⚠️ 以下所有内容总结都来自于 大语言模型的能力,如有错误,仅供参考,谨慎使用
🔴 请注意:千万不要用于严肃的学术场景,只能用于论文阅读前的初筛!
💗 如果您觉得我们的项目对您有帮助 ChatPaperFree ,还请您给我们一些鼓励!⭐️ HuggingFace免费体验
2025-04-08 更新
AdaCM$^2$: On Understanding Extremely Long-Term Video with Adaptive Cross-Modality Memory Reduction
Authors:Yuanbin Man, Ying Huang, Chengming Zhang, Bingzhe Li, Wei Niu, Miao Yin
The advancements in large language models (LLMs) have propelled the improvement of video understanding tasks by incorporating LLMs with visual models. However, most existing LLM-based models (e.g., VideoLLaMA, VideoChat) are constrained to processing short-duration videos. Recent attempts to understand long-term videos by extracting and compressing visual features into a fixed memory size. Nevertheless, those methods leverage only visual modality to merge video tokens and overlook the correlation between visual and textual queries, leading to difficulties in effectively handling complex question-answering tasks. To address the challenges of long videos and complex prompts, we propose AdaCM$^2$, which, for the first time, introduces an adaptive cross-modality memory reduction approach to video-text alignment in an auto-regressive manner on video streams. Our extensive experiments on various video