⚠️ 以下所有内容总结都来自于 大语言模型的能力,如有错误,仅供参考,谨慎使用
🔴 请注意:千万不要用于严肃的学术场景,只能用于论文阅读前的初筛!
💗 如果您觉得我们的项目对您有帮助 ChatPaperFree ,还请您给我们一些鼓励!⭐️ HuggingFace免费体验
2025-04-05 更新
F5R-TTS: Improving Flow Matching based Text-to-Speech with Group Relative Policy Optimization
Authors:Xiaohui Sun, Ruitong Xiao, Jianye Mo, Bowen Wu, Qun Yu, Baoxun Wang
We present F5R-TTS, a novel text-to-speech (TTS) system that integrates Gradient Reward Policy Optimization (GRPO) into a flow-matching based architecture. By reformulating the deterministic outputs of flow-matching TTS into probabilistic Gaussian distributions, our approach enables seamless integration of reinforcement learning algorithms. During pretraining, we train a probabilistically reformulated flow-matching based model which is derived from F5-TTS with an open-source dataset. In the subsequent reinforcement learning (RL) phase, we employ a GRPO-driven enhancement stage that leverages dual reward metrics: word error rate (WER) computed via automatic speech recognition and speaker similarity (SIM) assessed by verification models. Experimental results on zero-shot voice cloning demonstrate that F5R-TTS achieves significant improvements in both speech intelligibility (relatively 29.5% WER reduction) and speaker similarity (relatively 4.6% SIM score increase) compared to conventional flow-matching based TTS systems. Audio samples are available at https://frontierlabs.github.io/F5R.
我们提出了F5R-TTS,这是一种新型文本到语音(TTS)系统,它将梯度奖励策略优化(GRPO)集成到基于流匹配的架构中。我们通过将基于流匹配的TTS的确定性输出重新制定为概率高斯分布,我们的方法实现了强化学习算法的无缝集成。在预训练阶段,我们使用开源数据集对基于概率重构的流匹配模型进行了训练,该模型来源于F5-TTS。在随后的强化学习(RL)阶段,我们采用GRPO驱动的增强阶段,利用双重奖励指标:通过自动语音识别计算的词错误率(WER)和通过验证模型评估的说话人相似性(SIM)。在零样本语音克隆上的实验结果表明,F5R-TTS在语音清晰度(相对降低29.5%的WER)和说话人相似性(相对增加4.6%的SIM得分)方面均取得了显著的改进,与传统的基于流匹配的TTS系统相比。音频样本可在https://frontierlabs.github.io/F5R上找到。
论文及项目相关链接
Summary
