shen, Jin Wang, Sajjadur Rahman, Eser Kandogan
The text-to-SQL problem aims to translate natural language questions into SQL statements to ease the interaction between database systems and end users. Recently, Large Language Models (LLMs) have exhibited impressive capabilities in a variety of tasks, including text-to-SQL. While prior works have explored various strategies for prompting LLMs to generate SQL statements, they still fall short of fully harnessing the power of LLM due to the lack of (1) high-quality contextual information when constructing the prompts and (2) robust feedback mechanisms to correct translation errors. To address these challenges, we propose MageSQL, a text-to-SQL approach based on in-context learning over LLMs. MageSQL explores a suite of techniques that leverage the syntax and semantics of SQL queries to identify relevant few-shot demonstrations as context for prompting LLMs. In particular, we introduce a graph-based demonstration selection method – the first of its kind in the text-to-SQL problem – that leverages graph contrastive learning adapted with SQL-specific data augmentation strategies. Furthermore, an error correction module is proposed to detect and fix potential inaccuracies in the generated SQL query. We conduct comprehensive evaluations on several benchmarking datasets. The results show that our proposed methods outperform state-of-the-art methods by an obvious margin.
文本到SQL的问题旨在将自然语言问题翻译成SQL语句,以简化数据库系统与最终用户之间的交互。最近,大型语言模型(LLM)在各种任务中表现出了令人印象深刻的能力,包括文本到SQL。虽然先前的工作已经探索了各种策略来提示LLM生成SQL语句,但由于(1)在构建提示时缺乏高质量上下文信息,以及(2)缺乏纠正翻译错误的稳健反馈机制,它们还没有充分利用LLM的威力。为了解决这些挑战,我们提出了MageSQL,这是一种基于LLM的上下文学习的文本到SQL方法。MageSQL探索了一系列技术,利用SQL查询的语法和语义来识别相关的小规模演示作为提示LLM的上下文。特别是,我们引入了一种基于图的演示选择方法——这是文本到SQL问题中的第一种——该方法利用与SQL特定的数据增强策略相适应的图对比学习。此外,还提出了一个错误修正模块,用于检测和修复生成的SQL查询中可能存在的错误。我们在几个基准数据集上进行了全面评估。结果表明,我们提出的方法明显优于最先进的方法。
论文及项目相关链接
Summary
基于自然语言的大型语言模型(LLM)在文本到SQL转换任务中展现出强大的能力,但仍面临缺乏高质量上下文信息和纠错机制的问题。本研究提出了一种基于LLM的上下文学习的新方法MageSQL,利用SQL查询的语法和语义,通过图形对比学习等技术选取相关示范作为上下文提示。同时,MageSQL还具备错误修正模块,可以检测和修复生成的SQL查询中的潜在错误。在多个基准数据集上的综合评估显示,该方法显著优于现有技术。
Key Takeaways
- 大型语言模型(LLM)在文本到SQL转换任务中表现出强大的能力。
- 当前研究面临缺乏高质量上下文信息和纠错机制的挑战。
- MageSQL是一种基于LLM的上下文学习新方法,旨在解决这些问题。
- MageSQL利用SQL查询的语法和语义,通过图形对比学习等技术选取相关示范作为上下文提示。
- MageSQL具备错误修正模块,可以检测和修复生成的SQL查询中的潜在错误。
- 在多个基准数据集上的综合评估显示,MageSQL显著优于现有技术。
点此查看论文截图
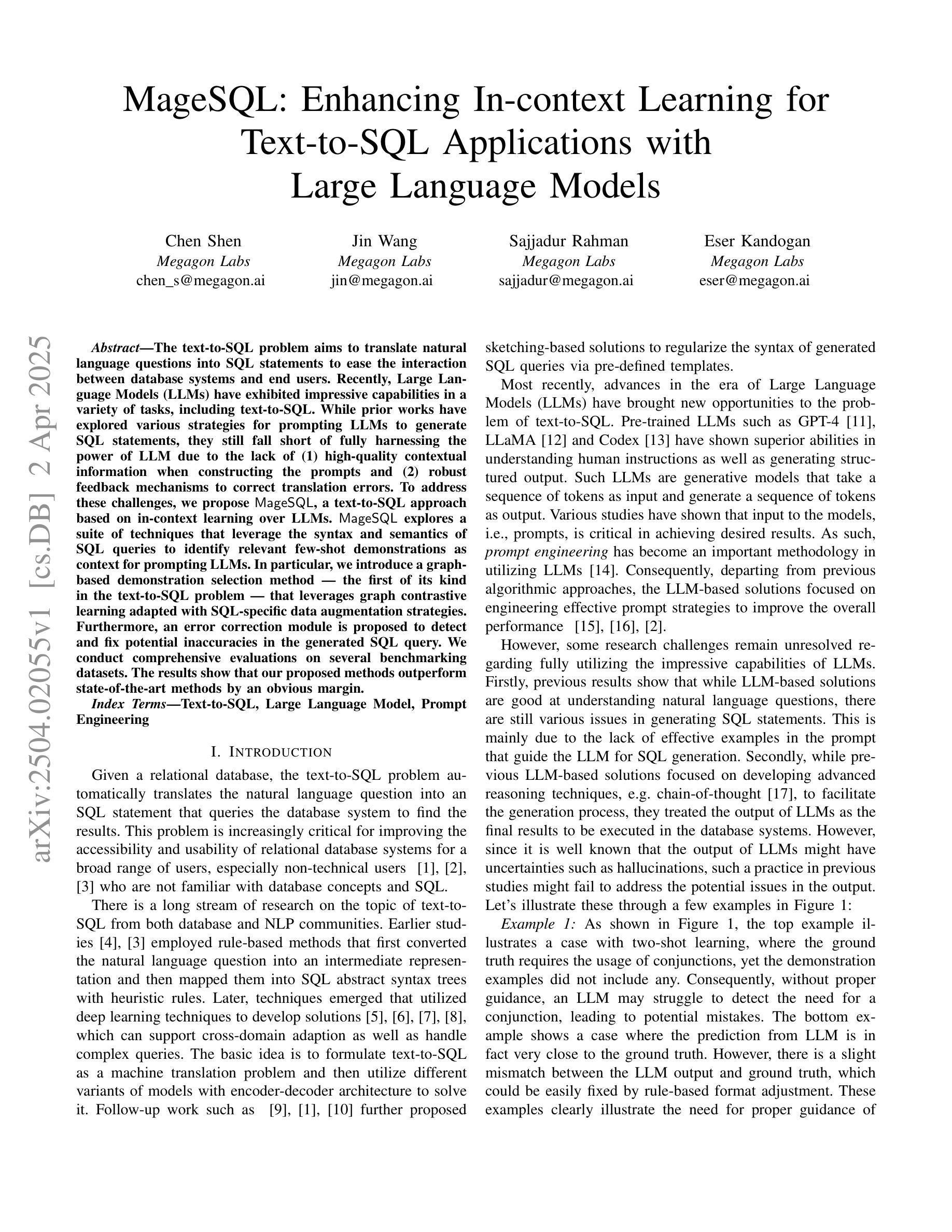
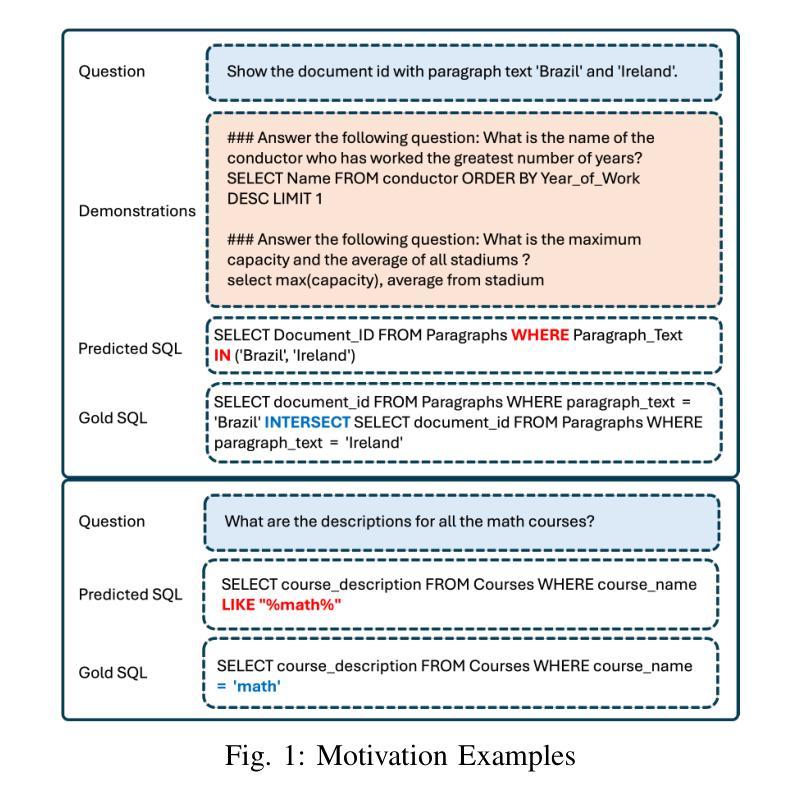
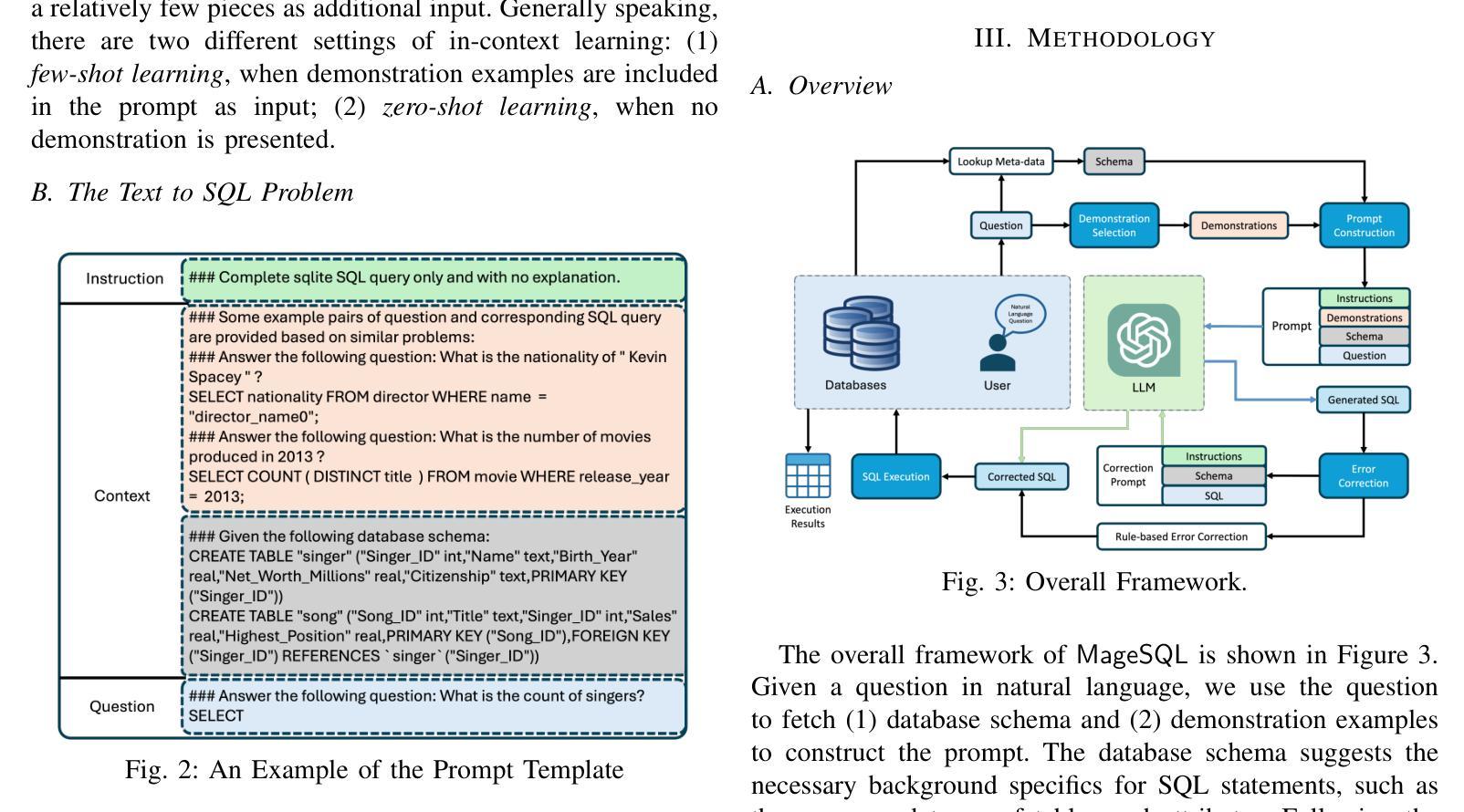
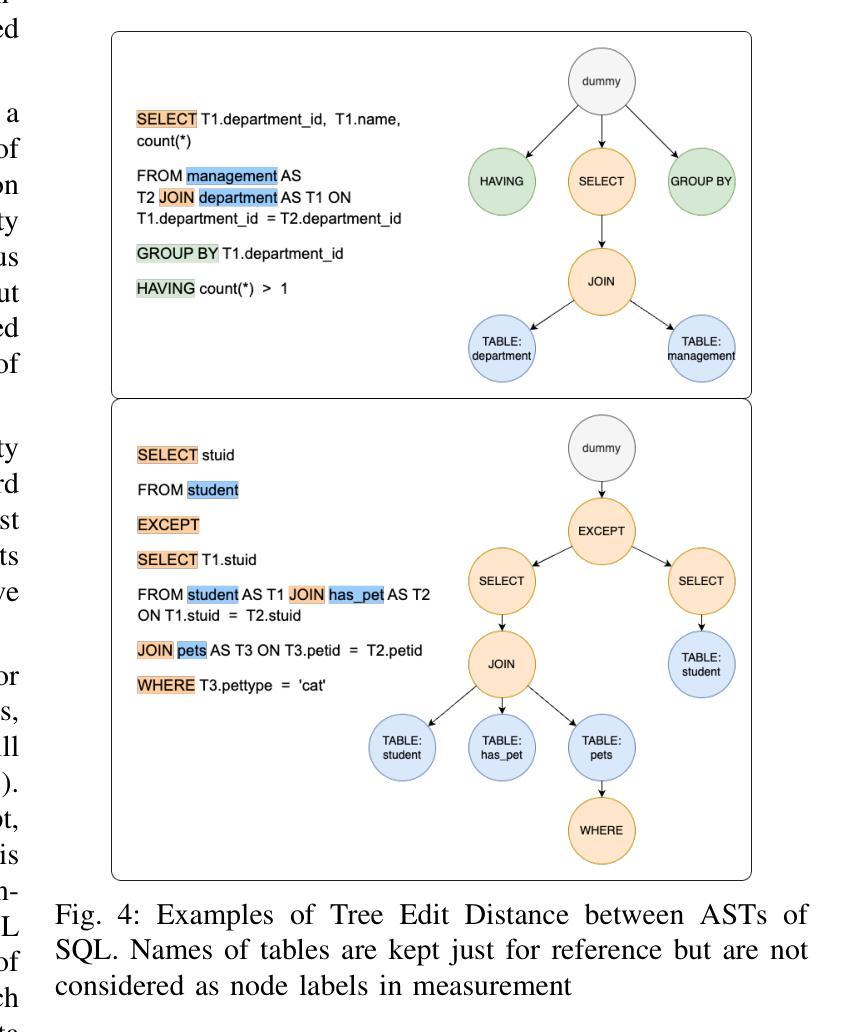
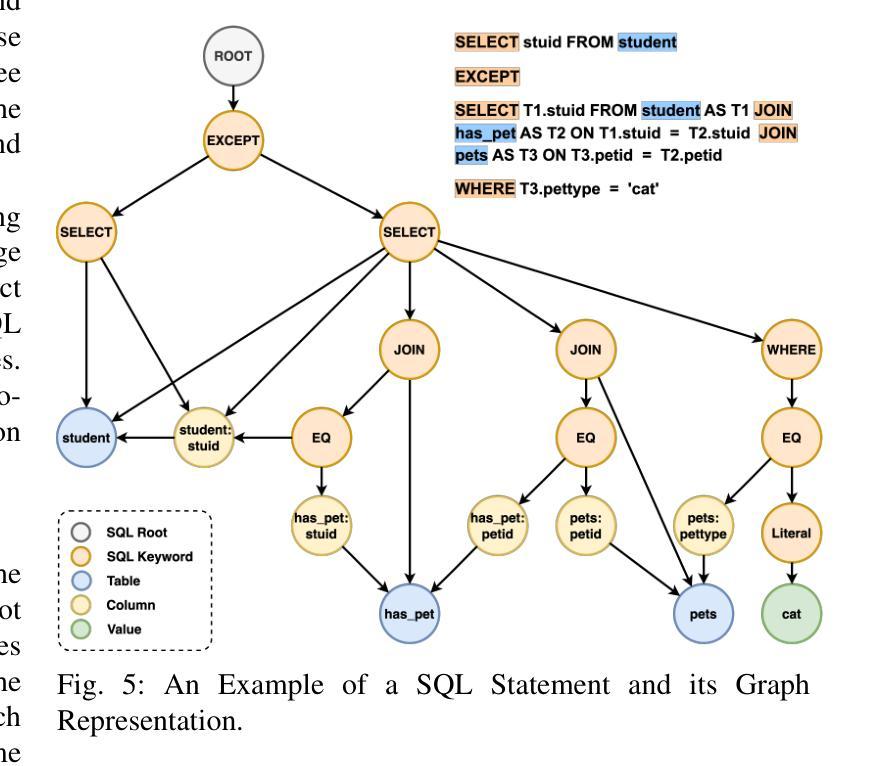
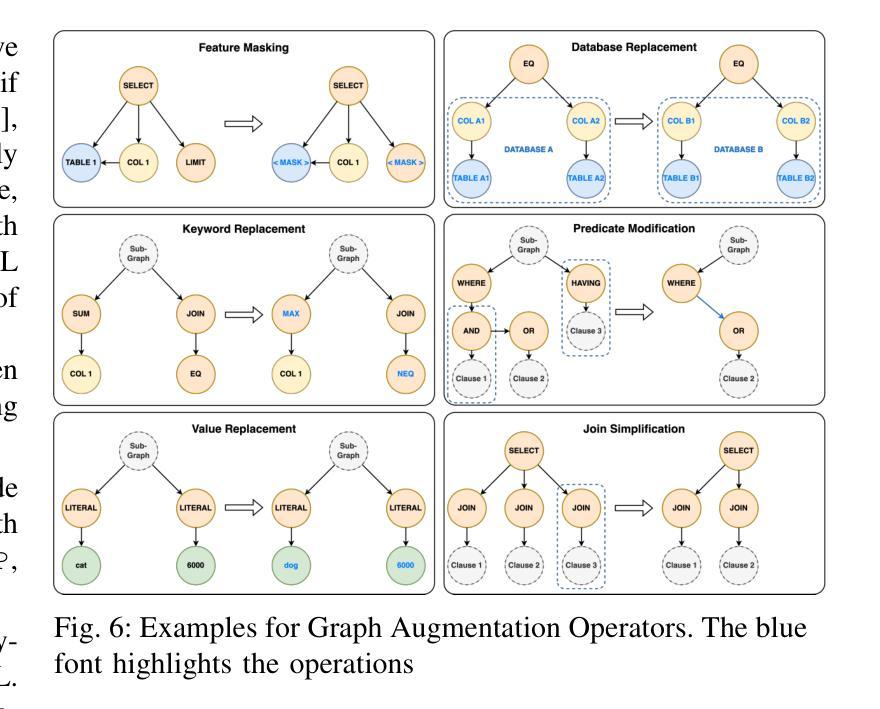
Model-Agnostic Meta-Learning for Fault Diagnosis of Induction Motors in Data-Scarce Environments with Varying Operating Conditions and Electric Drive Noise
Authors:Ali Pourghoraba, MohammadSadegh KhajueeZadeh, Ali Amini, Abolfazl Vahedi, Gholam Reza Agah, Akbar Rahideh