understanding tasks, such as video captioning, video question answering, and video classification, demonstrate that AdaCM$^2$ achieves state-of-the-art performance across multiple datasets while significantly reducing memory usage. Notably, it achieves a 4.5% improvement across multiple tasks in the LVU dataset with a GPU memory consumption reduction of up to 65%.
随着大型语言模型(LLM)的进步,通过将LLM与视觉模型相结合,推动了视频理解任务的改进。然而,大多数现有的基于LLM的模型(例如VideoLLaMA、VideoChat)仅限于处理短时视频。最近有人尝试通过提取和压缩视觉特征到固定内存大小来理解长视频。然而,这些方法只利用视觉模式来合并视频令牌,忽略了视觉和文本查询之间的相关性,导致难以有效地处理复杂的问答任务。为了解决长视频和复杂提示的挑战,我们提出了AdaCM$^2$,它首次引入了一种自适应跨模态内存缩减方法,以自适应回归的方式对齐视频流中的视频文本。我们在各种视频理解任务上进行了广泛实验,如视频描述、视频问答和视频分类,结果表明,AdaCM$^2$在多个数据集上实现了最新技术性能,同时大大降低了内存使用。值得注意的是,它在LVU数据集的多项任务上实现了4.5%的改进,GPU内存消耗减少了高达65%。
论文及项目相关链接
PDF CVPR 2025 Highlight
Summary:随着大型语言模型(LLM)的进步,视频理解任务得到了提升。通过将LLM与视觉模型结合,能够处理短视频。对于长视频,现有方法仅依赖视觉模态合并视频令牌,忽略了视觉与文本查询之间的相关性,难以处理复杂的问答任务。我们提出AdaCM$^2$模型,首次采用自适应跨模态内存缩减方法,以自适应方式实现视频流中的视频文本对齐。在多个视频理解任务上,AdaCM$^2$表现出卓越性能,并在LVU数据集上实现了高达4.5%的改进,同时GPU内存消耗减少了高达65%。
Key Takeaways:
- 大型语言模型的进步推动了视频理解任务的改进。
- 目前模型在处理短视频方面表现较好,但对长视频的理解存在挑战。
- 现有方法主要依赖视觉模态处理长视频,忽略了视觉与文本查询的相关性。
- AdaCM$^2$模型首次采用自适应跨模态内存缩减方法,实现视频流中的视频文本自适应对齐。
- AdaCM$^2$模型在多个视频理解任务上表现出卓越性能。
- 在LVU数据集上,AdaCM$^2$实现了高达4.5%的性能改进。