本文介绍了F5R-TTS,这是一种新型文本转语音(TTS)系统,它将梯度奖励策略优化(GRPO)集成到基于流匹配的架构中。通过改革确定性输出为概率高斯分布,实现了强化学习算法的无缝集成。实验结果表明,与常规流匹配TTS系统相比,F5R-TTS在语音清晰度(相对降低29.5%的单词错误率)和说话人相似性(相对提高4.6%的相似度得分)方面取得了显著改进。
Key Takeaways
- F5R-TTS是一种新型的文本转语音(TTS)系统,结合了流匹配架构和梯度奖励策略优化(GRPO)。
- 通过将确定性输出改革为概率高斯分布,F5R-TTS实现了强化学习算法的无缝集成。
- F5R-TTS在预训练阶段使用概率改革后的流匹配模型,该模型基于开源数据集F5-TTS派生。
- 在强化学习阶段,F5R-TTS采用GRPO驱动的增强阶段,利用双重奖励指标:通过自动语音识别计算的字错误率和通过验证模型评估的说话人相似性。
- 实验结果证明,F5R-TTS在语音清晰度方面有显著改进,相对降低了29.5%的字错误率。
- F5R-TTS在说话人相似性方面也有显著进步,相对提高了4.6%的相似度得分。
点此查看论文截图
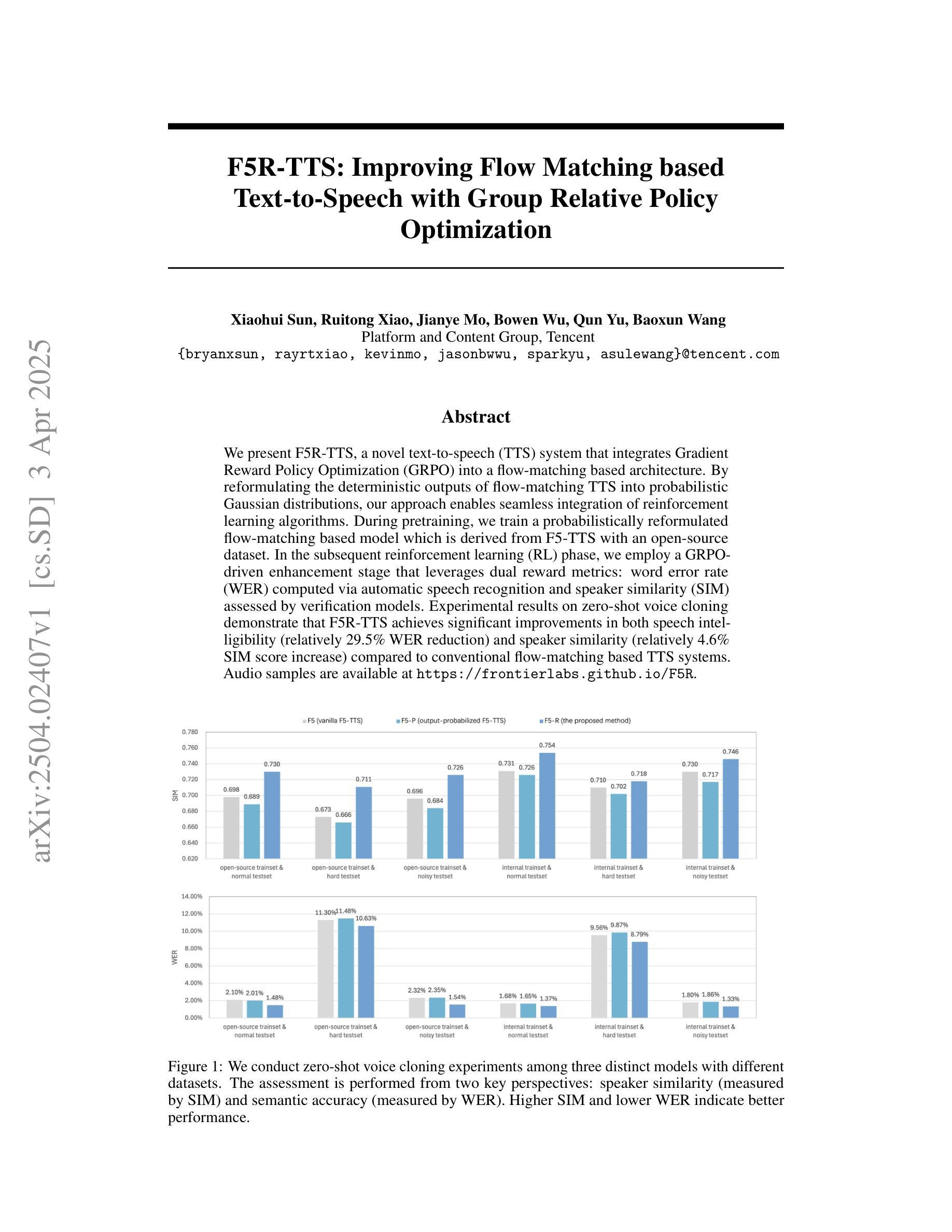
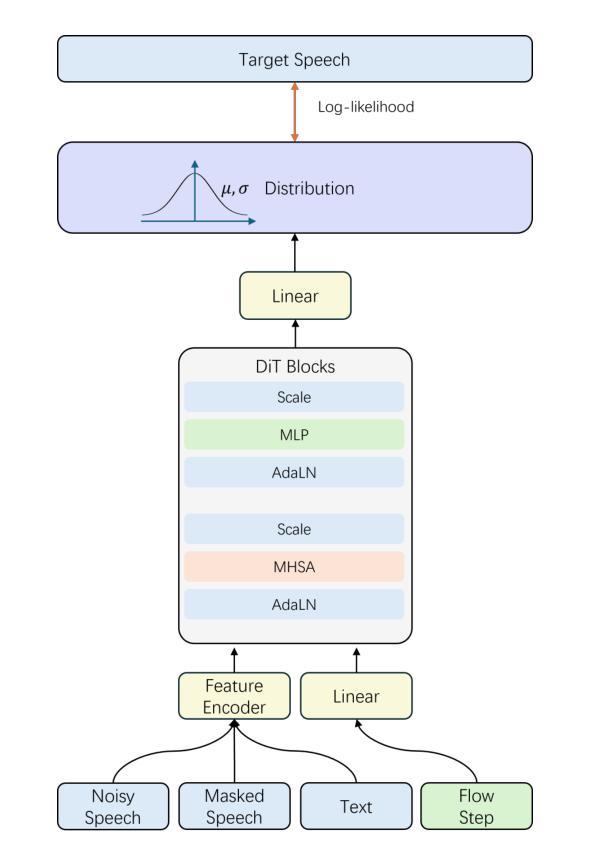
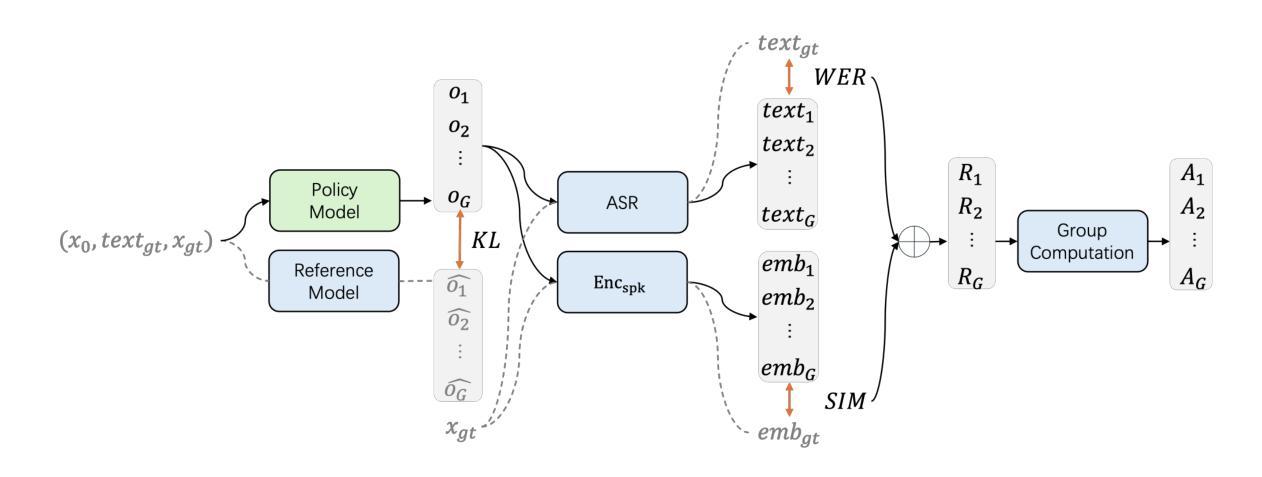
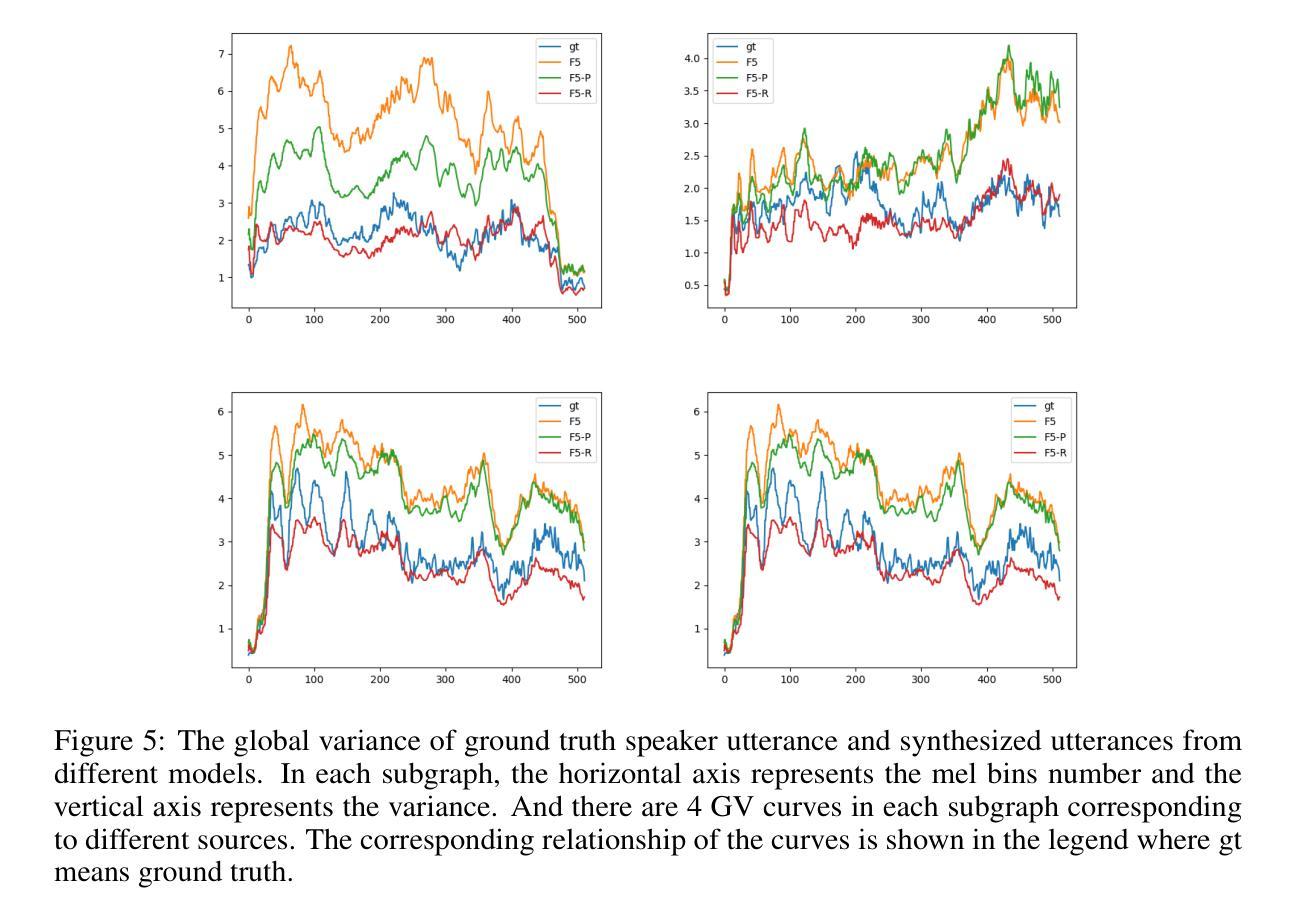
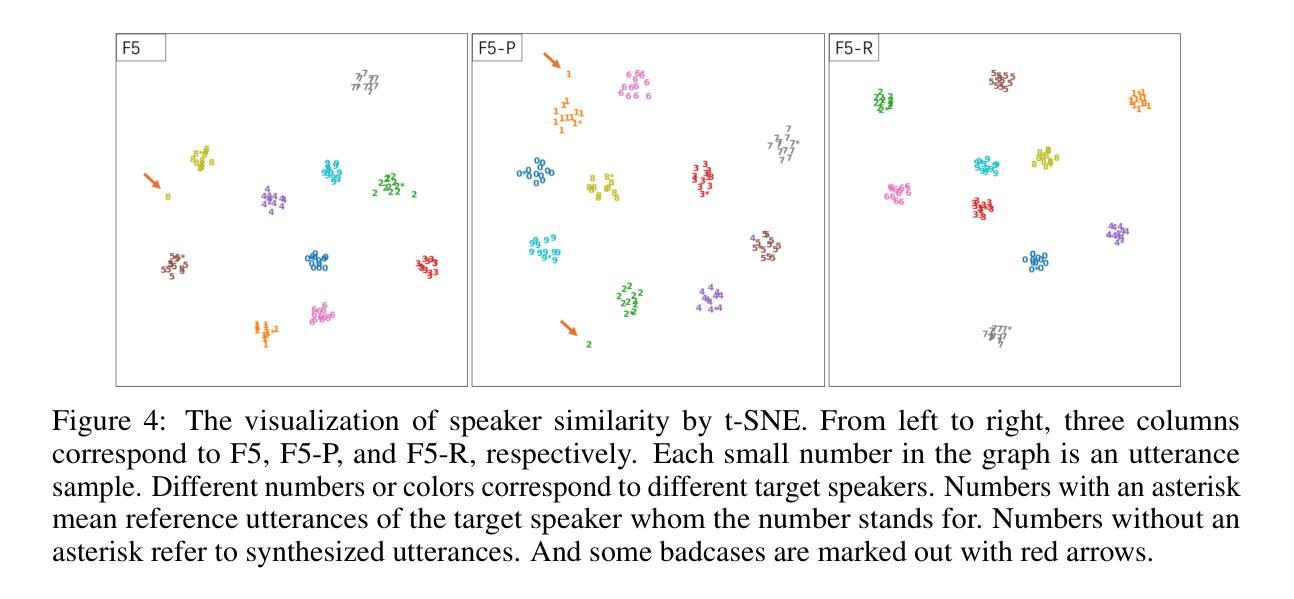
SocialGesture: Delving into Multi-person Gesture Understanding
Authors:Xu Cao, Pranav Virupaksha, Wenqi Jia, Bolin Lai, Fiona Ryan, Sangmin Lee, James M. Rehg
Previous research in human gesture recognition has largely overlooked multi-person interactions, which are crucial for understanding the social context of naturally occurring gestures. This limitation in existing datasets presents a significant challenge in aligning human gestures with other modalities like language and speech. To address this issue, we introduce SocialGesture, the first large-scale dataset specifically designed for multi-person gesture analysis. SocialGesture features a diverse range of natural scenarios and supports multiple gesture analysis tasks, including video-based recognition and temporal localization, providing a valuable resource for advancing the study of gesture during complex social interactions. Furthermore, we propose a novel visual question answering (VQA) task to benchmark vision language models’(VLMs) performance on social gesture understanding. Our findings highlight several limitations of current gesture recognition models, offering insights into future directions for improvement in this field. SocialGesture is available at huggingface.co/datasets/IrohXu/SocialGesture.