Reliable mechanical fault detection with limited data is crucial for the effective operation of induction machines, particularly given the real-world challenges present in industrial datasets, such as significant imbalances between healthy and faulty samples and the scarcity of data representing faulty conditions. This research introduces an innovative meta-learning approach to address these issues, focusing on mechanical fault detection in induction motors across diverse operating conditions while mitigating the adverse effects of drive noise in scenarios with limited data. The process of identifying faults under varying operating conditions is framed as a few-shot classification challenge and approached through a model-agnostic meta-learning strategy. Specifically, this approach begins with training a meta-learner across multiple interconnected fault-diagnosis tasks conducted under different operating conditions. In this stage, cross-entropy is utilized to optimize parameters and develop a robust representation of the tasks. Subsequently, the parameters of the meta-learner are fine-tuned for new tasks, enabling rapid adaptation using only a small number of samples. This method achieves excellent accuracy in fault detection across various conditions, even when data availability is restricted. The findings indicate that the proposed model outperforms other sophisticated techniques, providing enhanced generalization and quicker adaptation. The accuracy of fault diagnosis reaches a minimum of 99%, underscoring the model’s effectiveness for reliable fault identification.
在有限的条件下实现可靠的机械故障检测对于感应电机的有效运行至关重要,尤其是在面对工业数据集中的现实挑战时,如健康与故障样本之间的显著差异以及代表故障状况的数据稀缺。本研究引入了一种创新的元学习方法来解决这些问题,专注于在多种操作条件下对感应电机进行机械故障检测,同时减轻在数据有限的情况下驱动噪声的不利影响。在不同操作条件下识别故障的过程被构建为一个小样本分类挑战,并通过模型无关的元学习策略来解决。具体来说,该方法首先在一个由多个操作条件下的互联故障诊断任务组成的元学习器上进行训练。在这个阶段,利用交叉熵来优化参数并发展出任务的稳健表示。随后,元学习器的参数被微调以适应新任务,使用少量样本即可实现快速适应。即使在数据有限的情况下,该方法在各种条件下的故障检测中也实现了出色的准确性。研究结果表明,所提出的模型优于其他复杂技术,提供了增强的泛化能力和更快的适应速度。故障诊断的准确性至少达到99%,突显了该模型在可靠故障识别方面的有效性。
论文及项目相关链接
Summary
本研究引入了一种元学习的方法来解决有限的工业数据下的机械故障检测问题。通过模型无关的元学习策略,对在不同操作条件下的故障检测任务进行训练和学习。此模型不仅能实现不同操作条件下的可靠机械故障检测,并在数据量小的情况下减少噪声干扰的负面影响,还具有针对新任务的快速适应和卓越的诊断准确性。
Key Takeaways
- 研究背景强调了机械故障检测在工业环境中的重要性,特别是在数据有限的情况下。
- 提出了一种创新的元学习方法来解决机械故障检测问题。
- 方法涉及在多种操作条件下进行故障检测任务的训练和学习。
- 使用交叉熵优化参数,并开发稳健的任务表示。
- 模型可以通过微调快速适应新任务,即使只有少量样本。
- 故障检测准确率极高,最低达到99%,证明了模型的有效性。
点此查看论文截图
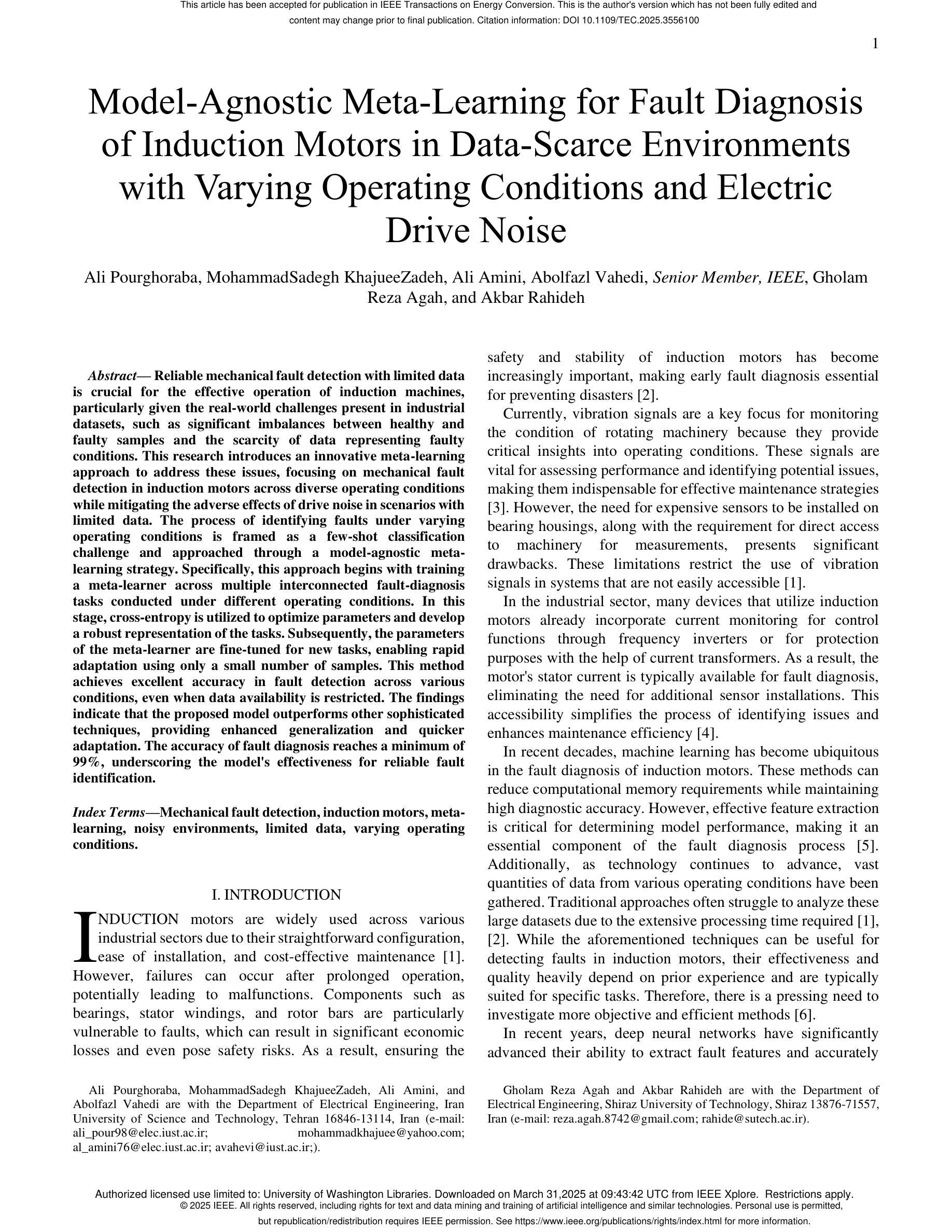
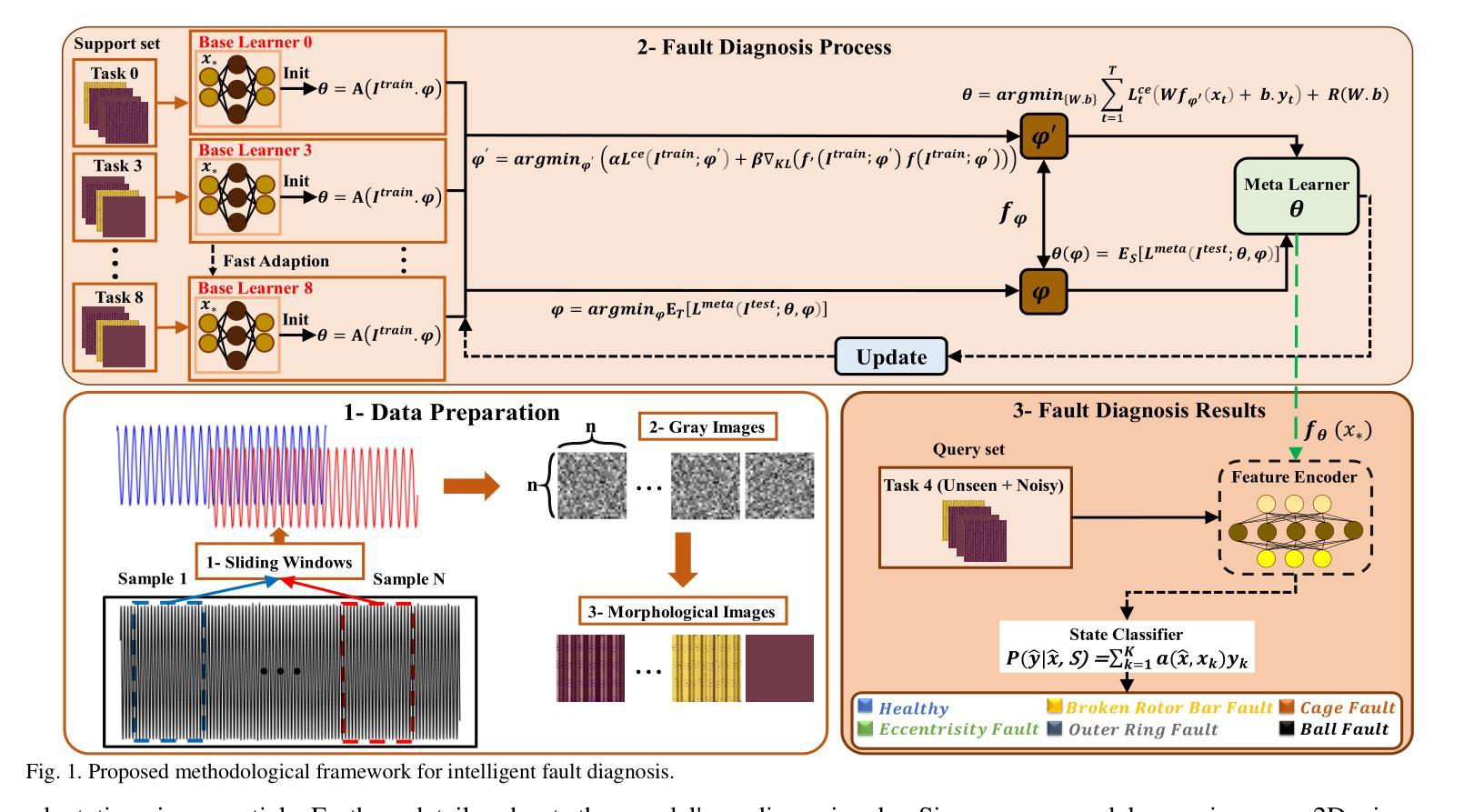
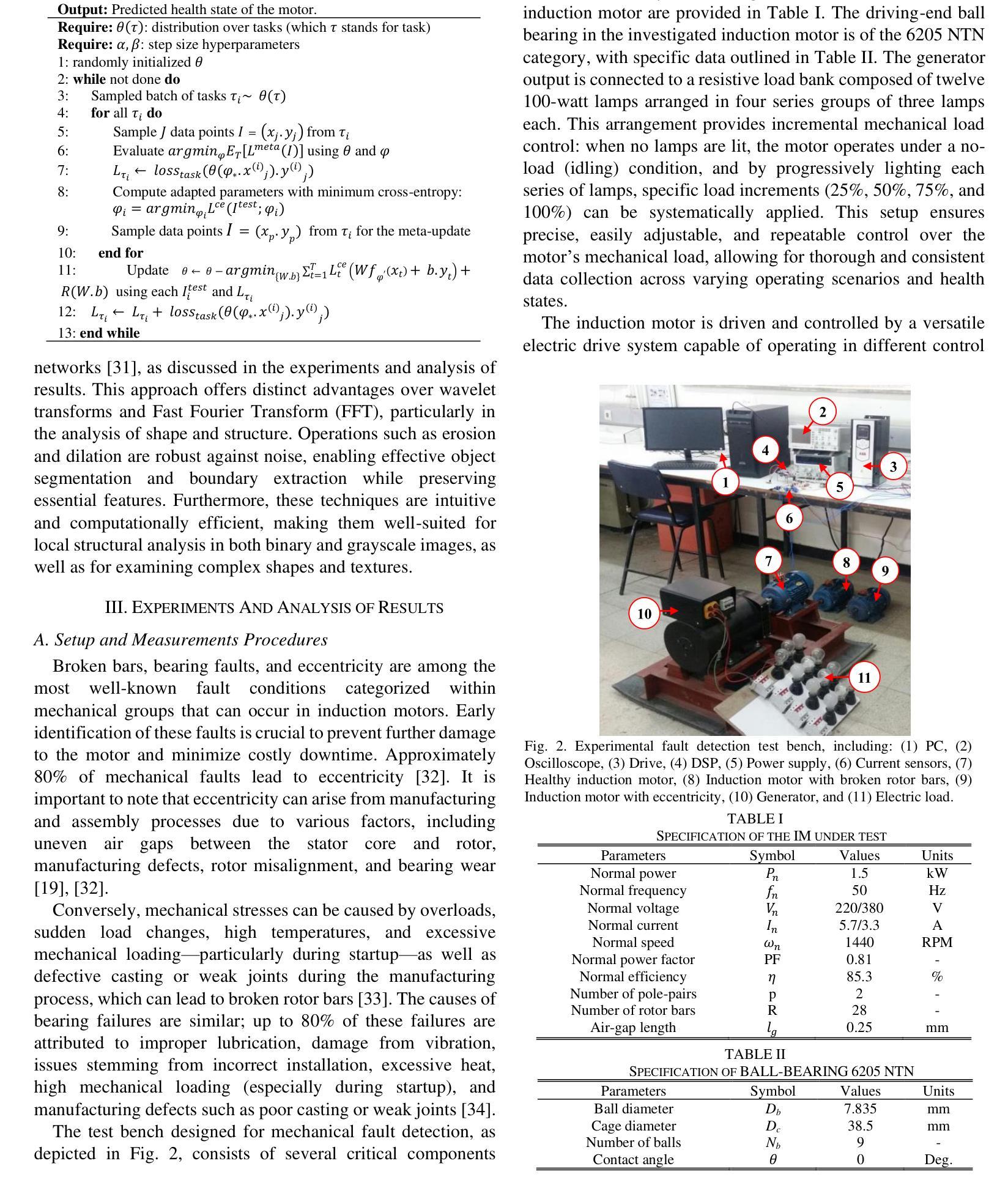
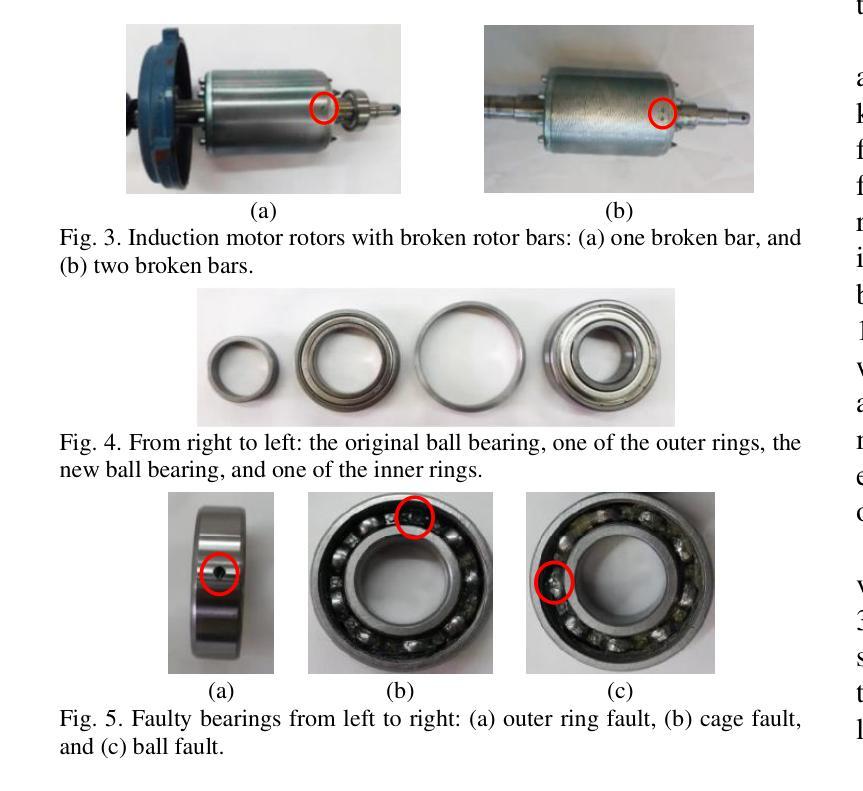
TAMT: Temporal-Aware Model Tuning for Cross-Domain Few-Shot Action Recognition
Authors:Yilong Wang, Zilin Gao, Qilong Wang, Zhaofeng Chen, Peihua Li, Qinghua Hu
Going beyond few-shot action recognition (FSAR), cross-domain FSAR (CDFSAR) has attracted recent research interests by solving the domain gap lying in source-to-target transfer learning. Existing CDFSAR methods mainly focus