⚠️ 以下所有内容总结都来自于 大语言模型的能力,如有错误,仅供参考,谨慎使用
🔴 请注意:千万不要用于严肃的学术场景,只能用于论文阅读前的初筛!
💗 如果您觉得我们的项目对您有帮助 ChatPaperFree ,还请您给我们一些鼓励!⭐️ HuggingFace免费体验
2025-04-05 更新
OmniTalker: Real-Time Text-Driven Talking Head Generation with In-Context Audio-Visual Style Replication
Authors:Zhongjian Wang, Peng Zhang, Jinwei Qi, Guangyuan Wang Sheng Xu, Bang Zhang, Liefeng Bo
Recent years have witnessed remarkable advances in talking head generation, owing to its potential to revolutionize the human-AI interaction from text interfaces into realistic video chats. However, research on text-driven talking heads remains underexplored, with existing methods predominantly adopting a cascaded pipeline that combines TTS systems with audio-driven talking head models. This conventional pipeline not only introduces system complexity and latency overhead but also fundamentally suffers from asynchronous audiovisual output and stylistic discrepancies between generated speech and visual expressions. To address these limitations, we introduce OmniTalker, an end-to-end unified framework that simultaneously generates synchronized speech and talking head videos from text and reference video in real-time zero-shot scenarios, while preserving both speech style and facial styles. The framework employs a dual-branch diffusion transformer architecture: the audio branch synthesizes mel-spectrograms from text, while the visual branch predicts fine-grained head poses and facial dynamics. To bridge modalities, we introduce a novel audio-visual fusion module that integrates cross-modal information to ensure temporal synchronization and stylistic coherence between audio and visual outputs. Furthermore, our in-context reference learning module effectively captures both speech and facial style characteristics from a single reference video without introducing an extra style extracting module. To the