先前关于人类动作识别的研究在很大程度上忽视了多人交互,这对于理解自然发生的动作的社会背景至关重要。现有数据集的这个局限性给将人类动作与其他模式(如语言和语音)对齐带来了很大的挑战。为了解决这个问题,我们引入了SocialGesture数据集,这是专门为多人动作分析设计的第一个大规模数据集。SocialGesture涵盖了各种各样的自然场景,支持多个动作分析任务,包括基于视频的识别和时序定位,为在复杂的社交互动中研究动作提供了一个宝贵的资源。此外,我们还提出了一种新的视觉问答(VQA)任务,以评估视觉语言模型在社交动作理解方面的性能。我们的研究结果突出了当前动作识别模型的几个局限性,为改进这一领域提供了深刻的见解。SocialGesture数据集可在huggingface.co/datasets/IrohXu/SocialGesture找到。
论文及项目相关链接
PDF CVPR 2025
Summary:
现有手势识别研究多忽略多人交互,这对于理解自然手势的社会背景是一大挑战。为解决此问题,推出SocialGesture数据集,专为多人手势分析设计。它包含多种自然场景,支持视频识别和时间定位等任务,并为复杂社交互动中的手势理解提供资源。此外,还提出新的视觉问答任务来评估视觉语言模型对社交手势的理解性能。SocialGesture在huggingface.co/datasets/IrohXu/SocialGesture可用。
Key Takeaways:
- 现有手势识别研究忽略了多人交互的重要性。
- SocialGesture数据集是专为多人手势分析设计的,包含了丰富的自然场景和多种任务。
- 该数据集支持视频手势识别的任务和时间定位任务。
- 提出了一个视觉问答任务来评估视觉语言模型对社交手势的理解性能。
- 当前的手势识别模型存在局限性,需要改进。
- SocialGesture数据集可用于推动手势识别研究的进步。
点此查看论文截图
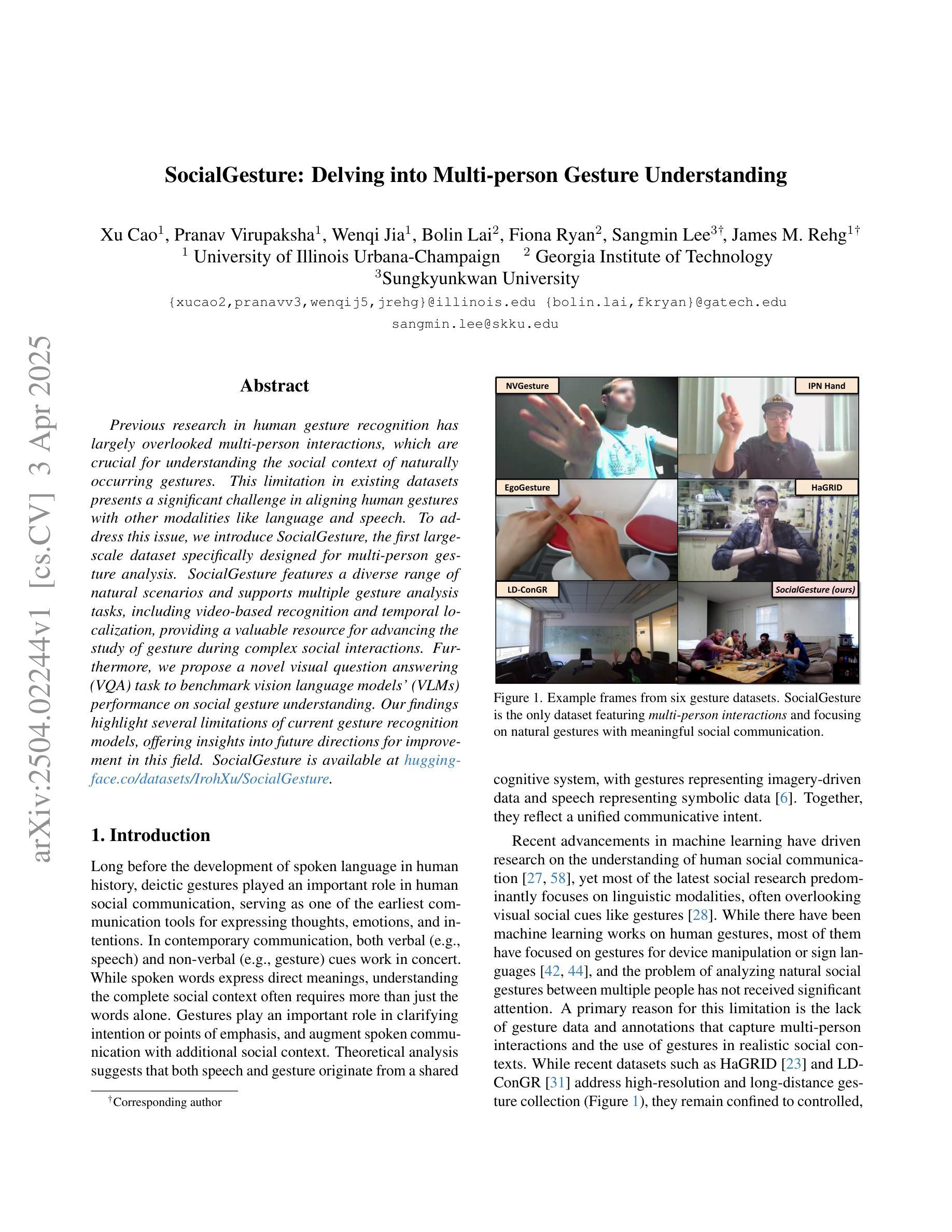
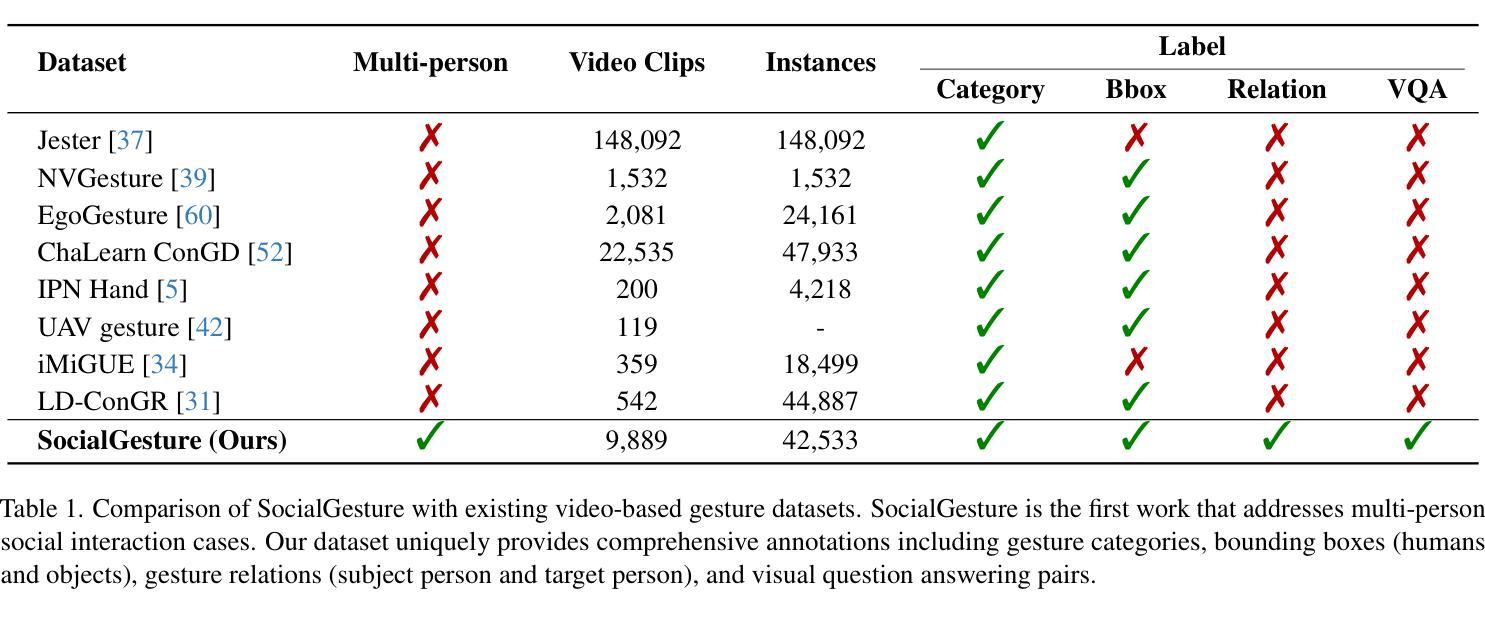
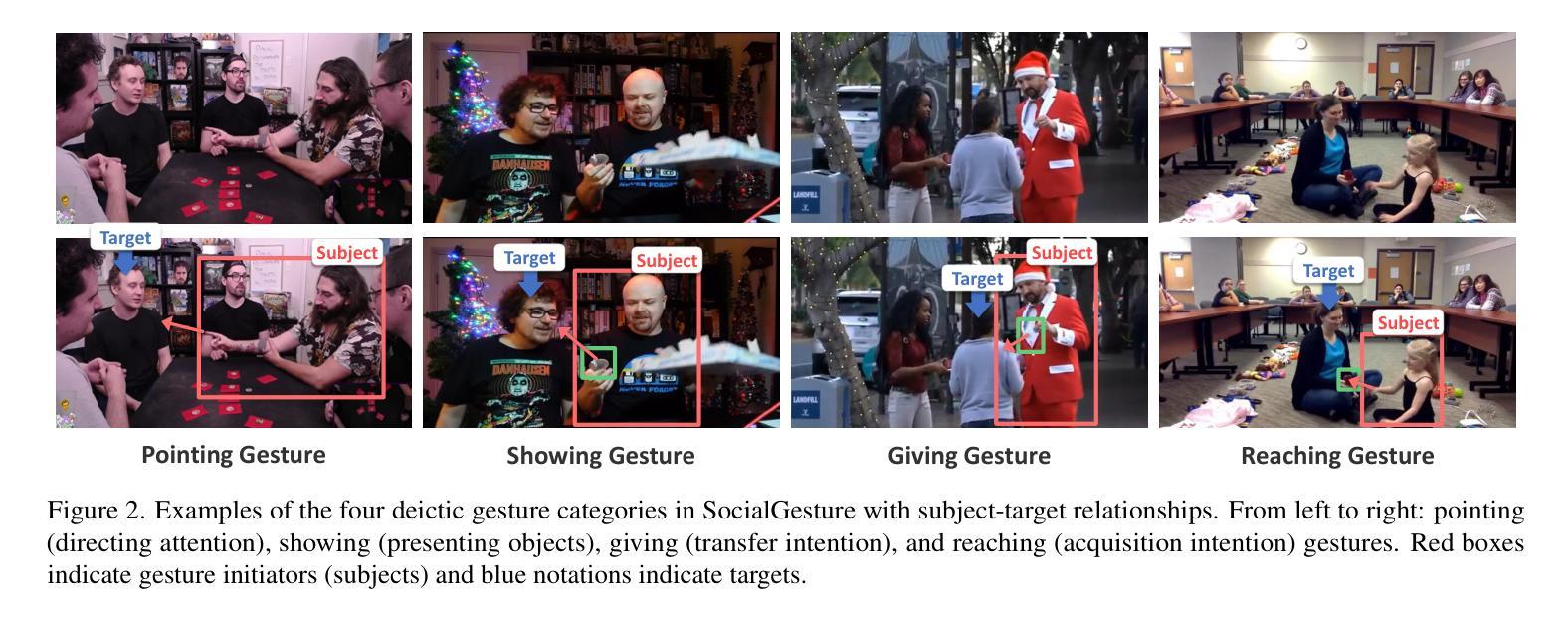
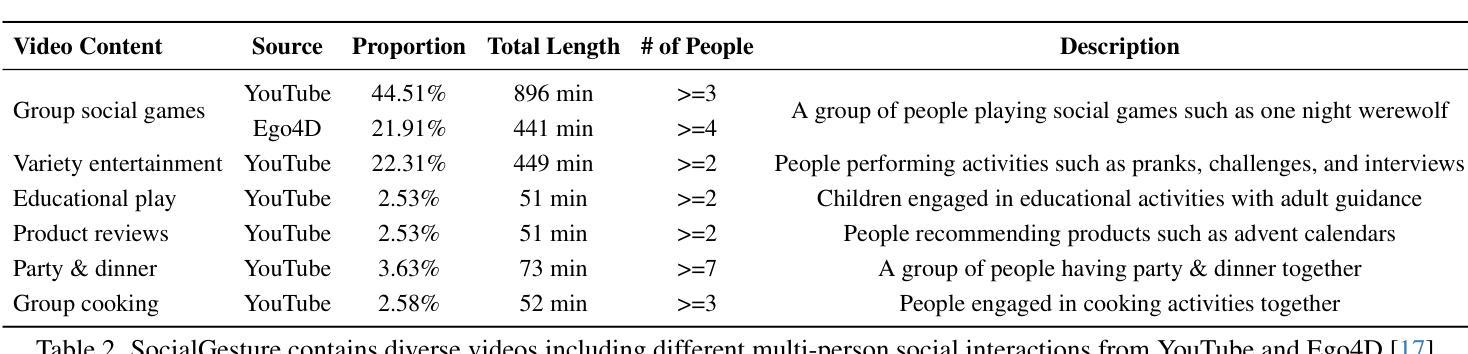