on joint training of source and target data to mitigate the side effect of domain gap. However, such kind of methods suffer from two limitations: First, pair-wise joint training requires retraining deep models in case of one source data and multiple target ones, which incurs heavy computation cost, especially for large source and small target data. Second, pre-trained models after joint training are adopted to target domain in a straightforward manner, hardly taking full potential of pre-trained models and then limiting recognition performance. To overcome above limitations, this paper proposes a simple yet effective baseline, namely Temporal-Aware Model Tuning (TAMT) for CDFSAR. Specifically, our TAMT involves a decoupled paradigm by performing pre-training on source data and fine-tuning target data, which avoids retraining for multiple target data with single source. To effectively and efficiently explore the potential of pre-trained models in transferring to target domain, our TAMT proposes a Hierarchical Temporal Tuning Network (HTTN), whose core involves local temporal-aware adapters (TAA) and a global temporal-aware moment tuning (GTMT). Particularly, TAA learns few parameters to recalibrate the intermediate features of frozen pre-trained models, enabling efficient adaptation to target domains. Furthermore, GTMT helps to generate powerful video representations, improving match performance on the target domain. Experiments on several widely used video benchmarks show our TAMT outperforms the recently proposed counterparts by 13%$\sim$31%, achieving new state-of-the-art CDFSAR results.
