⚠️ 以下所有内容总结都来自于 大语言模型的能力,如有错误,仅供参考,谨慎使用
🔴 请注意:千万不要用于严肃的学术场景,只能用于论文阅读前的初筛!
💗 如果您觉得我们的项目对您有帮助 ChatPaperFree ,还请您给我们一些鼓励!⭐️ HuggingFace免费体验
2025-04-08 更新
Multimodal Diffusion Bridge with Attention-Based SAR Fusion for Satellite Image Cloud Removal
Authors:Yuyang Hu, Suhas Lohit, Ulugbek S. Kamilov, Tim K. Marks
Deep learning has achieved some success in addressing the challenge of cloud removal in optical satellite images, by fusing with synthetic aperture radar (SAR) images. Recently, diffusion models have emerged as powerful tools for cloud removal, delivering higher-quality estimation by sampling from cloud-free distributions, compared to earlier methods. However, diffusion models initiate sampling from pure Gaussian noise, which complicates the sampling trajectory and results in suboptimal performance. Also, current methods fall short in effectively fusing SAR and optical data. To address these limitations, we propose Diffusion Bridges for Cloud Removal, DB-CR, which directly bridges between the cloudy and cloud-free image distributions. In addition, we propose a novel multimodal diffusion bridge architecture with a two-branch backbone for multimodal image restoration, incorporating an efficient backbone and dedicated cross-modality fusion blocks to effectively extract and fuse features from synthetic aperture radar (SAR) and optical images. By formulating cloud removal as a diffusion-bridge problem and leveraging this tailored architecture, DB-CR achieves high-fidelity results while being computationally efficient. We evaluated DB-CR on the SEN12MS-CR cloud-removal dataset, demonstrating that it achieves state-of-the-art results.
深度学习通过融合合成孔径雷达(SAR)图像,在解决光学卫星图像中的去云挑战方面取得了一些成功。最近,扩散模型作为去云的有力工具崭露头角,通过从无云分布中进行采样,与早期方法相比,实现了更高质量估计。然而,扩散模型从纯高斯噪声开始进行采样,这使得采样轨迹复杂化,导致性能不佳。此外,当前的方法在有效地融合SAR和光学数据方面还存在不足。为了克服这些局限性,我们提出了去云扩散桥(DB-CR),它直接连接了带云和无云图像分布之间的桥梁。此外,我们提出了一种新型的多模态扩散桥架构,该架构具有两分支主干,用于多模态图像恢复,结合了高效的主干和专用的跨模态融合块,以有效地从合成孔径雷达(SAR)和光学图像中提取和融合特征。通过将去云问题表述为扩散桥问题并利用这一量身定制的架构,DB-CR在获得高保真结果的同时还具有计算效率高的特点。我们在SEN12MS-CR去云数据集上评估了DB-CR,结果表明它达到了最先进的水平。
论文及项目相关链接
Summary
本文介绍了深度学习在解决光学卫星图像中的云去除挑战方面的进展,特别是通过合成孔径雷达(SAR)图像融合的方法。虽然扩散模型在云去除方面展现出强大的潜力,但由于其从纯高斯噪声开始采样,导致采样轨迹复杂且性能不佳。为了克服这些限制,提出了名为“DB-CR”的扩散桥云去除方法,该方法直接连接带云和去云图像分布,并采用了新颖的多模态扩散桥架构,实现高效的多模态图像恢复。DB-CR在云去除问题上表现出卓越效果。
Key Takeaways
- 深度学习已成功应用于光学卫星图像中的云去除挑战。
- 扩散模型是云去除的有力工具,但采样过程复杂且性能有待提升。
- 当前方法难以有效融合SAR和光学数据。
- 提出了名为“DB-CR”的扩散桥云去除方法,直接连接带云和去云图像分布。
- DB-CR采用新颖的多模态扩散桥架构,包括两分支骨干网和多模态特征融合模块,提高特征提取与融合能力。
- DB-CR方法在云去除问题上实现高保真度且计算高效的结果。
点此查看论文截图
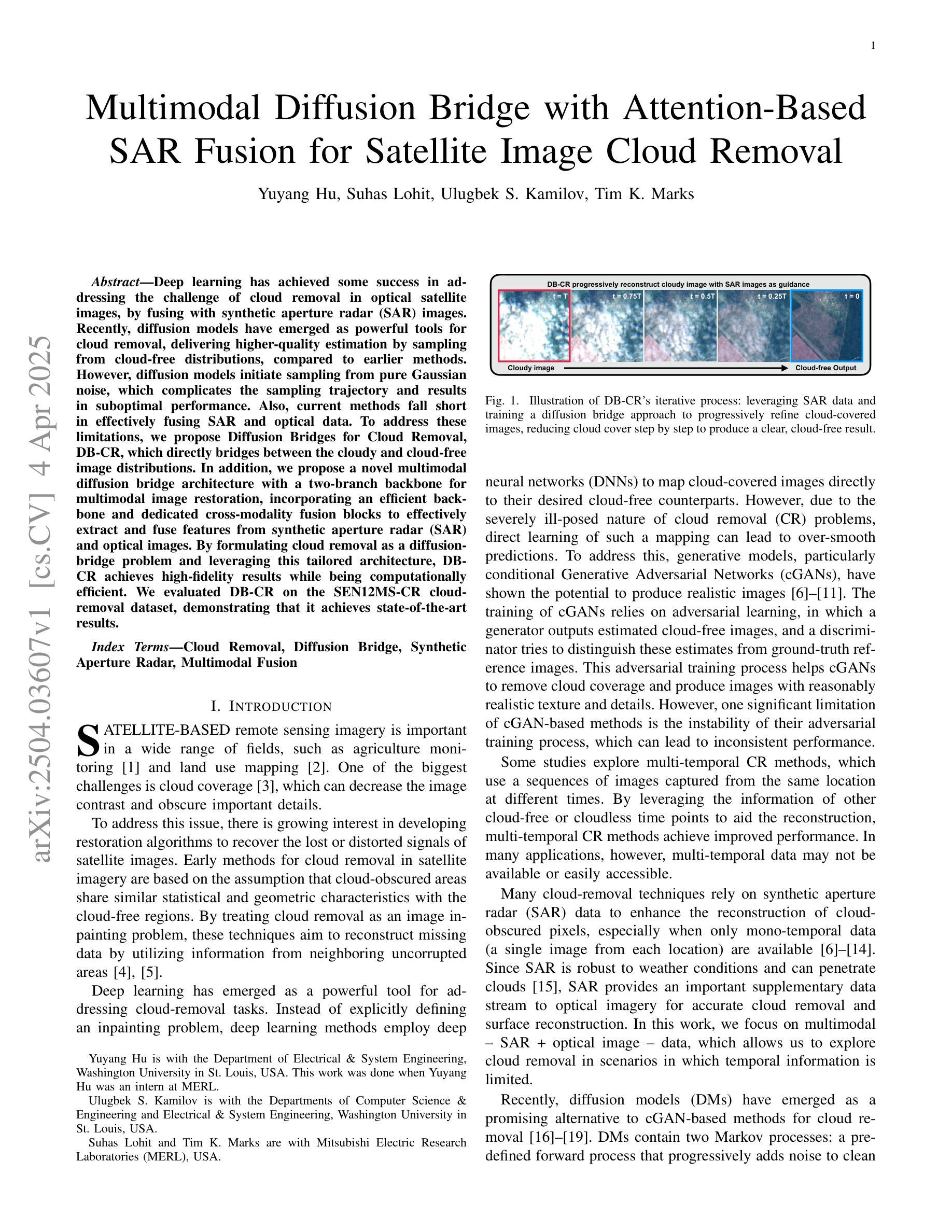
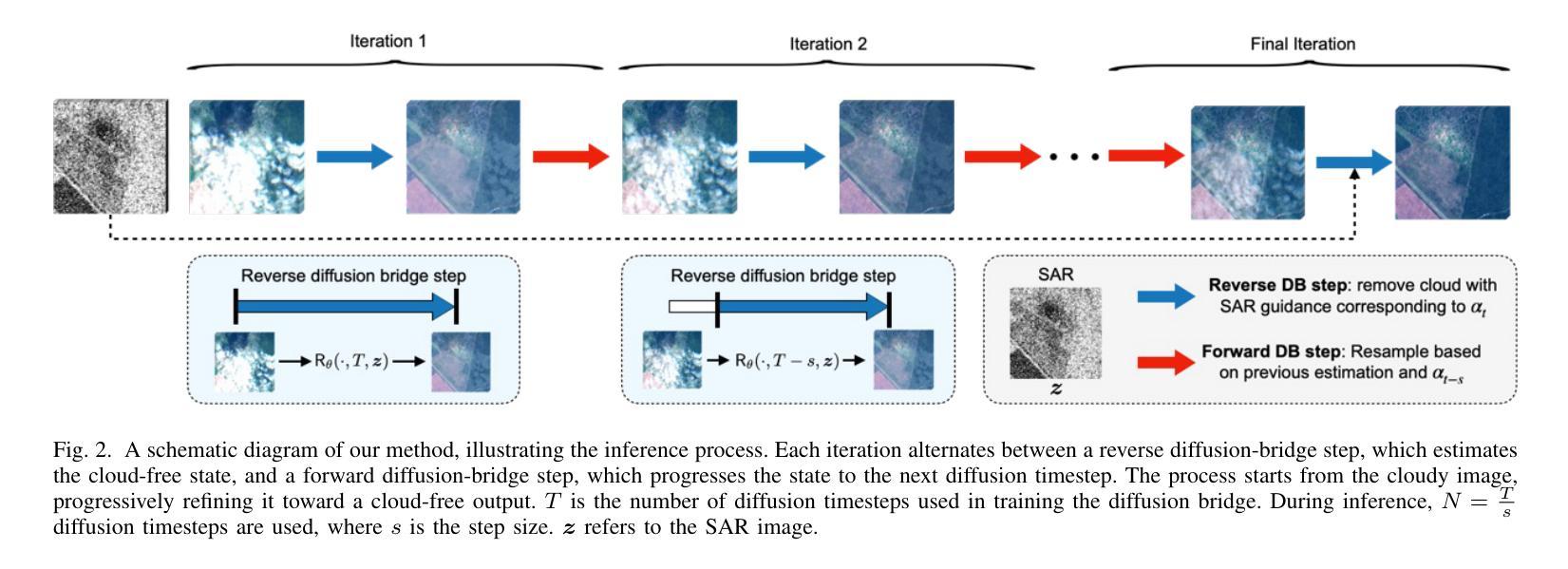
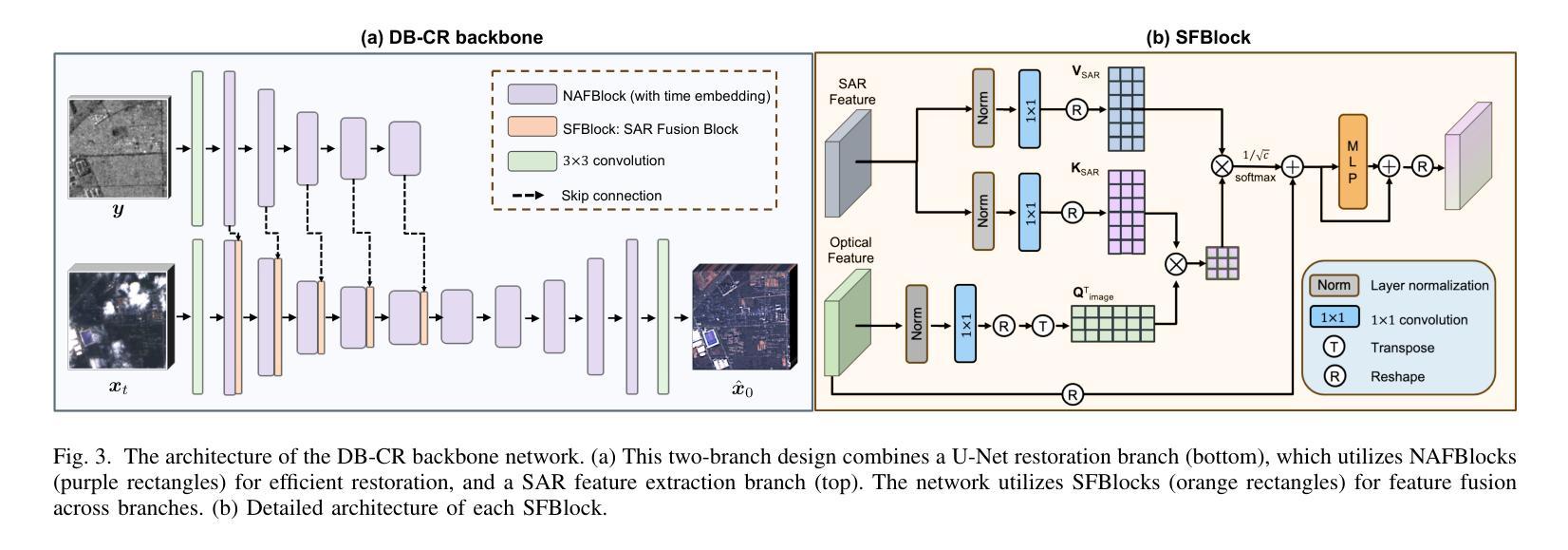
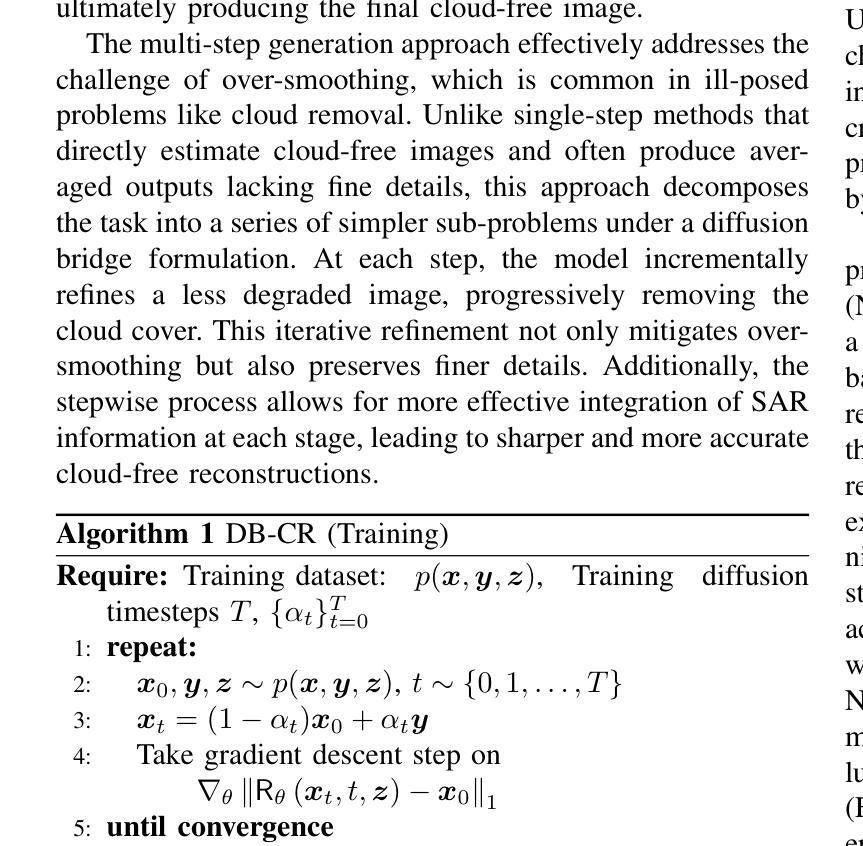
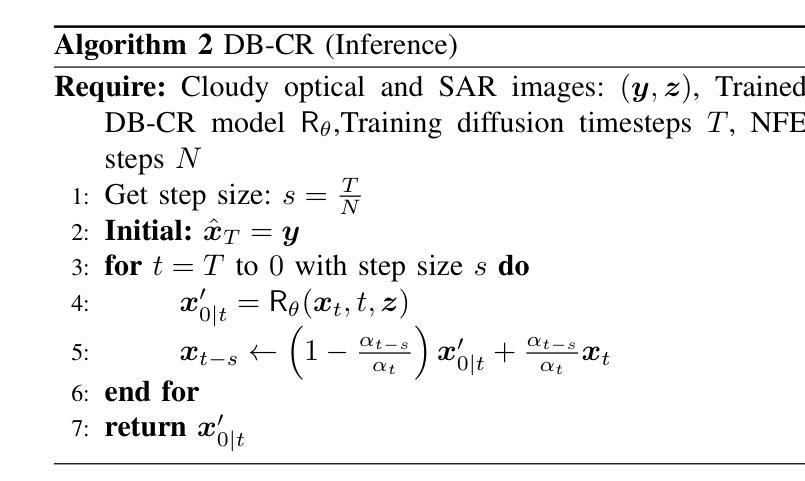
BUFF: Bayesian Uncertainty Guided Diffusion Probabilistic Model for Single Image Super-Resolution
Authors:Zihao He, Shengchuan Zhang, Runze Hu, Yunhang Shen, Yan Zhang
Super-resolution (SR) techniques are critical for enhancing image quality, particularly in scenarios where high-resolution imagery is essential yet limited by hardware constraints. Existing diffusion models for SR have relied predominantly on Gaussian models for noise generation, which often fall short when dealing with the complex and variable texture inherent in natural scenes. To address these deficiencies, we introduce the Bayesian Uncertainty Guided Diffusion Probabilistic Model (BUFF). BUFF distinguishes itself by incorporating a Bayesian network to generate high-resolution uncertainty masks. These masks guide the diffusion process, allowing for the adjustment of noise intensity in a manner that is both context-aware and adaptive. This novel approach not only enhances the fidelity of super-resolved images to their original high-resolution counterparts but also significantly mitigates artifacts and blurring in areas characterized by complex textures and fine details. The model demonstrates exceptional robustness against complex noise patterns and showcases superior adaptability in handling textures and edges within images. Empirical evidence, supported by visual results, illustrates the model’s robustness, especially in challenging scenarios, and its effectiveness in addressing common SR issues such as blurring. Experimental evaluations conducted on the DIV2K dataset reveal that BUFF achieves a notable improvement, with a +0.61 increase compared to baseline in SSIM on BSD100, surpassing traditional diffusion approaches by an average additional +0.20dB PSNR gain. These findings underscore the potential of Bayesian methods in enhancing diffusion processes for SR, paving the way for future advancements in the field.
超分辨率(SR)技术对于提高图像质量至关重要,特别是在需要高分辨率图像但由于硬件限制而受限的场景中尤为重要。现有的扩散模型主要应用于SR中的噪声生成,主要依赖于高斯模型,在处理自然场景中固有的复杂多变纹理时,往往表现不佳。为了解决这些不足,我们引入了贝叶斯不确定性引导扩散概率模型(BUFF)。BUFF通过融入贝叶斯网络来生成高分辨率不确定性掩膜,从而实现自我区分。这些掩膜引导扩散过程,使噪声强度的调整具有情境感知和适应性。这种新颖的方法不仅提高了超分辨率图像的保真度,使其更接近原始高分辨率图像,还显著减轻了复杂纹理和细节区域的伪影和模糊。该模型在应对复杂的噪声模式时表现出出色的稳健性,在处理图像中的纹理和边缘时展现出卓越的适应性。有视觉结果支持的实证证据表明,该模型在具有挑战性的场景中特别稳健,并且在解决SR的常见问题(如模糊)方面非常有效。在DIV2K数据集上进行的实验评估表明,BUFF在BSD100上的SSIM指数提高了+0.61,与传统扩散方法相比,平均PSNR增益提高了+0.20dB。这些发现凸显了贝叶斯方法在增强SR中的扩散过程的潜力,为未来的技术进步奠定了基础。