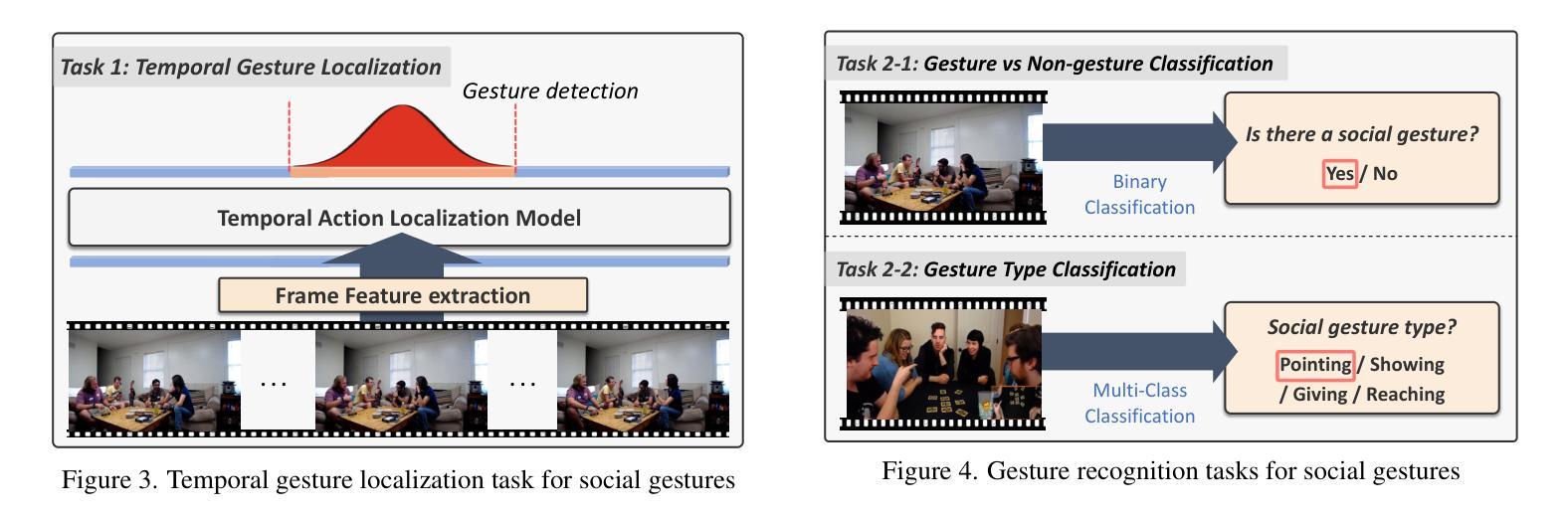
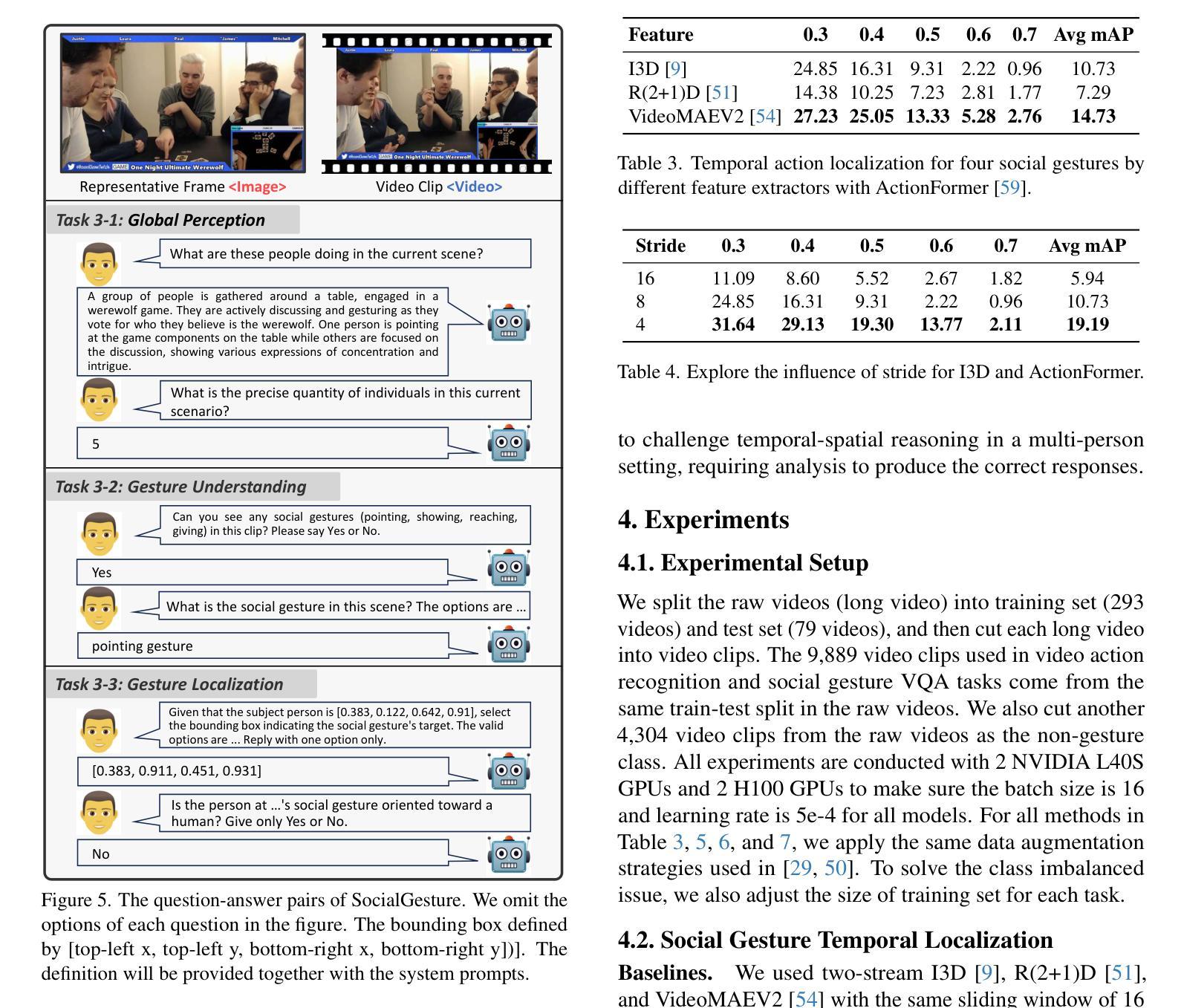
DuplexMamba: Enhancing Real-time Speech Conversations with Duplex and Streaming Capabilities
Authors:Xiangyu Lu, Wang Xu, Haoyu Wang, Hongyun Zhou, Haiyan Zhao, Conghui Zhu, Tiejun Zhao, Muyun Yang
Real-time speech conversation is essential for natural and efficient human-machine interactions, requiring duplex and streaming capabilities. Traditional Transformer-based conversational chatbots operate in a turn-based manner and exhibit quadratic computational complexity that grows as the input size increases. In this paper, we propose DuplexMamba, a Mamba-based end-to-end multimodal duplex model for speech-to-text conversation. DuplexMamba enables simultaneous input processing and output generation, dynamically adjusting to support real-time streaming. Specifically, we develop a Mamba-based speech encoder and adapt it with a Mamba-based language model. Furthermore, we introduce a novel duplex decoding