超越少样本动作识别(FSAR),跨域FSAR(CDFSAR)最近引起了研究兴趣,解决了源到目标迁移学习中的域差距问题。现有的CDFSAR方法主要集中在源和目标数据的联合训练,以减轻域差距的副作用。然而,这种方法存在两个局限性:首先,配对联合训练需要在源数据单一而目标数据多样的情况下重新训练深度模型,这带来了沉重的计算成本,特别是在源数据量大而目标数据量小的情况下。其次,联合训练后的预训练模型直接应用于目标域,很难充分利用预训练模型的潜力,从而限制了识别性能。为了克服上述局限性,本文提出了一种简单有效的基线方法,即用于CDFSAR的Temporal-Aware模型调整(TAMT)。具体来说,我们的TAMT通过源数据预训练和目标数据微调的方式实现了分离的模式,避免了使用单一源数据对多个目标数据进行重新训练。为了有效且高效地探索预训练模型转移到目标域的潜力,我们的TAMT提出了分层时间调整网络(HTTN),其核心包括局部时间感知适配器(TAA)和全局时间感知时刻调整(GTMT)。特别是,TAA学习少量参数以重新校准冻结的预训练模型的中间特征,使其能够高效地适应目标域。此外,GTMT有助于生成强大的视频表示,提高在目标域上的匹配性能。在几个广泛使用的视频基准测试上的实验表明,我们的TAMT相较于最近提出的同类产品,性能提高了13%~31%,取得了最新的CDFSAR结果。
论文及项目相关链接
PDF Accepted by CVPR 2025; Project page: https://github.com/TJU-YDragonW/TAMT
Summary
该文本介绍了跨域少样本动作识别(CDFSAR)的研究现状。现有方法主要通过联合训练源和目标数据来减少域差距的副作用,但存在计算成本高昂和利用预训练模型潜力不足的问题。为克服这些限制,本文提出了名为Temporal-Aware Model Tuning(TAMT)的基线方法。TAMT采用解耦范式,先在源数据进行预训练,再对目标数据进行微调,避免了为多个目标数据重新训练的需求。同时,TAMT提出了Hierarchical Temporal Tuning Network(HTTN),包括局部时间感知适配器(TAA)和全局时间感知时刻微调(GTMT)。TAA学习少量参数来重新校准冻结的预训练模型的中间特征,适应目标域。GTMT则有助于生成强大的视频表示,提高目标域的匹配性能。实验表明,TAMT相较于现有方法表现更优,取得了最新的CDFSAR结果。
Key Takeaways
- 跨域少样本动作识别(CDFSAR)是近期研究热点,旨在解决源到目标域转移学习中的域差距问题。
- 现有CDFSAR方法主要通过联合训练源和目标数据来减少域差距,但存在计算成本高和预训练模型潜力利用不足的问题。
- 本文提出的Temporal-Aware Model Tuning(TAMT)方法采用解耦范式,先预训练源数据,再微调目标数据,避免重训多个目标数据。