论文及项目相关链接
PDF 9 pages, 5 figures, AAAI 2025
Summary
本文介绍了一种基于贝叶斯不确定性引导扩散概率模型(BUFF)的超分辨率技术。该模型通过引入贝叶斯网络生成高分辨率不确定性掩膜,指导扩散过程,自适应地调整噪声强度,从而提高超分辨率图像的保真度,并显著减少复杂纹理和细节区域的伪影和模糊。模型在复杂噪声模式下表现出强大的稳健性,并在处理图像纹理和边缘方面表现出优异的适应性。在DIV2K数据集上的实验评估显示,与基线相比,BUFF在BSD100上的SSIM增加了0.61,平均PSNR增益比传统扩散方法高出+0.20dB。这显示了贝叶斯方法在增强扩散过程的超分辨率技术中的潜力。
Key Takeaways
- BUFF模型引入贝叶斯网络生成高分辨率不确定性掩膜,用于指导扩散过程。
- 该模型能够自适应地调整噪声强度,提高超分辨率图像的保真度。
- BUFF模型在复杂纹理和细节区域的伪影和模糊减少方面表现出色。
- 模型在复杂噪声模式下具有强大的稳健性。
- BUFF模型在处理图像纹理和边缘方面表现出优异的适应性。
- 在DIV2K数据集上的实验评估显示,与基线相比,BUFF模型在SSIM和PSNR指标上均有显著提高。
点此查看论文截图
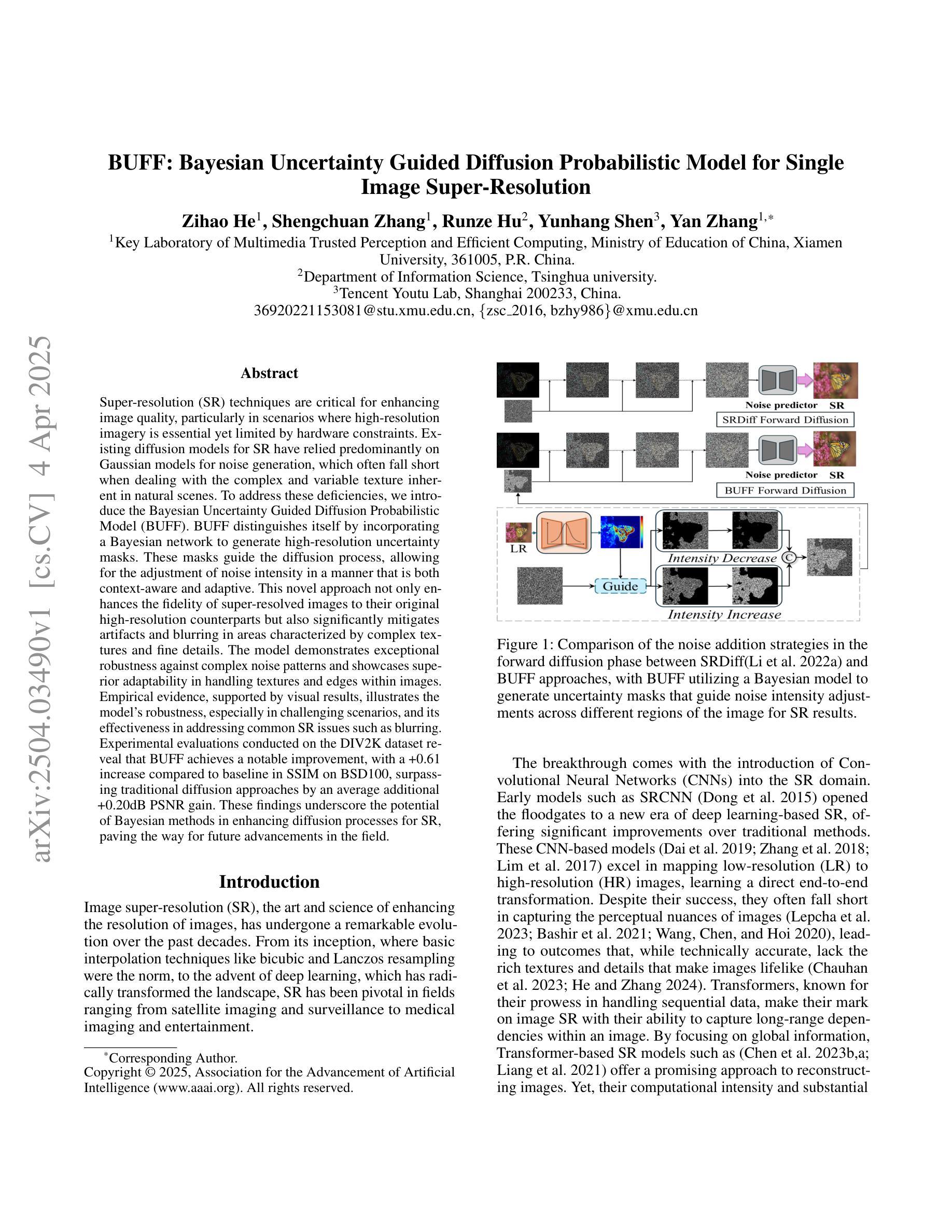
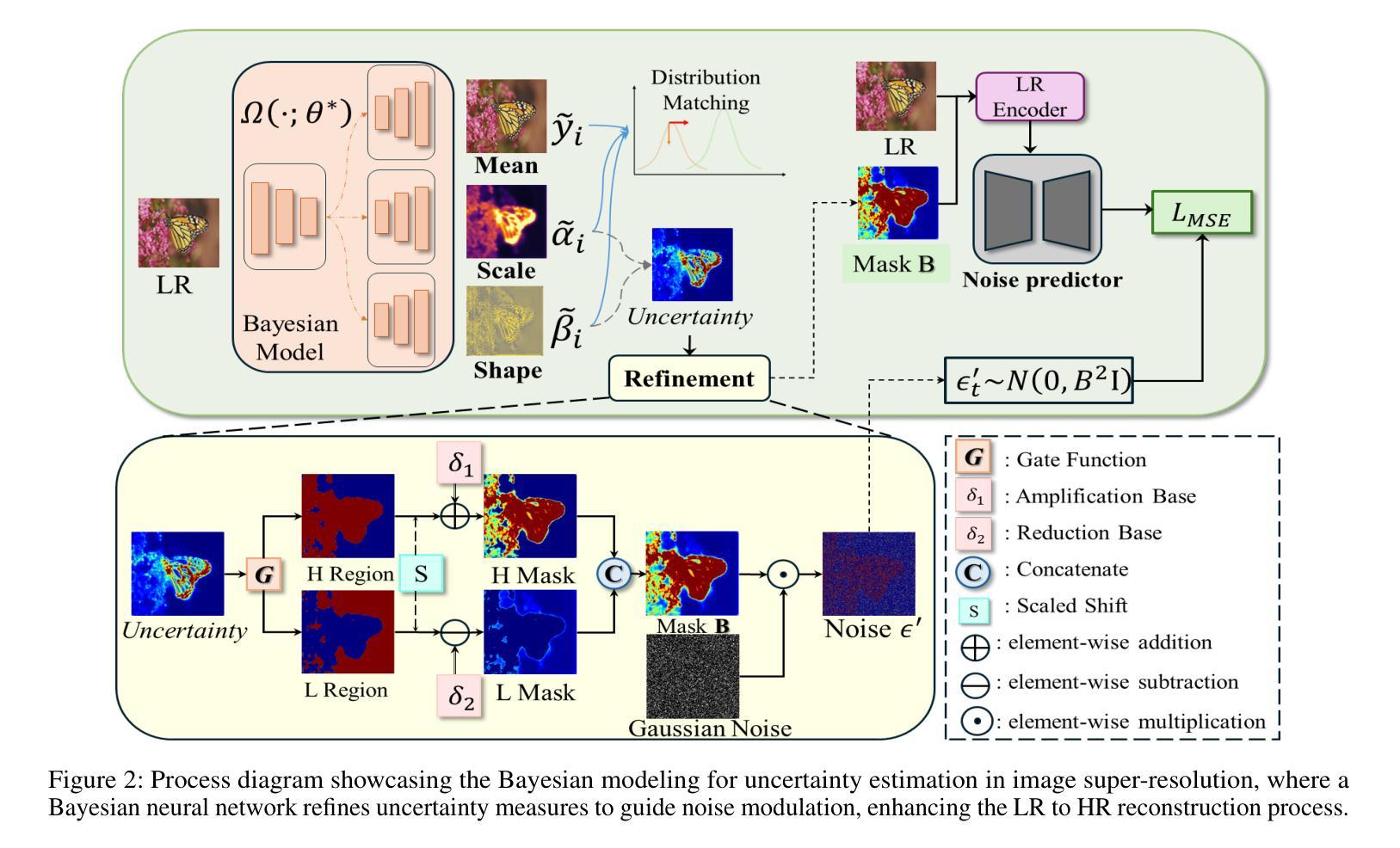
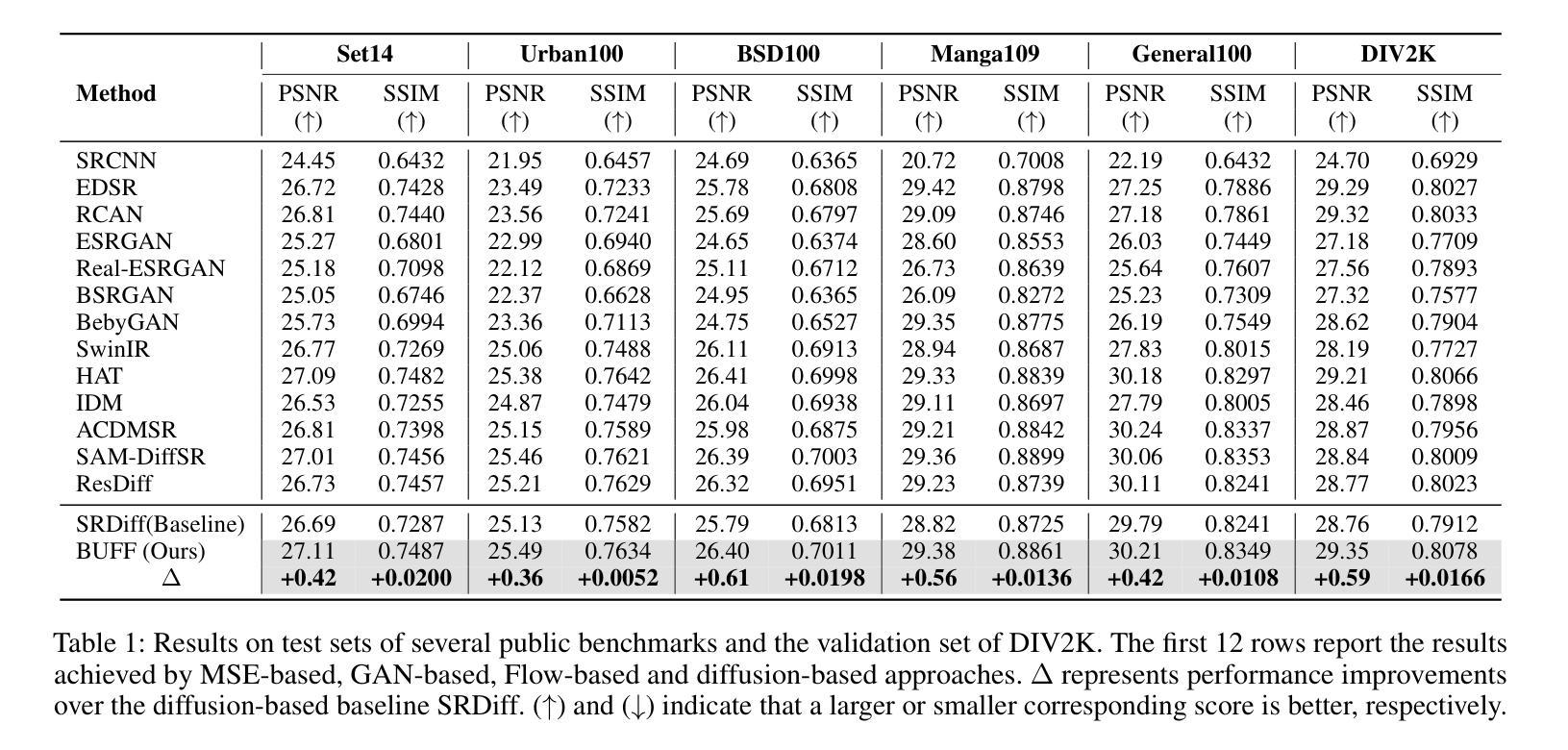
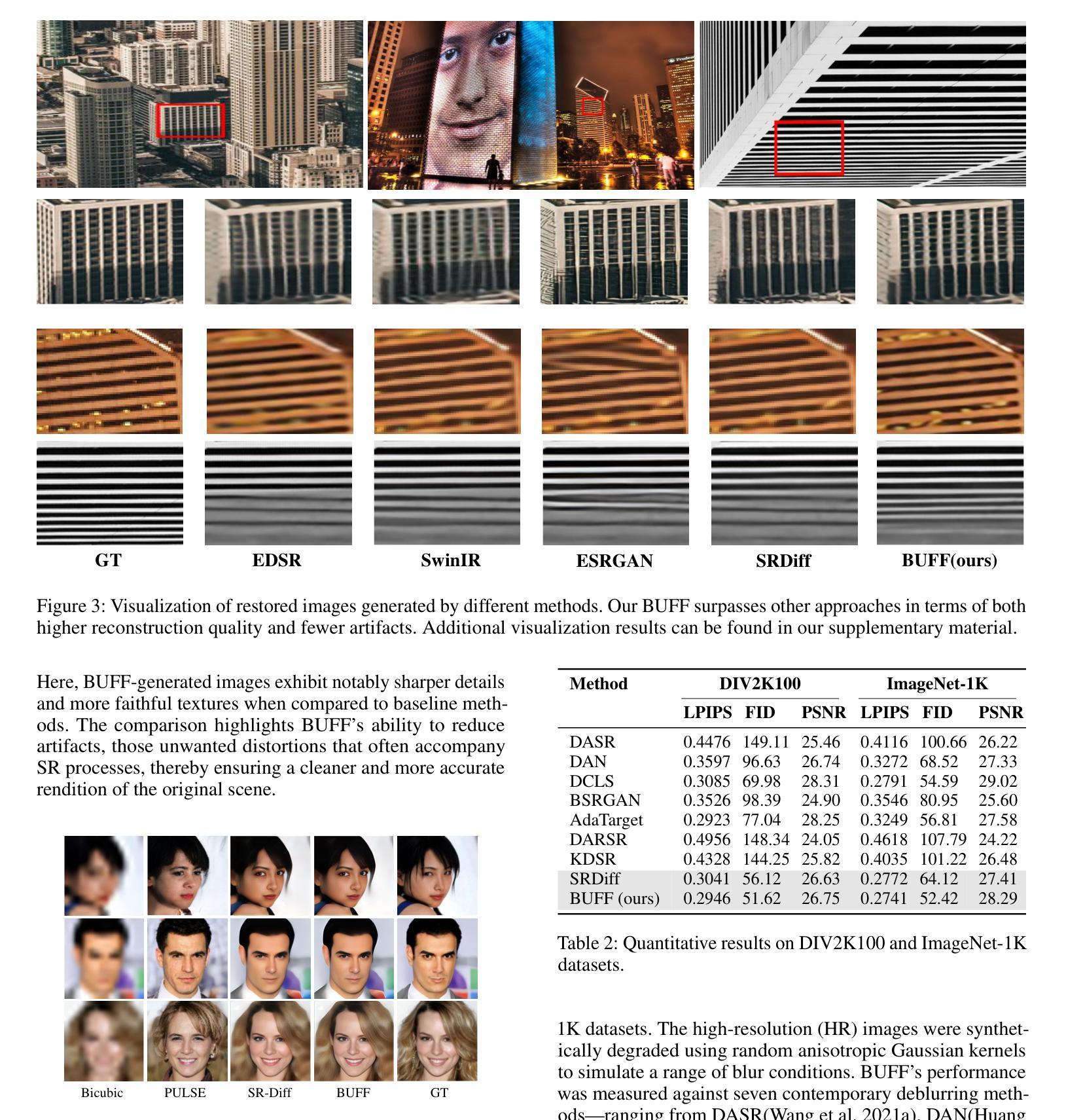
Dynamic Importance in Diffusion U-Net for Enhanced Image Synthesis
Authors:Xi Wang, Ziqi He, Yang Zhou
Traditional diffusion models typically employ a U-Net architecture. Previous studies have unveiled the roles of attention blocks in the U-Net. However, they overlook the dynamic evolution of their importance during the inference process, which hinders their further exploitation to improve image applications. In this study, we first theoretically proved that, re-weighting the outputs of the Transformer blocks within the U-Net is a “free lunch” for improving the signal-to-noise ratio during the sampling process. Next, we proposed Importance Probe to uncover and quantify the dynamic shifts in importance of the Transformer blocks throughout the denoising process. Finally, we design an adaptive importance-based re-weighting schedule tailored to specific image generation and editing tasks. Experimental results demonstrate that, our approach significantly improves the efficiency of the inference process, and enhances the aesthetic quality of the samples with identity consistency. Our method can be seamlessly integrated into any U-Net-based architecture. Code: https://github.com/Hytidel/UNetReweighting
传统扩散模型通常采用U-Net架构。之前的研究已经揭示了注意力块在U-Net中的作用。然而,他们忽略了推理过程中注意力块重要性动态变化的影响,这阻碍了进一步利用来提高图像应用的效果。在本研究中,我们首先从理论上证明了重新调整U-Net中Transformer块的输出,可以提高采样过程中的信噪比,这是一个“免费午餐”。接下来,我们提出了重要性探针(Importance Probe),以揭示并量化降噪过程中Transformer块重要性的动态变化。最后,我们设计了一种基于特定图像生成和编辑任务的自适应重要性重新加权调度策略。实验结果表明,我们的方法显著提高了推理过程的效率,并提高了样本的一致性和美学质量。我们的方法可以无缝集成到任何基于U-Net的架构中。代码:https://github.com/Hytidel/UNetReweighting。
论文及项目相关链接
PDF Accepted to ICME 2025. Appendix & Code: https://github.com/Hytidel/UNetReweighting
Summary
本文研究了传统扩散模型中的U-Net架构,并指出以往研究忽视了注意力块在推理过程中的动态重要性变化。本研究通过理论证明了重新加权U-Net中Transformer块的输出可以提高采样过程中的信号噪声比。同时,提出了重要性探针(Importance Probe)来揭示和量化去噪过程中Transformer块重要性的动态变化。最终设计了一种基于自适应重要性的重新加权调度,适用于特定的图像生成和编辑任务。实验结果表明,该方法显著提高了推理过程的效率,并提高了样本的美学质量和一致性。
Key Takeaways
- 传统扩散模型主要采用U-Net架构,但对其内部机制的研究不够深入。
- 本研究强调了Transformer块在U-Net中的重要性及其动态变化的重要性。
- 通过理论证明,重新加权Transformer块的输出能够提高信号噪声比,改善采样过程。
- 提出了一种名为“重要性探针”的技术来量化Transformer块在推理过程中的重要性变化。
- 设计了一种自适应重要性的重新加权调度策略,适用于图像生成和编辑任务。
- 实验结果表明,该方法能够提高推理效率并改善图像质量,同时保持一致性。
点此查看论文截图
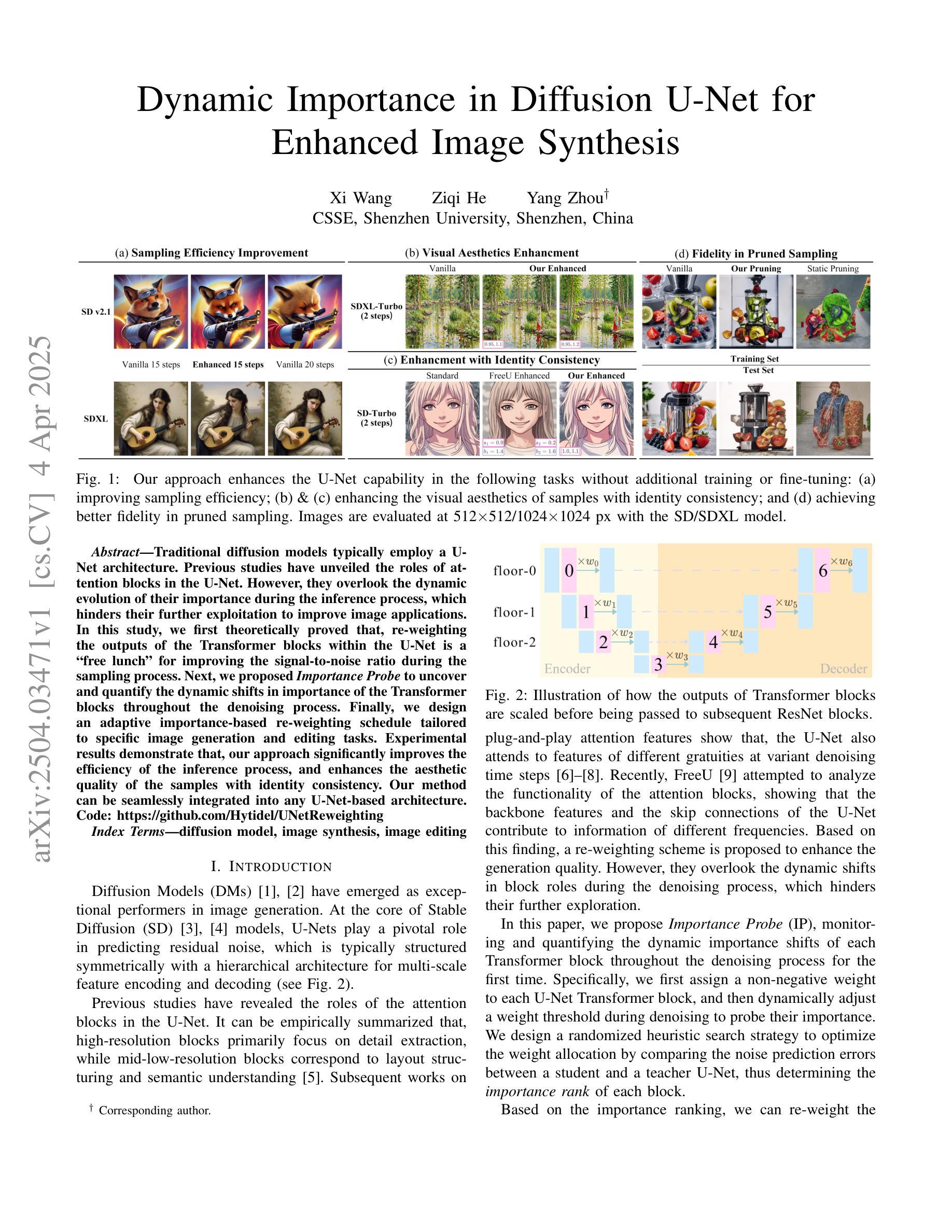
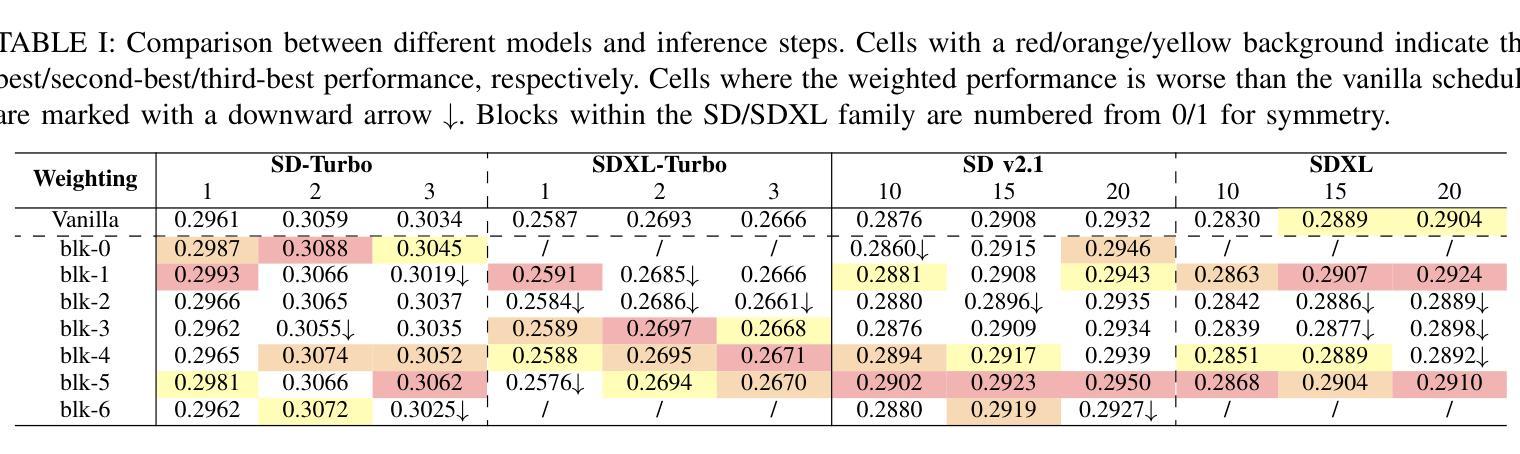
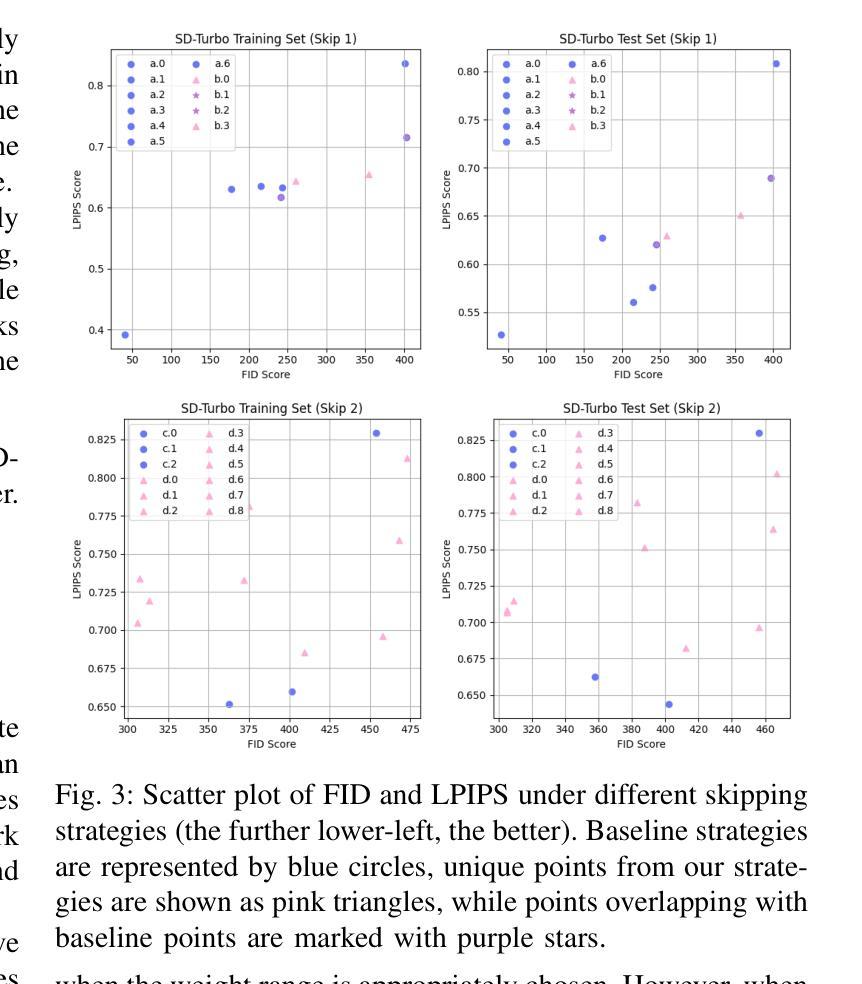
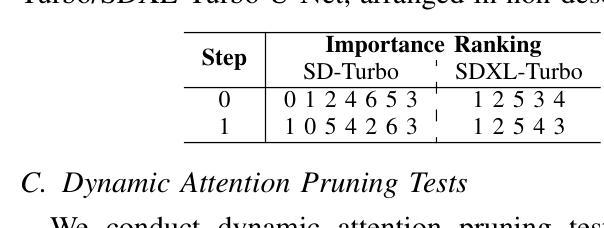
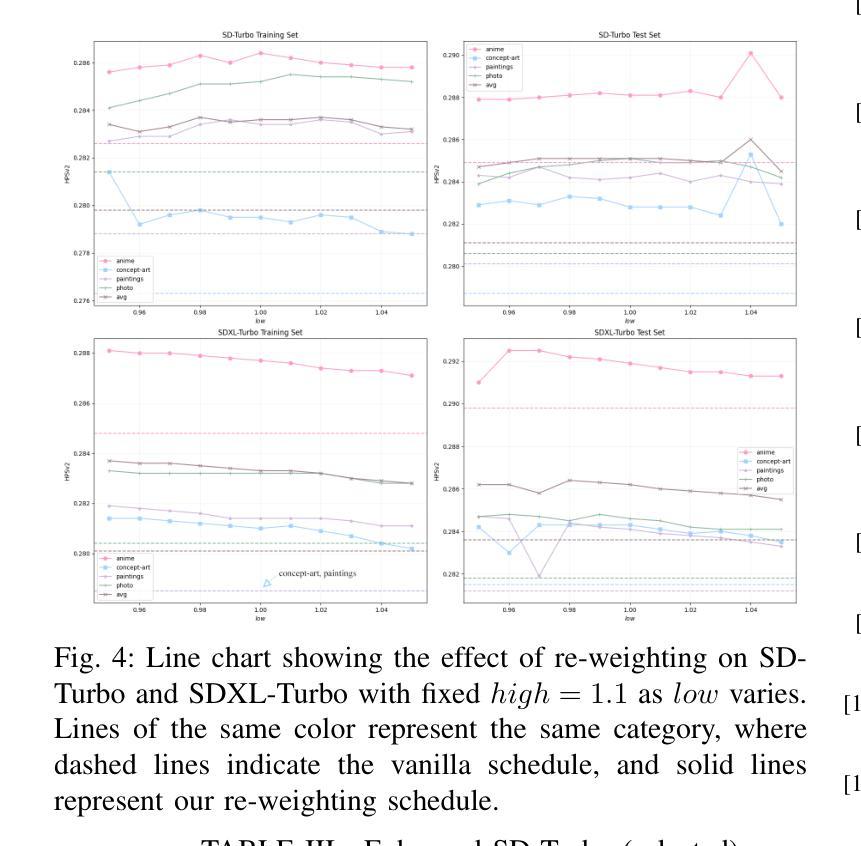
FaR: Enhancing Multi-Concept Text-to-Image Diffusion via Concept Fusion and Localized Refinement
Authors:Gia-Nghia Tran, Quang-Huy Che, Trong-Tai Dam Vu, Bich-Nga Pham, Vinh-Tiep Nguyen, Trung-Nghia Le, Minh-Triet Tran
Generating multiple new concepts remains a challenging problem in the text-to-image task. Current methods often overfit when trained on a small number of samples and struggle with attribute leakage, particularly for class-similar subjects (e.g., two specific dogs). In this paper, we introduce Fuse-and-Refine (FaR), a novel approach that tackles these challenges through two key contributions: Concept Fusion technique and Localized Refinement loss function. Concept Fusion systematically augments the training data by separating reference subjects from backgrounds and recombining them into composite images to increase diversity. This augmentation technique tackles the overfitting problem by mitigating the narrow distribution of the limited training samples. In addition, Localized Refinement loss function is introduced to preserve subject representative attributes by aligning each concept’s attention map to its correct region. This approach effectively prevents attribute leakage by ensuring that the diffusion model distinguishes similar subjects without mixing their attention maps during the denoising process. By fine-tuning specific modules at the same time, FaR balances the learning of new concepts with the retention of previously learned knowledge. Empirical results show that FaR not only prevents overfitting and attribute leakage while maintaining photorealism, but also outperforms other state-of-the-art methods.