strategy that enables DuplexMamba to process input and generate output simultaneously. Experimental results demonstrate that DuplexMamba successfully implements duplex and streaming capabilities while achieving performance comparable to several recently developed Transformer-based models in automatic speech recognition (ASR) tasks and voice assistant benchmark evaluations. Our code and model are released.
实时语音对话对于自然高效的人机交互至关重要,这需要双向和流式处理能力。传统的基于Transformer的对话聊天机器人以回合制的方式运行,表现出随着输入量增加而二次方的计算复杂性。在本文中,我们提出了基于Mamba的端到端双向模型DuplexMamba,用于语音到文本的对话。DuplexMamba可以同时对输入进行处理并生成输出,动态调整以支持实时流式传输。具体来说,我们开发了基于Mamba的语音编码器并将其与基于Mamba的语言模型相结合。此外,我们引入了一种新型的双向解码策略,使DuplexMamba能够同时处理输入并生成输出。实验结果表明,DuplexMamba成功实现了双向和流式处理能力,同时在自动语音识别(ASR)任务和语音助手基准评估中实现了与最近开发的几个基于Transformer的模型相当的性能。我们的代码和模型已经发布。
论文及项目相关链接
PDF 12 pages, 6 figures
摘要
本文提出一种基于Mamba的端到端多模态双向对话模型DuplexMamba,用于语音到文本的对话。DuplexMamba可实现实时语音对话,同时具备流式处理能力,动态调整以支持实时流媒体传输。该模型采用基于Mamba的语音编码器和基于Mamba的语言模型,并引入了一种新型双向解码策略,可实现输入处理和输出生成的同步进行。实验结果表明,DuplexMamba成功实现了双向和流式处理能力,同时在自动语音识别(ASR)任务和语音助手基准评估中实现了与最新Transformer模型相当的性能。我们的代码和模型已发布。