- TAMT的Hierarchical Temporal Tuning Network(HTTN)包括局部时间感知适配器(TAA)和全局时间感知时刻微调(GTMT),能更有效地探索预训练模型的潜力并适应目标域。
- TAA通过学习少量参数重新校准预训练模型的中间特征,适应目标域。
- GTMT有助于生成强大的视频表示,提高目标域的匹配性能。
点此查看论文截图
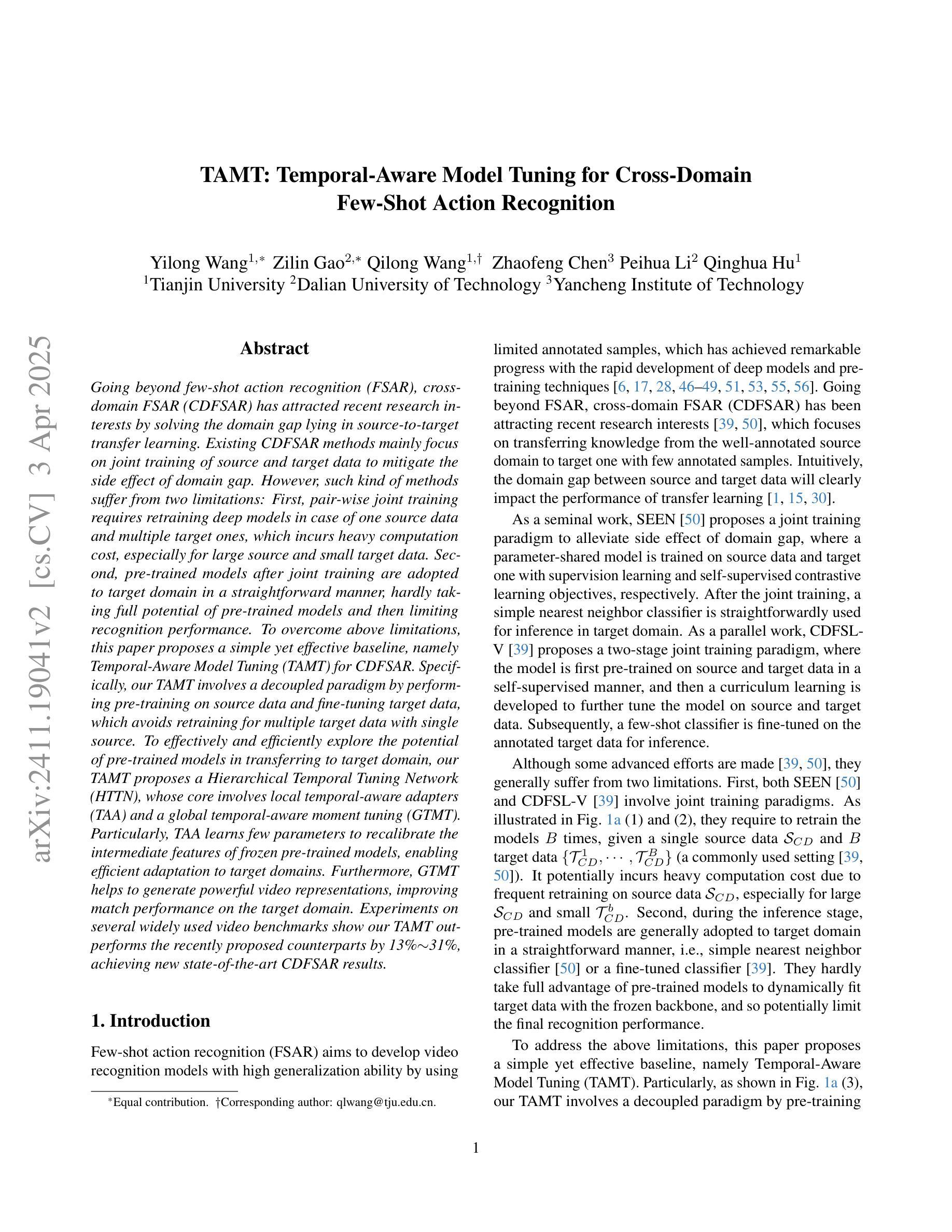
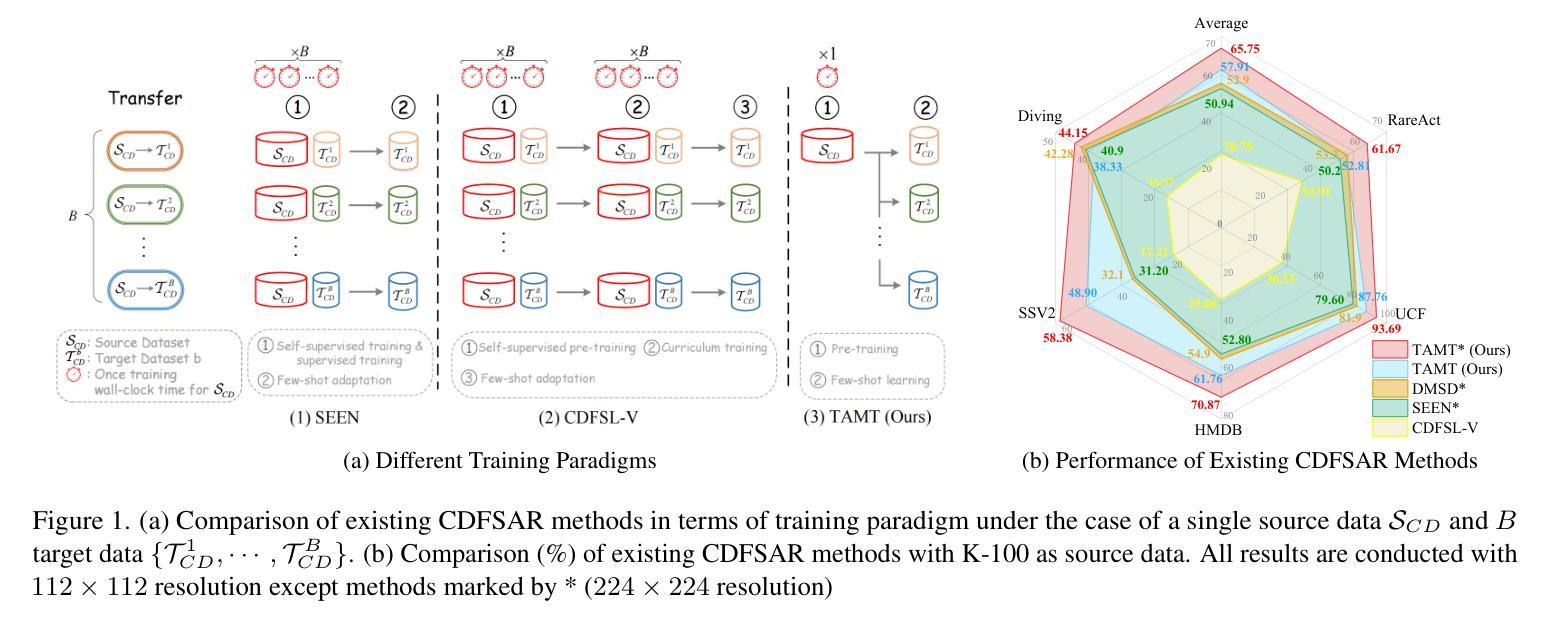
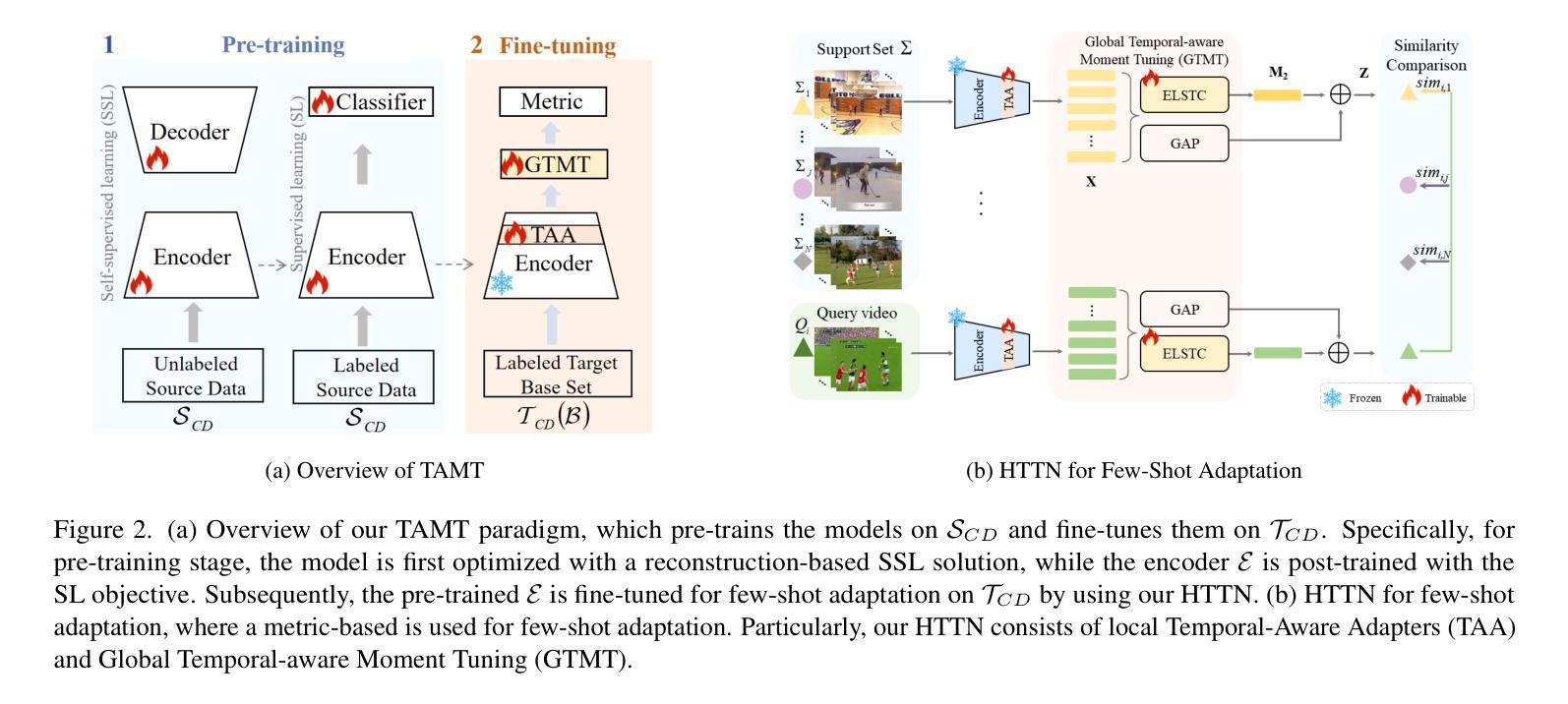
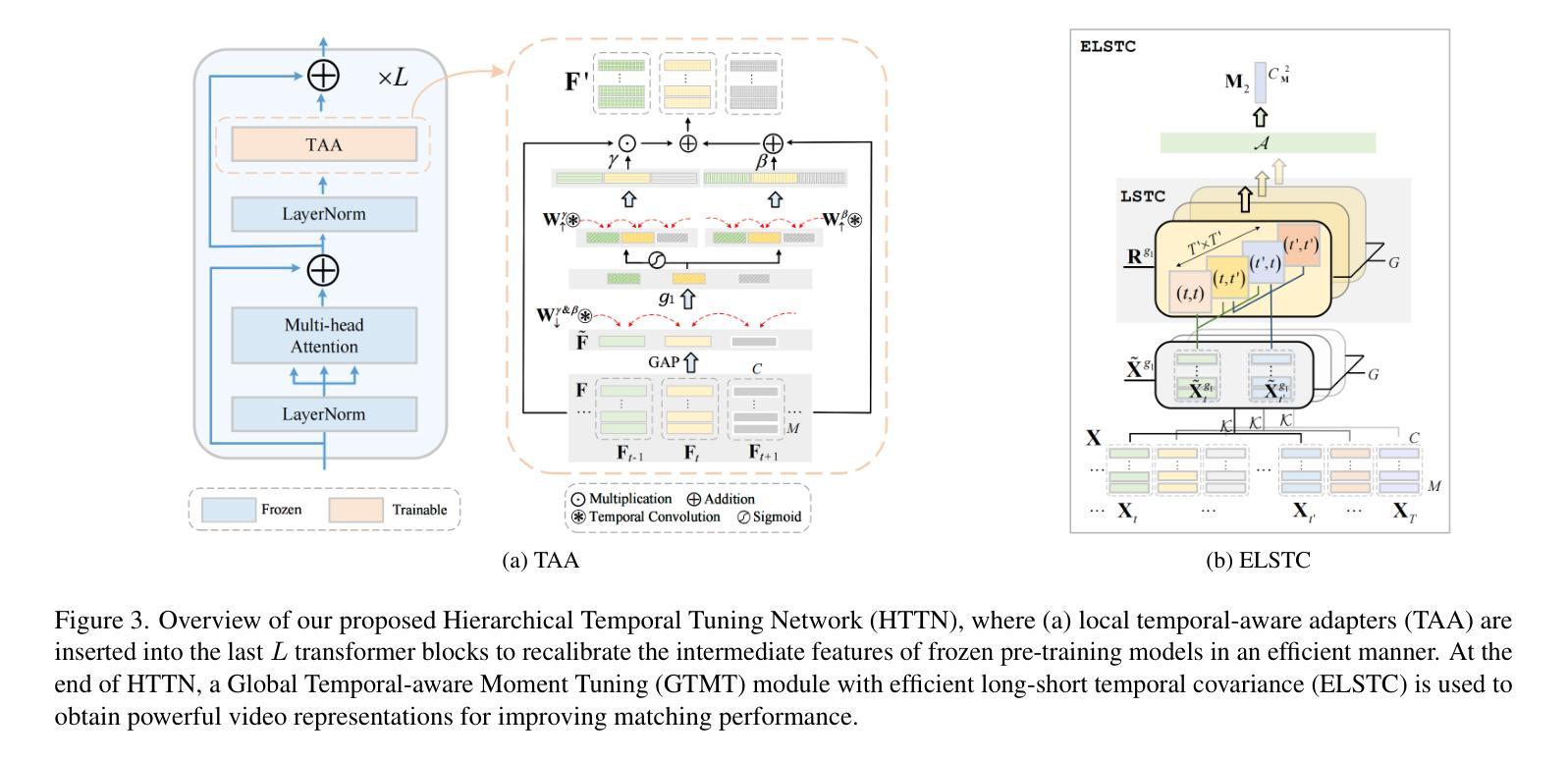
ICPL: Few-shot In-context Preference Learning via LLMs
Authors:Chao Yu, Qixin Tan, Hong Lu, Jiaxuan Gao, Xinting Yang, Yu Wang, Yi Wu, Eugene Vinitsky
Preference-based reinforcement learning is an effective way to handle tasks where rewards are hard to specify but can be exceedingly inefficient as preference learning is often tabula rasa. We demonstrate that Large Language Models (LLMs) have native preference-learning capabilities that allow them to achieve sample-efficient preference learning, addressing this challenge. We propose In-Context Preference Learning (ICPL), which uses in-context learning capabilities of LLMs to reduce human query inefficiency. ICPL uses the task description and basic environment code to create sets of reward functions which are iteratively refined by placing human feedback over videos of the resultant policies into the context of an LLM and then requesting better rewards. We first demonstrate ICPL’s effectiveness through a synthetic preference study, providing quantitative evidence that it significantly outperforms baseline preference-based methods with much higher