在文本到图像的任务中,生成多个新概念仍然是一个具有挑战性的问题。当前的方法在少量样本上进行训练时经常会出现过拟合的情况,并且在属性泄露方面存在困难,特别是对于类似类别的主题(例如两只特定的狗)。在本文中,我们介绍了Fuse-and-Refine(FaR),这是一种通过两个关键贡献解决这些挑战的新方法:Concept Fusion技术和Localized Refinement损失函数。Concept Fusion通过分离参考主题和背景并将其重新组合成复合图像来系统地增强训练数据,从而增加多样性。这种增强技术通过减轻有限训练样本的狭窄分布来解决过拟合问题。此外,还引入了Localized Refinement损失函数,通过将对每个概念的注意力图与其正确区域对齐来保留主题代表性属性。这种方法有效地防止了属性泄露,确保扩散模型在降噪过程中区分类似主题,而不会混淆其注意力图。通过同时微调特定模块,FaR在学习新概念和保留已学知识之间取得了平衡。经验结果表明,FaR在保持逼真性的同时,防止了过拟合和属性泄露,并且优于其他最先进的方法。
论文及项目相关链接
Summary
本文提出一种名为Fuse-and-Refine(FaR)的新方法,用于解决文本到图像生成任务中的多个新概念生成难题。该方法通过概念融合技术和局部细化损失函数两大关键贡献,解决了小样本训练时的过拟合问题和类似类别的属性泄露问题。概念融合通过分离参考主体与背景并重新组合成复合图像,增加了训练的多样性。局部细化损失函数则确保每个概念的注意力图与其正确区域对齐,防止属性泄露。实验结果显示,FaR在防止过拟合和属性泄露的同时保持高度逼真感,并优于其他先进方法。
Key Takeaways
- 当前文本到图像生成方法在生成多个新概念时面临挑战,特别是在小样本训练时容易过拟合,且面临类似类别的属性泄露问题。
- 引入的Fuse-and-Refine(FaR)方法通过概念融合技术和局部细化损失函数解决这些问题。
- 概念融合通过分离和重组参考主体与背景,增加训练数据的多样性,缓解过拟合问题。
- 局部细化损失函数确保每个概念的注意力图正确对齐,防止属性泄露。
- FaR方法通过同时微调特定模块,平衡新概念的学习与已学知识的保留。
- 实证研究结果显示,FaR在防止过拟合和属性泄露的同时保持图像的逼真度。
点此查看论文截图
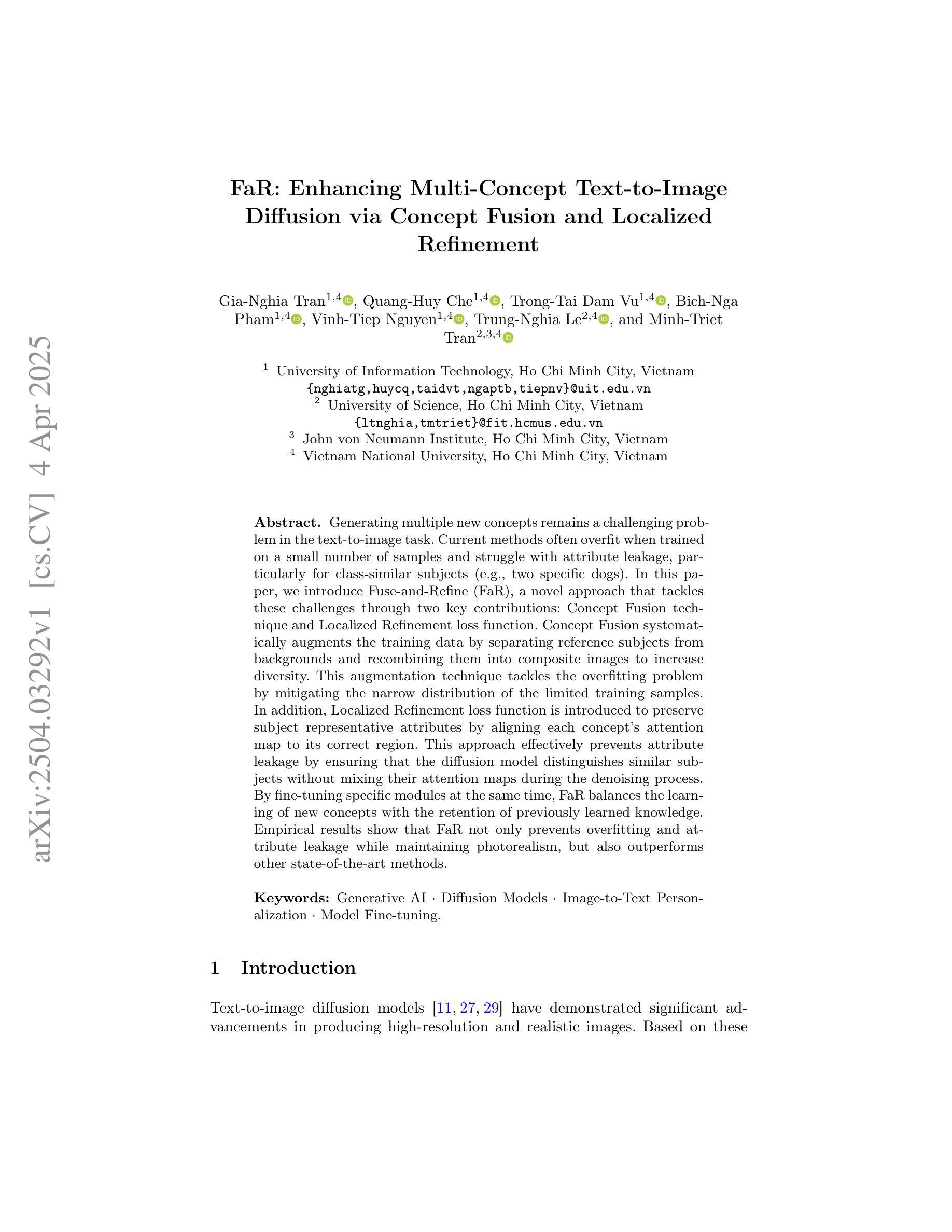
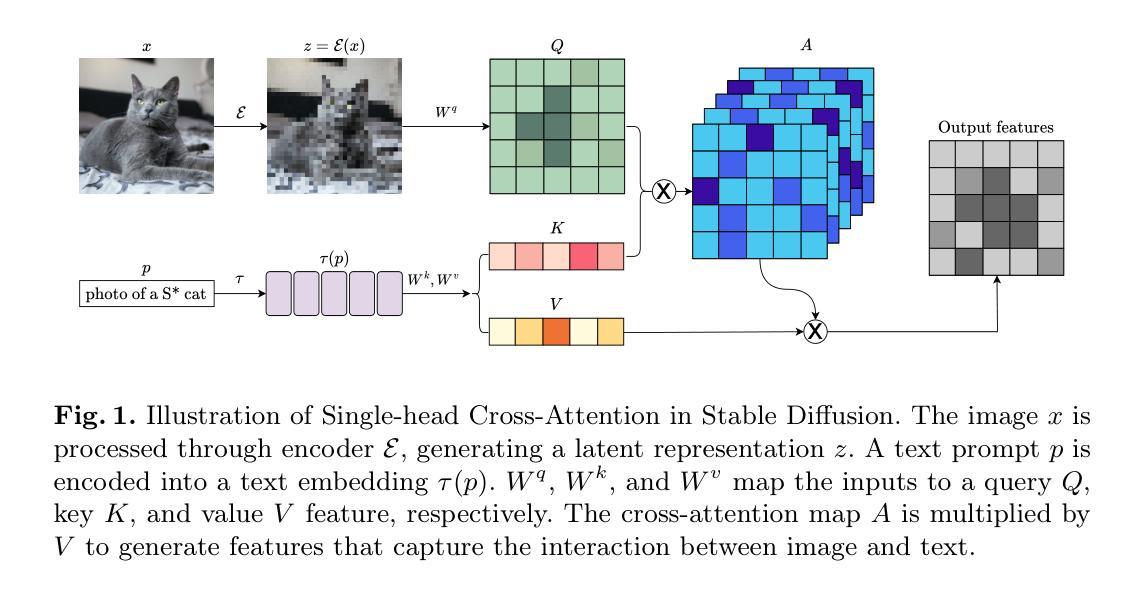
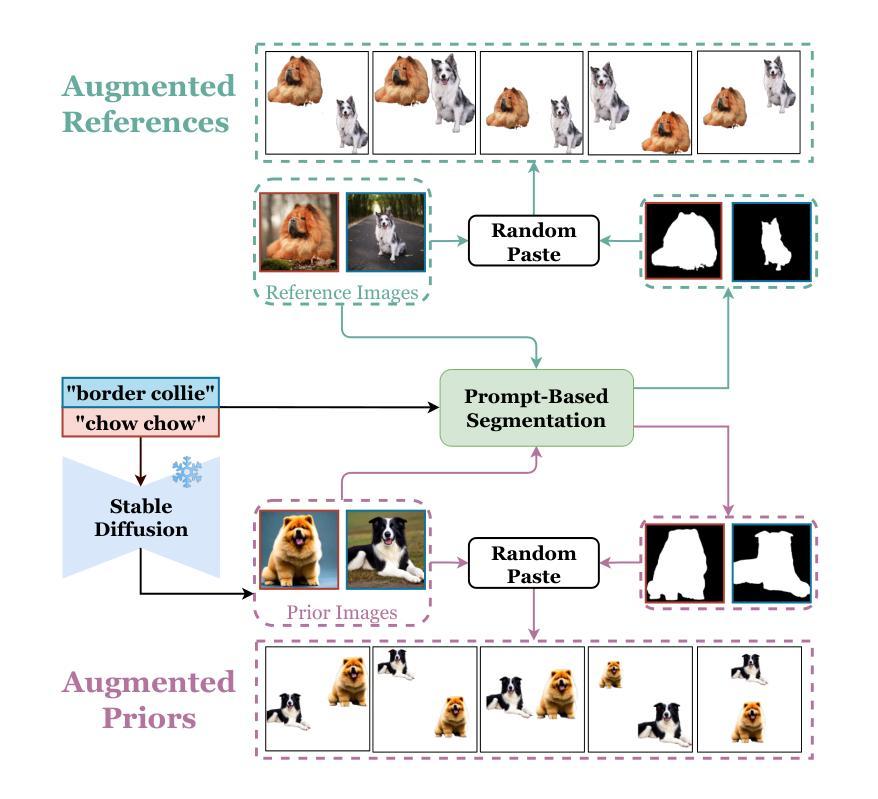
How I Warped Your Noise: a Temporally-Correlated Noise Prior for Diffusion Models
Authors:Pascal Chang, Jingwei Tang, Markus Gross, Vinicius C. Azevedo
Video editing and generation methods often rely on pre-trained image-based diffusion models. During the diffusion process, however, the reliance on rudimentary noise sampling techniques that do not preserve correlations present in subsequent frames of a video is detrimental to the quality of the results. This either produces high-frequency flickering, or texture-sticking artifacts that are not amenable to post-processing. With this in mind, we propose a novel method for preserving temporal correlations in a sequence of noise samples. This approach is materialized by a novel noise representation, dubbed $\int$-noise (integral noise), that reinterprets individual noise samples as a continuously integrated noise field: pixel values do not represent discrete values, but are rather the integral of an underlying infinite-resolution noise over the pixel area. Additionally, we propose a carefully tailored transport method that uses $\int$-noise to accurately advect noise samples over a sequence of frames, maximizing the correlation between different frames while also preserving the noise properties. Our results demonstrate that the proposed $\int$-noise can be used for a variety of tasks, such as video restoration, surrogate rendering, and conditional video generation. See https://warpyournoise.github.io/ for video results.
视频编辑和生成方法通常依赖于预先训练的基于图像的扩散模型。然而,在扩散过程中,依赖于简单的噪声采样技术会损害结果的质量,因为这些技术不会保留视频中后续帧中存在的相关性。这会产生高频闪烁或纹理粘贴伪影,这些伪影不利于进行后处理。鉴于此,我们提出了一种保留噪声样本序列中时间相关性的新方法。该方法通过一种新型噪声表示来实现,称为$\int$-噪声(积分噪声),它将单个噪声样本重新解释为连续集成的噪声场:像素值不代表离散值,而是基本无限分辨率噪声在像素区域内的积分。此外,我们提出了一种精心定制的传输方法,该方法使用$\int$-噪声准确地将噪声样本传输到一系列帧上,最大限度地提高不同帧之间的相关性,同时保留噪声属性。我们的结果表明,所提出的$\int$-噪声可用于多种任务,如视频修复、替代渲染和条件视频生成。视频结果请参见:[https://warpyournoise.github.io/] 。
论文及项目相关链接
PDF Accepted at ICLR 2024 (Oral)
摘要
视频编辑与生成方法常依赖于预训练的图像扩散模型。当前方法中的噪声采样技术简陋,无法保留视频后续帧中的相关性,影响结果质量,导致高频闪烁或纹理粘贴等瑕疵,难以进行后期处理。为此,我们提出一种保留噪声样本中时序相关性的新方法,通过一种名为$\int$-noise(积分噪声)的新型噪声表征实现,它将单个噪声样本重新解释为连续整合的噪声场:像素值不代表离散值,而是底层无限分辨率噪声在像素区域内的积分。我们还提出了一种精心设计的传输方法,利用$\int$-noise准确地将噪声样本传输到一系列帧上,最大化帧间的相关性,同时保留噪声特性。实验证明,$\int$-noise可用于视频修复、代理渲染和条件视频生成等多项任务。
关键见解
- 当前视频编辑和生成方法依赖预训练的图像扩散模型,但噪声采样技术简陋,导致结果质量下降。
- 积分噪声($\int$-noise)是一种新型噪声表征,将噪声样本解释为连续整合的噪声场。
- $\int$-noise能提高像素值的连续性,通过积分形式表示,使像素值成为底层无限分辨率噪声在像素区域内的积分。
- 提出了一种基于$\int$-noise的传输方法,能准确地将噪声样本传输到一系列帧上。
- 该方法能最大化帧间的相关性,同时保留噪声特性。
- $\int$-noise可用于多种任务,包括视频修复、代理渲染和条件视频生成。
- 可在warpyournoise.github.io网站查看利用$\int$-noise的视频结果。
点此查看论文截图
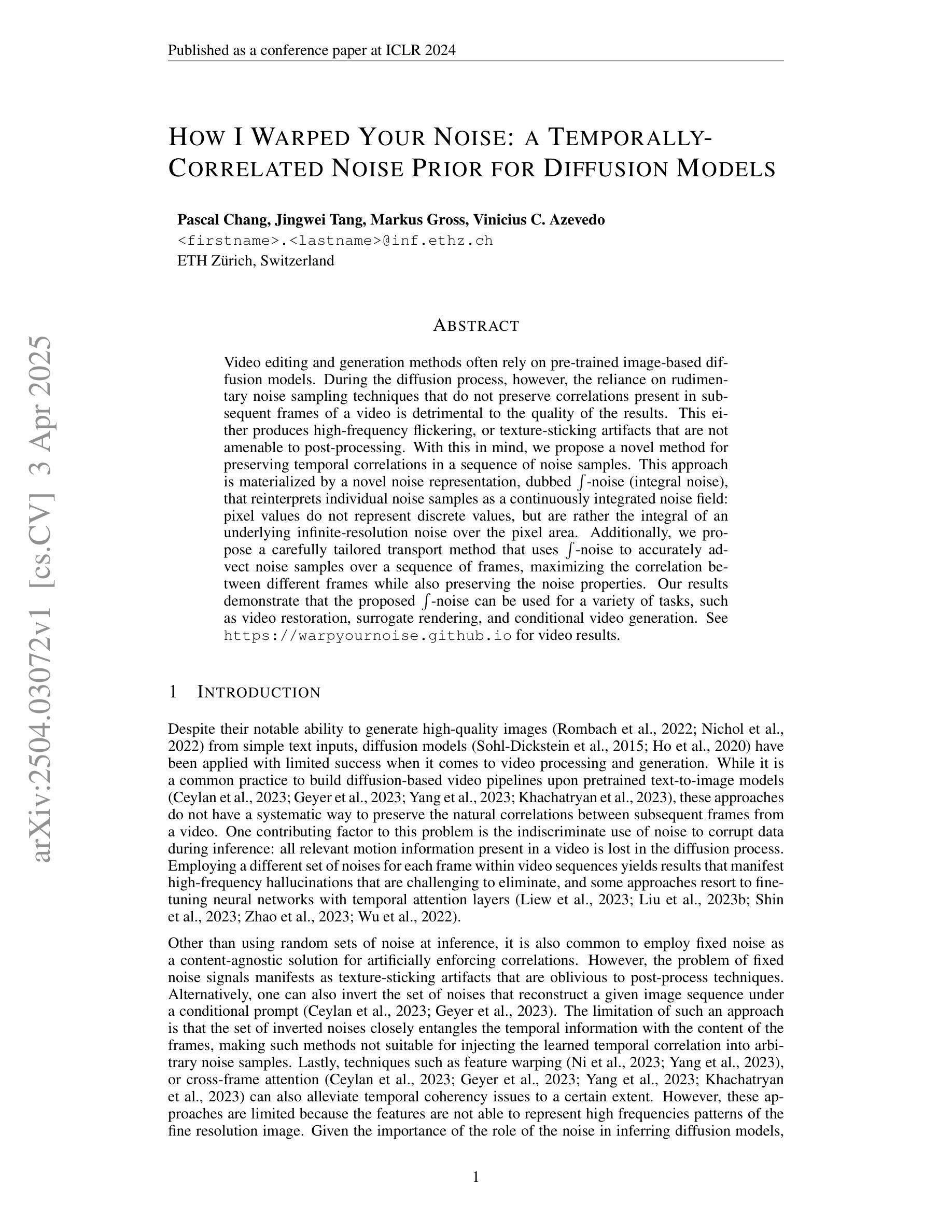
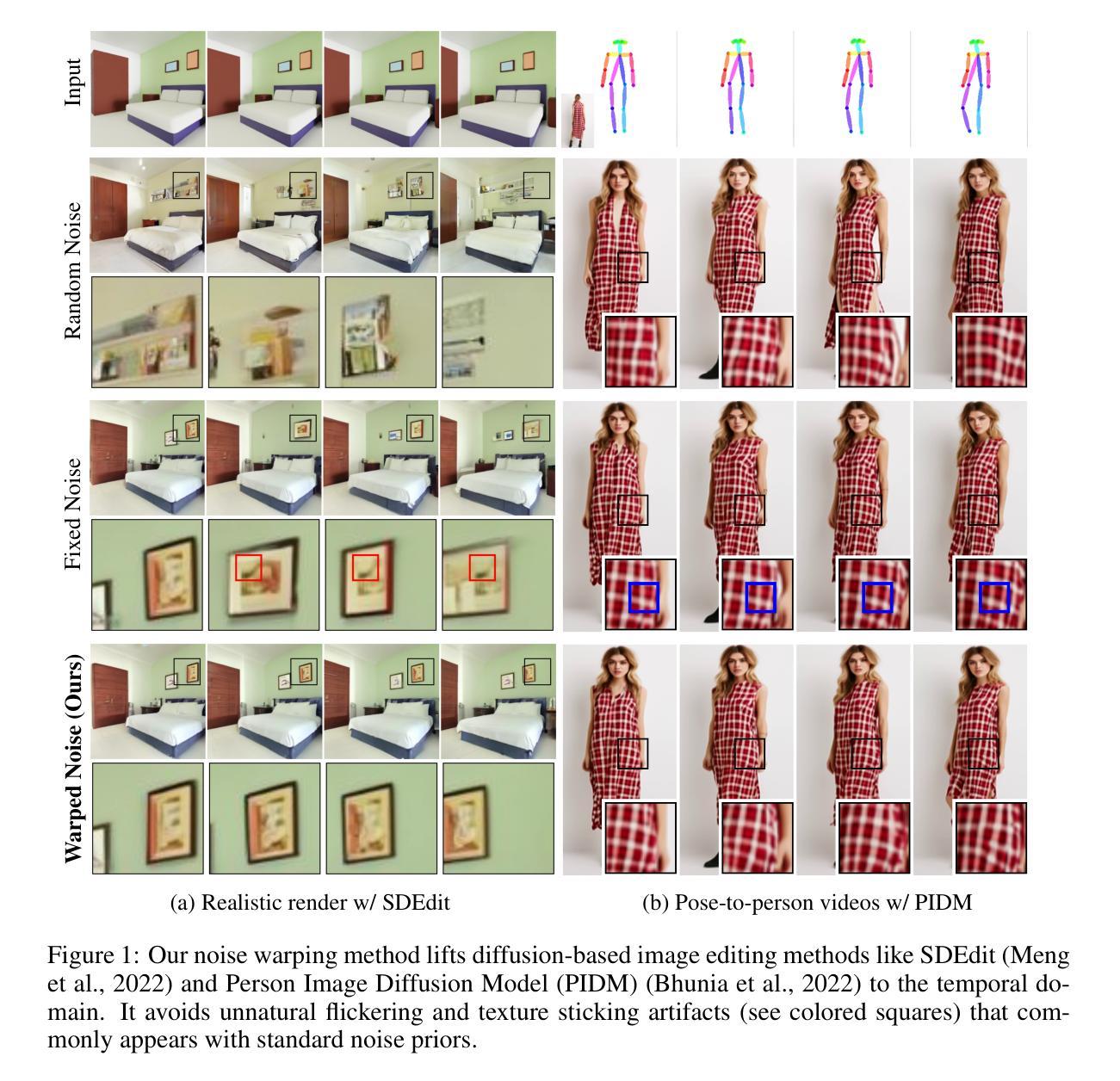
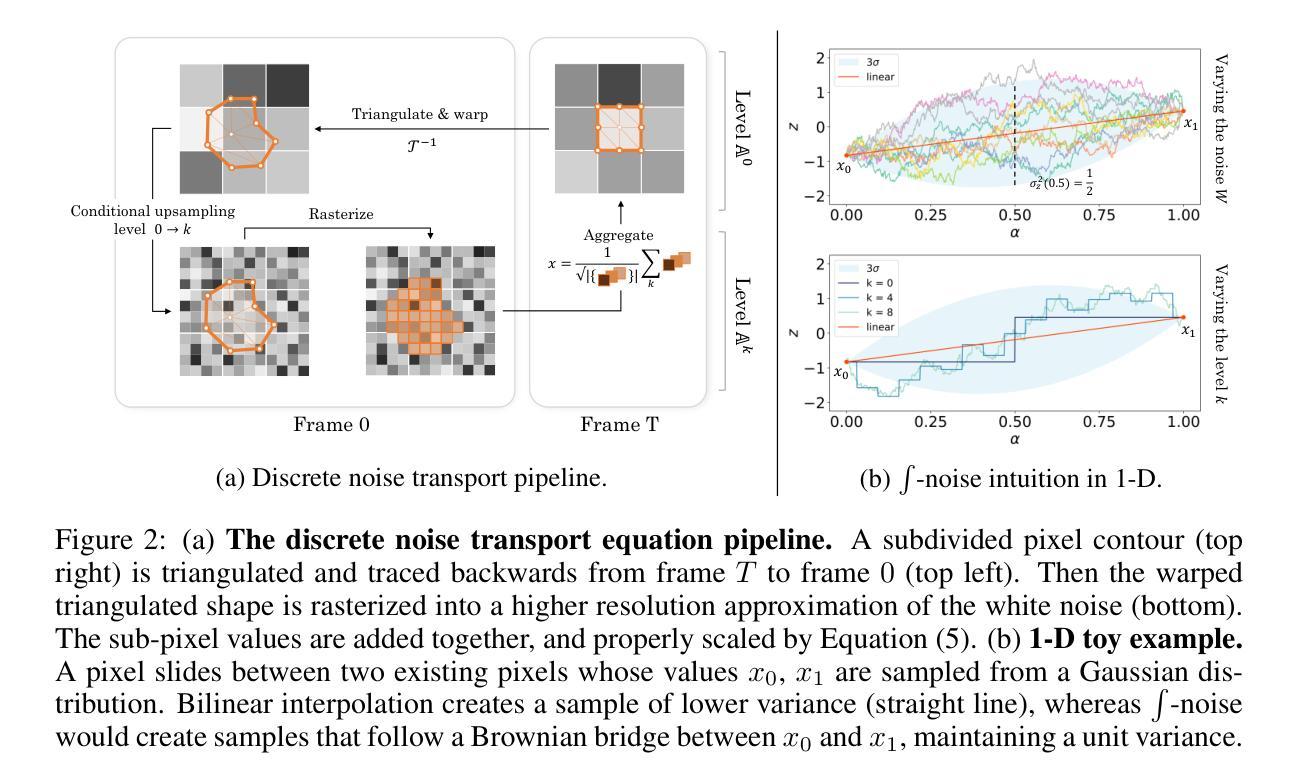
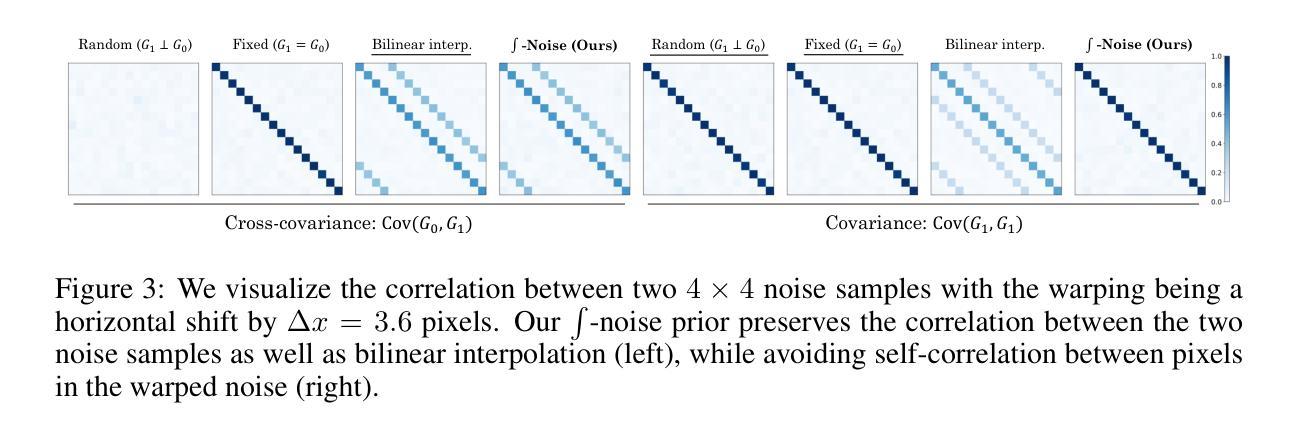
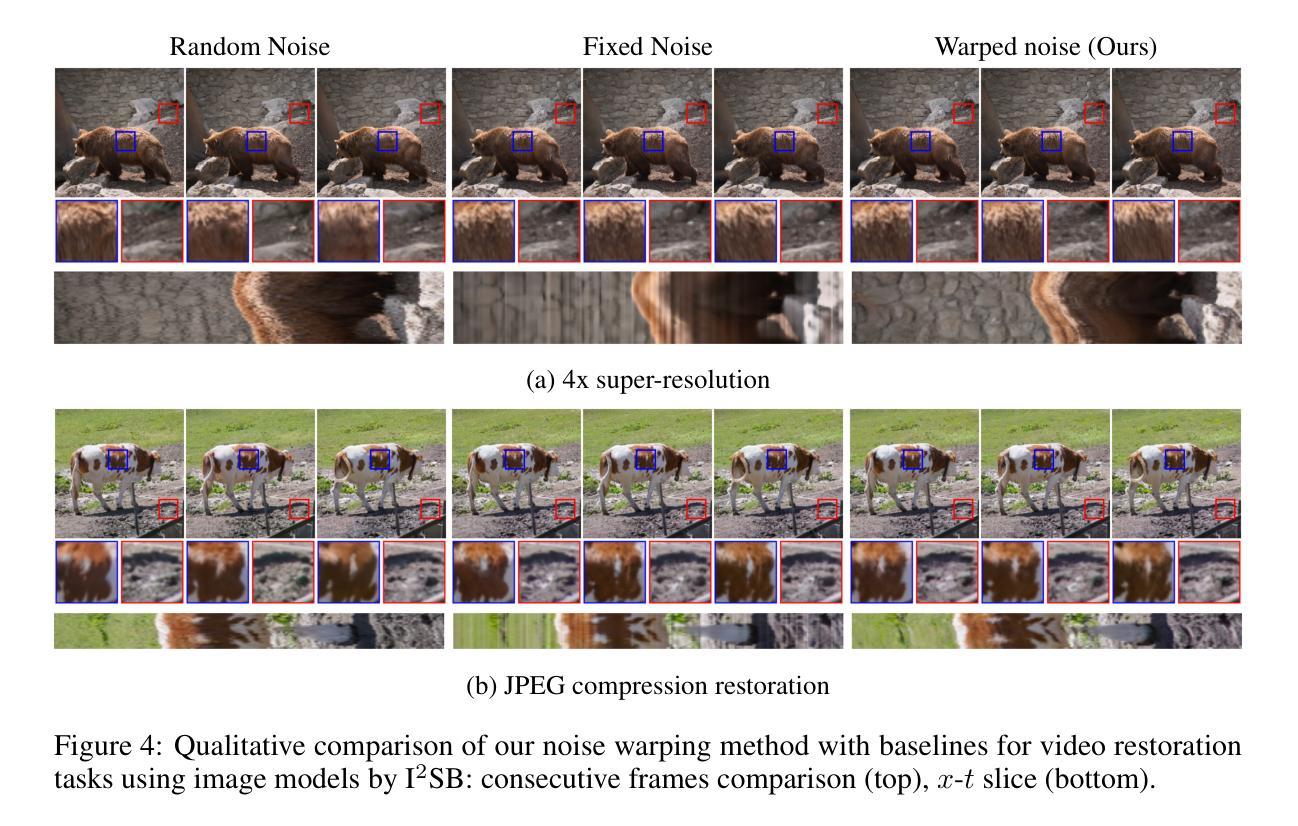
Comprehensive Relighting: Generalizable and Consistent Monocular Human Relighting and Harmonization
Authors:Junying Wang, Jingyuan Liu, Xin Sun, Krishna Kumar Singh, Zhixin Shu, He Zhang, Jimei Yang, Nanxuan Zhao, Tuanfeng Y. Wang, Simon S. Chen, Ulrich Neumann, Jae Shin Yoon
This paper introduces Comprehensive Relighting, the first all-in-one approach that can both control and harmonize the lighting from an image or video of humans with arbitrary body parts from any scene. Building such a generalizable model is extremely challenging due to the lack of dataset, restricting existing image-based relighting models to a specific scenario (e.g., face or static human). To address this challenge, we repurpose a pre-trained diffusion model as a general image prior and jointly model the human relighting and background harmonization in the coarse-to-fine framework. To further enhance the temporal coherence of the relighting, we introduce an unsupervised temporal lighting model that learns the lighting cycle consistency from many real-world videos without any ground truth. In inference time, our temporal lighting module is combined with the diffusion models through the spatio-temporal feature blending algorithms without extra training; and we apply a new guided refinement as a post-processing to preserve the high-frequency details from the input image. In the experiments, Comprehensive Relighting shows a strong generalizability and lighting temporal coherence, outperforming existing image-based human relighting and harmonization methods.