要点
- DuplexMamba是一个基于Mamba的端到端多模态双向模型,用于语音到文本的对话。
- 实现实时语音对话和流式处理功能。
- 动态调整以支持实时流媒体传输。
- 采用基于Mamba的语音编码器和语言模型。
- 引入新型双向解码策略,实现输入处理和输出生成的同步。
- 在ASR任务和语音助手基准评估中表现优秀。
点此查看论文截图
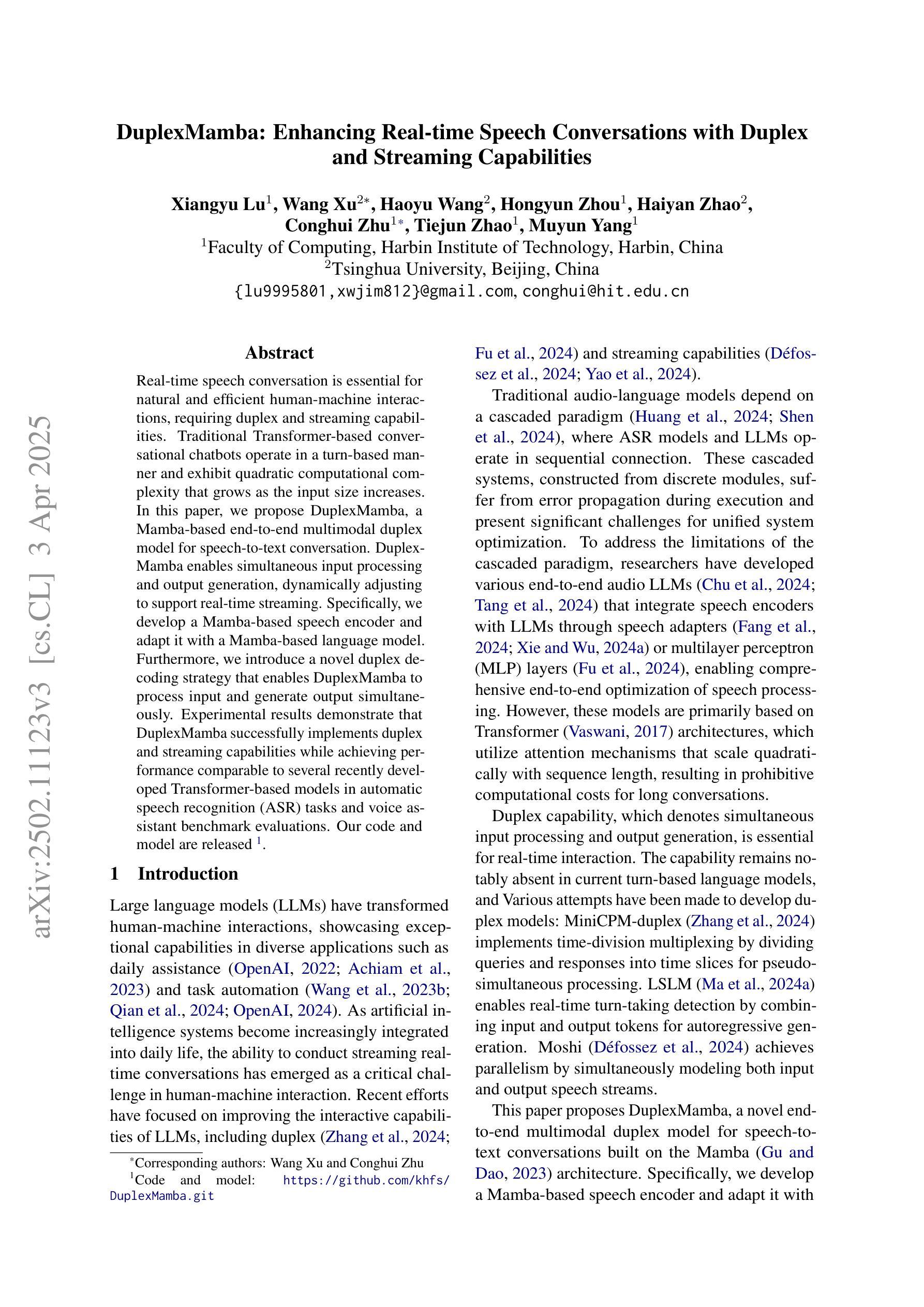
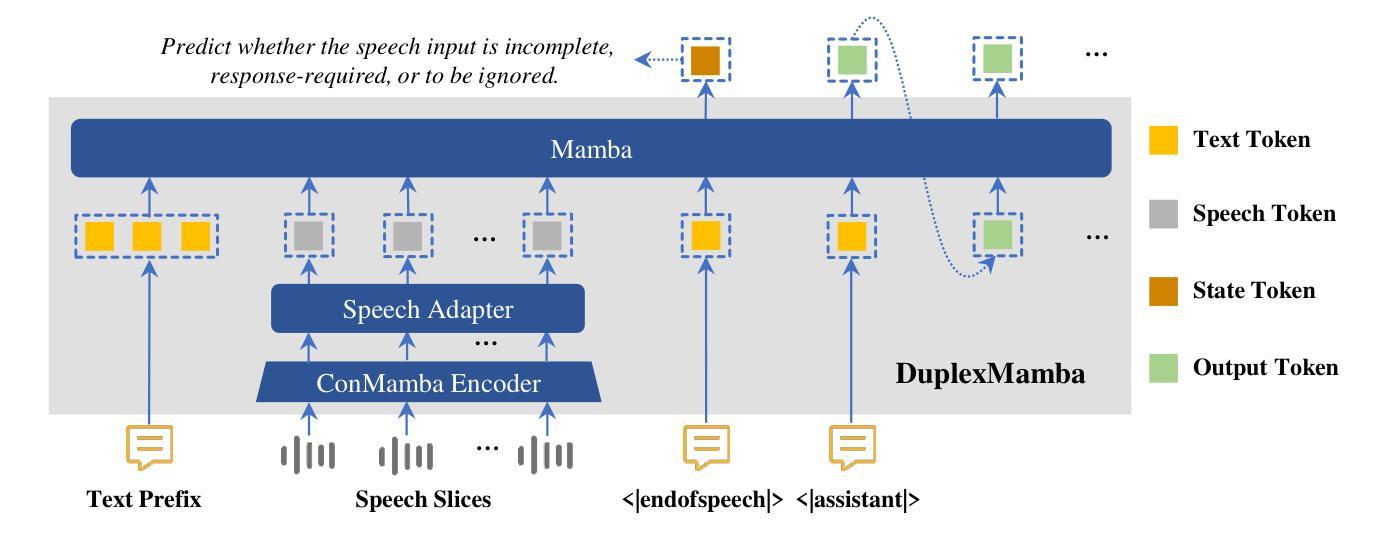
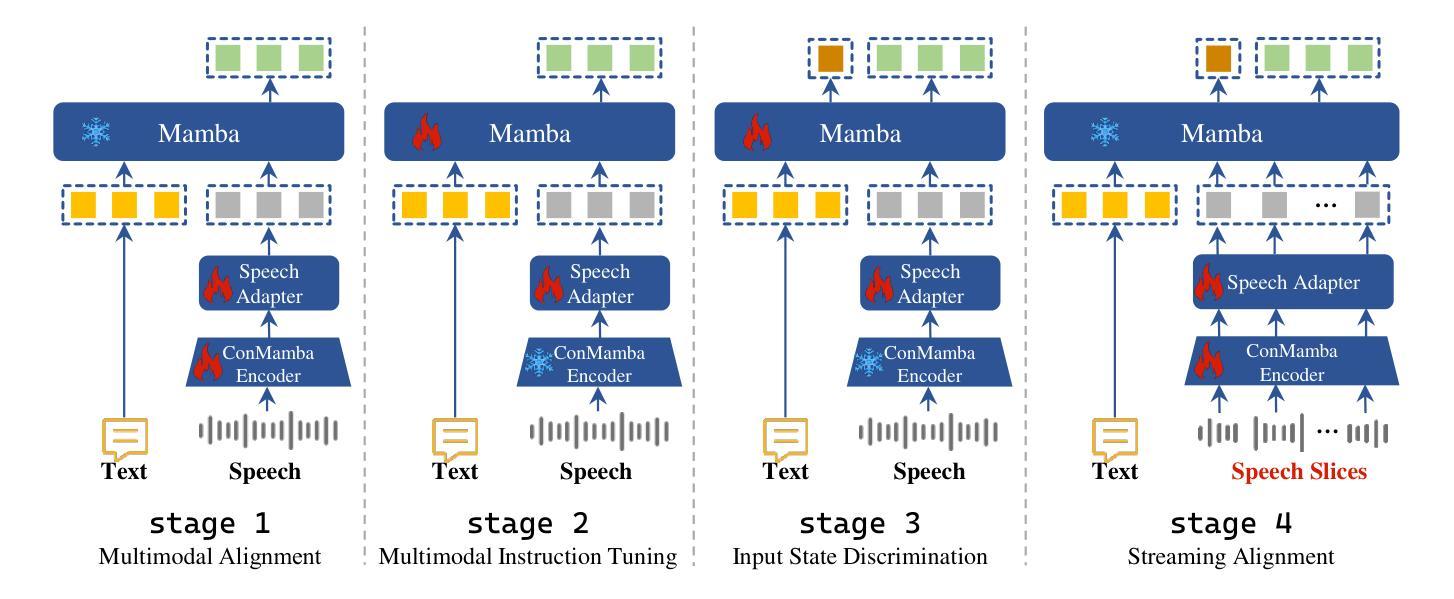
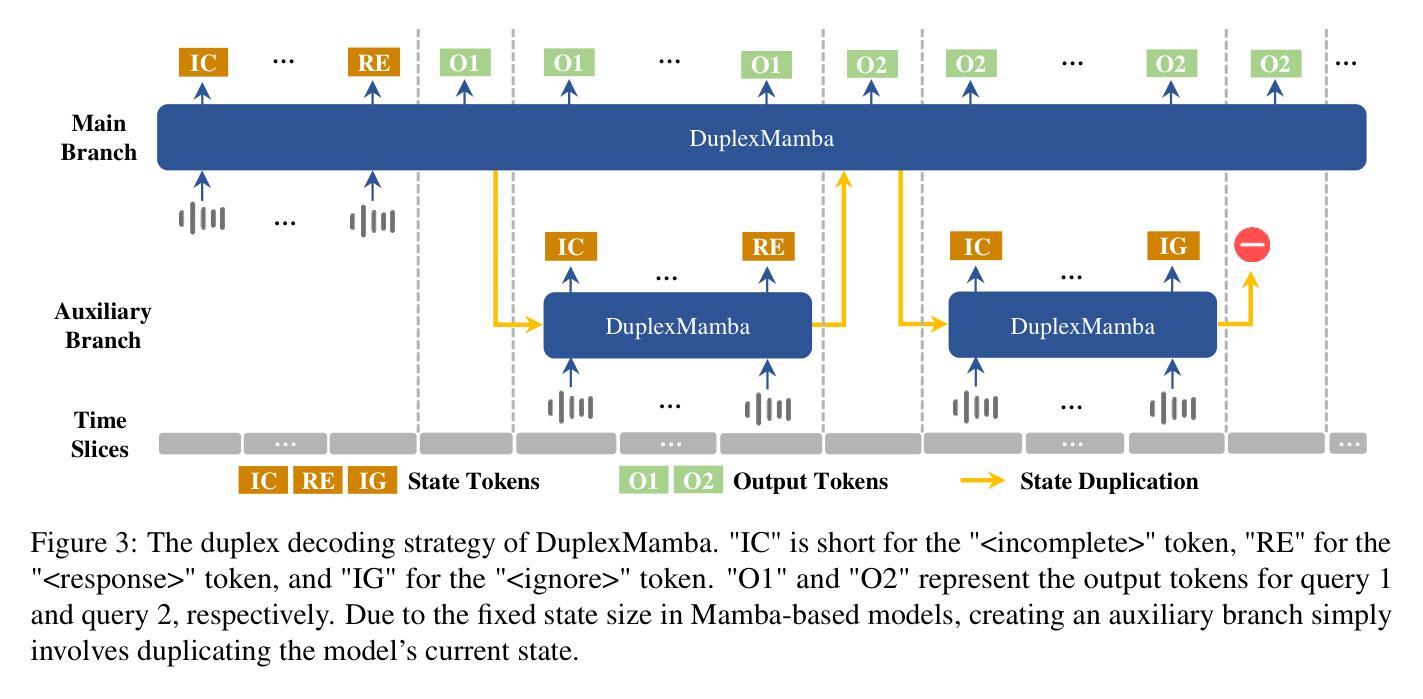
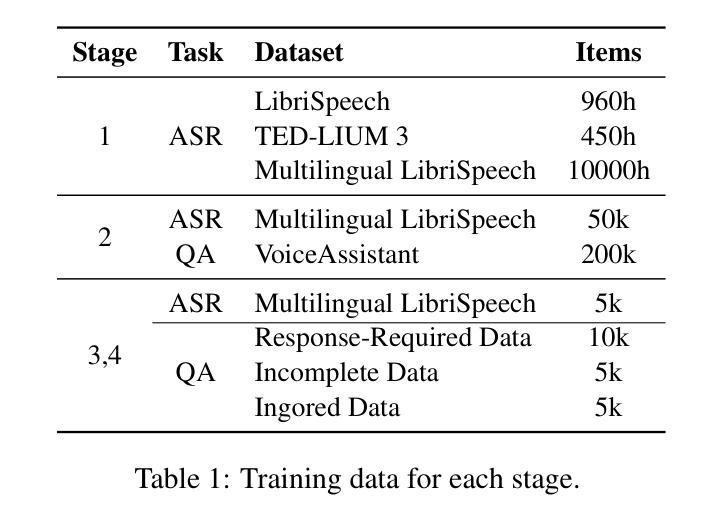
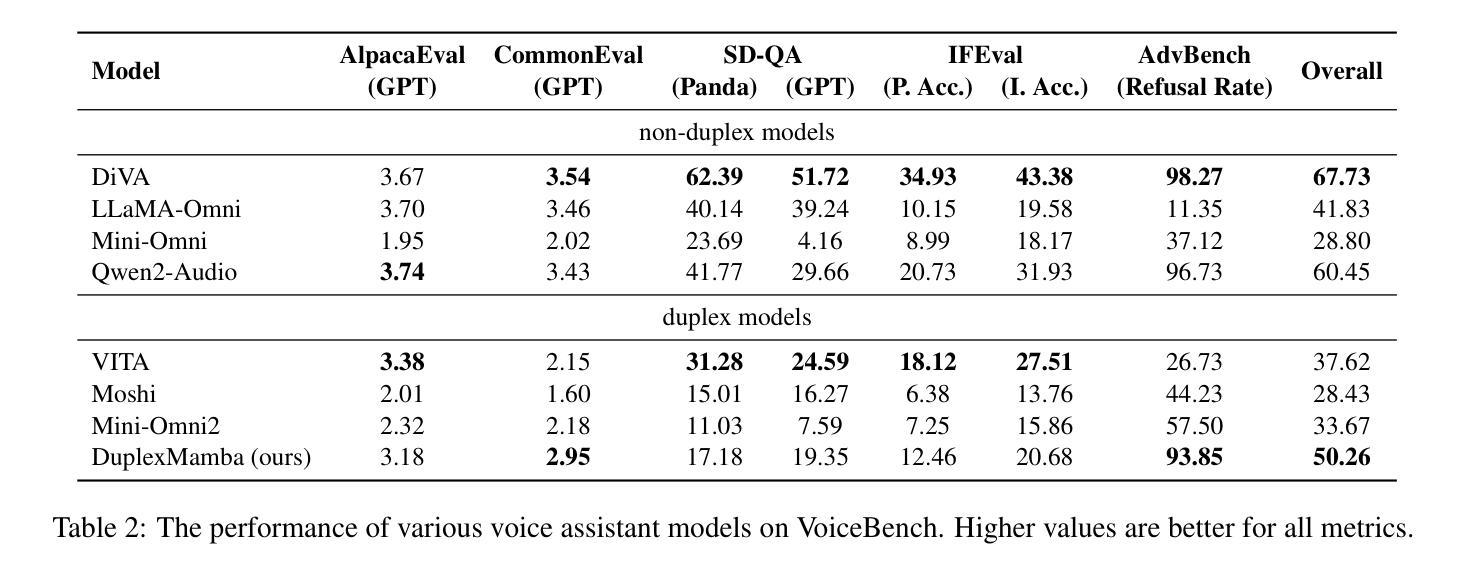
Ichigo: Mixed-Modal Early-Fusion Realtime Voice Assistant
Authors:Alan Dao, Dinh Bach Vu, Huy Hoang Ha
Large Language Models (LLMs) have revolutionized natural language processing, but their application to speech-based tasks remains challenging due to the complexities of integrating audio and text modalities. This paper introduces Ichigo, a mixed-modal model that seamlessly processes interleaved sequences of speech and text. Utilizing a tokenized early-fusion approach, Ichigo quantizes