点此查看论文截图
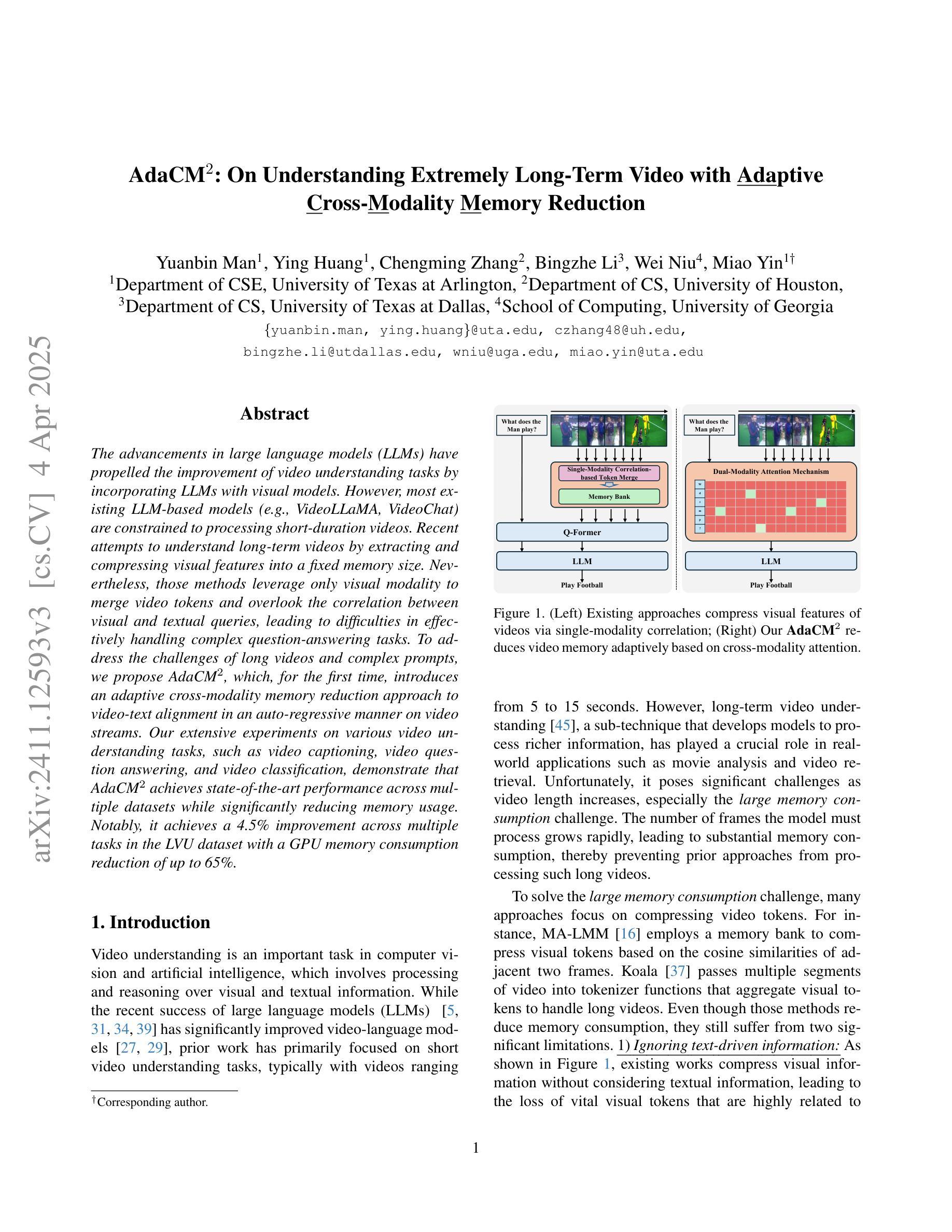
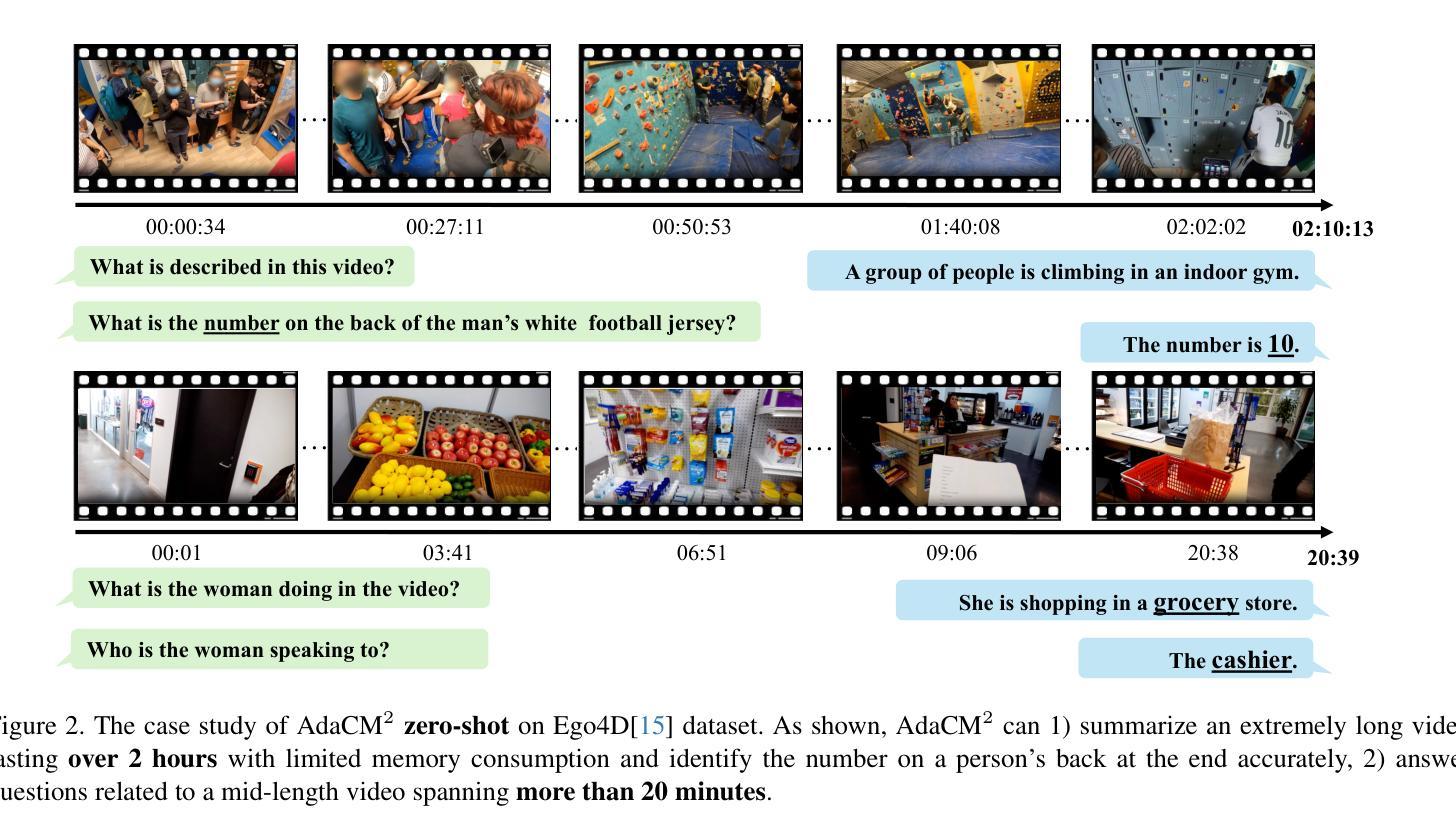
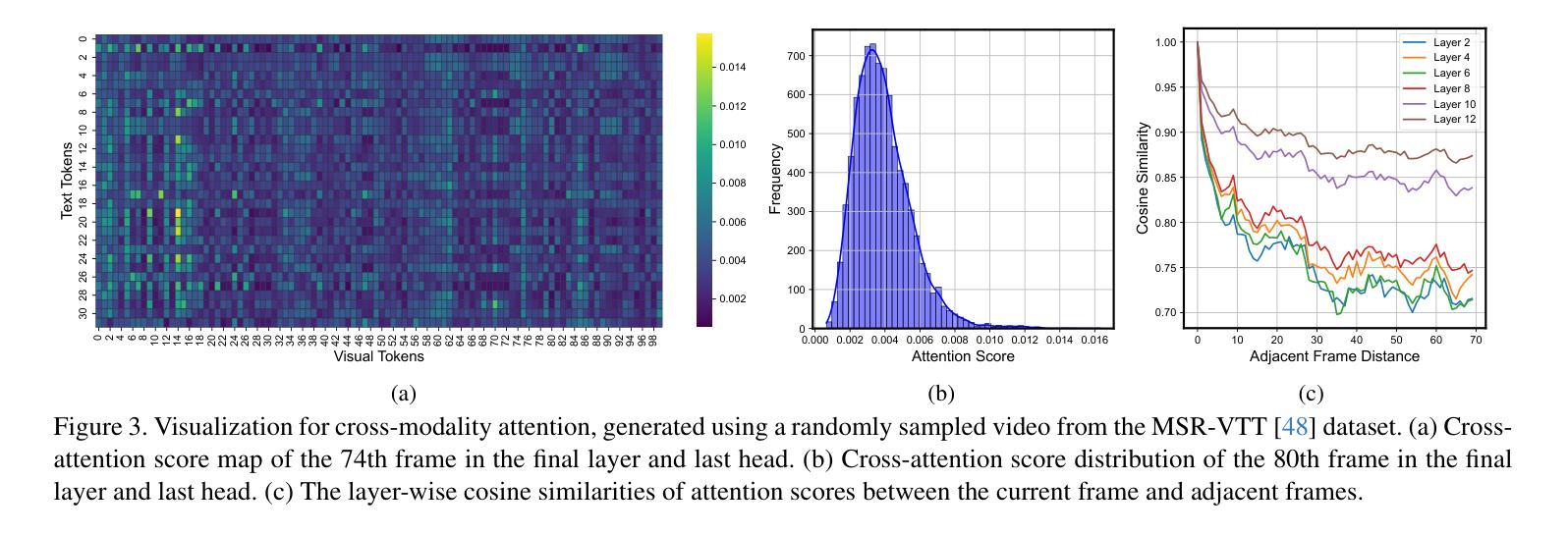
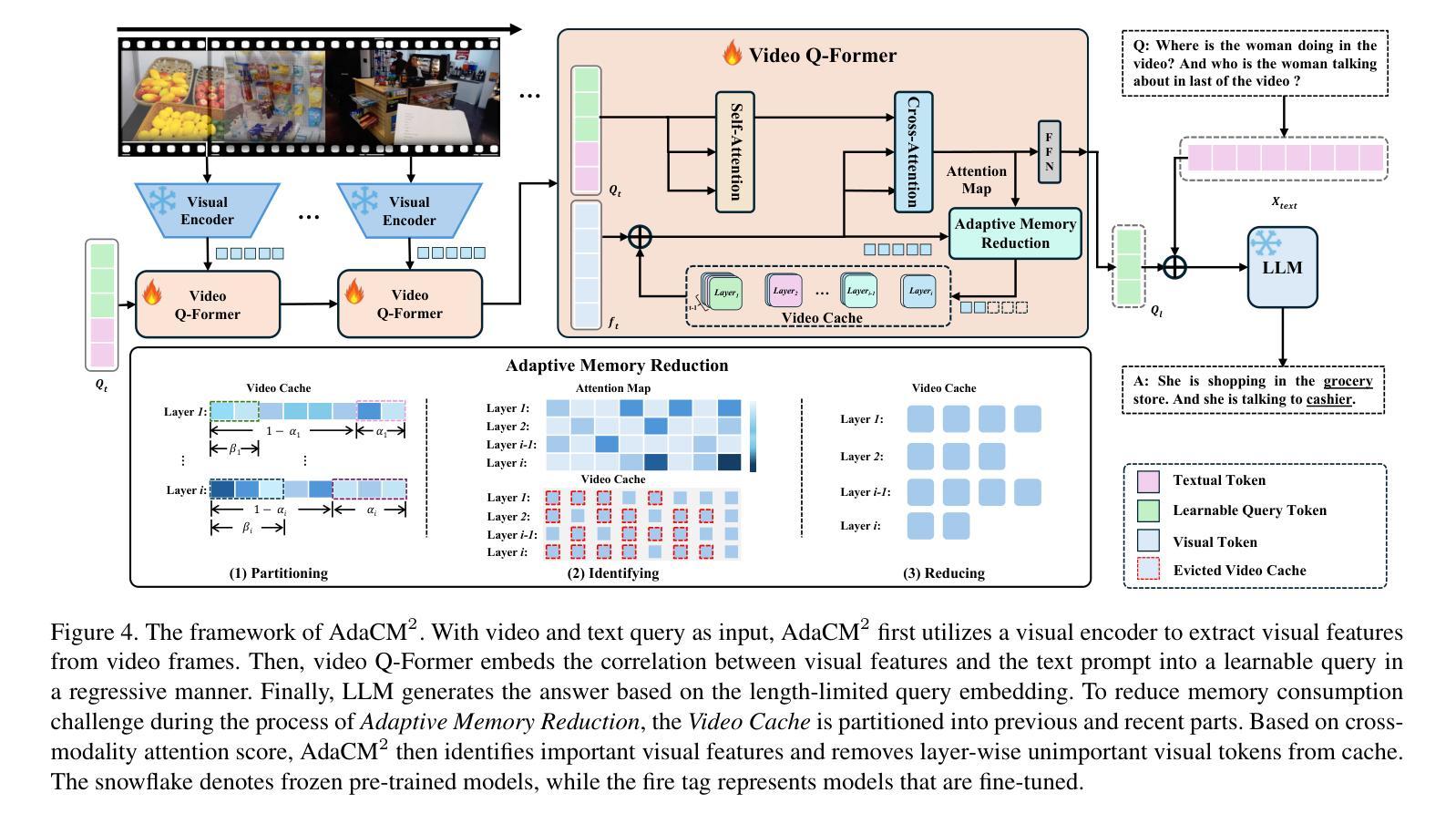
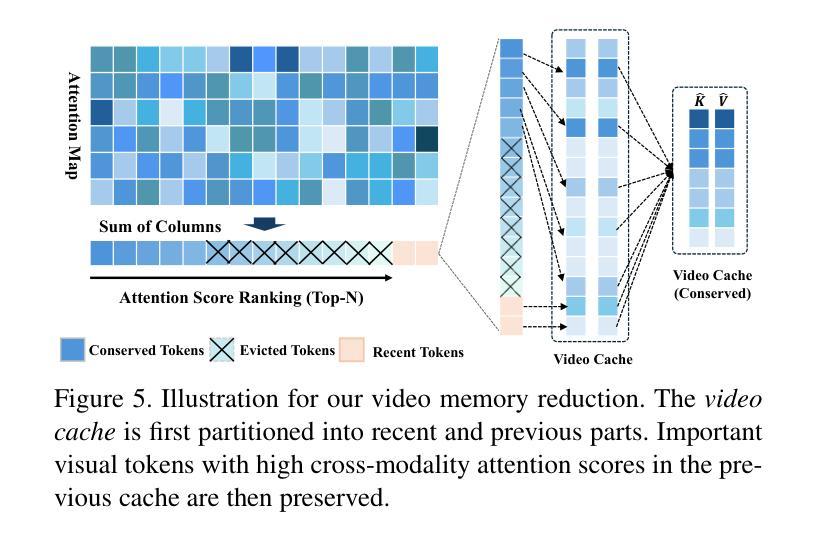
Motion-Grounded Video Reasoning: Understanding and Perceiving Motion at Pixel Level
Authors:Andong Deng, Tongjia Chen, Shoubin Yu, Taojiannan Yang, Lincoln Spencer, Yapeng Tian, Ajmal Saeed Mian, Mohit Bansal, Chen Chen
In this paper, we introduce Motion-Grounded Video Reasoning, a new motion understanding task that requires generating visual answers (video segmentation masks) according to the input question, and hence needs implicit spatiotemporal reasoning and grounding. This task extends existing spatiotemporal grounding work focusing on explicit action/motion grounding, to a more general format by enabling implicit reasoning via questions. To facilitate the development of the new task, we collect a large-scale dataset called GROUNDMORE, which comprises 1,715 video clips, 249K object masks that are deliberately designed with 4 question types (Causal, Sequential, Counterfactual, and Descriptive) for benchmarking deep and comprehensive motion reasoning abilities. GROUNDMORE uniquely requires models to generate visual