本文介绍了全面重照明技术,这是一种全新的全栈方法,既能控制也能协调来自图像或视频的人物的任意身体部分在任何场景中的照明。构建这种通用模型极具挑战性,因为缺乏数据集,现有的基于图像的重新照明模型仅限于特定场景(例如脸部或静态人物)。为了应对这一挑战,我们将预训练的扩散模型重新定位为通用图像先验,并在由粗到细的框架中联合模拟人物重新照明和背景协调。为了进一步提高重新照明的时序一致性,我们引入了一种无监督的时序照明模型,该模型可以从许多真实世界的视频中学习照明周期的一致性,无需任何真实数据。在推理阶段,我们的时序照明模块通过时空特征融合算法与扩散模型相结合,无需额外训练;我们还应用了一种新的引导精炼作为后处理,以保留输入图像的高频细节。在实验中,全面重照明技术表现出强大的通用性和照明时序一致性,优于现有的基于图像的重新照明和协调方法。
论文及项目相关链接
PDF Project page:https://junyingw.github.io/paper/relighting. Accepted by CVPR 2025
Summary
本文提出了全面的重照明技术,该技术能在图像或视频中同时控制和协调人体各部位的照明。通过建立通用模型来应对数据集缺失的挑战,使得现有基于图像的重照明模型局限于特定场景(如面部或静态人体)。本文利用预训练的扩散模型作为通用图像先验,并在粗到细的框架中联合建模人体重照明和背景协调。为了进一步提高重照明的时空一致性,引入了无监督的时空光照模型,该模型从众多真实视频中学习光照周期一致性,无需任何真实数据。在推理过程中,将时空光照模块与扩散模型通过时空特征融合算法相结合,并应用新的引导细化作为后处理以保留输入图像的高频细节。实验表明,全面重照明技术具有良好的通用性和光照时间一致性,优于现有的基于图像的人体重照明和协调方法。
Key Takeaways
- 提出了全新的全面重照明技术,该技术可控制并协调图像或视频中人体任意部位的照明。
- 利用预训练的扩散模型作为通用图像先验,实现了模型的广泛应用。
- 在粗到细的框架中联合建模人体重照明和背景协调。
- 引入无监督的时空光照模型,提高了重照明的时空一致性。
- 在推理过程中结合了时空光照模块与扩散模型,通过时空特征融合算法实现。
- 采用新的引导细化后处理来保留输入图像的高频细节。
点此查看论文截图
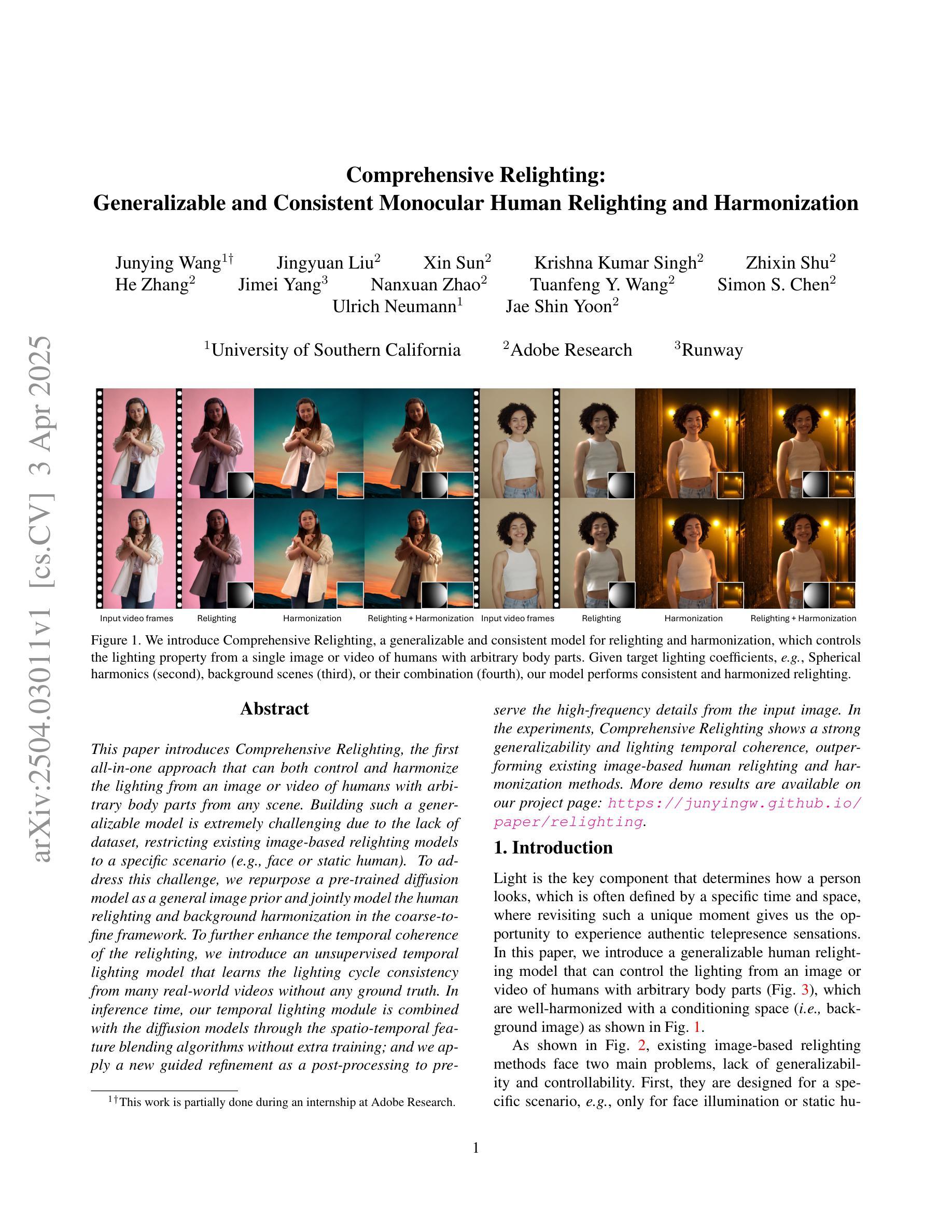
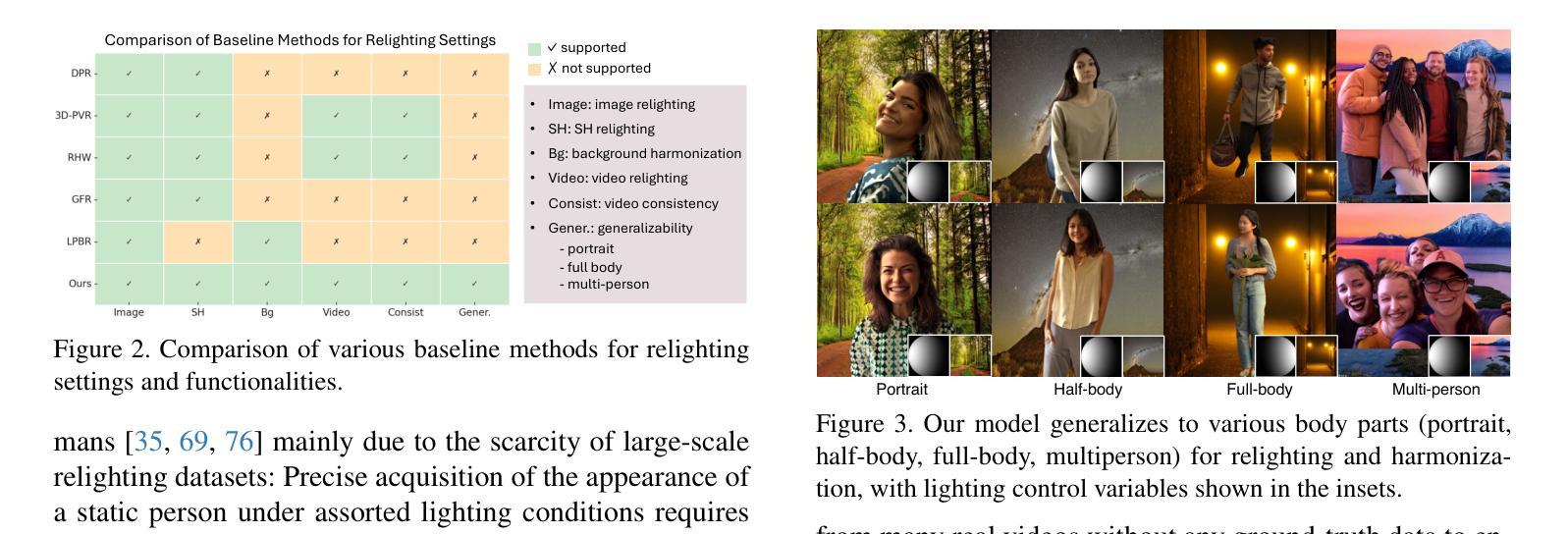
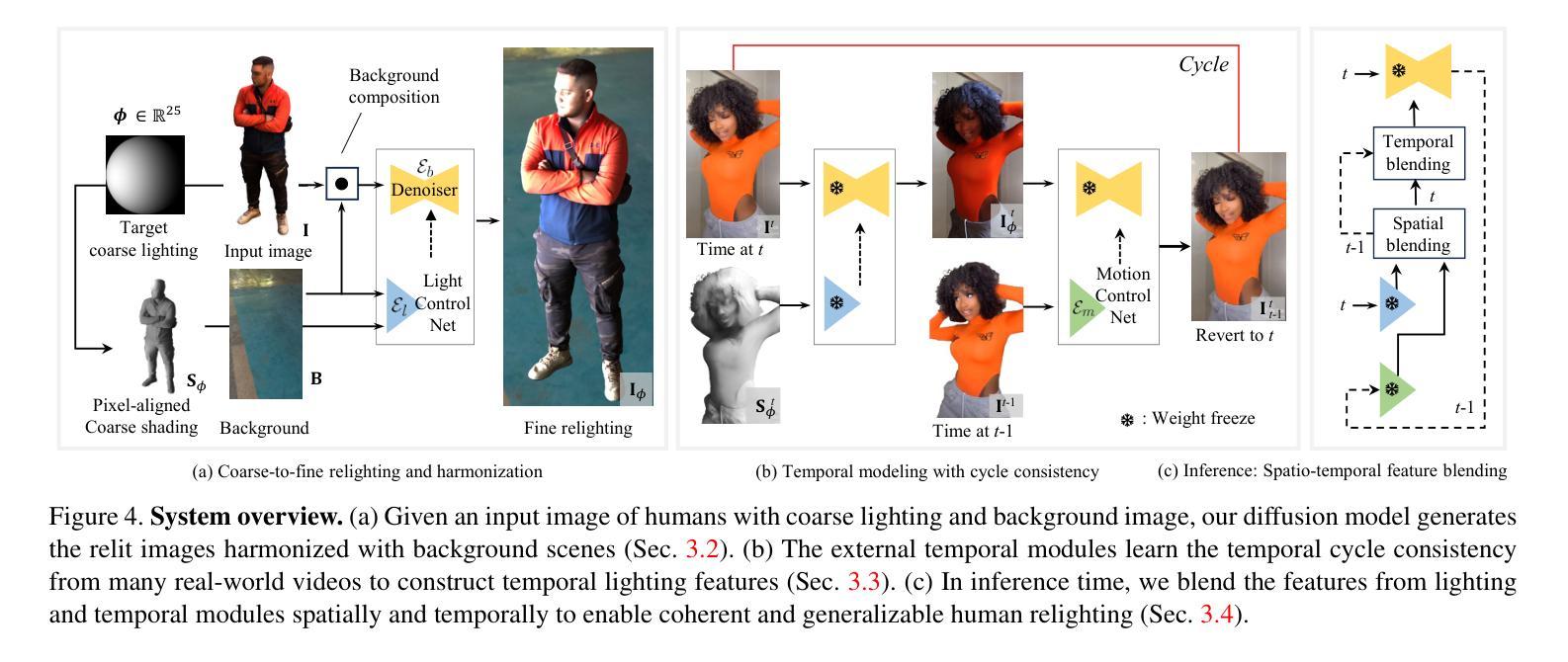
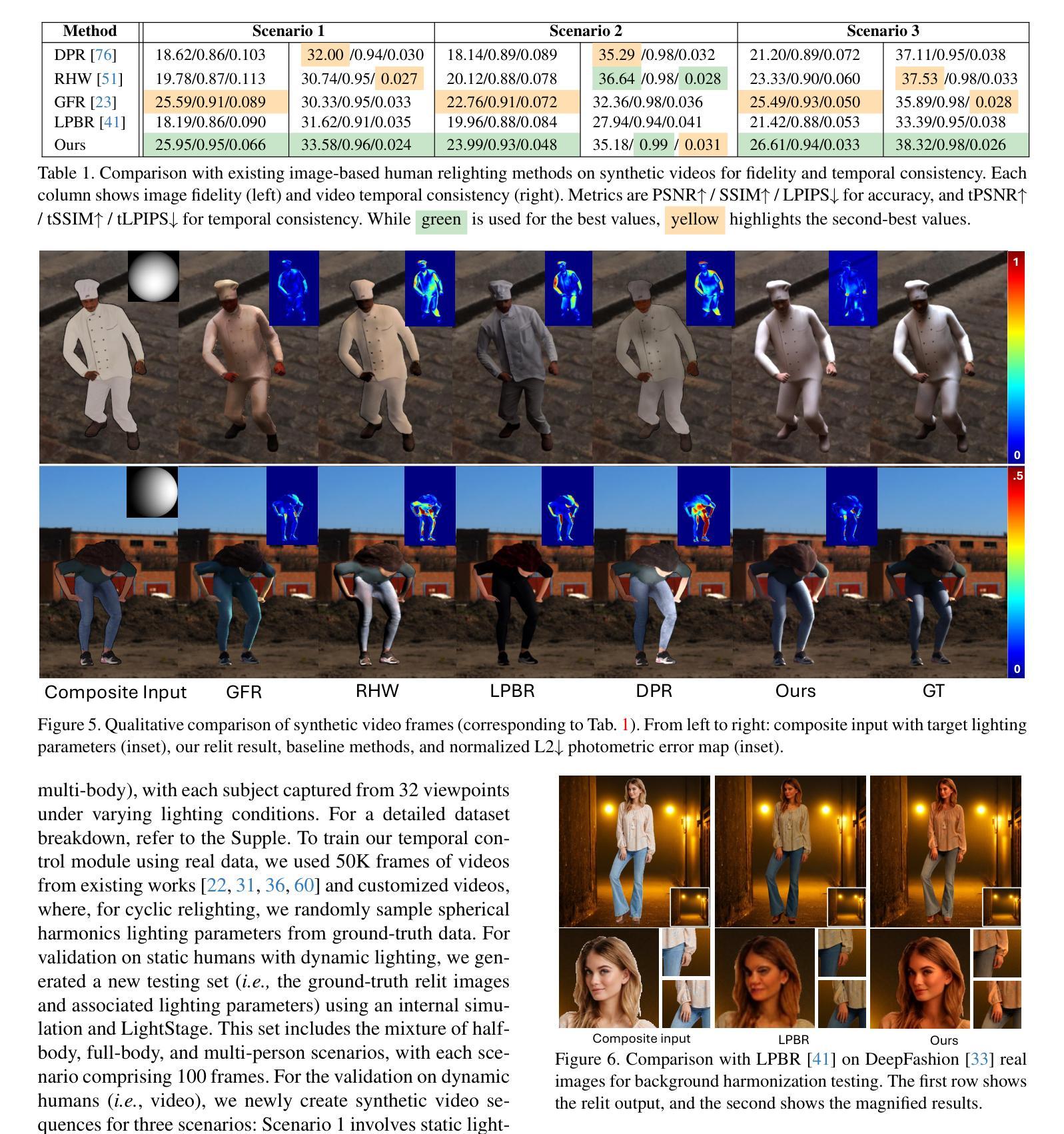
DiSRT-In-Bed: Diffusion-Based Sim-to-Real Transfer Framework for In-Bed Human Mesh Recovery
Authors:Jing Gao, Ce Zheng, Laszlo A. Jeni, Zackory Erickson
In-bed human mesh recovery can be crucial and enabling for several healthcare applications, including sleep pattern monitoring, rehabilitation support, and pressure ulcer prevention. However, it is difficult to collect large real-world visual datasets in this domain, in part due to privacy and expense constraints, which in turn presents significant challenges for training and deploying deep learning models. Existing in-bed human mesh estimation methods often rely heavily on real-world data, limiting their ability to generalize across different in-bed scenarios, such as varying coverings and environmental settings. To address this, we propose a Sim-to-Real Transfer Framework for in-bed human mesh recovery from overhead depth images, which leverages large-scale synthetic data alongside limited or no real-world samples. We introduce a diffusion model that bridges the gap between synthetic data and real data to support generalization in real-world in-bed pose and body inference scenarios. Extensive experiments and ablation studies validate the effectiveness of our framework, demonstrating significant improvements in robustness and adaptability across diverse healthcare scenarios.
卧床人体网格恢复对于多种医疗健康应用至关重要,包括睡眠模式监测、康复支持和压力溃疡预防。然而,由于隐私和经费等方面的限制,在这个领域收集大规模现实世界视觉数据集是非常困难的,这给深度学习的训练和部署带来了巨大挑战。现有的卧床人体网格估计方法往往严重依赖于现实世界数据,限制了它们在不同卧床场景中的泛化能力,如不同的覆盖物和环境设置。为了解决这一问题,我们提出了一个基于Sim-to-Real迁移框架的卧床人体网格恢复方法,该方法利用大规模合成数据以及有限或无现实世界样本。我们引入了一个扩散模型来弥补合成数据和真实数据之间的差距,以支持在现实世界卧床姿势和人体推断场景中的泛化。大量的实验和消融研究验证了我们的框架的有效性,表明它在各种医疗健康场景中显著提高了稳健性和适应性。
论文及项目相关链接
PDF 16 pages, 19 figures. Accepted to CVPR 2025
Summary
在医疗保健应用中,床位人体网格恢复非常重要且至关重要,包括睡眠模式监测、康复支持和压力溃疡预防等。然而,由于其隐私和成本等限制因素,在此领域收集大型真实世界视觉数据集变得十分困难,给深度学习模型的训练和部署带来了巨大挑战。为了解决这个问题,我们提出了一个基于合成到真实场景的转移框架,用于从头顶深度图像中进行床位人体网格恢复。该框架利用大规模合成数据以及有限的真实世界样本或无真实世界样本。我们引入了一种扩散模型,以缩小合成数据和真实数据之间的差距,支持真实世界床位姿势和人体推断场景的泛化。经过广泛的实验和消融研究,验证了该框架的有效性,在多种医疗保健场景中表现出了显著的稳健性和适应性改进。
Key Takeaways
- 床位人体网格恢复在医疗保健应用中至关重要,如睡眠监测、康复支持和压力溃疡预防等。
- 收集真实世界视觉数据集存在困难,隐私和成本是主要限制因素。
- 提出了一种基于合成到真实场景的转移框架,用于从头顶深度图像进行床位人体网格恢复。
- 框架利用大规模合成数据和有限的真实世界样本或无真实世界样本。
- 引入扩散模型以缩小合成数据和真实数据之间的差距。
- 框架经过广泛实验和消融研究验证,表现显著改进。
点此查看论文截图
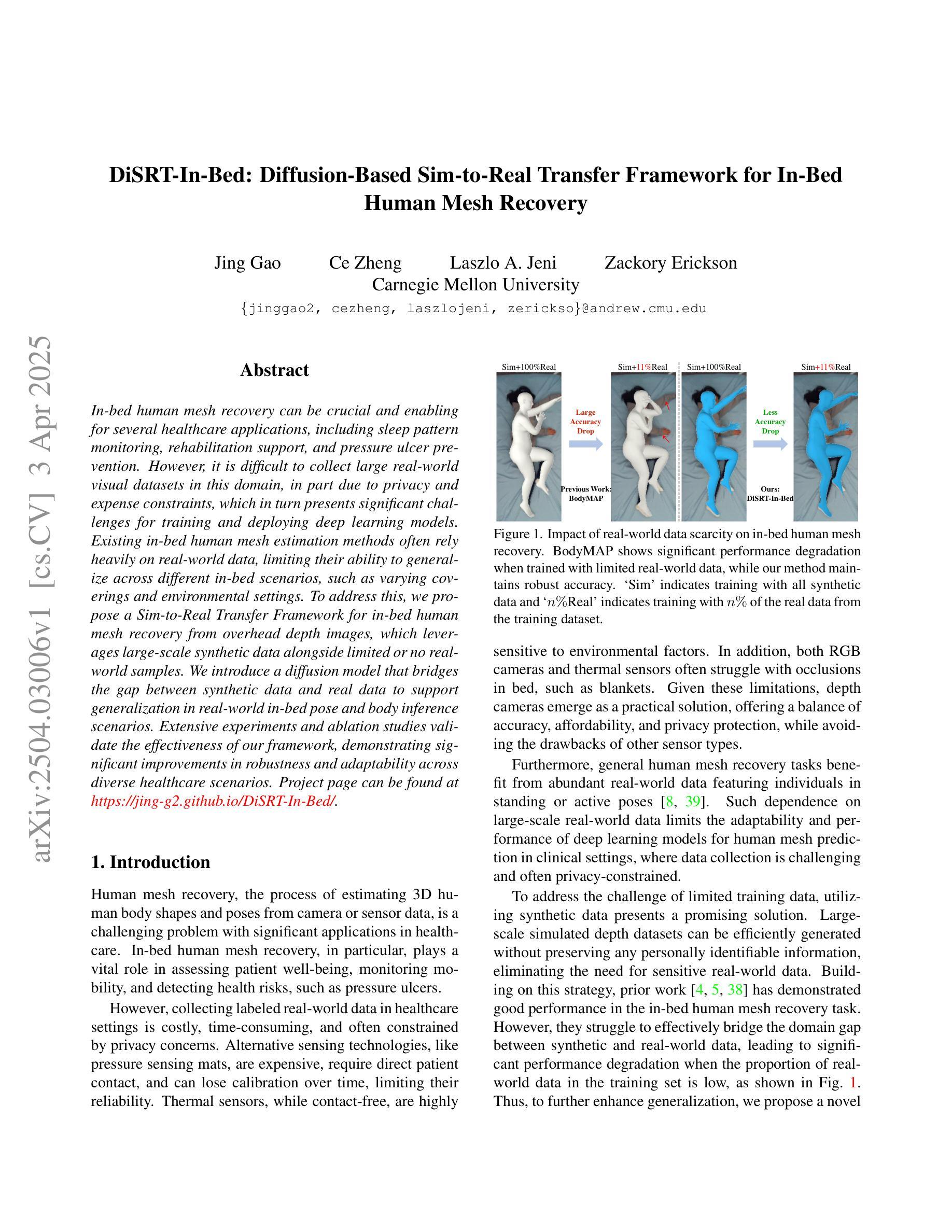
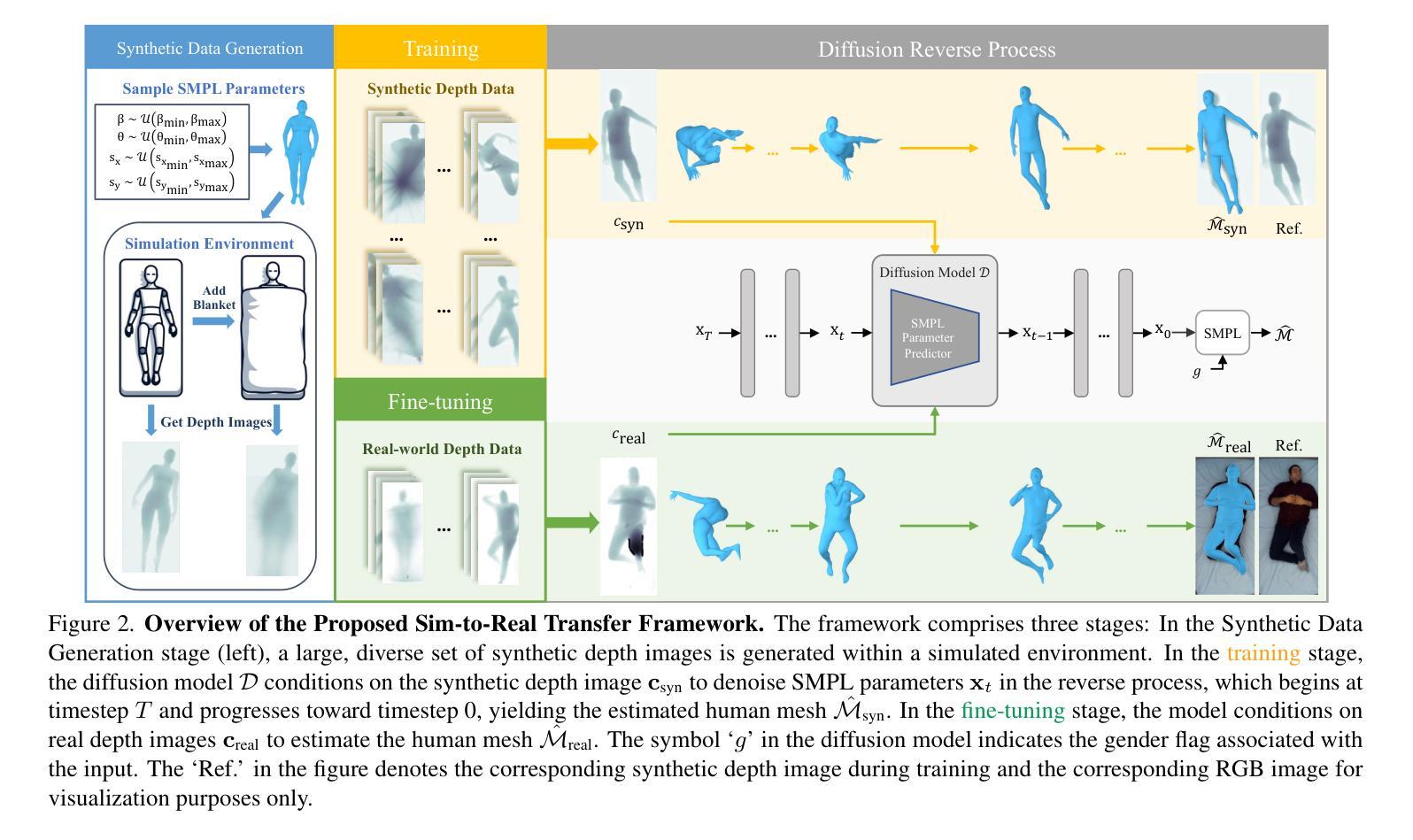
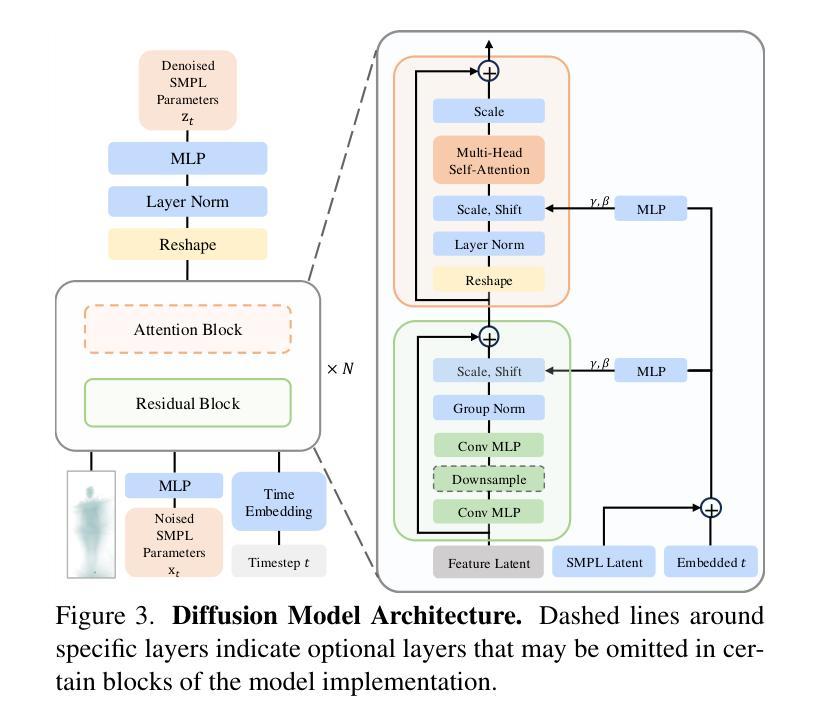
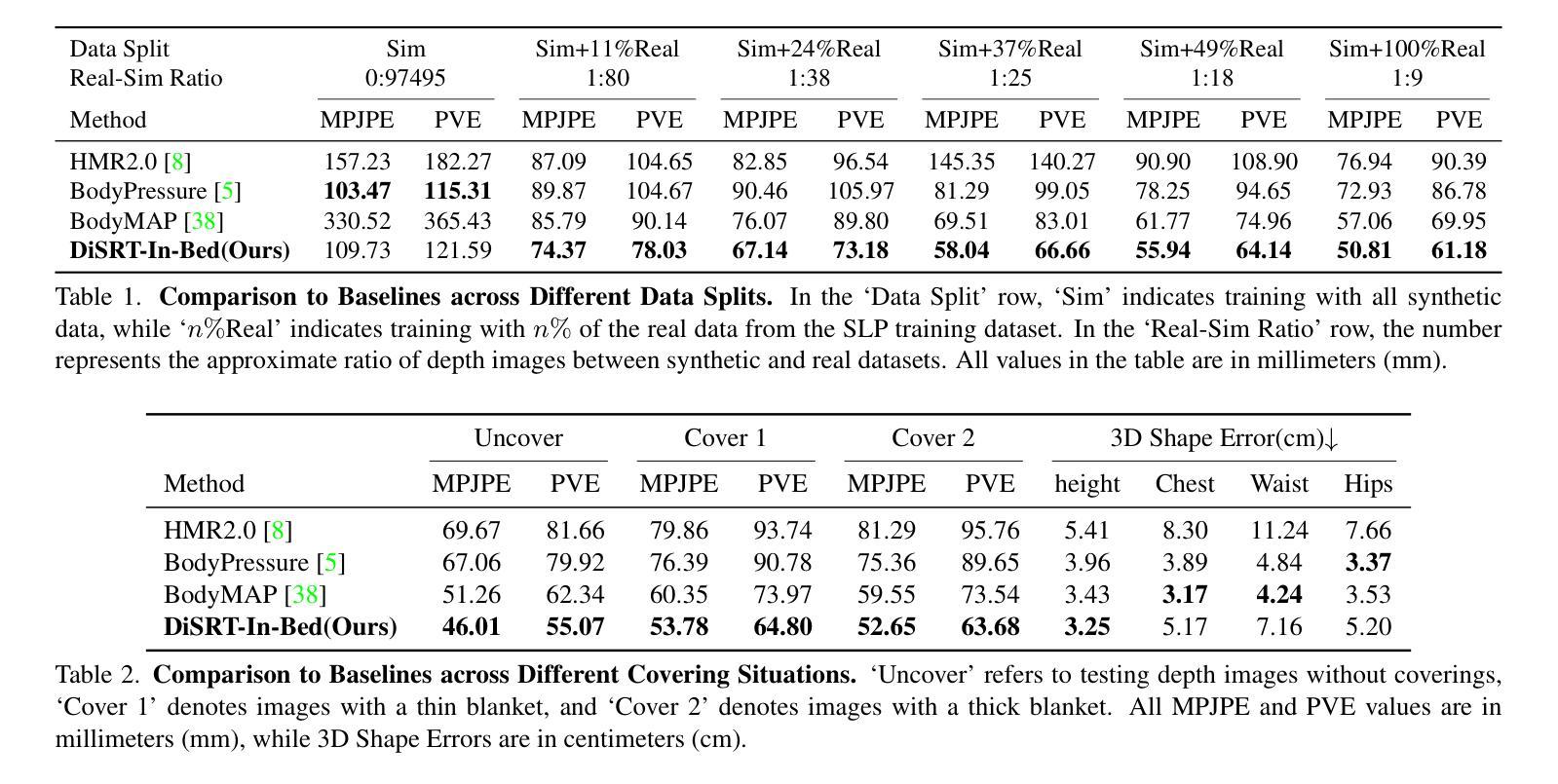
VLIPP: Towards Physically Plausible Video Generation with Vision and Language Informed Physical Prior
Authors:Xindi Yang, Baolu Li, Yiming Zhang, Zhenfei Yin, Lei Bai, Liqian Ma, Zhiyong Wang, Jianfei Cai, Tien-Tsin Wong, Huchuan Lu, Xu Jia
Video diffusion models (VDMs) have advanced significantly in recent years, enabling the generation of highly realistic videos and drawing the attention of the community in their potential as world simulators. However, despite their capabilities, VDMs often fail to produce physically plausible videos due to an inherent lack of understanding of physics, resulting in incorrect dynamics and event sequences. To address this limitation, we propose a novel two-stage image-to-video generation framework that explicitly incorporates physics with vision and language informed physical prior. In the first stage, we employ a Vision Language Model (VLM) as a coarse-grained motion planner, integrating chain-of-thought and physics-aware reasoning to predict a rough motion trajectories/changes that approximate real-world physical dynamics while ensuring the inter-frame consistency. In the second stage, we use the predicted motion trajectories/changes to guide the video generation of a VDM. As the predicted motion trajectories/changes are rough, noise is added during inference to provide freedom to the VDM in generating motion with more fine details. Extensive experimental results demonstrate that our framework can produce physically plausible motion, and comparative evaluations highlight the notable superiority of our approach over existing methods. More video results are available on our Project Page: https://madaoer.github.io/projects/physically_plausible_video_generation.