speech into discrete tokens and employs a uniform transformer-based architecture for both speech and text modalities. This method enables joint reasoning and generation across modalities without the need for separate adapters. We present a comprehensive training methodology, including pre-training on multilingual speech recognition datasets and fine-tuning on a curated instruction dataset. Ichigo demonstrates state-of-the-art performance on speech question-answering benchmarks, outperforming existing open-source speech language models and achieving comparable results to cascaded systems. Notably, Ichigo exhibits a latency of just 111 ms to first token generation, significantly lower than current models. Our approach not only advances the field of multimodal AI but also provides a framework for smaller research teams to contribute effectively to open-source speech-language models.
大型语言模型(LLM)已经彻底改变了自然语言处理的格局,但在基于语音的任务中,由于其整合音频和文本模态的复杂性,其应用仍然具有挑战性。本文介绍了一种名为Ichigo的混合模态模型,它能够无缝处理语音和文本交织的序列。Ichigo采用了一种早期融合方法,将语音量化为离散标记,并采用统一的基于转换器的架构处理语音和文本模态。这种方法能够在不需要单独适配器的情况下进行跨模态的联合推理和生成。我们提出了一种全面的训练方法,包括在多语言语音识别数据集上进行预训练和在精选指令数据集上进行微调。在语音问答基准测试中,Ichigo展现出最先进的技术性能,在现有开源语音语言模型上取得了卓越表现,并达到了级联系统的可比结果。值得注意的是,Ichigo的首个标记生成延迟仅为111毫秒,远低于当前模型。我们的方法不仅推动了多模态人工智能领域的发展,而且为小型研究团队有效地贡献开源语音语言模型提供了框架。
论文及项目相关链接
总结
大型语言模型在自然语言处理领域带来了革命性的变革,但在语音任务方面的应用仍面临挑战,主要体现在音频和文本模态的整合复杂性上。本文介绍了Ichigo这一混合模态模型,它能无缝处理语音和文本交织的序列。Ichigo采用早期融合方法,将语音量化为离散令牌,并采用统一的基于转换器的架构处理语音和文本模态。这种方法无需单独适配器即可实现跨模态的联合推理和生成。我们提出了一种全面的训练方法,包括在多语言语音识别数据集上进行预训练和在精选指令数据集上进行微调。Ichigo在语音问答基准测试中表现出卓越的性能,不仅优于现有开源语音语言模型,而且达到了级联系统的水平。值得注意的是,Ichigo的首个令牌生成延迟时间仅为111毫秒,显著低于当前模型。我们的方法不仅推动了多模态人工智能领域的发展,而且为小型研究团队有效地参与开源语音语言模型提供了框架。
关键见解
- Ichigo是一个混合模态模型,能够无缝处理语音和文本的交织序列。
- 采用早期融合方法和离散令牌化技术处理语音数据。
- 使用统一的基于转换器的架构整合语音和文本模态。
- 全面的训练策略包括预训练和微调阶段。
- Ichigo在语音问答方面表现出卓越性能,与级联系统相比具有竞争力。
- Ichigo的首个令牌生成延迟时间较低,为111毫秒。
点此查看论文截图
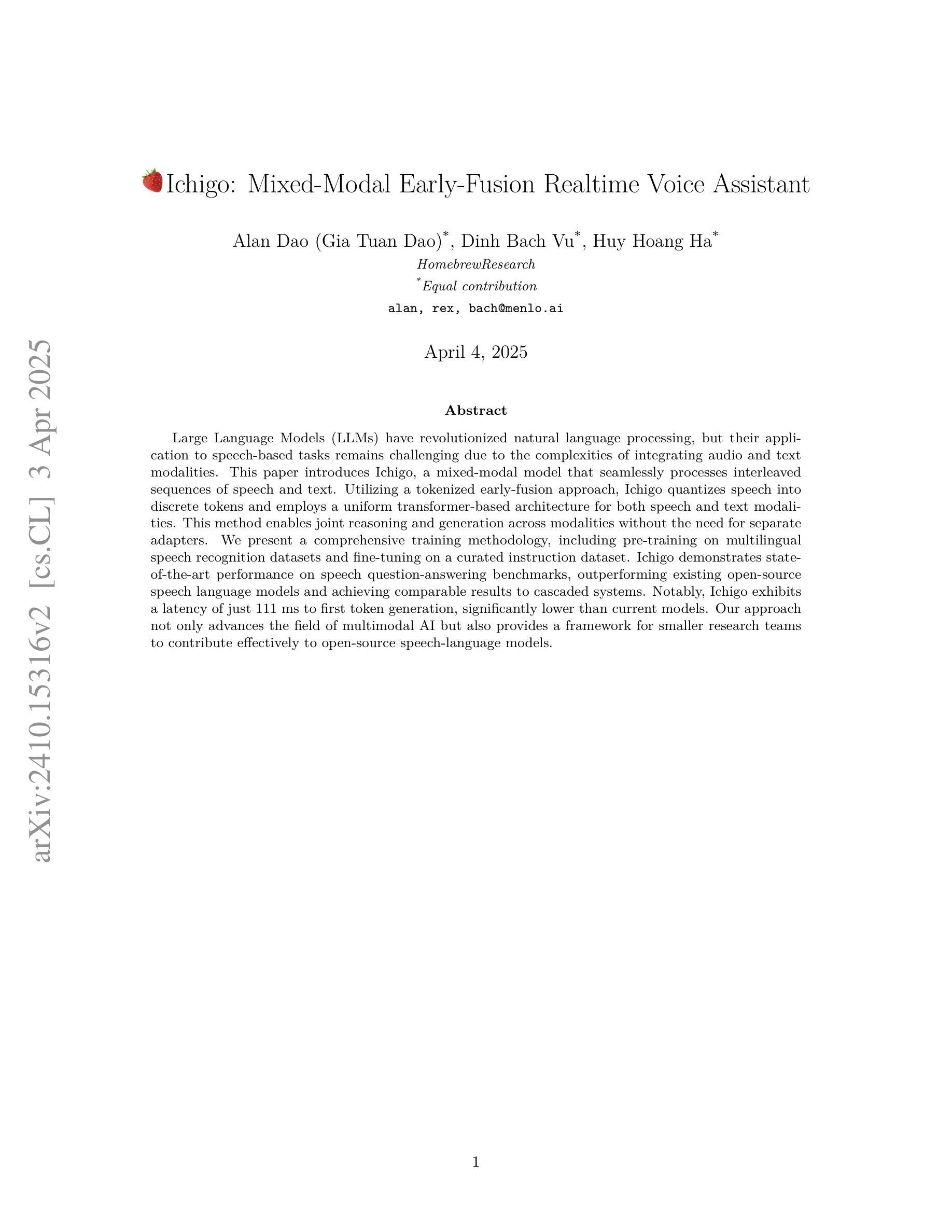
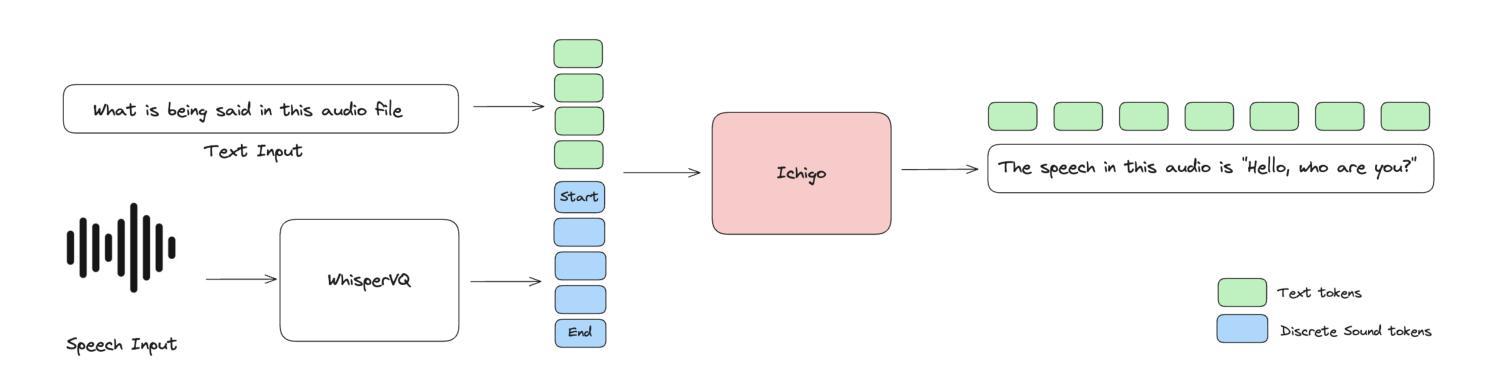
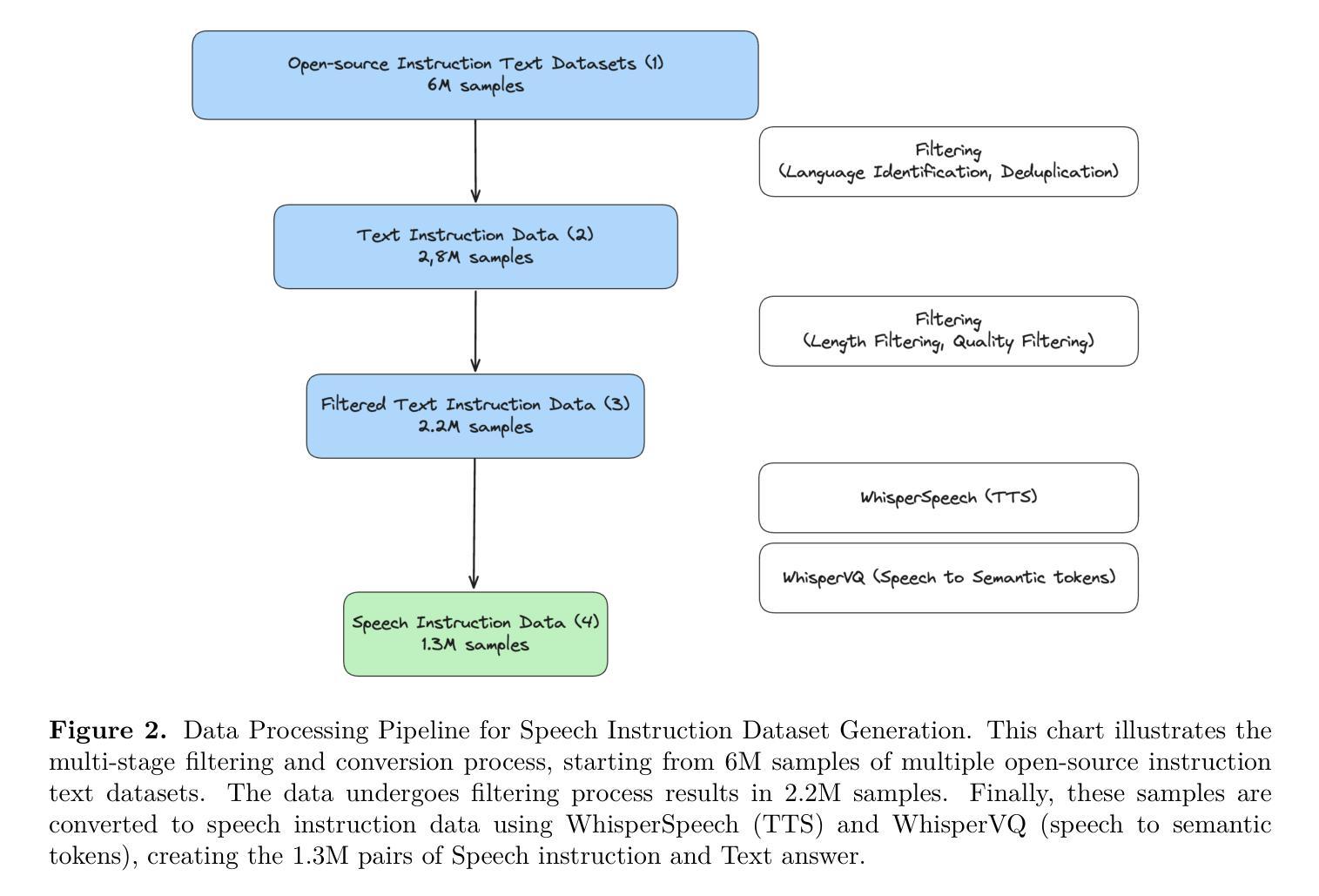