answers, providing a more concrete and visually interpretable response than plain texts. It evaluates models on both spatiotemporal grounding and reasoning, fostering to address complex challenges in motion-related video reasoning, temporal perception, and pixel-level understanding. Furthermore, we introduce a novel baseline model named Motion-Grounded Video Reasoning Assistant (MORA). MORA incorporates the multimodal reasoning ability from the Multimodal LLM, the pixel-level perception capability from the grounding model (SAM), and the temporal perception ability from a lightweight localization head. MORA achieves respectable performance on GROUNDMORE outperforming the best existing visual grounding baseline model by an average of 21.5% relatively. We hope this novel and challenging task will pave the way for future advancements in robust and general motion understanding via video reasoning segmentation
本文介绍了基于动作的视频推理(Motion-Grounded Video Reasoning),这是一个新的动作理解任务。它需要根据输入的问题生成视觉答案(视频分割掩码),因此需要进行隐性的时空推理和定位。此任务扩展了现有的时空定位工作,侧重于通过问题实现隐性推理,以一种更通用的格式呈现。为了促进新任务的发展,我们收集了一个大规模数据集,称为GROUNDMORE。该数据集包含1715个视频剪辑和24.9万个对象掩码,这些掩码经过精心设计,包含四种类型的问题(因果、顺序、反事实和描述性),用于基准测试深度和全面的运动推理能力。GROUNDMORE要求模型生成视觉答案,提供比纯文本更具体和视觉可解释的响应。