performance and orders of magnitude greater efficiency. We observe that these improvements are not solely coming from LLM grounding in the task but that the quality of the rewards improves over time, indicating preference learning capabilities. Additionally, we perform a series of real human preference-learning trials and observe that ICPL extends beyond synthetic settings and can work effectively with humans-in-the-loop.
基于偏好的强化学习是处理奖励难以明确指定的任务的一种有效方法,但其效率低下的主要原因是偏好学习往往是空白的。我们证明大型语言模型(LLM)具有天生的偏好学习能力,允许它们实现高效的样本偏好学习,从而应对这一挑战。我们提出了上下文偏好学习(ICPL),它利用LLM的上下文学习能力来减少人类查询的不效率。ICPL使用任务描述和基本环境代码来创建奖励函数集,通过放置人类反馈在结果政策的视频上,将其置于LLM的语境中,然后进行更好的奖励,从而迭代优化这些函数集。我们首先通过一个合成偏好研究来证明ICPL的有效性,定量证据表明它显著优于基于偏好的基准方法,具有更高的性能和更高的效率。我们发现这些改进不仅来自LLM在任务中的基础,而且随着时间的推移奖励的质量有所提高,这显示了其偏好学习能力。此外,我们还进行了一系列真实的人类偏好学习试验,并观察到ICPL超出合成设置,可以有效地与人类合作。
论文及项目相关链接
Summary
大语言模型(LLM)具有内在偏好学习能力,可实现样本高效的偏好学习,解决了奖励难以指定的问题。我们提出基于上下文偏好学习(ICPL),利用LLM的上下文学习能力减少人类查询的不效率。ICPL利用任务描述和基本环境代码来创建奖励函数集,通过向LLM提供视频的反馈来改善奖励。在合成偏好研究中证明ICPL的有效性显示其在性能上明显优于基于偏好的基准方法,并具有更高的效率。此外,我们还发现奖励质量随时间提高,表明偏好学习能力的重要性。同时,我们在真实的人类偏好学习试验中发现ICPL不仅适用于合成设置,还能有效地与人类合作。
Key Takeaways
- 大语言模型(LLM)具有内在的偏好学习能力。
- ICPL利用LLM进行样本高效的偏好学习,解决了奖励难以指定的问题。
- ICPL使用任务描述和基本环境代码来创建奖励函数集,并利用人类反馈改善奖励函数。
- 合成偏好研究显示ICPL性能优于基准方法,并具有更高的效率。
- ICPL的奖励质量随时间提高,显示了其偏好学习能力的重要性。
- ICPL在真实的人类偏好学习试验中被证明是有效的。
点此查看论文截图
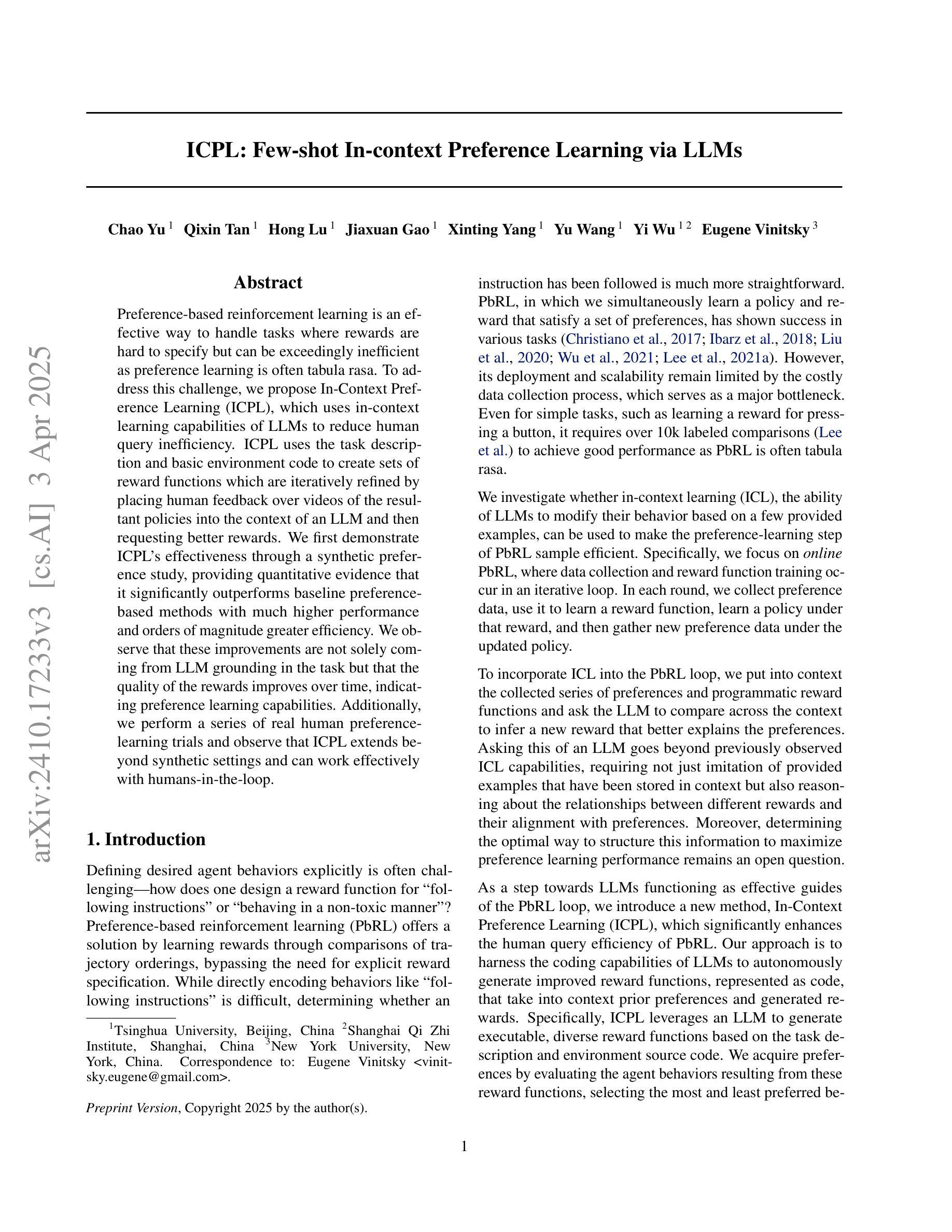
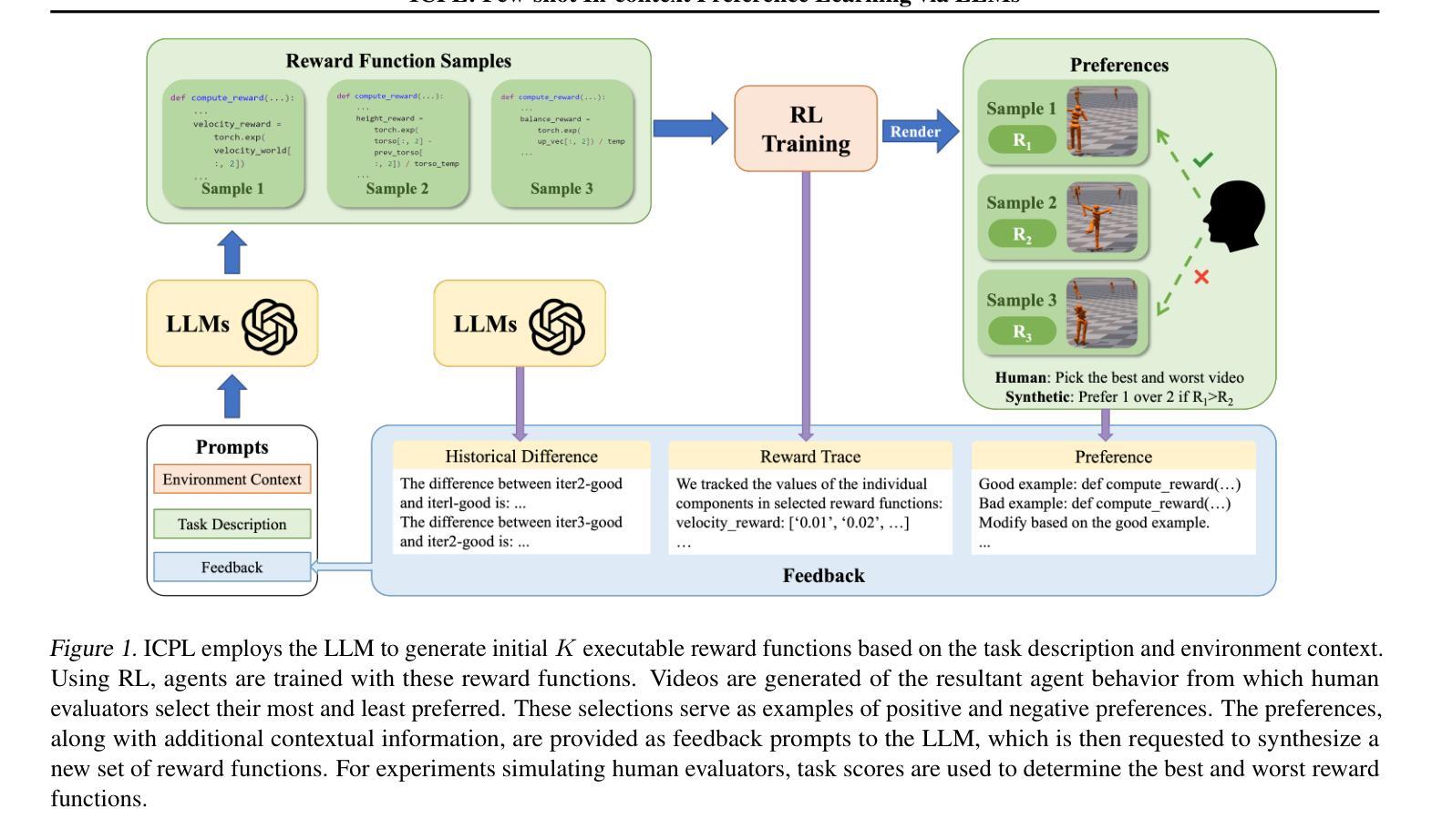
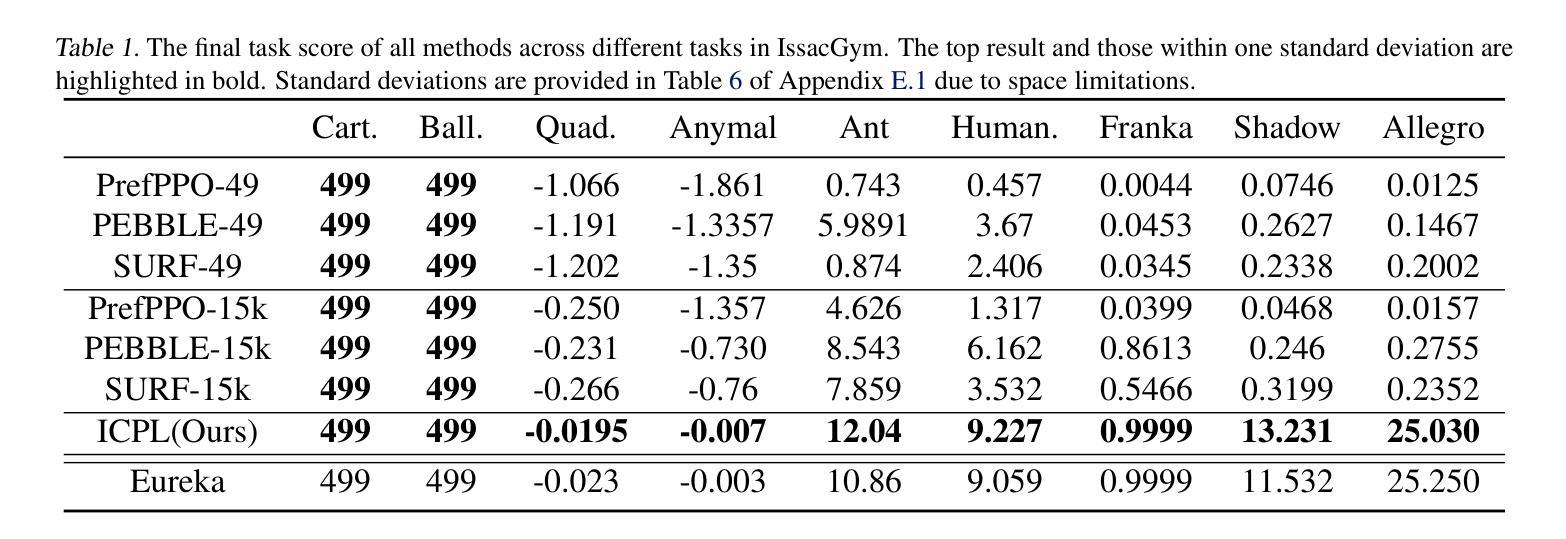