视频扩散模型(VDMs)近年来取得了显著进展,能够生成高度逼真的视频,并作为世界模拟器引起了社区的广泛关注。然而,尽管VDMs具有强大的能力,但由于缺乏对物理的内在理解,它们往往无法生成物理上合理的视频,从而导致动态和事件序列不正确。为了解决这个问题,我们提出了一种新颖的两阶段图像到视频生成框架,该框架显式地将物理与视觉和语言相结合,形成物理先验。在第一阶段,我们采用视觉语言模型(VLM)作为粗略的运动规划器,通过融入思考和物理感知推理来预测大致的运动轨迹或变化,以近似现实世界的物理动态并确保帧间的一致性。在第二阶段,我们使用预测的运动轨迹或变化来指导VDM的视频生成。由于预测的运动轨迹或变化是粗略的,因此在推理过程中加入了噪声,为VDM生成具有更多细节的运动提供了自由。大量的实验结果表明,我们的框架可以生成物理上合理的运动,并且与其他方法的比较评估凸显了我们方法的显著优势。更多视频结果请访问我们的项目页面:https://madaoer.github.io/projects/physically_plausible_video_generation 。
论文及项目相关链接
PDF 18 pages, 11 figures
摘要
视频扩散模型(VDMs)近年发展迅速,能生成高度逼真的视频,作为世界模拟器引起了人们的关注。然而,由于缺乏对物理的内在理解,VDMs往往无法生成物理上可行的视频,导致动态和事件序列不正确。为解决这一限制,我们提出一种新颖的两阶段图像到视频生成框架,显式地将物理与视觉和语言相结合,形成物理先验。第一阶段,我们采用视觉语言模型(VLM)作为粗粒度运动规划器,整合思维链和物理感知推理,预测大致的运动轨迹/变化,近似真实世界的物理动态,同时确保帧间一致性。在第二阶段,我们使用预测的运动轨迹/变化来指导VDM的视频生成。由于预测的运动轨迹/变化是粗略的,因此在推理过程中加入了噪声,为VDM生成具有更多细节的运动提供了自由。大量的实验结果表明,我们的框架能生成物理上可行的运动,对比评估凸显了我们的方法相较于现有方法的显著优势。更多视频结果请访问我们的项目页面:链接。
关键见解
- VDMs虽能生成高度逼真的视频,但缺乏物理理解,导致生成的视频在物理上不可行。
- 提出一种新颖的两阶段图像到视频生成框架,显式结合物理、视觉和语言。
- 在第一阶段,使用VLM作为粗粒度运动规划器,预测大致的运动轨迹/变化,模拟真实世界的物理动态。
- 第二阶段利用预测的运动轨迹/变化指导VDM的视频生成。
- 通过添加噪声到预测的运动轨迹/变化,为VDM生成更精细的运动细节提供自由。
- 实验结果表明,该框架能生成物理上可行的运动。
点此查看论文截图
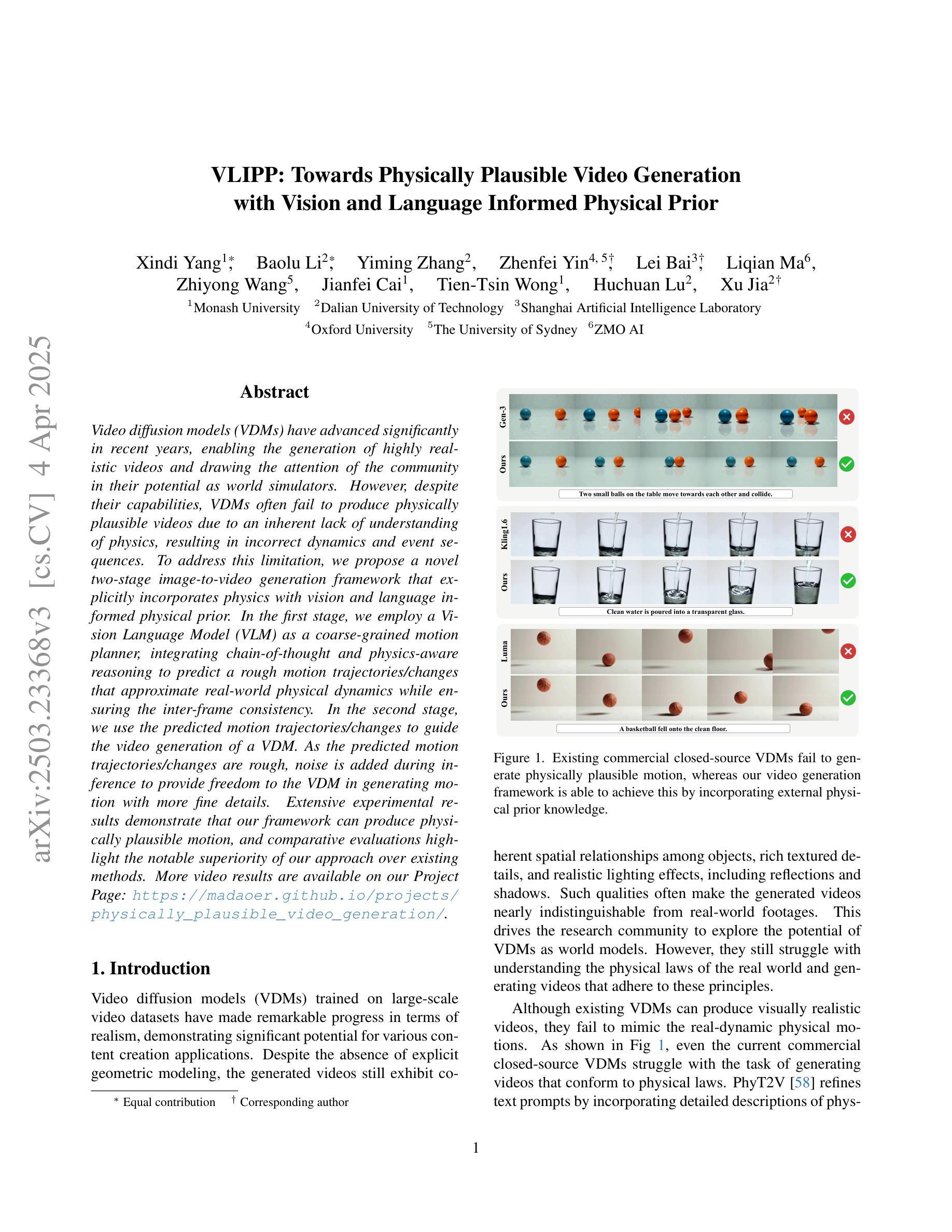
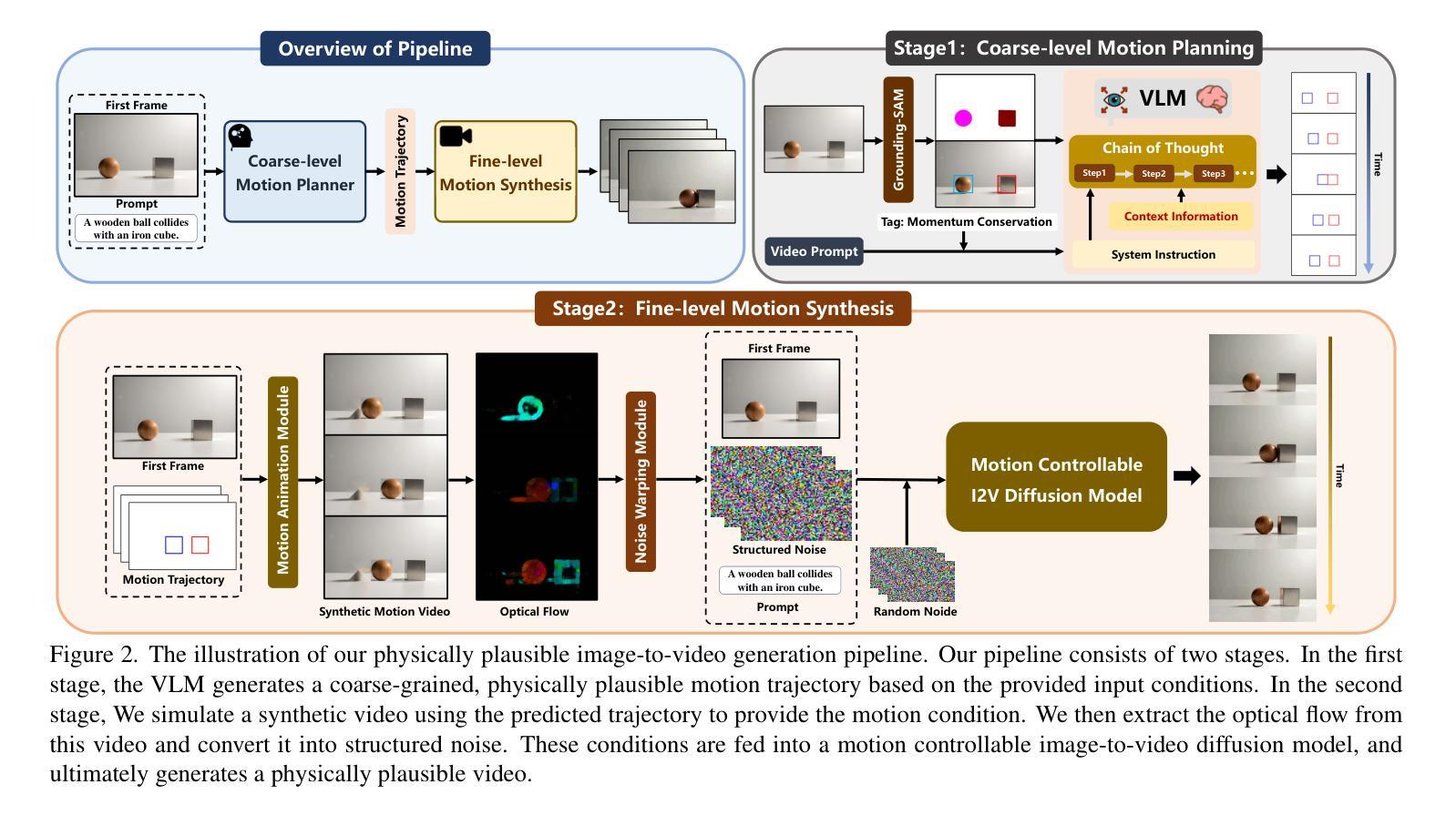
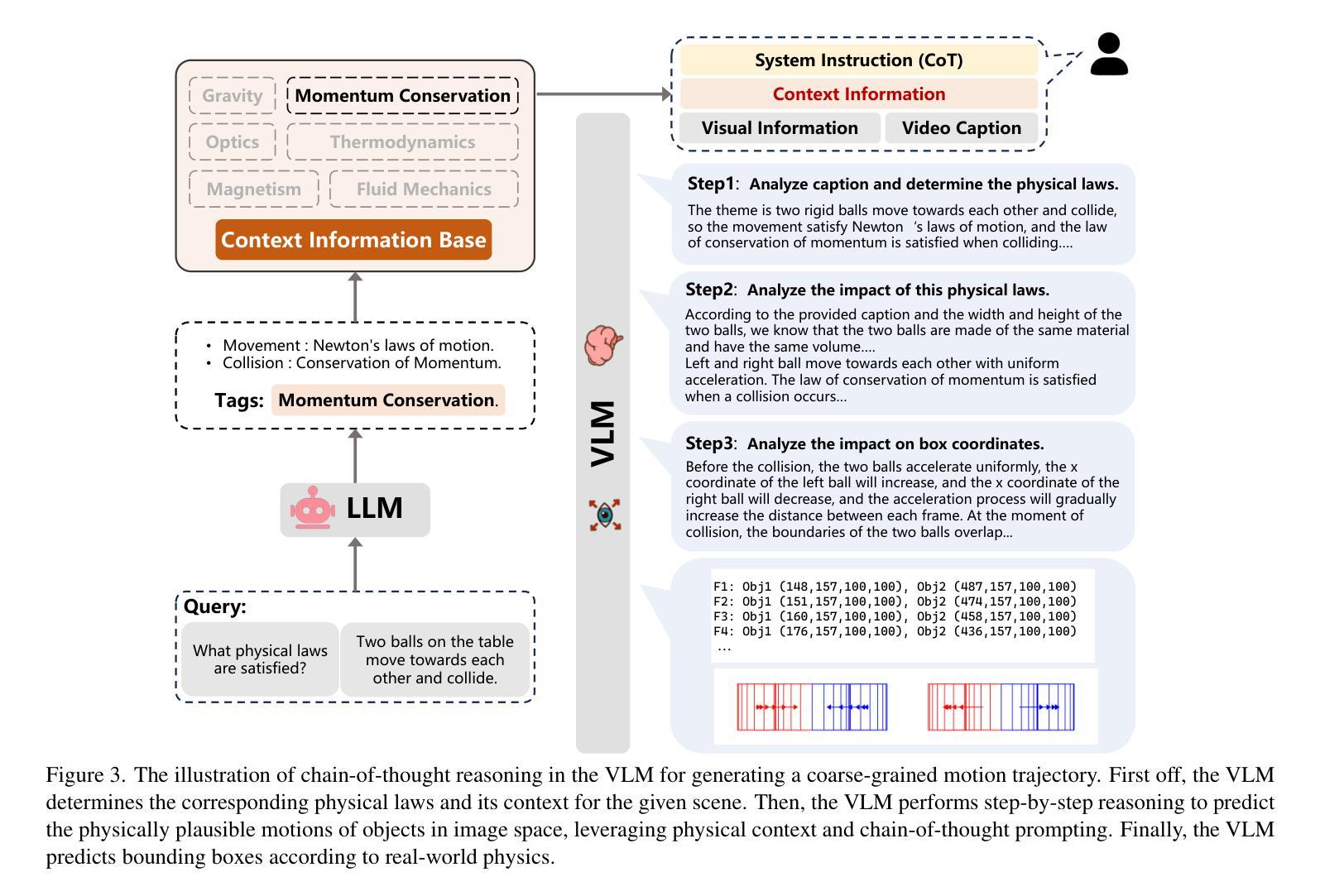
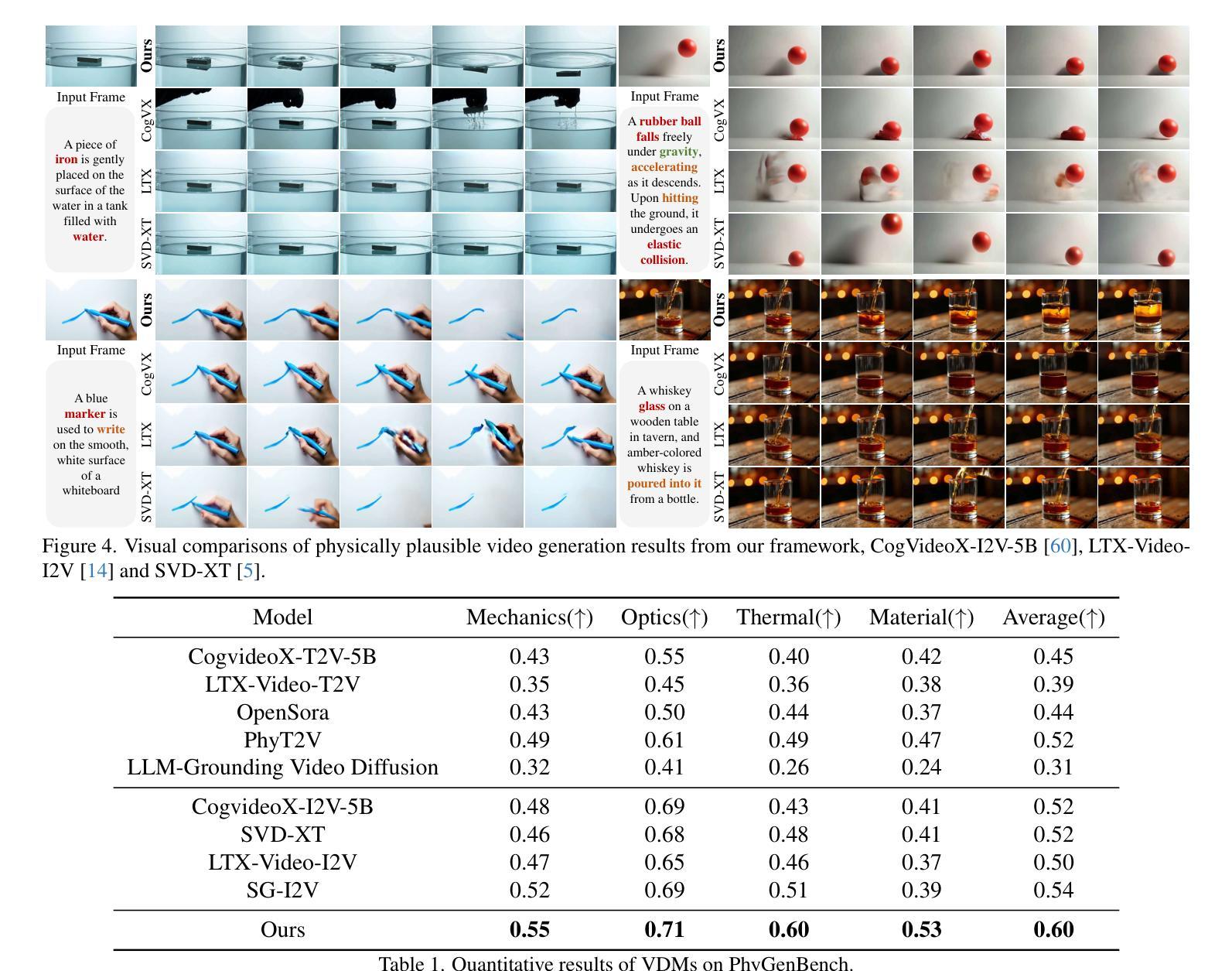
Segment Any-Quality Images with Generative Latent Space Enhancement
Authors:Guangqian Guo, Yong Guo, Xuehui Yu, Wenbo Li, Yaoxing Wang, Shan Gao
Despite their success, Segment Anything Models (SAMs) experience significant performance drops on severely degraded, low-quality images, limiting their effectiveness in real-world scenarios. To address this, we propose GleSAM, which utilizes Generative Latent space Enhancement to boost robustness on low-quality images, thus enabling generalization across various image qualities. Specifically, we adapt the concept of latent diffusion to SAM-based segmentation frameworks and perform the generative diffusion process in the latent space of SAM to reconstruct high-quality representation, thereby improving segmentation. Additionally, we introduce two techniques to improve compatibility between the pre-trained diffusion model and the segmentation framework. Our method can be applied to pre-trained SAM and SAM2 with only minimal additional learnable parameters, allowing for efficient optimization. We also construct the LQSeg dataset with a greater diversity of degradation types and levels for training and evaluating the model. Extensive experiments demonstrate that GleSAM significantly improves segmentation robustness on complex degradations while maintaining generalization to clear images. Furthermore, GleSAM also performs well on unseen degradations, underscoring the versatility of our approach and dataset.