best of our knowledge, OmniTalker presents the first unified framework that jointly models speech style and facial style in a zero-shot setting, achieving real-time inference speed of 25 FPS. Extensive experiments demonstrate that our method surpasses existing approaches in generation quality, particularly excelling in style preservation and audio-video synchronization.
近年来,基于文本生成对话视频的技术取得了显著进展,这归功于其对人类和人工智能之间的交互所带来的革命性影响,使之从文本界面变为现实对话视频成为可能。然而,基于文本驱动的对谈式头像的研究仍处于未完全开发阶段。现有方法主要采用了结合文本语音转换系统和对声音驱动的对话头部模型的级联管道技术。这种传统管道技术不仅增加了系统的复杂性和延迟开销,还面临着视听输出不同步和生成语音与面部表情之间风格差异等根本性问题。为了克服这些局限性,我们推出了OmniTalker,这是一个端到端的统一框架,能够从文本和参考视频中实时生成同步语音和对话视频内容。该框架在零样本场景中同时生成同步语音和对话视频内容,同时保留语音和面部风格。该框架采用双分支扩散转换器架构:音频分支从文本中合成梅尔频谱图,而视觉分支则预测精细的头部姿势和面部动态。为了弥合不同感官之间的鸿沟,我们引入了一种新颖的视听融合模块,该模块可以集成跨模态信息以确保音频和视频输出之间的时间同步和风格一致性。此外,我们的上下文参考学习模块有效地从单个参考视频中捕获语音和面部风格特征,无需引入额外的风格提取模块。据我们所知,OmniTalker是一个首次联合在零样本环境中共同构建语音风格和面部风格的统一框架,其推理速度达到每秒25帧。大量实验表明,我们的方法在生成质量上超过了现有方法,尤其在风格保持和音视频同步方面表现出色。