它评估了模型的时空定位和推理能力,有助于解决运动相关视频推理、时间感知和像素级理解的复杂挑战。此外,我们介绍了一种新的基线模型,名为Motion-Grounded Video Reasoning Assistant(MORA)。MORA结合了多模态LLM的多模态推理能力、定位模型(SAM)的像素级感知能力以及轻量化定位头的时间感知能力。MORA在GROUNDMORE上表现出令人尊重的性能,相对于现有的最佳视觉定位基线模型,平均提高了21.5%的性能。我们希望这一新颖而具有挑战性的任务将为未来通过视频推理分割进行稳健和通用的运动理解铺平道路。
论文及项目相关链接
PDF CVPR 2025
Summary
本文提出了一项新的运动理解任务——基于动作的视频推理(Motion-Grounded Video Reasoning),要求根据输入问题生成视觉答案(视频分割掩膜),需要进行隐式时空推理和定位。为支持此新任务的发展,收集了一个大规模数据集GROUNDMORE,包含1715个视频片段和24.9万个对象掩膜,设计为四种问题类型(因果、时序、反事实和描述),以评估深度和综合运动推理能力。此外,还介绍了一种新型基线模型MORA,结合多模态推理能力、像素级感知能力和时间感知能力,在GROUNDMORE上表现优异,相对现有最佳视觉定位基线模型平均提高了21.5%的性能。期望此新任务能为未来通过视频推理分割进行稳健和通用运动理解的研究铺平道路。
Key Takeaways
- 引入了新的运动理解任务——基于动作的视频推理(Motion-Grounded Video Reasoning)。
- 需要根据输入问题生成视觉答案(视频分割掩膜),要求隐式时空推理和定位能力。
- 建立了大规模数据集GROUNDMORE,包含多样化视频片段和对象掩膜,用于评估深度和综合运动推理能力。
- 数据集包含四种问题类型,旨在测试多种运动推理技能。
- 介绍了新型基线模型MORA,结合了多模态推理、像素级感知和时间感知能力。
- MORA在GROUNDMORE数据集上的性能表现优于现有模型。
点此查看论文截图
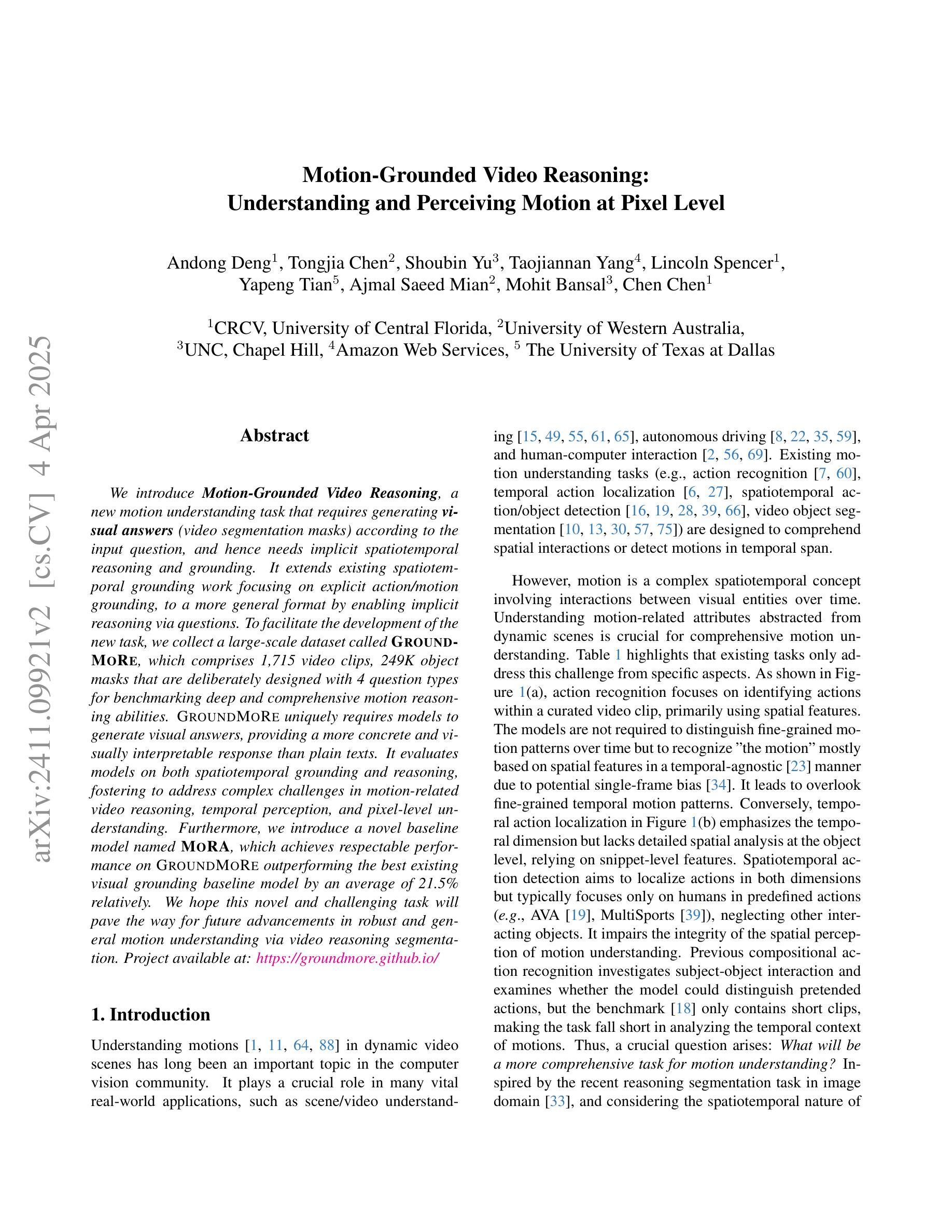
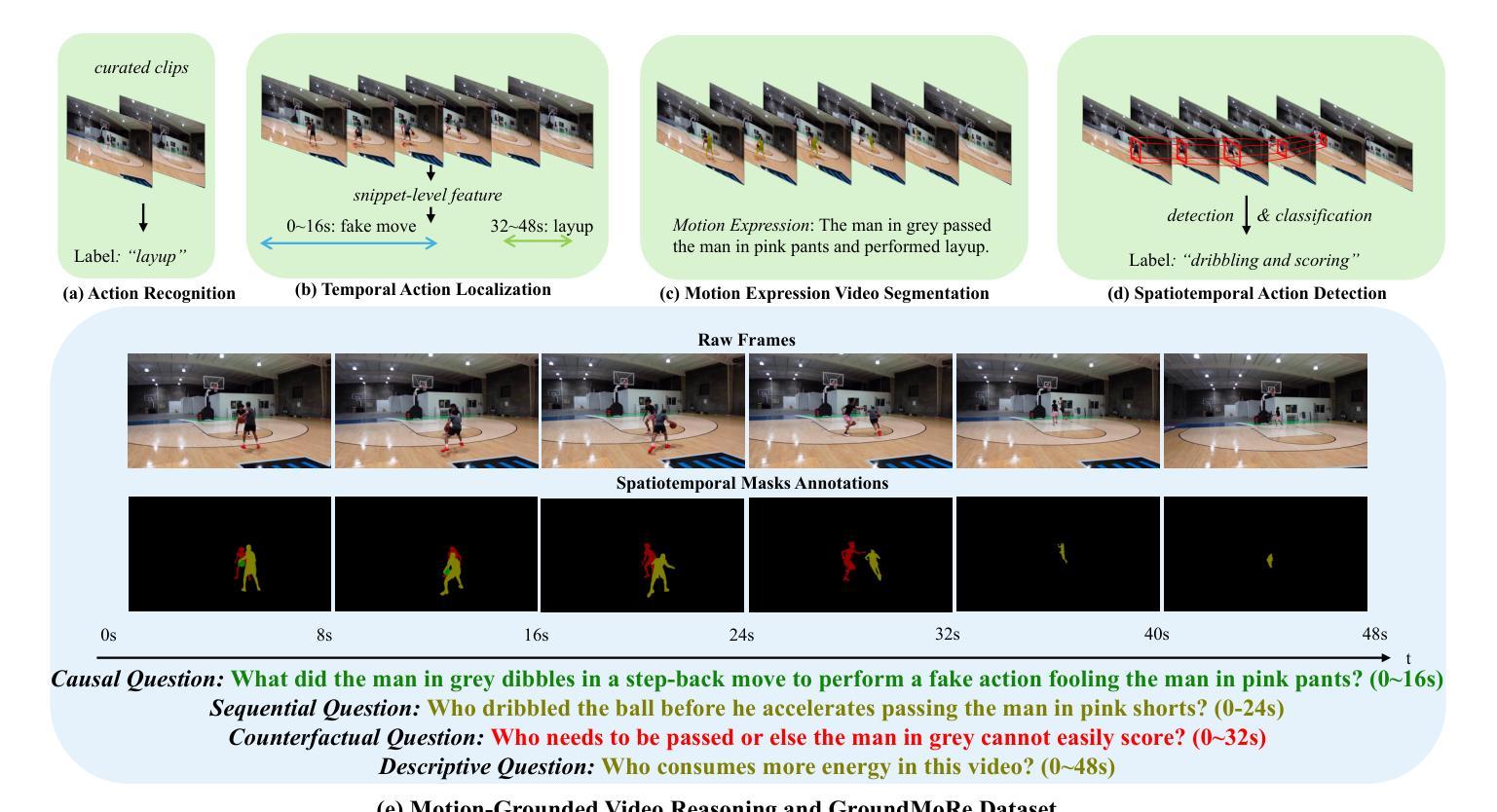
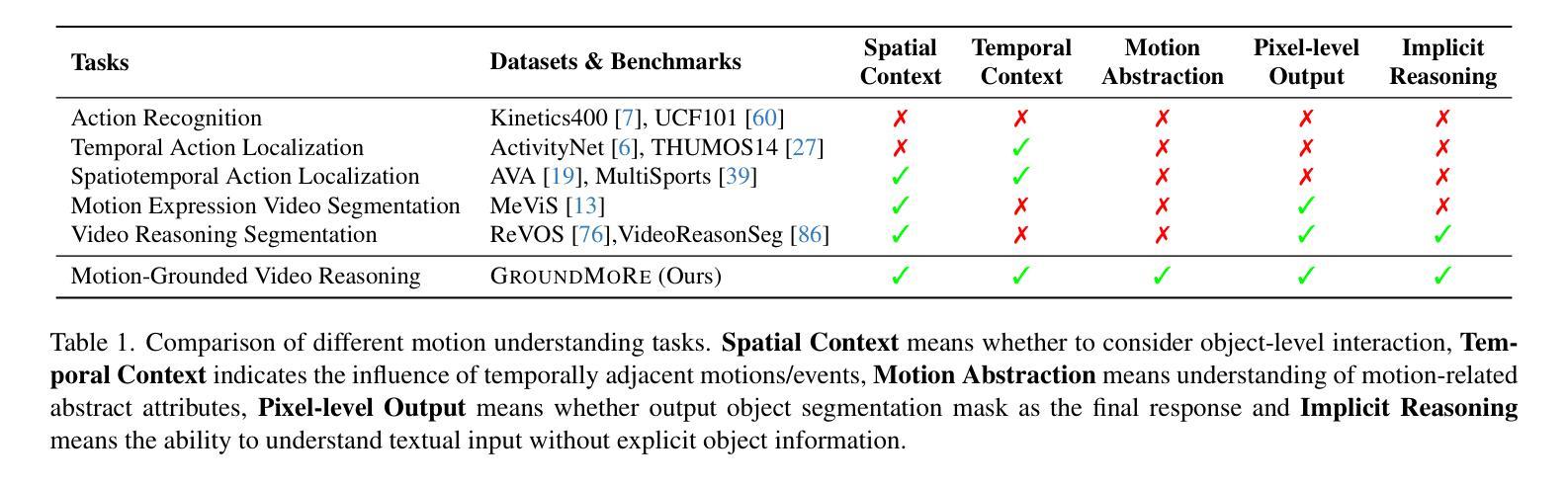
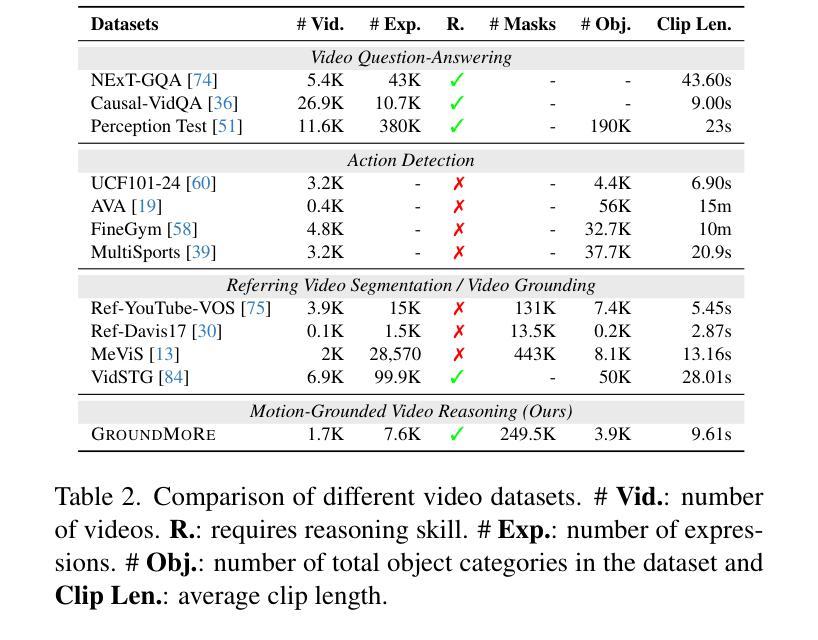
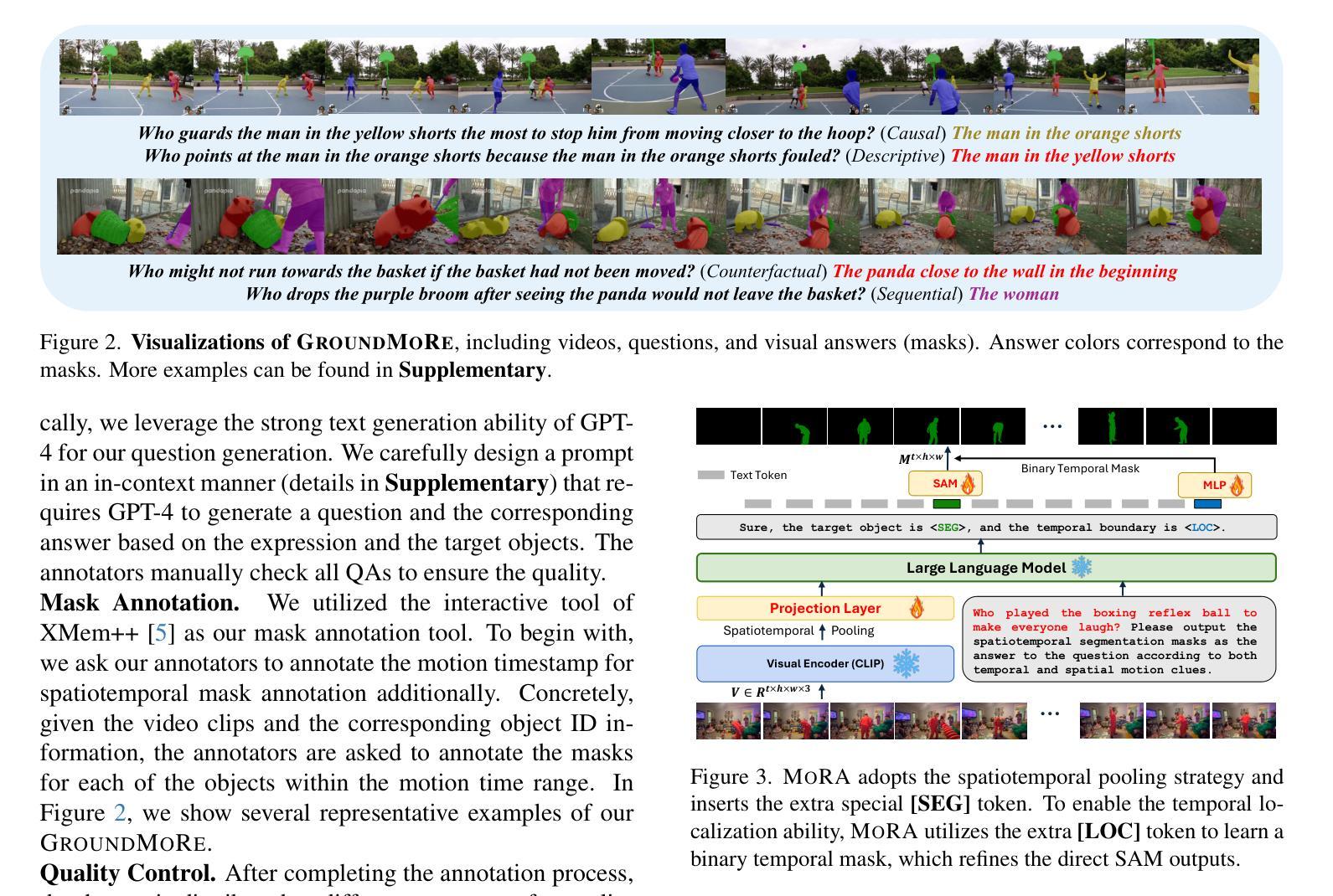
Spacewalk-18: A Benchmark for Multimodal and Long-form Procedural Video Understanding in Novel Domains
Authors:Zitian Tang, Rohan Myer Krishnan, Zhiqiu Yu, Chen Sun
Learning from (procedural) videos has increasingly served as a pathway for embodied agents to acquire skills from human demonstrations. To do this, video understanding models must be able to obtain structured understandings, such as the temporal segmentation of a demonstration into sequences of actions and skills, and to generalize the understandings to novel environments, tasks, and problem domains. In pursuit of this goal, we introduce Spacewalk-18, a benchmark containing two tasks: (1) step recognition and (2) video question answering, over a dataset of temporally segmented and labeled tasks in International Space Station spacewalk recordings. In tandem, the two tasks quantify a model’s ability to: (1) generalize to novel domains; (2) utilize long temporal context and multimodal (e.g. visual and speech) information. Our extensive experimental analysis highlights the challenges of Spacewalk-18, but also suggests best practices for domain generalization and long-form understanding. Notably, we discover a promising adaptation via summarization technique that leads to significant performance improvement without model fine-tuning. The Spacewalk-18 benchmark is released at https://brown-palm.github.io/Spacewalk-18/.