尽管取得了成功,但Segment Anything Models(SAM)在严重退化、低质量的图像上性能显著下降,限制了其在现实场景中的有效性。为了解决这一问题,我们提出了GleSAM。它利用生成潜在空间增强技术来提高对低质量图像的稳健性,从而实现跨各种图像质量的泛化。具体来说,我们适应基于SAM的分割框架中的潜在扩散概念,并在SAM的潜在空间中执行生成扩散过程,以重建高质量表示,从而提高分割效果。此外,我们引入了两种技术来提高预训练扩散模型和分割框架之间的兼容性。我们的方法可以应用于预训练的SAM和SAM2,并且只需要极少量的额外可学习参数,从而实现高效优化。我们还构建了LQSeg数据集,包含更多类型和程度的退化类型,用于训练和评估模型。大量实验表明,GleSAM在复杂退化情况下显著提高分割稳健性,同时保持对清晰图像的泛化能力。此外,GleSAM在未见过的退化情况下也表现良好,突显了我们方法和数据集的通用性。
论文及项目相关链接
PDF Accepted by CVPR2025
Summary
SAM模型在处理低质量图像时性能显著下降,限制了其在现实场景中的应用。为解决这一问题,我们提出了GleSAM模型,利用生成潜在空间增强技术提高低质量图像上的稳健性,从而实现跨不同图像质量的泛化。我们在SAM分割框架中引入潜在扩散的概念,在SAM的潜在空间中进行生成扩散过程,重建高质量表示,从而提高分割性能。此外,我们还引入了两种技术来提高预训练扩散模型和分割框架之间的兼容性。我们的方法可以应用于预训练的SAM和SAM2模型,只需极少量的额外可学习参数,就能实现高效优化。我们还构建了LQSeg数据集,包含更多类型和程度的退化,用于训练和评估模型。实验表明,GleSAM在复杂退化情况下显著提高分割稳健性,同时保持对清晰图像的泛化能力,并在未见退化情况下表现良好。
Key Takeaways
- SAM模型在处理低质量图像时性能下降,限制了其在现实场景的应用。
- GleSAM模型通过利用生成潜在空间增强技术提高SAM模型在低质量图像上的稳健性。
- GleSAM引入潜在扩散概念到SAM分割框架中,提高分割性能。
- GleSAM只需要极少量的额外可学习参数,能够高效优化。
- LQSeg数据集用于训练和评估模型,包含更多类型和程度的退化。
- GleSAM在复杂退化情况下显著提高分割稳健性。
点此查看论文截图
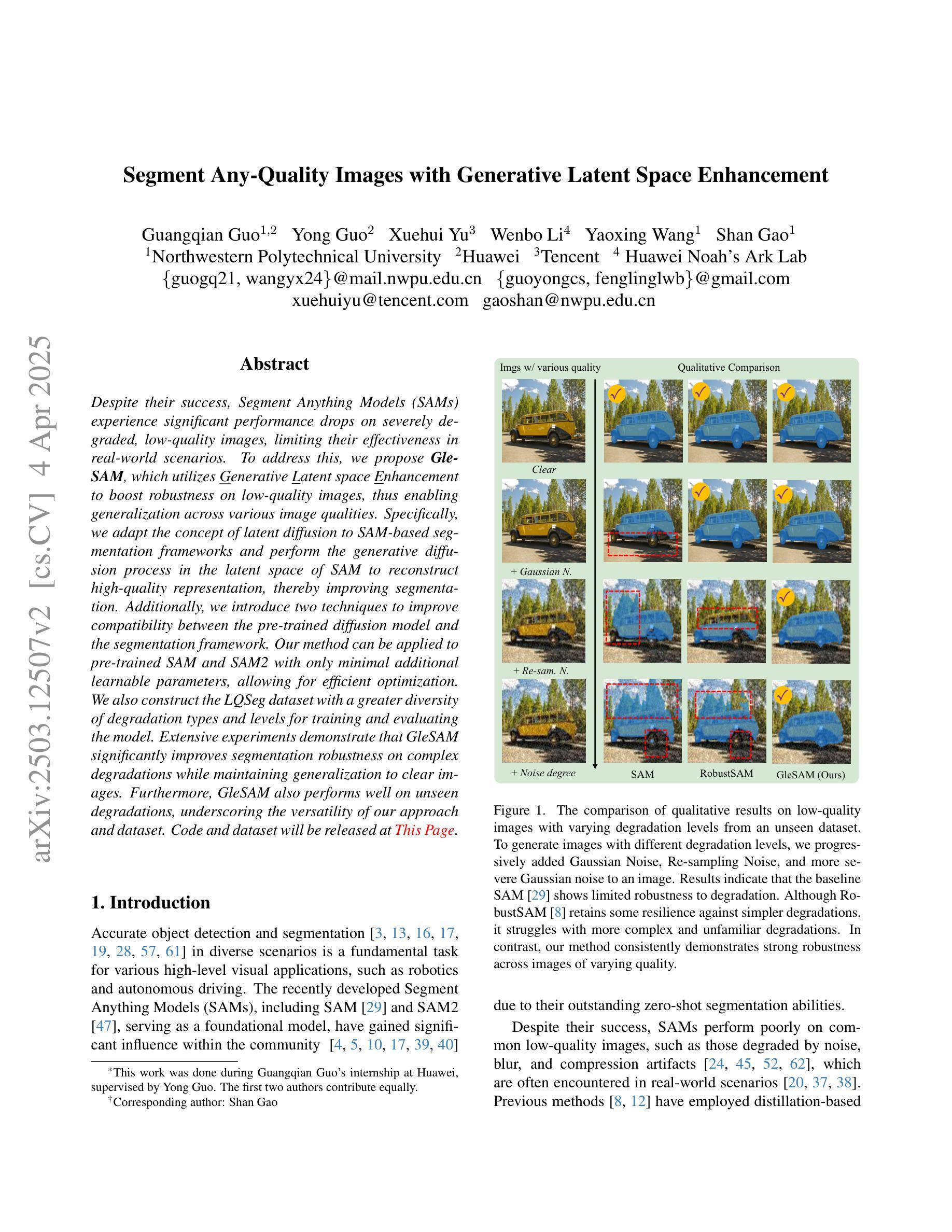
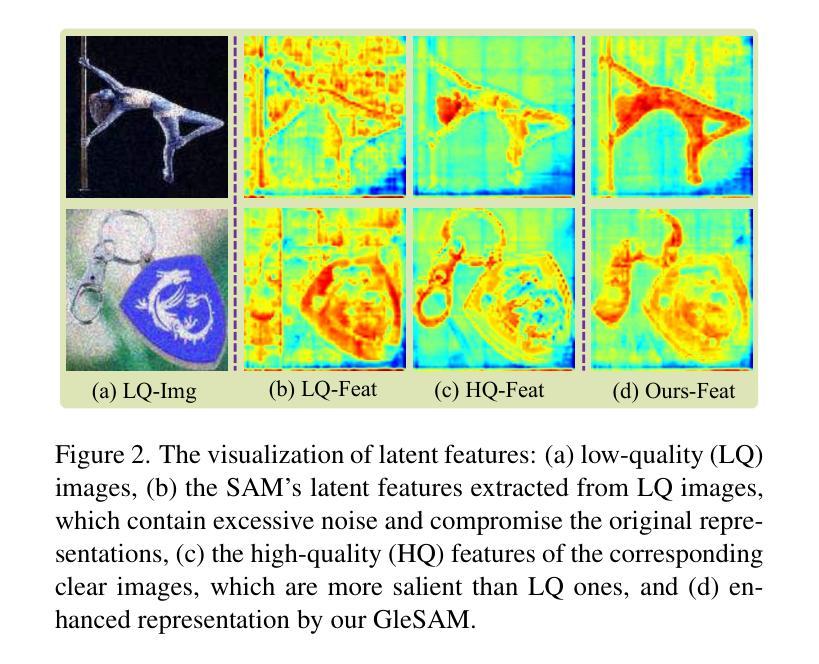
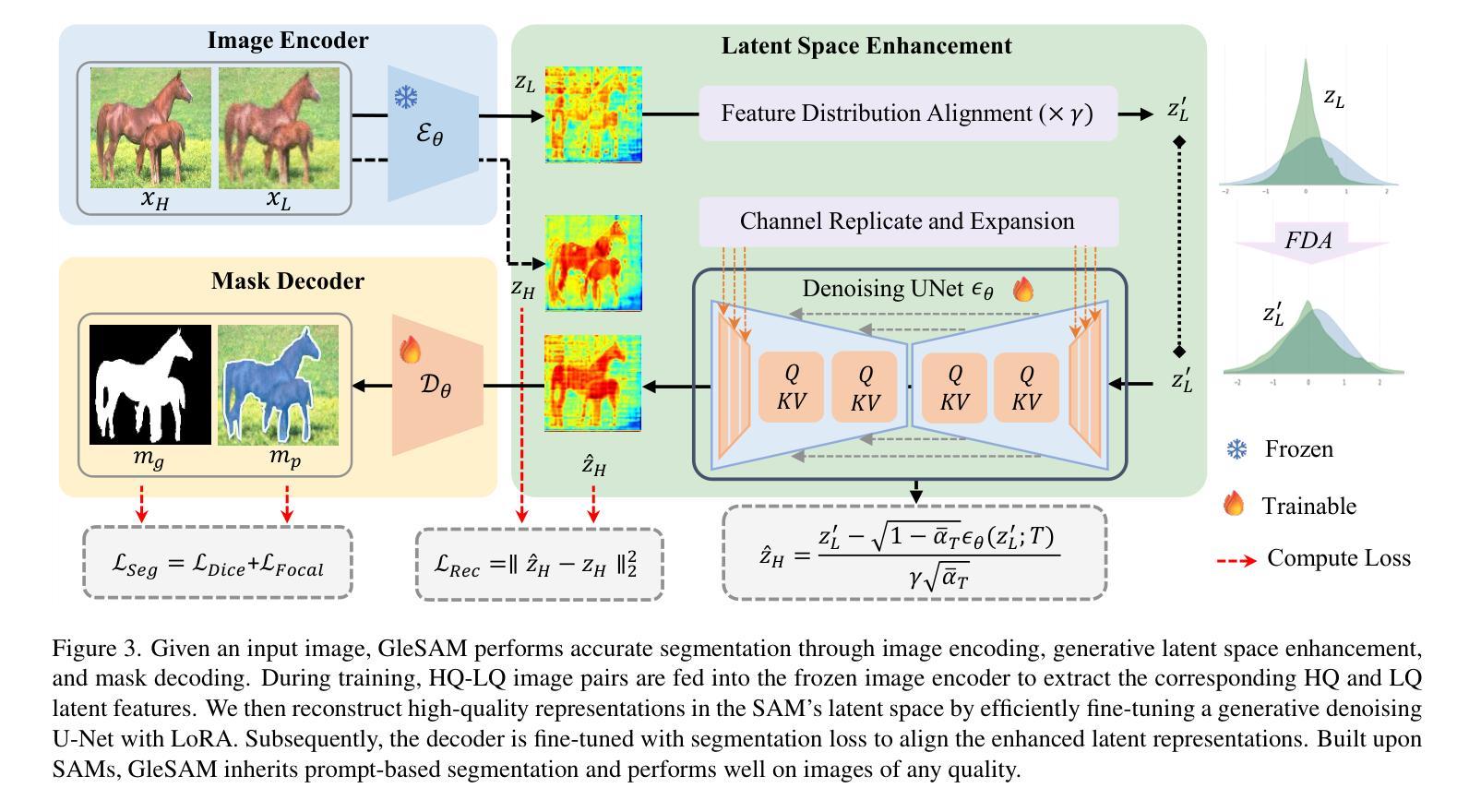
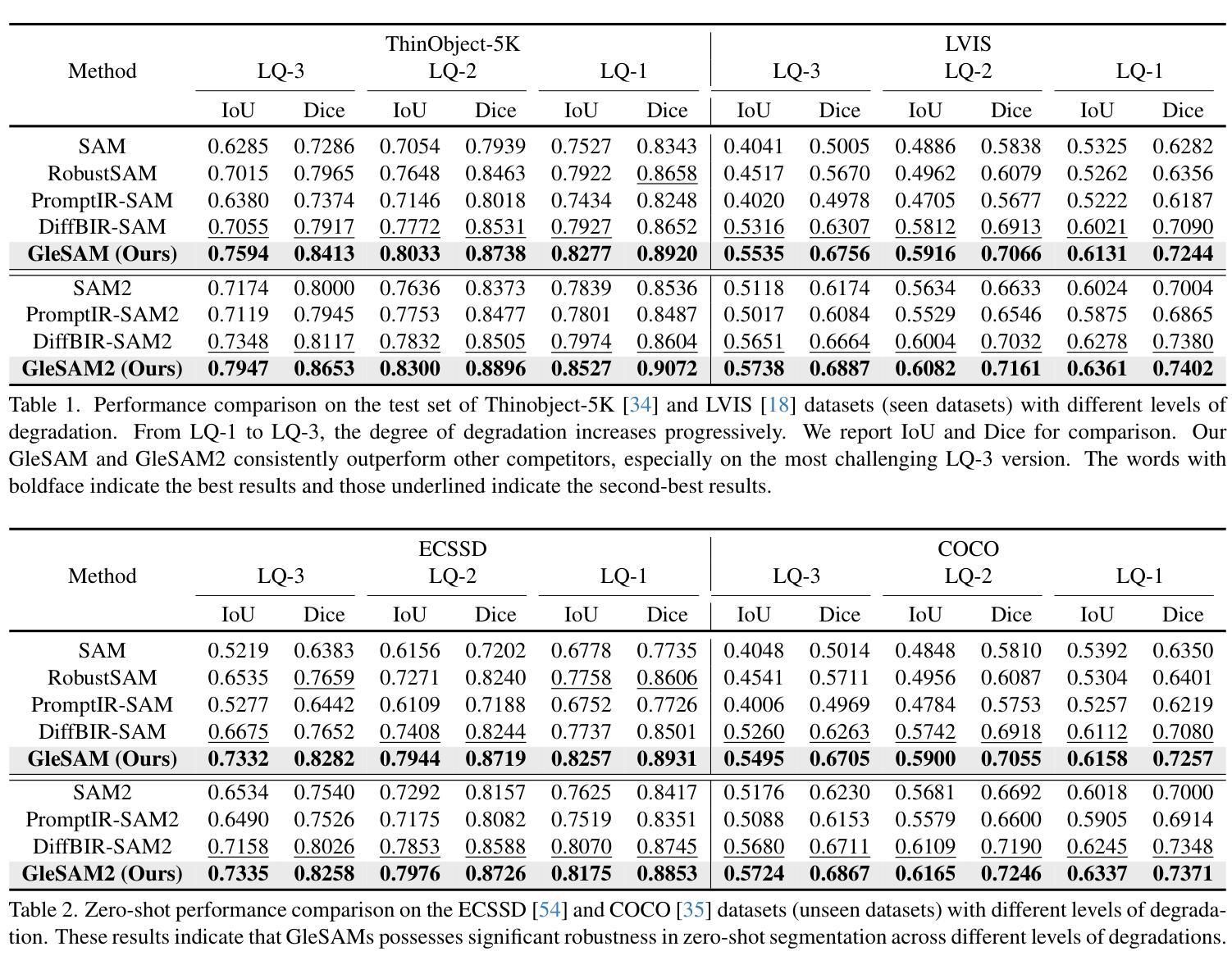
Training-Free Style and Content Transfer by Leveraging U-Net Skip Connections in Stable Diffusion
Authors:Ludovica Schaerf, Andrea Alfarano, Fabrizio Silvestri, Leonardo Impett
Recent advances in diffusion models for image generation have led to detailed examinations of several components within the U-Net architecture for image editing. While previous studies have focused on the bottleneck layer (h-space), cross-attention, self-attention, and decoding layers, the overall role of the skip connections of the U-Net itself has not been specifically addressed. We conduct thorough analyses on the role of the skip connections and find that the residual connections passed by the third encoder block carry most of the spatial information of the reconstructed image, splitting the content from the style, passed by the remaining stream in the opposed decoding layer. We show that injecting the representations from this block can be used for text-based editing, precise modifications, and style transfer. We compare our method, SkipInject, to state-of-the-art style transfer and image editing methods and demonstrate that our method obtains the best content alignment and optimal structural preservation tradeoff.
关于扩散模型在图像生成方面的最新进展,引发了人们对图像编辑中U-Net架构内部几个组件的详细研究。虽然之前的研究主要集中在瓶颈层(h空间)、跨注意力、自注意力以及解码层上,但尚未对U-Net本身的跳跃连接的整体作用进行专门研究。我们对跳跃连接的作用进行了深入分析,发现通过第三个编码器块的残差连接携带了重建图像的大部分空间信息,将从风格中分离出的内容传递给相对解码层中的剩余流。我们证明了注入此块的表示可用于基于文本编辑、精确修改和风格转换。我们将我们的SkipInject方法与最先进的风格转换和图像编辑方法进行了比较,并证明我们的方法在内容对齐和最佳结构保留方面取得了最佳平衡。
论文及项目相关链接
PDF Accepted to CVPR Workshop on AI for Creative Visual Content Generation Editing and Understanding 2025
Summary
近期关于扩散模型在图像生成领域的研究进展引发了人们对U-Net架构中不同组件的深入研究。研究集中在瓶颈层(h-space)、跨注意力、自注意力以及解码层等方面,但尚未具体探讨U-Net的跳跃连接的整体作用。本研究对跳跃连接进行了深入分析,发现通过第三个编码器块的残差连接传递了重建图像的大部分空间信息,实现了内容和风格的分离,并在相对应的解码层传递剩余信息。本研究展示了注入此块表示的方法可用于基于文本编辑、精确修改和风格转换。对比其他先进的风格转换和图像编辑方法,证明本研究方法在内容对齐和结构保持方面取得最佳平衡。
Key Takeaways
- 跳跃连接在图像编辑的U-Net架构中扮演着重要角色,尤其是通过第三个编码器块的残差连接传递了重建图像的大部分空间信息。
- 通过跳跃连接实现了内容和风格的分离,有助于精确修改和风格转换。
- 本研究提出的方法——SkipInject,在文本编辑、精确修改和风格转换方面表现出良好的性能。
- SkipInject与其他先进的风格转换和图像编辑方法相比,在内容对齐和结构保持方面取得了最佳平衡。
- 研究结果突出了跳跃连接在扩散模型中的重要性,为未来研究提供了新方向。
- 该方法具有潜在应用于各种图像编辑场景,如修复、合成和美化等。
点此查看论文截图
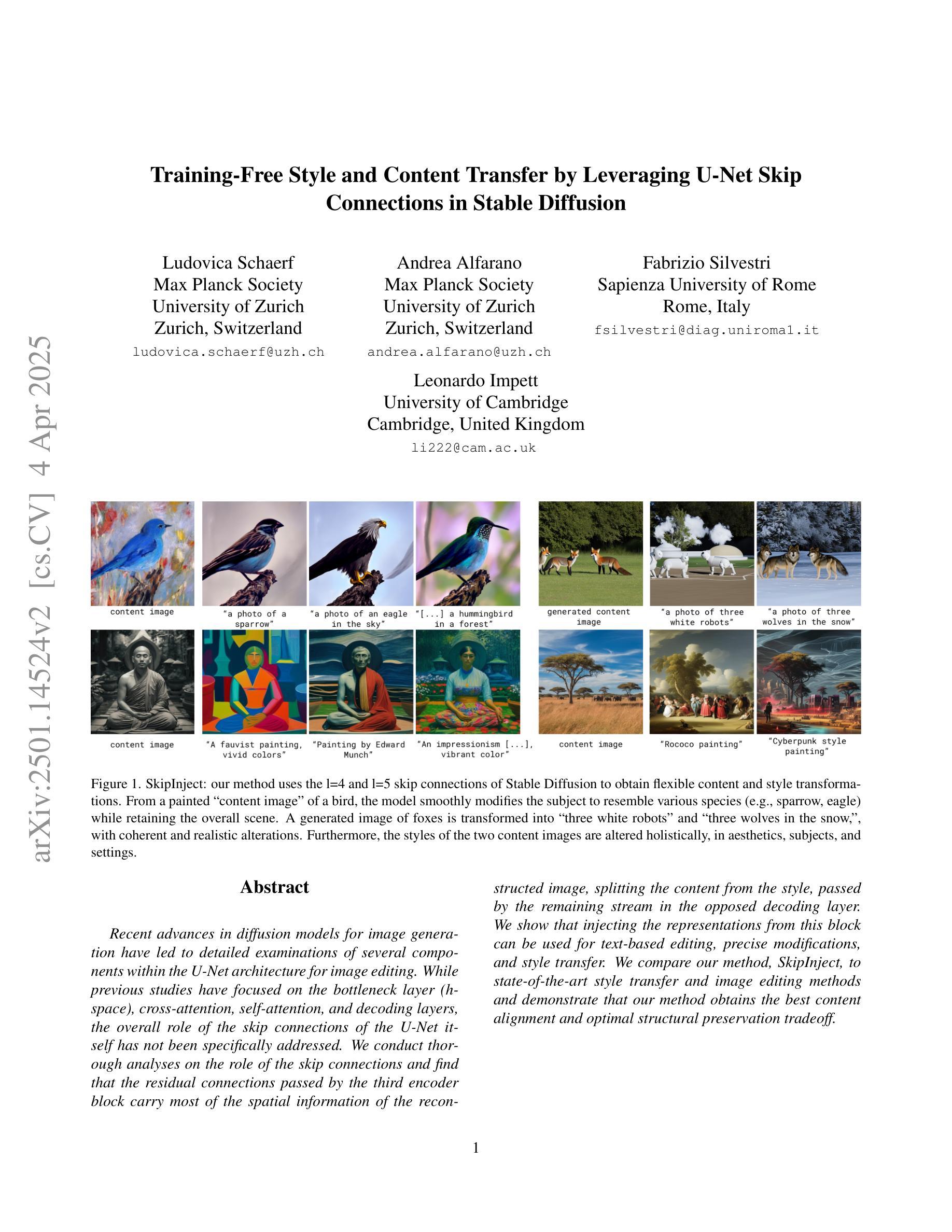
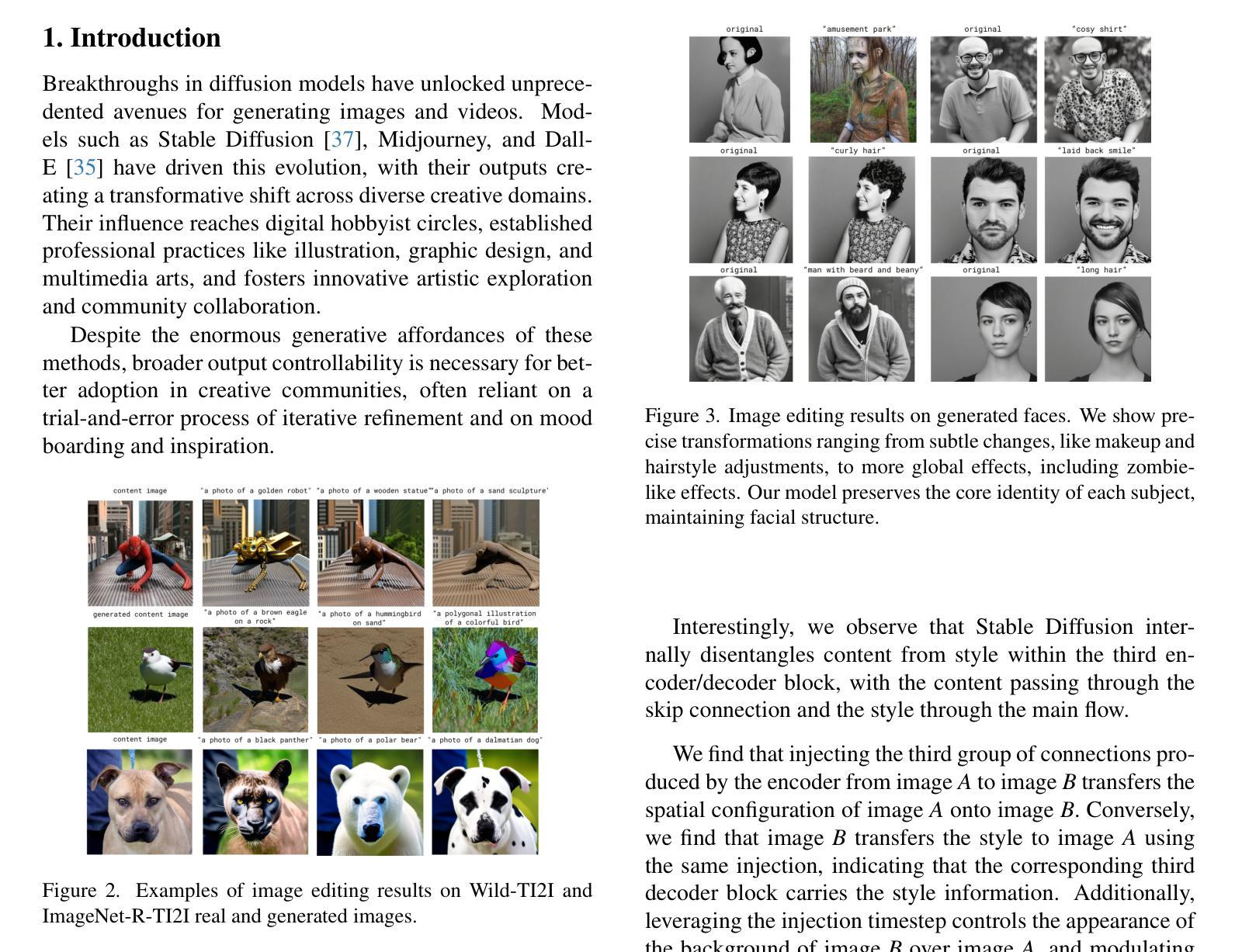
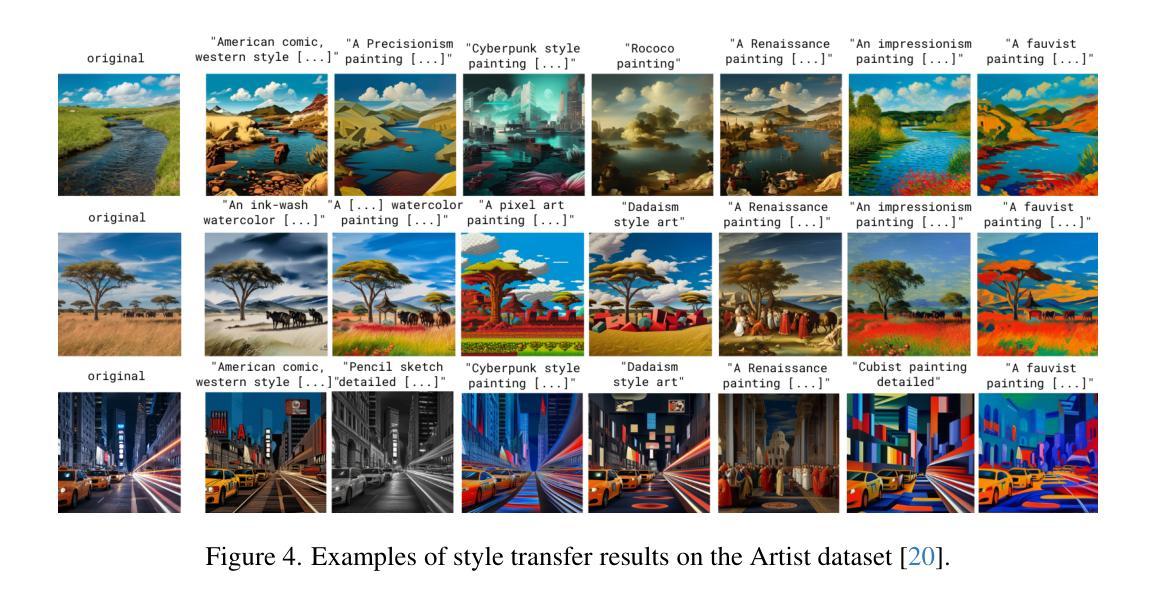
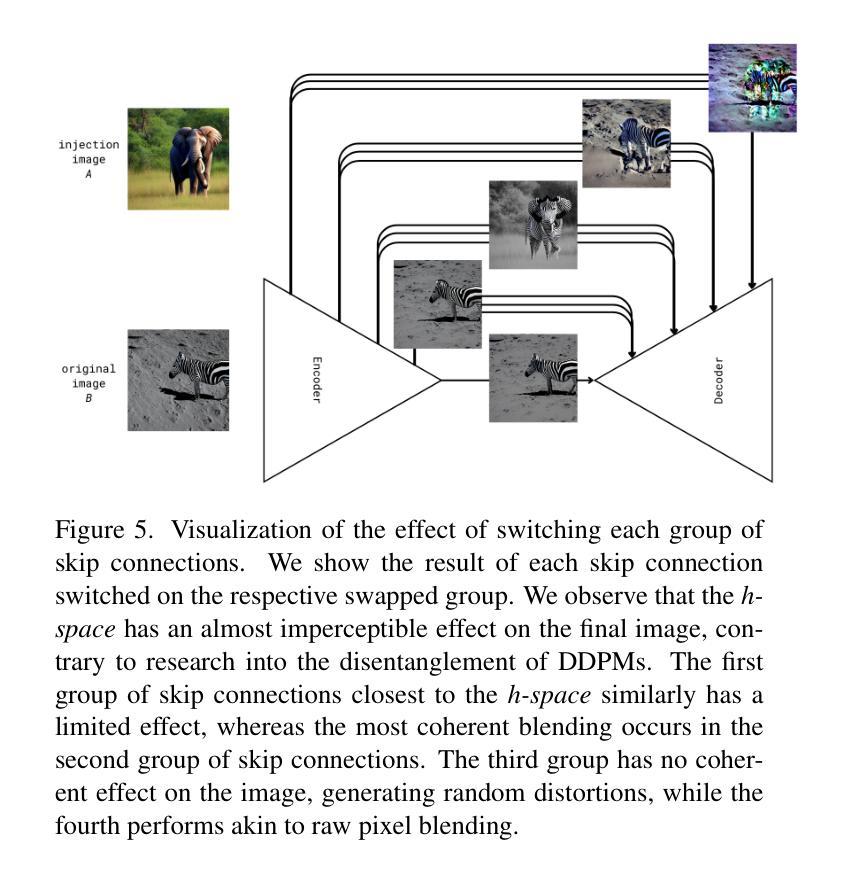
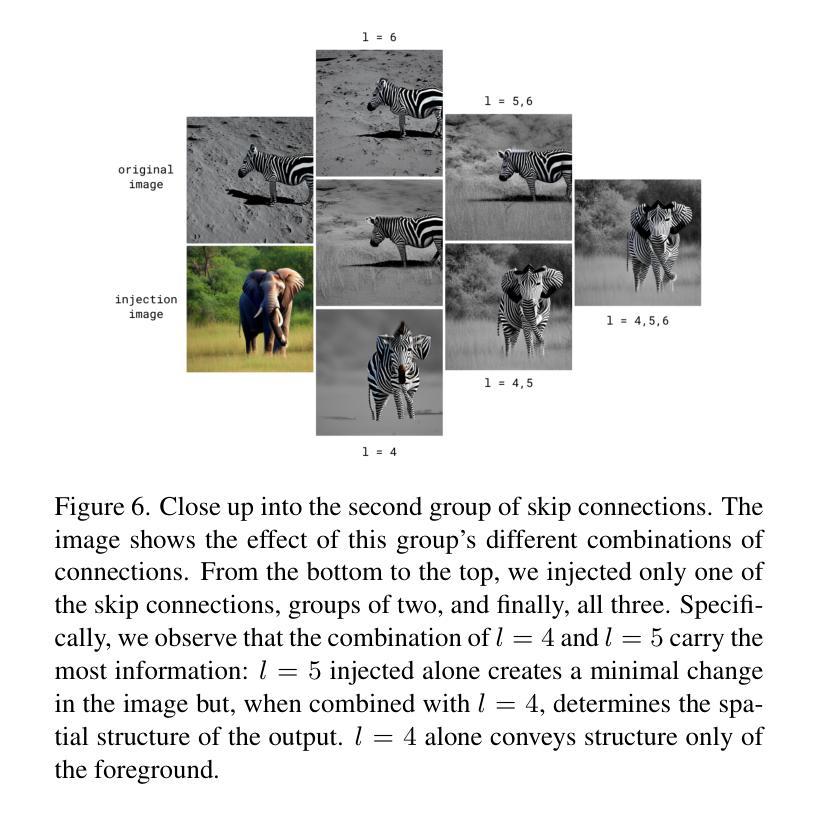
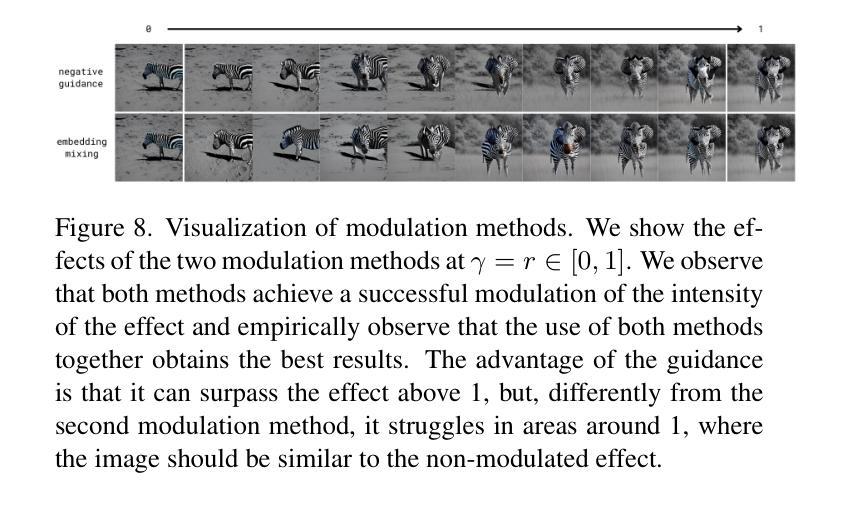
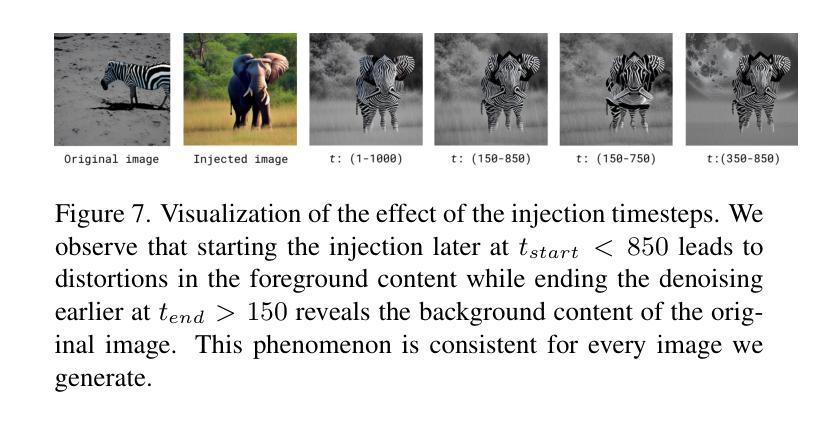
Zero-Shot Image Restoration Using Few-Step Guidance of Consistency Models (and Beyond)
Authors:Tomer Garber, Tom Tirer
In recent years, it has become popular to tackle image restoration tasks with a single pretrained diffusion model (DM) and data-fidelity guidance, instead of training a dedicated deep neural network per task. However, such “zero-shot” restoration schemes currently require many Neural Function Evaluations (NFEs) for performing well, which may be attributed to the many NFEs needed in the original generative functionality of the DMs. Recently, faster variants of DMs have been explored for image generation. These include Consistency Models (CMs), which can generate samples via a couple of NFEs. However, existing works that use guided CMs for restoration still require tens of NFEs or fine-tuning of the model per task that leads to performance drop if the assumptions during the fine-tuning are not accurate. In this paper, we propose a zero-shot restoration scheme that uses CMs and operates well with as little as 4 NFEs. It is based on a wise combination of several ingredients: better initialization, back-projection guidance, and above all a novel noise injection mechanism. We demonstrate the advantages of our approach for image super-resolution, deblurring and inpainting. Interestingly, we show that the usefulness of our noise injection technique goes beyond CMs: it can also mitigate the performance degradation of existing guided DM methods when reducing their NFE count.