论文及项目相关链接
PDF Project Page https://humanaigc.github.io/omnitalker
Summary
本文介绍了OmniTalker框架,该框架能在零样本场景中实时生成同步的语音和谈话头视频。OmniTalker采用端到端的统一架构,能同时从文本和参考视频中生成内容,保持语音风格和面部风格的一致性。该框架采用双分支扩散转换器结构,包括音频分支用于从文本合成梅尔频谱图,视觉分支用于预测精细头部姿态和面部动态。引入了一种新颖的音频视觉融合模块,确保音频和视觉输出之间的时序同步和风格一致性。此外,OmniTalker的上下文参考学习模块能有效捕捉参考视频中的语音和面部风格特征,无需额外的风格提取模块。OmniTalker是首个在零样本设置中联合建模语音风格和面部风格的框架,实现了实时推理速度25帧每秒,并且在生成质量上超越了现有方法,尤其在风格保持和音视频同步方面表现突出。
Key Takeaways
- OmniTalker是一个实时生成同步语音和谈话头视频的端到端统一框架。
- OmniTalker能从文本和参考视频中生成内容,并同时保持语音风格和面部风格的一致性。
- 该框架采用双分支扩散转换器结构,包括音频分支和视觉分支,分别负责合成梅尔频谱图和预测头部姿态及面部动态。
- 引入了音频视觉融合模块,确保音频和视觉输出的同步和风格一致性。
- OmniTalker的上下文参考学习模块能捕捉参考视频中的语音和面部风格特征,无需额外的风格提取步骤。
- OmniTalker实现了实时推理速度25帧每秒,并在生成质量上超越了现有方法。
点此查看论文截图
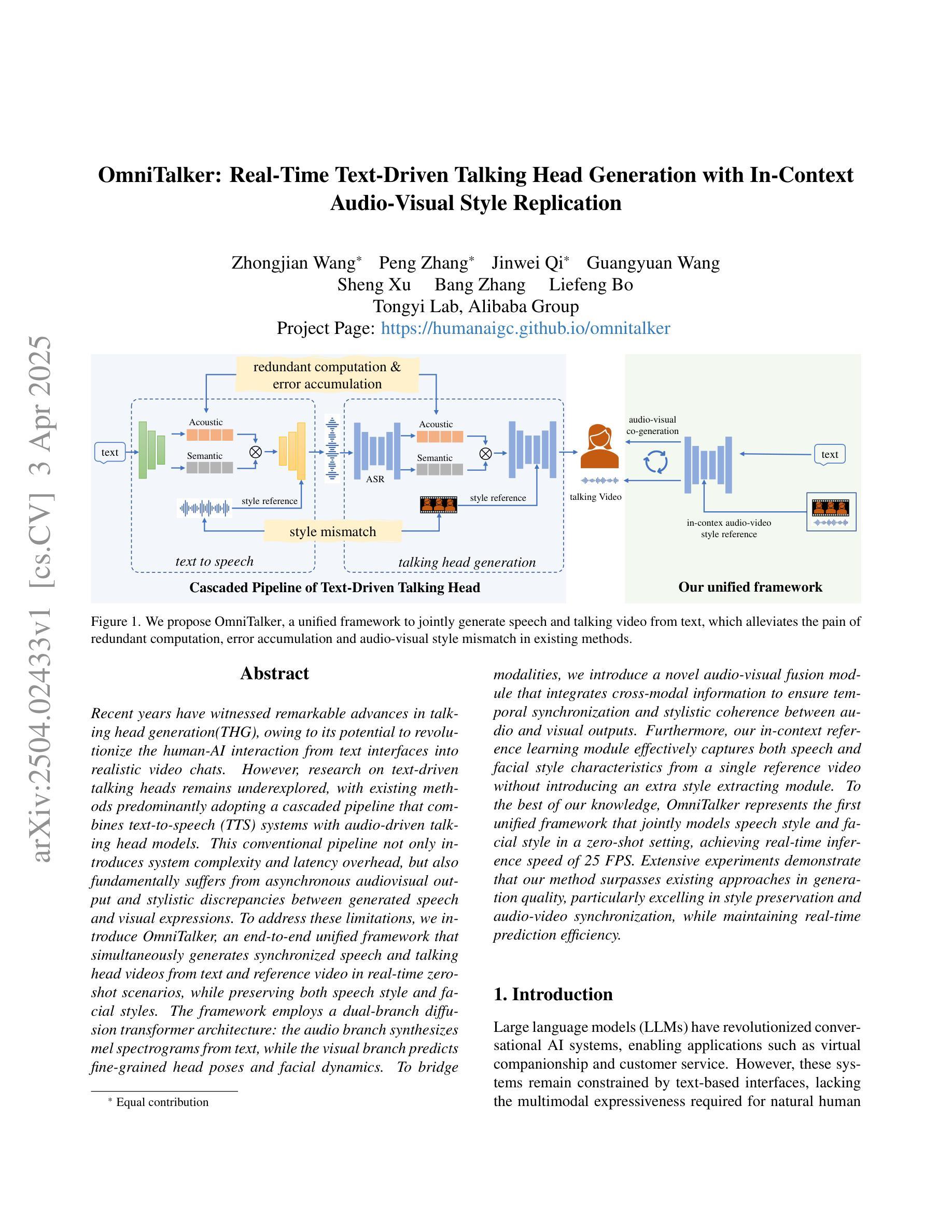
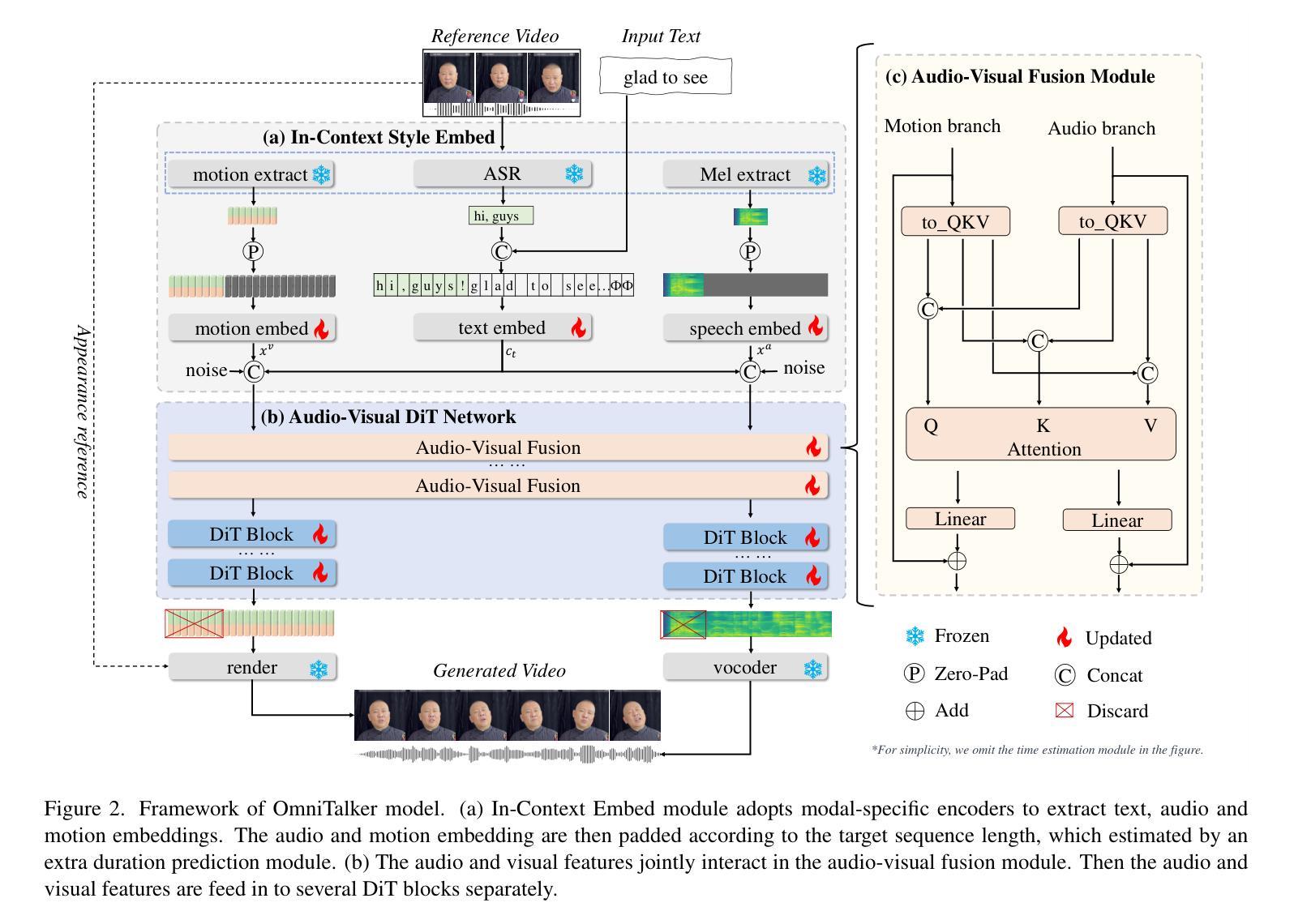
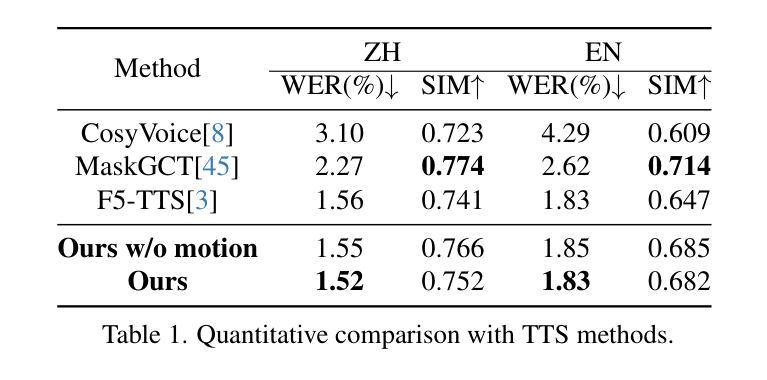
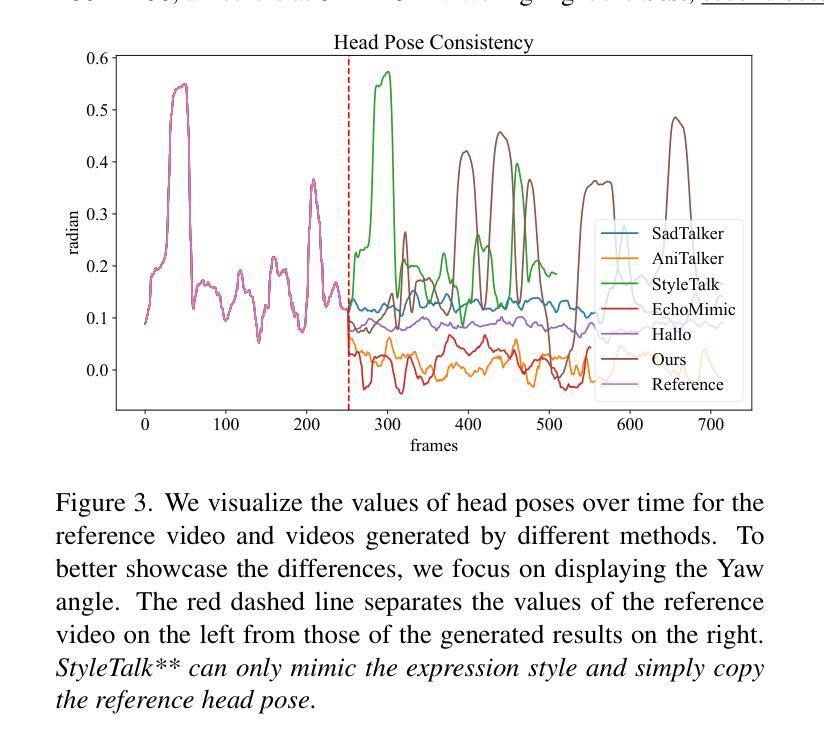
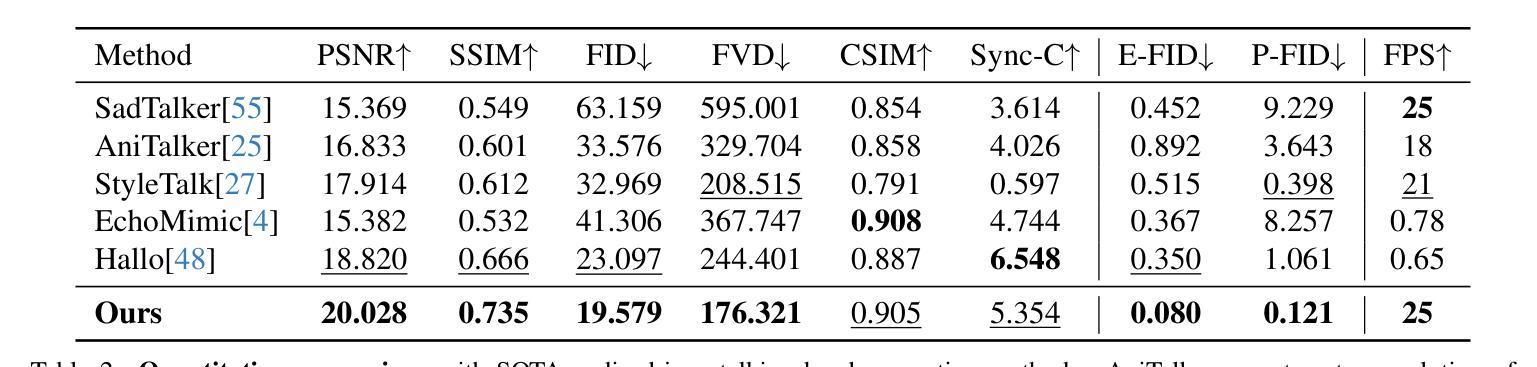