从(程序性)视频中学习已逐渐成为实体代理从人类演示中获取知识的重要途径。为此,视频理解模型必须能够获得结构化理解,例如将演示内容按时间分割成动作和技能的序列,并将这些理解推广到新的环境、任务和领域。为实现这一目标,我们推出了Spacewalk-18基准测试,包含两个任务:(1)步骤识别;(2)视频问答,数据集为国际空间站太空行走记录中按时间分割并标记的任务。这两个任务同时衡量模型的能力:(1)推广到新的领域;(2)利用长时间的上下文和多模式(例如视觉和语音)信息。我们广泛的实验分析突出了Spacewalk-18的挑战,同时也提出了针对领域推广和长形式理解的最佳实践。值得注意的是,我们发现了一种有前景的摘要技术改编方法,在无需调整模型的情况下显著提高了性能。Spacewalk-18基准测试发布在https://brown-palm.github.io/Spacewalk-18/上。
论文及项目相关链接
PDF Under submission. Code and models will be released at https://brown-palm.github.io/Spacewalk-18/
Summary
本文介绍了视频理解模型在获取技能方面的作用,并指出模型需要具备结构化理解能力,如将演示分解为动作序列和技能的时间分割等,并能够推广至新环境、任务和领域。为达到此目标,文章引入了Spacewalk-18基准测试,包含两个任务:步骤识别和基于国际空间站太空行走记录的视频问答。通过这两个任务,可以量化模型在新领域的泛化能力、利用长期时间上下文和多模态信息的能力。文章还分析了Spacewalk-18的挑战,提出了针对领域泛化和长时理解的最佳实践,并发现了一种摘要技术的方法可以显著提高性能,无需对模型进行微调。
Key Takeaways
- 视频理解模型已成为获取技能的重要工具,尤其对于实体代理来说。
- 模型需要具备结构化理解能力,包括时间分割和动作序列识别等。
- 文章介绍了Spacewalk-18基准测试,该测试旨在评估模型的泛化能力和使用长期时间上下文和多模态信息的能力。
- Spacewalk-18包含两个任务:步骤识别和基于太空行走记录的视频问答。
- 文章对Spacewalk-18基准测试的挑战进行了深入剖析,强调了其实践中的难点。
- 文章发现了一种通过摘要技术提高模型性能的方法,无需进行模型微调。
点此查看论文截图
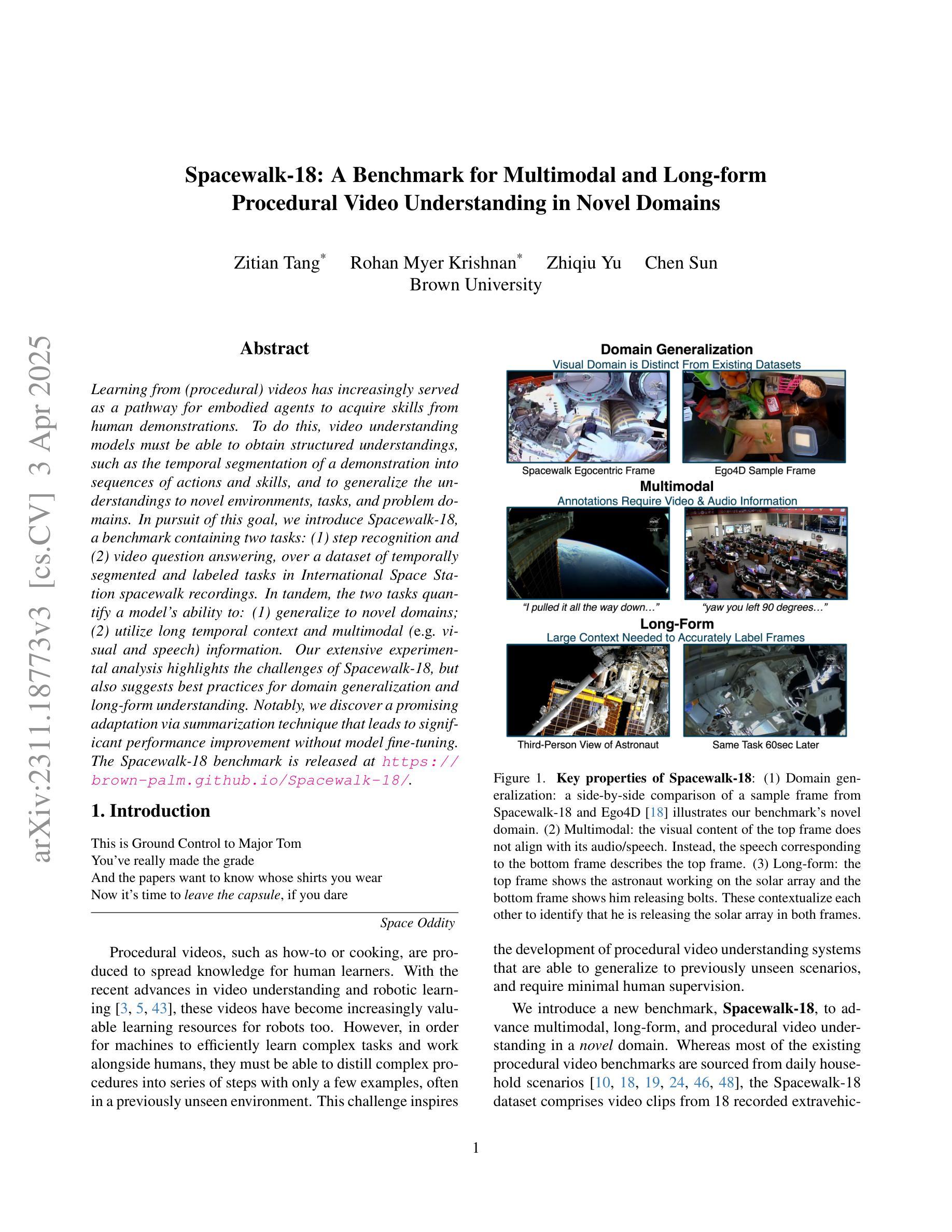
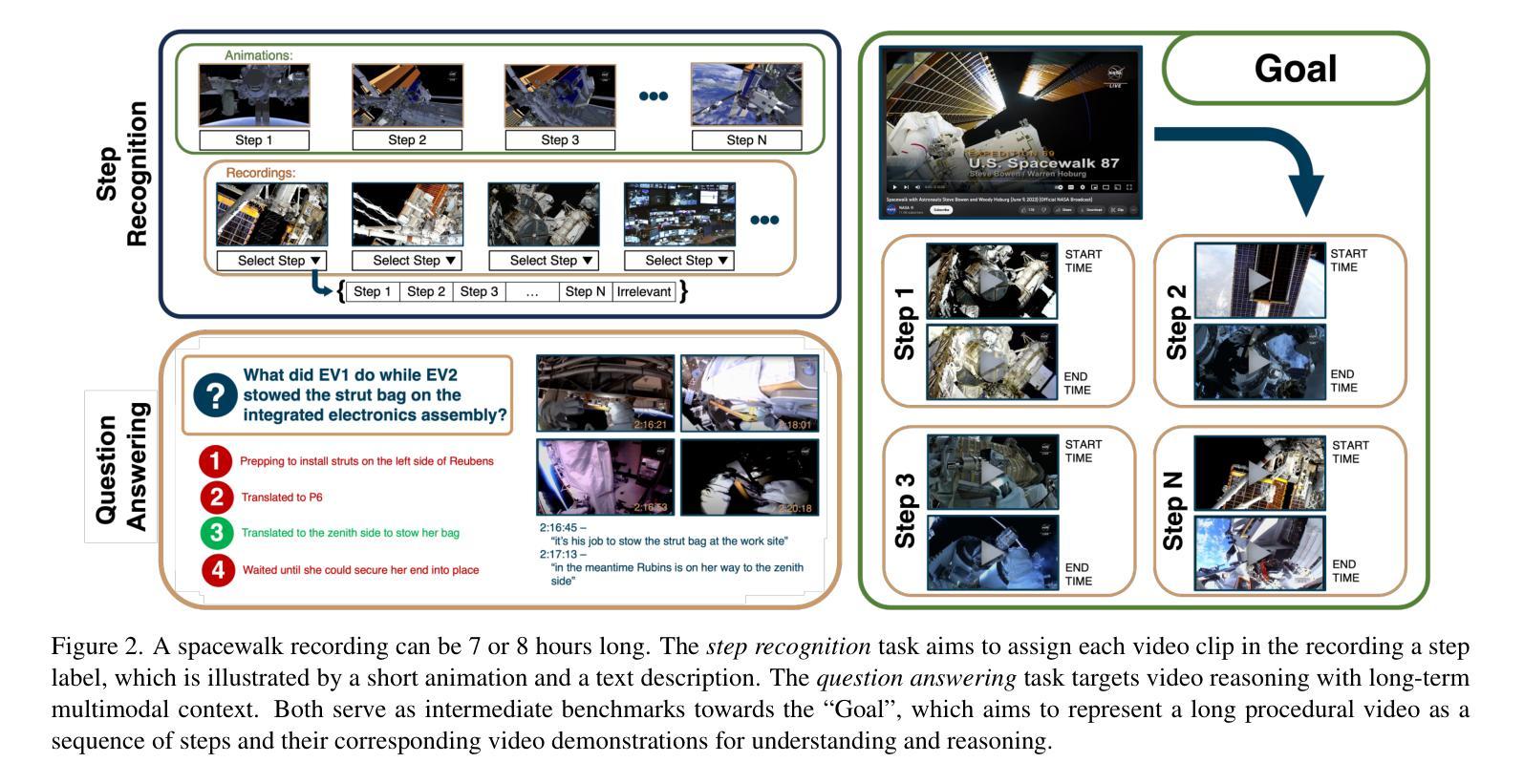
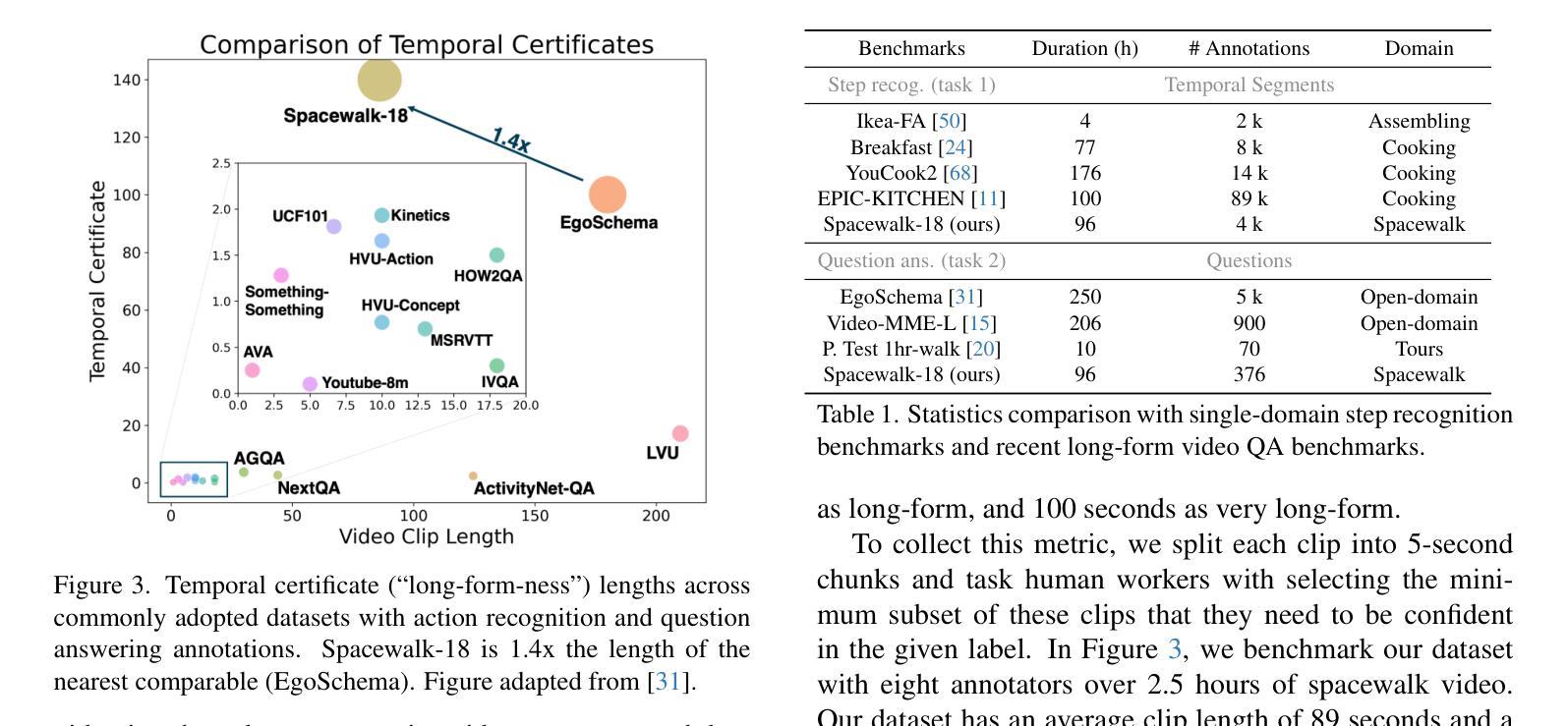