近年来,使用单个预训练的扩散模型(DM)和数据保真度指导来解决图像恢复任务变得非常流行,而不是针对每个任务训练一个专用的深度神经网络。然而,这样的“零射击”恢复方案目前需要大量的神经功能评估(NFE)才能表现良好,这可能是由于DM的原始生成功能需要大量的NFE。最近,已经探索了用于图像生成的更快的DM变体。这包括一致性模型(CM),可以通过几个NFE生成样本。然而,现有作品在使用指导性的CM进行恢复时,仍需要数十次的NFE或对模型的微调,如果在微调期间的假设不准确,会导致性能下降。在本文中,我们提出了一种使用CM的零射击恢复方案,仅需极少的4个NFE即可有效运行。它基于明智地结合了多种成分:更好的初始化、反向投影指导和最重要的是一种新型噪声注入机制。我们展示了我们的方法在图像超分辨率、去模糊和图像修复方面的优势。有趣的是,我们证明了我们的噪声注入技术的实用性超出了CM的范围:它还可以缓解现有指导型DM方法减少NFE计数时的性能下降问题。
论文及项目相关链接
PDF CVPR 2025 (camera-ready). Code can be found at: https://github.com/tirer-lab/CM4IR
Summary
本文提出一种基于一致性模型(CMs)的零射图像恢复方案,使用少量(仅需4次)神经功能评估(NFE)即可实现图像超分辨率、去模糊和修复等功能。该方案通过优化初始化、反向投影引导和新型噪声注入机制,不仅提高了CMs的效率,而且能够缓解现有引导扩散模型方法减少NFE计数时的性能下降问题。
Key Takeaways
- 提出了基于一致性模型(CMs)的零射图像恢复方案。
- 使用较少的神经功能评估(NFE)次数(仅需4次)即可实现图像恢复。
- 通过优化初始化、反向投影引导和新型噪声注入机制,提高了恢复效果。
- 新型噪声注入技术不仅适用于一致性模型,还可缓解现有引导扩散模型方法减少NFE时的性能下降。
- 该方案对图像超分辨率、去模糊和修复等任务有优势。
- 此方法提供了一种更高效的图像恢复途径,具有广泛的应用前景。
- 研究为扩散模型在图像恢复任务中的应用提供了新的思路和方法。
点此查看论文截图
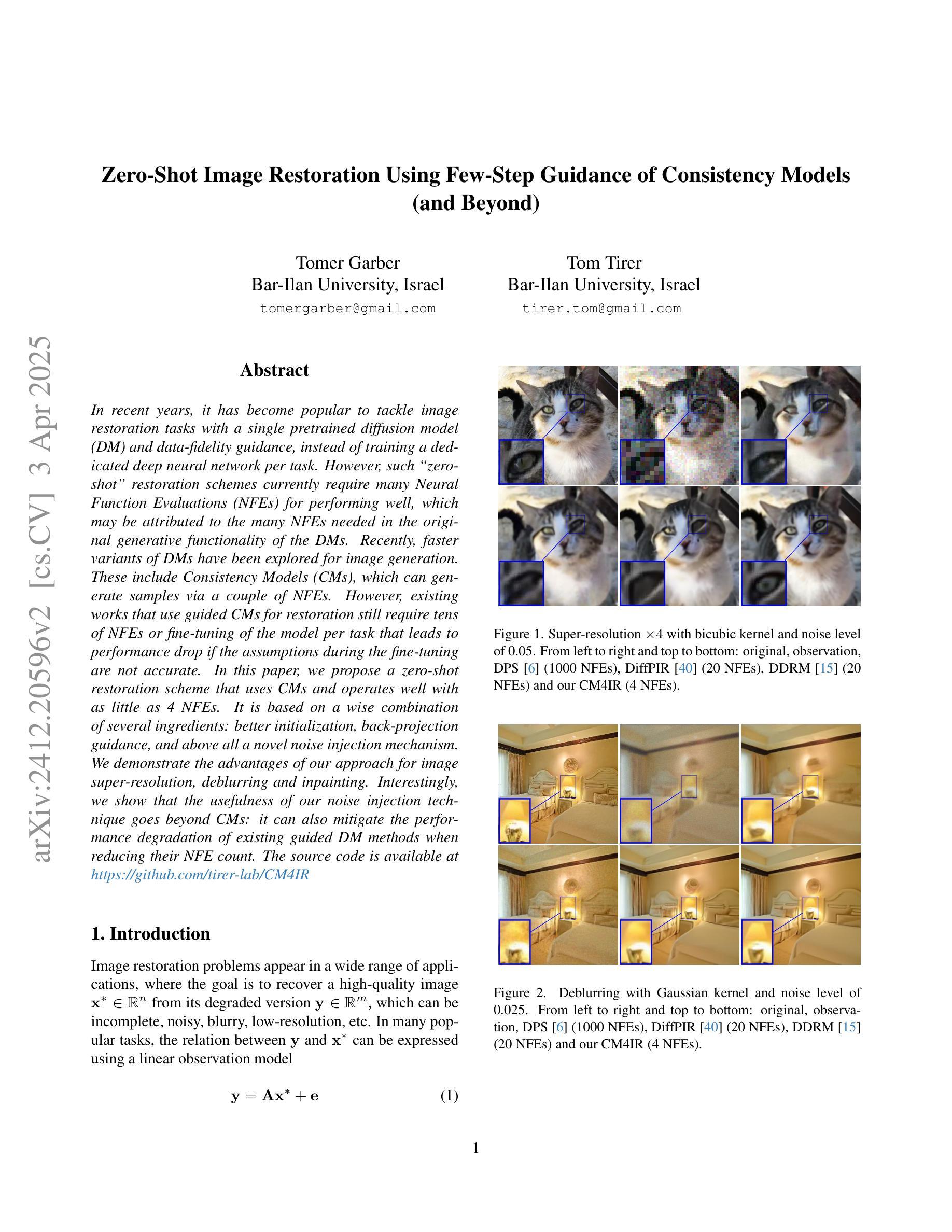
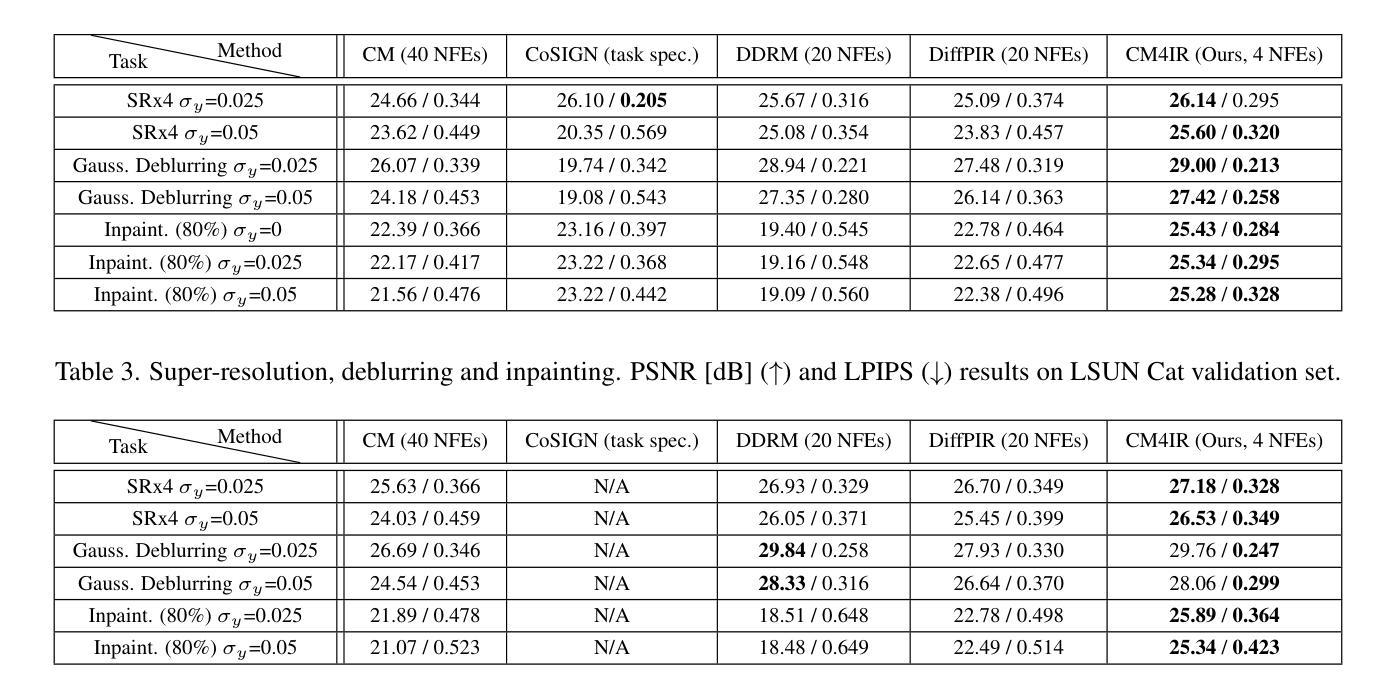

FADA: Fast Diffusion Avatar Synthesis with Mixed-Supervised Multi-CFG Distillation
Authors:Tianyun Zhong, Chao Liang, Jianwen Jiang, Gaojie Lin, Jiaqi Yang, Zhou Zhao
Diffusion-based audio-driven talking avatar methods have recently gained attention for their high-fidelity, vivid, and expressive results. However, their slow inference speed limits practical applications. Despite the development of various distillation techniques for diffusion models, we found that naive diffusion distillation methods do not yield satisfactory results. Distilled models exhibit reduced robustness with open-set input images and a decreased correlation between audio and video compared to teacher models, undermining the advantages of diffusion models. To address this, we propose FADA (Fast Diffusion Avatar Synthesis with Mixed-Supervised Multi-CFG Distillation). We first designed a mixed-supervised loss to leverage data of varying quality and enhance the overall model capability as well as robustness. Additionally, we propose a multi-CFG distillation with learnable tokens to utilize the correlation between audio and reference image conditions, reducing the threefold inference runs caused by multi-CFG with acceptable quality degradation. Extensive experiments across multiple datasets show that FADA generates vivid videos comparable to recent diffusion model-based methods while achieving an NFE speedup of 4.17-12.5 times. Demos are available at our webpage http://fadavatar.github.io.
基于扩散的音频驱动对话式化身方法因其高保真、生动、表达性强的结果而近期备受关注。然而,其缓慢的推理速度限制了实际应用。尽管已经开发了各种用于扩散模型的蒸馏技术,但我们发现简单的扩散蒸馏方法并不能产生令人满意的结果。与原始模型相比,蒸馏模型对开放集输入图像的稳健性降低,音频和视频的关联性减弱,这削弱了扩散模型的优势。为了解决这个问题,我们提出了FADA(具有混合监督多CFG蒸馏的快速扩散化身合成法)。我们首先设计了一种混合监督损失,以利用不同质量的数据,增强模型的整体能力和稳健性。此外,我们提出了一种具有可学习标记的多CFG蒸馏法,以利用音频和参考图像条件之间的相关性,减少因多CFG导致的三倍推理运行,同时保持可接受的质量下降。在多个数据集上的广泛实验表明,FADA生成的视频生动程度与最新的扩散模型方法相当,同时实现了4.17至12.5倍的NFE加速。相关演示请访问我们的网页:网页链接。
论文及项目相关链接
PDF CVPR 2025, Homepage https://fadavatar.github.io/
Summary
本文介绍了基于扩散的音频驱动说话人偶方法的高保真、生动、表达性强的结果,但其缓慢的推理速度限制了实际应用。为了解决这一问题,作者提出了FADA(带有混合监督多CFG蒸馏的快速扩散化身合成)。FADA利用不同质量的数据设计了一种混合监督损失,提高了模型的整体能力和鲁棒性。此外,作者还提出了一种带有可学习令牌的多CFG蒸馏方法,利用音频和参考图像条件之间的相关性,减少了多CFG引起的三倍推理运行的可接受的性能下降。实验表明,FADA生成的视频生动,与基于扩散模型的最新方法相当,同时实现了NFE速度的4.17至12.5倍提升。
Key Takeaways
- 扩散模型在音频驱动的说话人偶方法中表现出高保真、生动和表达性强的结果。
- 现有方法的推理速度较慢,限制了实际应用。
- FADA方法通过混合监督损失提高模型的整体能力和鲁棒性。
- FADA利用音频和参考图像条件之间的相关性,提出多CFG蒸馏方法。
- FADA方法减少了多CFG引起的推理运行次数。
- 实验证明FADA生成的视频质量生动,与最新扩散模型相当。
点此查看论文截图
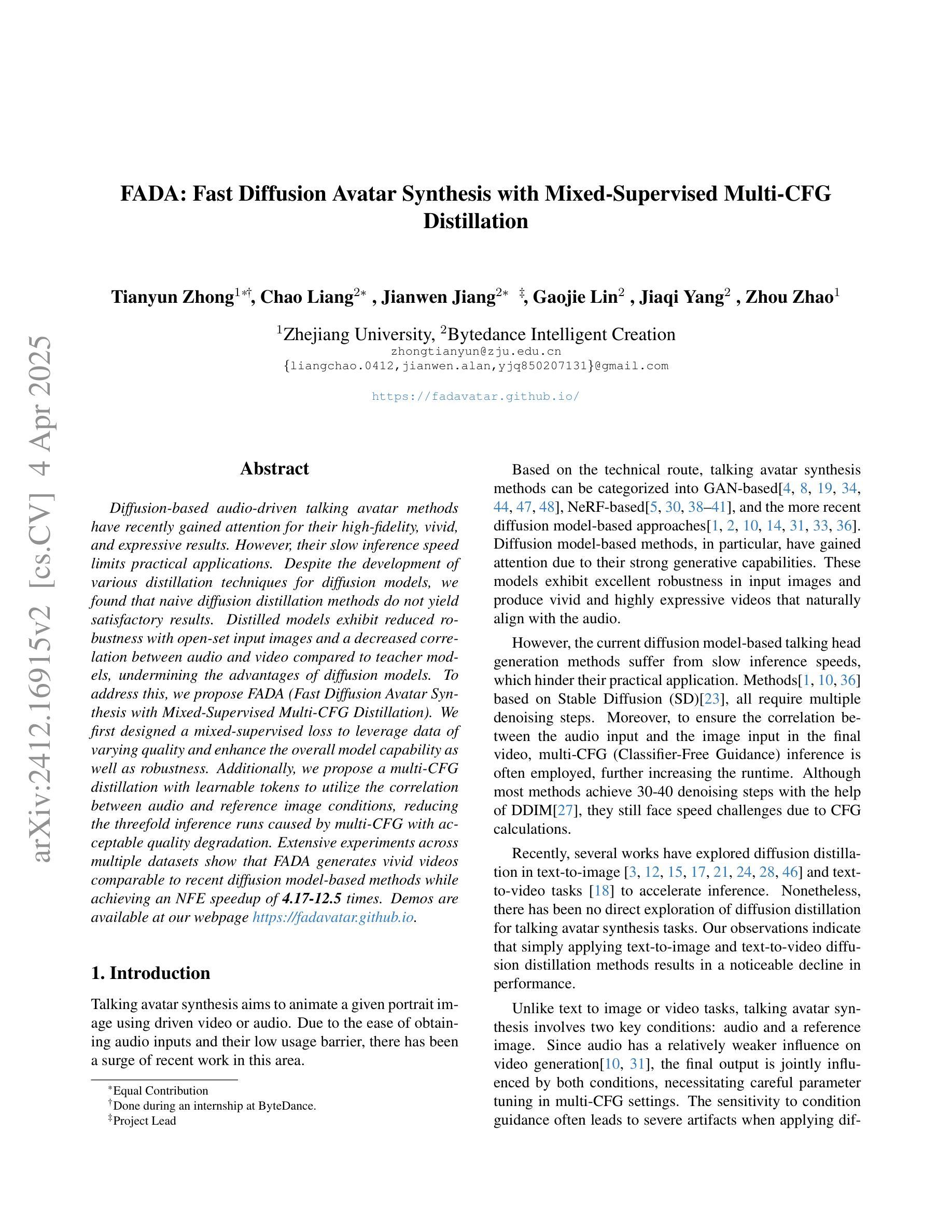
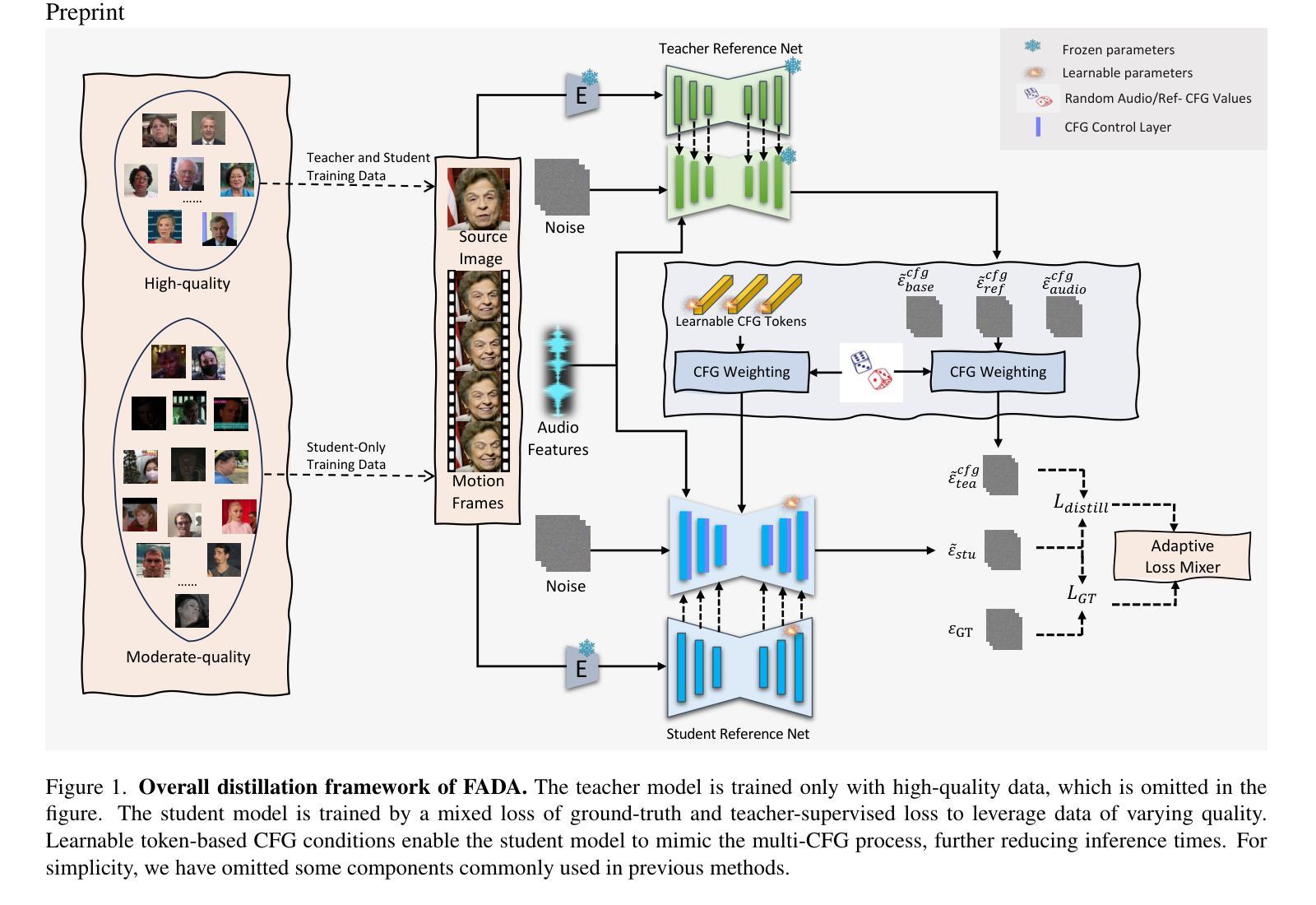
Edge-SD-SR: Low Latency and Parameter Efficient On-device Super-Resolution with Stable Diffusion via Bidirectional Conditioning
Authors:Mehdi Noroozi, Isma Hadji, Victor Escorcia, Anestis Zaganidis, Brais Martinez, Georgios Tzimiropoulos
There has been immense progress recently in the visual quality of Stable Diffusion-based Super Resolution (SD-SR). However, deploying large diffusion models on computationally restricted devices such as mobile phones remains impractical due to the large model size and high latency. This is compounded for SR as it often operates at high res (e.g. 4Kx3K). In this work, we introduce Edge-SD-SR, the first parameter efficient and low latency diffusion model for image super-resolution. Edge-SD-SR consists of ~169M parameters, including UNet, encoder and decoder, and has a complexity of only ~142 GFLOPs. To maintain a high visual quality on such low compute budget, we introduce a number of training strategies: (i) A novel conditioning mechanism on the low resolution input, coined bidirectional conditioning, which tailors the SD model for the SR task. (ii) Joint training of the UNet and encoder, while decoupling the encodings of the HR and LR images and using a dedicated schedule. (iii) Finetuning the decoder using the UNet’s output to directly tailor the decoder to the latents obtained at inference time. Edge-SD-SR runs efficiently on device, e.g. it can upscale a 128x128 patch to 512x512 in 38 msec while running on a Samsung S24 DSP, and of a 512x512 to 2048x2048 (requiring 25 model evaluations) in just ~1.1 sec. Furthermore, we show that Edge-SD-SR matches or even outperforms state-of-the-art SR approaches on the most established SR benchmarks.
最近,基于Stable Diffusion的超分辨率(SD-SR)在视觉质量方面取得了巨大的进步。然而,由于模型规模庞大和延迟较高,将大型扩散模型部署在计算受限的设备上(如手机)仍然不切实际。对于超分辨率(SR)任务来说,这种情况更为严重,因为它通常在高分辨率(例如4Kx3K)下运行。在这项工作中,我们引入了Edge-SD-SR,这是第一个用于图像超分辨率的参数高效、低延迟的扩散模型。Edge-SD-SR包含约1.69亿个参数,包括UNet、编码器和解码器,复杂度仅为约142 GFLOPs。为了在这种低计算预算下保持高视觉质量,我们引入了许多训练策略:(i)一种新型的针对低分辨率输入的条件机制,称为双向条件机制,该机制为SD模型定制了SR任务。(ii)联合训练UNet和编码器,同时解耦高低分辨率图像的编码并使用专门的调度方案。(iii)使用UNet的输出对解码器进行微调,以直接针对推理时间获得的潜在表示定制解码器。Edge-SD-SR在设备上运行高效,例如,它可以在Samsung S24 DSP上运行,将128x128的补丁放大到512x512需要38毫秒,而将512x512放大到2048x2048(需要25次模型评估)只需约1.1秒。此外,我们还表明,Edge-SD-SR在最成熟的超分辨率基准测试中达到了或超越了最先进的超分辨率方法。
论文及项目相关链接
PDF Accepted to CVPR 2025
摘要
近期Stable Diffusion基于Super Resolution(SD-SR)的视觉质量取得了巨大进展。然而,在移动设备等计算受限的设备上部署大型扩散模型仍不实际。本研究引入Edge-SD-SR,这是一个参数高效、低延迟的图像超分辨率扩散模型。Edge-SD-SR包含约1.69亿个参数,包括UNet、编码器和解码器,复杂度仅为约142 GFLOPs。为了在这种低计算预算上保持高视觉质量,我们采用了多种训练策略。Edge-SD-SR在设备上运行高效,例如,在三星S24 DSP上运行可将一个128x128的补丁放大到512x512,耗时仅38毫秒,将一个512x512放大到2048x2048(需要25次模型评估)也仅需约1.1秒。此外,我们证明Edge-SD-SR在最具代表性的超分辨率基准测试中,匹配甚至超越了最先进的超分辨率方法。
关键见解
- Edge-SD-SR是首个针对图像超分辨率的参数高效、低延迟的扩散模型。
- Edge-SD-SR模型包含约169M参数,复杂度为~142 GFLOPs,实现了在有限计算资源下的高效运行。
- 引入新型条件机制——双向条件,针对超分辨率任务定制SD模型。
- 联合训练UNet和编码器,同时解码高分辨率和低分辨率图像的编码,并使用专用时间表。
- 通过利用UNet的输出对解码器进行微调,使其适应推理时间获得的潜在表示。
- Edge-SD-SR在移动设备上的运行效率极高,如放大图像补丁的速度非常快。
- Edge-SD-SR在超分辨率基准测试中表现优异,甚至超越现有先进技术。
点此查看论文截图
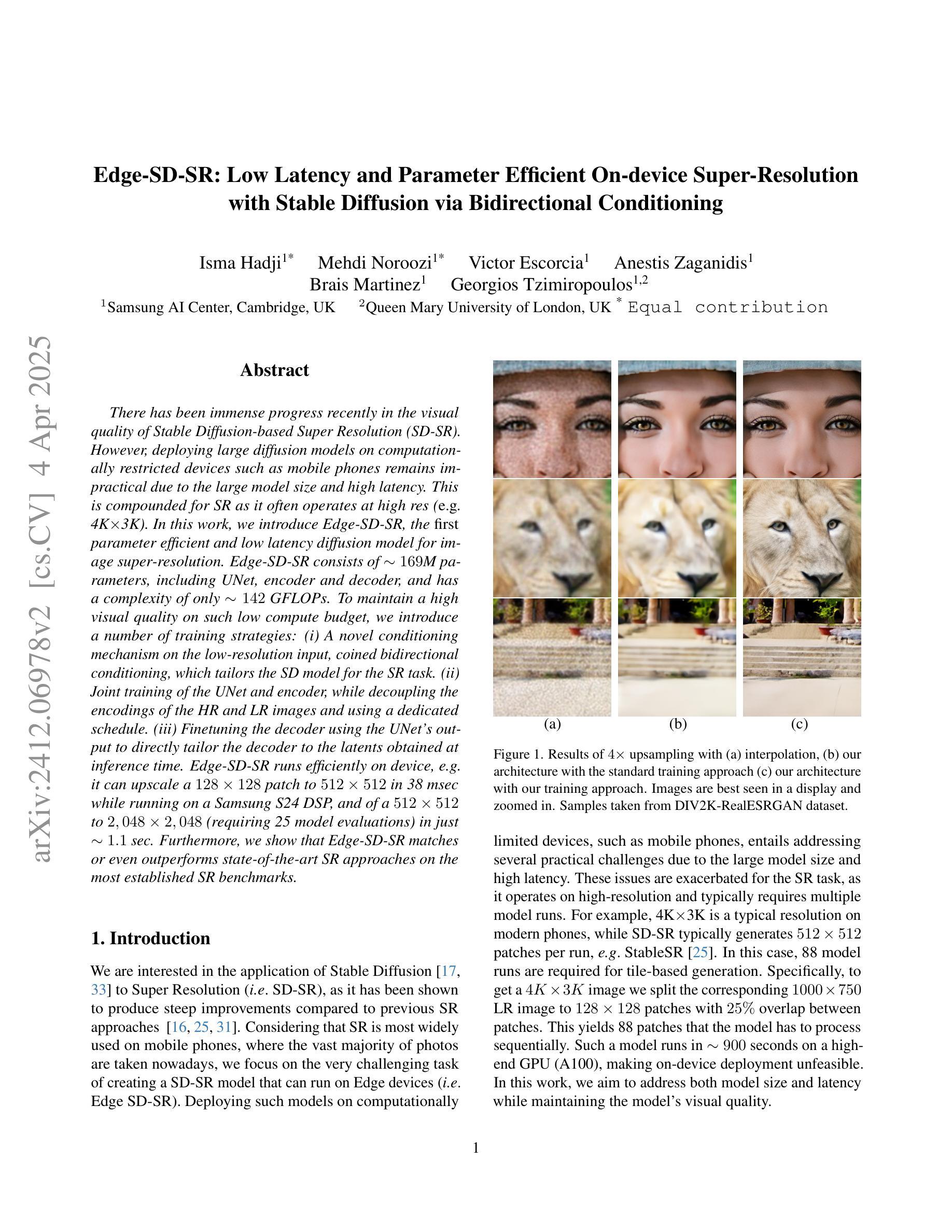
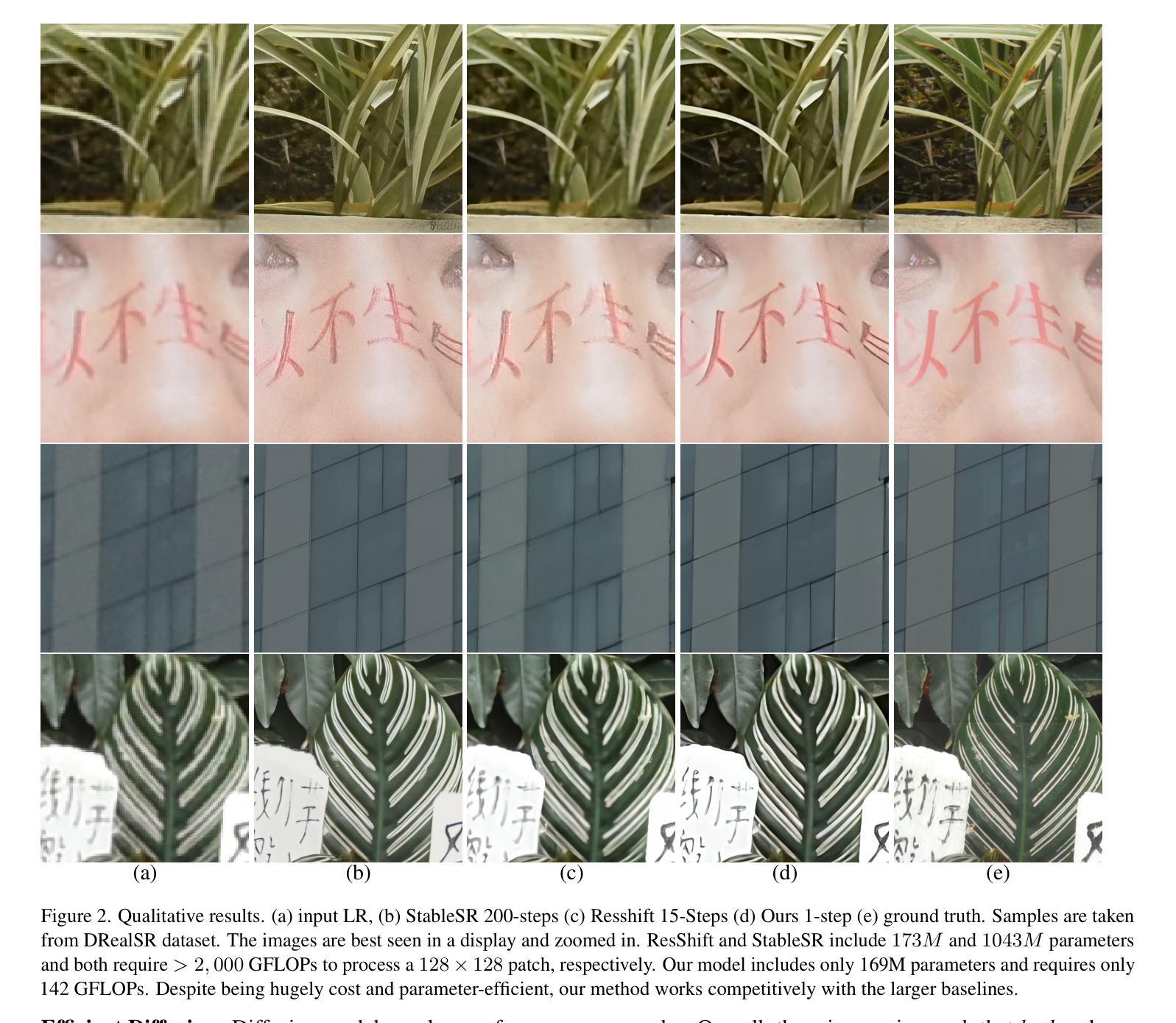
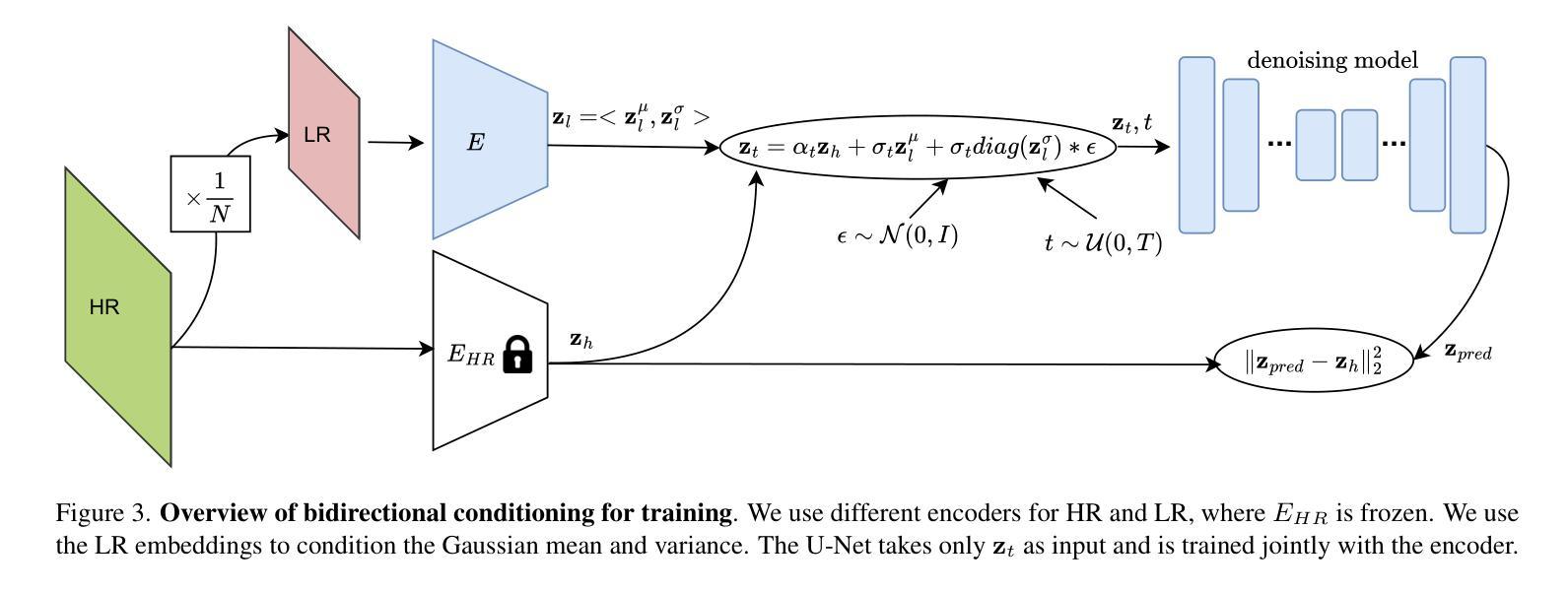
Minority-Focused Text-to-Image Generation via Prompt Optimization
Authors:Soobin Um, Jong Chul Ye
We investigate the generation of minority samples using pretrained text-to-image (T2I) latent diffusion models. Minority instances, in the context of T2I generation, can be defined as ones living on low-density regions of text-conditional data distributions. They are valuable for various applications of modern T2I generators, such as data augmentation and creative AI. Unfortunately, existing pretrained T2I diffusion models primarily focus on high-density regions, largely due to the influence of guided samplers (like CFG) that are essential for high-quality generation. To address this, we present a novel framework to counter the high-density-focus of T2I diffusion models. Specifically, we first develop an online prompt optimization framework that encourages emergence of desired properties during inference while preserving semantic contents of user-provided prompts. We subsequently tailor this generic prompt optimizer into a specialized solver that promotes generation of minority features by incorporating a carefully-crafted likelihood objective. Extensive experiments conducted across various types of T2I models demonstrate that our approach significantly enhances the capability to produce high-quality minority instances compared to existing samplers. Code is available at https://github.com/soobin-um/MinorityPrompt.
我们研究了使用预训练的文本到图像(T2I)潜在扩散模型生成少数样本。在T2I生成的背景下,少数实例可以被定义为存在于文本条件数据分布的低密度区域的实例。它们对于现代T2I生成器的各种应用,如数据增强和创意AI,都是非常有价值的。然而,现有的预训练T2I扩散模型主要关注高密度区域,这主要是因为引导采样器(如CFG)的影响,对于高质量生成至关重要。为了解决这一问题,我们提出了一个新型框架来对抗T2I扩散模型的高密度聚焦。具体来说,我们首先开发了一个在线提示优化框架,该框架在推理过程中鼓励所需属性的出现,同时保留用户提供的提示的语义内容。随后,我们将这个通用提示优化器定制为一个专用求解器,通过引入精心设计的可能性目标来促进少数特征的产生。对多种类型的T2I模型进行的广泛实验表明,我们的方法相较于现有采样器,在生成高质量少数样本方面能力显著增强。代码可通过https://github.com/soobin-um/MinorityPrompt获取。
论文及项目相关链接
PDF CVPR 2025 (Oral), 21 pages, 10 figures
Summary
本文研究了使用预训练的文本到图像(T2I)潜在扩散模型生成少数样本的方法。少数实例在T2I生成中指的是在文本条件数据分布的低密度区域的样本,对于现代T2I生成器的各种应用,如数据增强和创意AI,具有重要的价值。然而,现有的预训练T2I扩散模型主要关注高密度区域,这主要是由于引导采样器(如CFG)的影响,对于高质量生成至关重要。为解决这个问题,本文提出了一个框架来对抗T2I扩散模型的高密度关注。首先,我们开发了一个在线提示优化框架,该框架在推理过程中鼓励所需属性的出现,同时保留用户提供的提示的语义内容。随后,我们将这个通用的提示优化器定制成一个专用求解器,通过引入精心设计的似然目标来促进少数特征的产生。在多种类型的T2I模型上进行的广泛实验表明,与现有的采样器相比,我们的方法显著提高了生成少数实例的能力。
Key Takeaways
- 研究了如何利用预训练的文本到图像(T2I)潜在扩散模型生成少数样本。
- 少数实例指的是在文本条件数据分布的低密度区域的样本,对现代T2I生成器的应用至关重要。
- 现有预训练T2I扩散模型主要关注高密度区域,这是由于引导采样器的影响。
- 提出一个框架来对抗T2I扩散模型的高密度关注,包括在线提示优化和专用求解器的开发。
- 在线提示优化框架在推理过程中鼓励所需属性的出现,同时保留用户提供的提示的语义内容。
- 专用求解器通过引入精心设计的似然目标来促进少数特征的产生。
点此查看论文截图
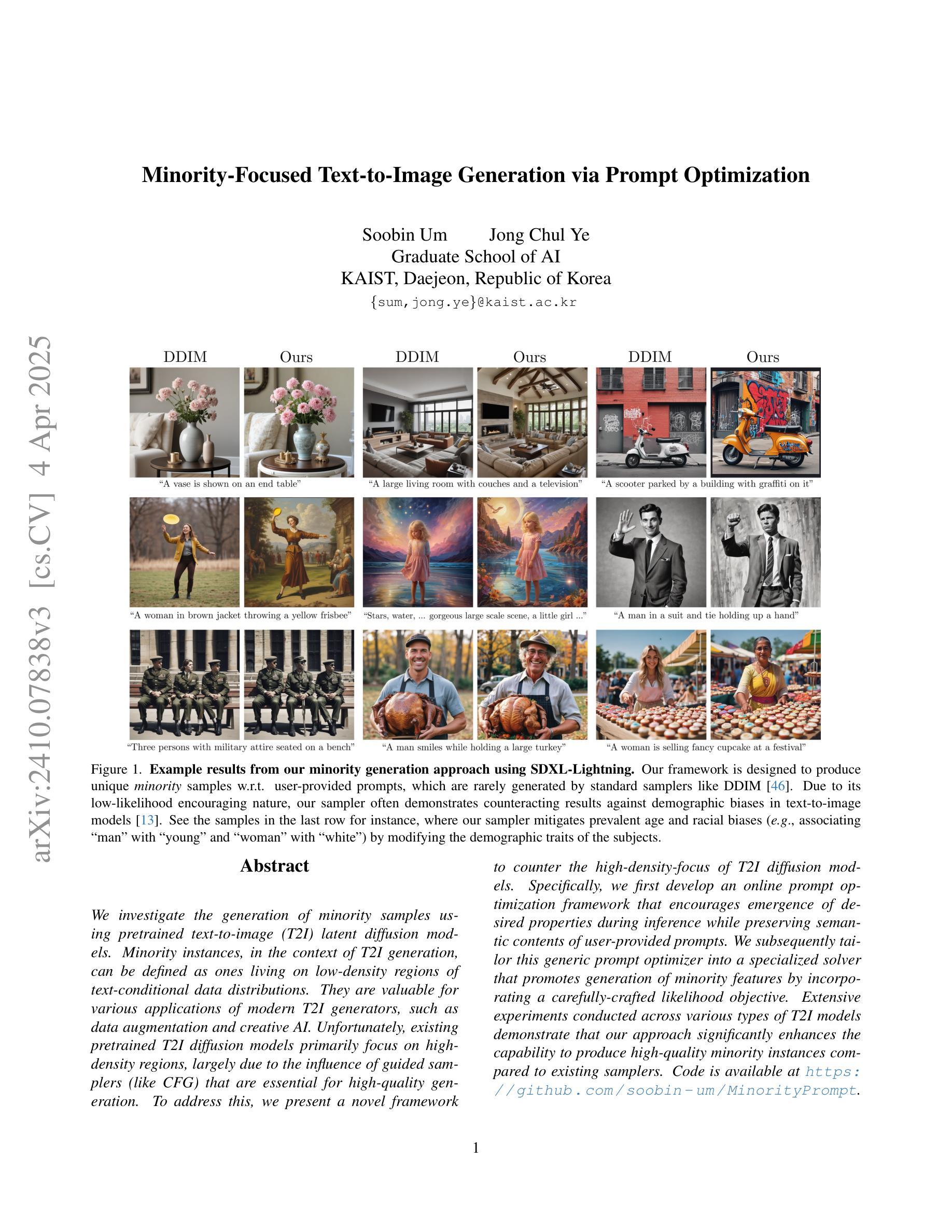
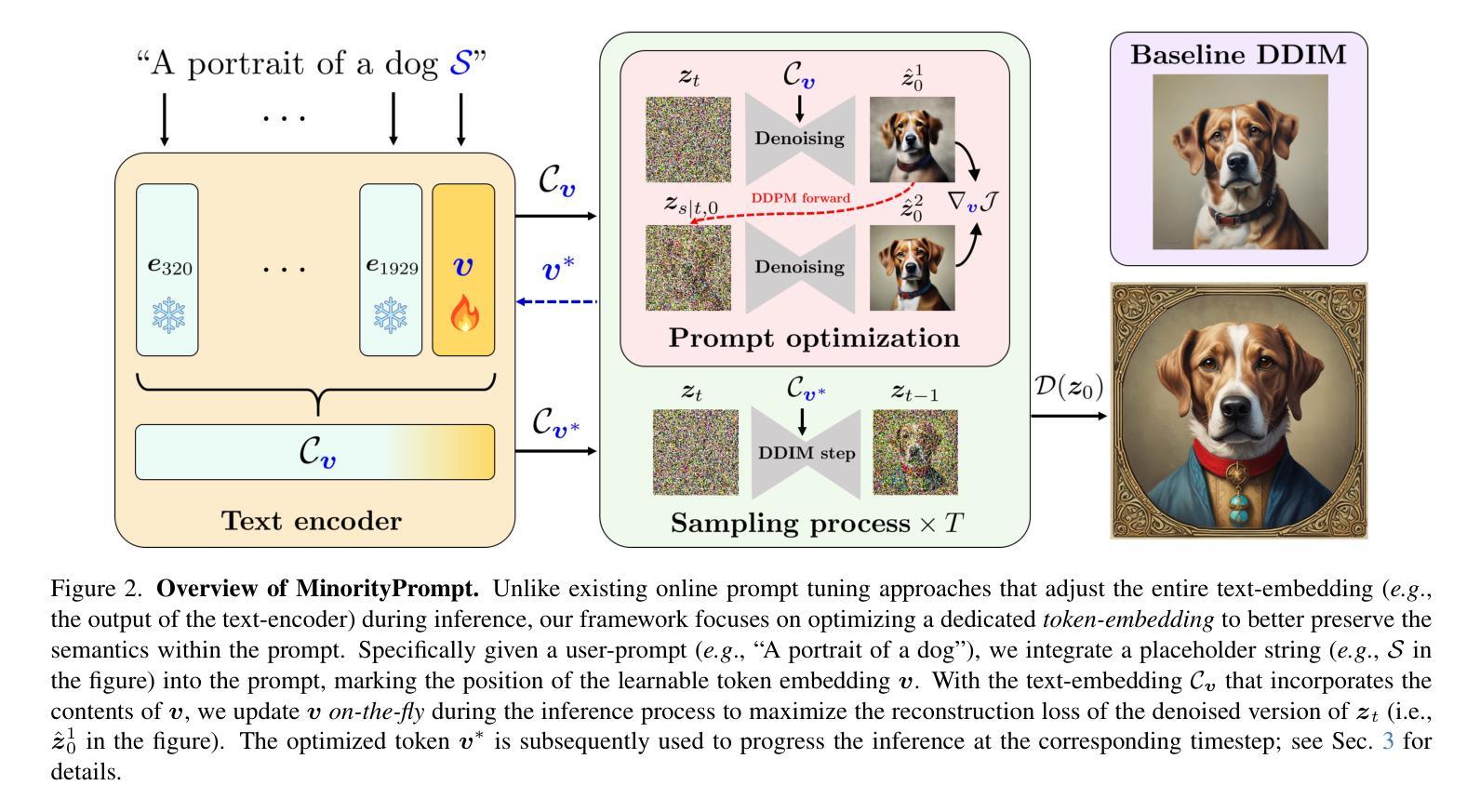
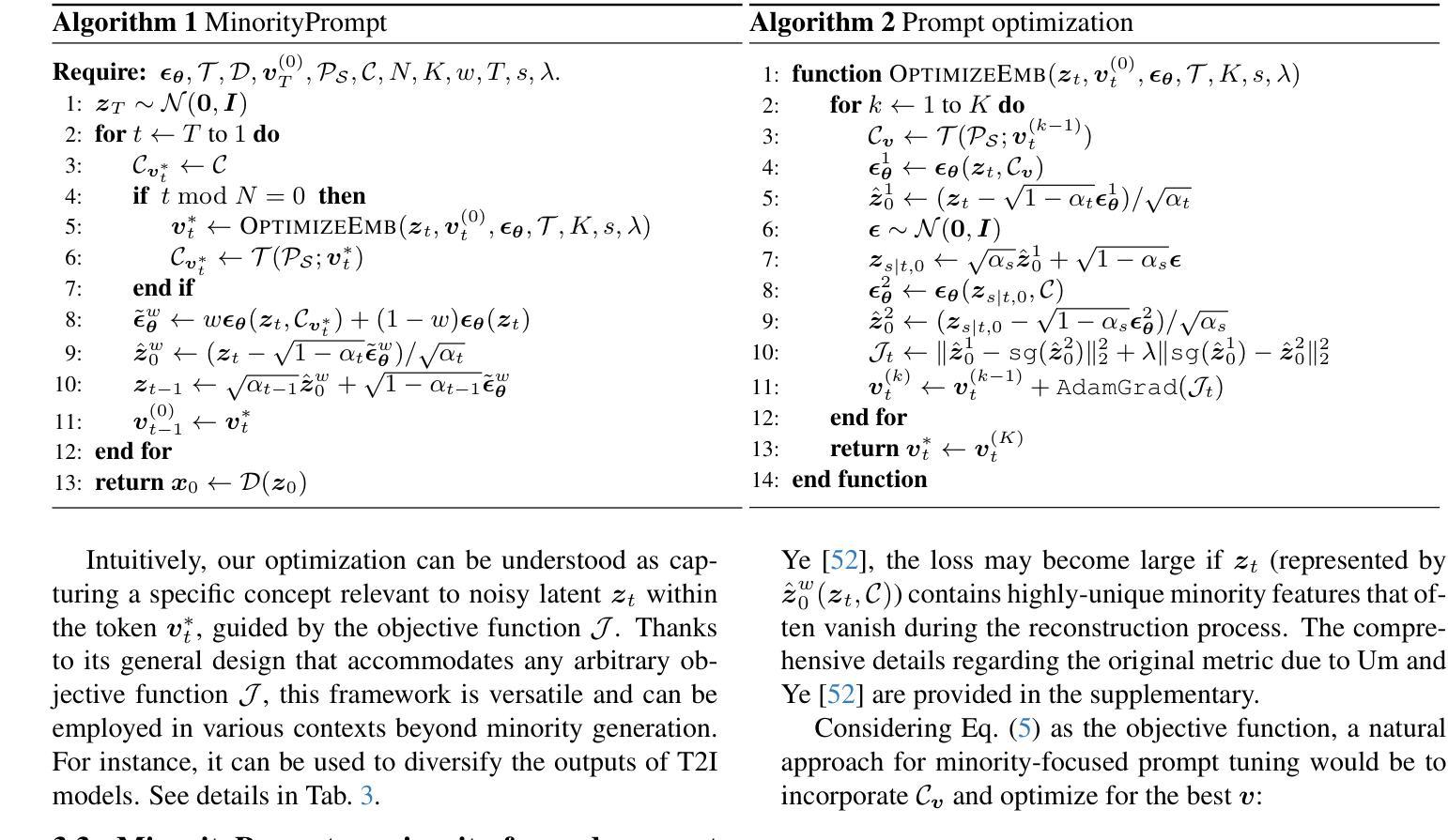
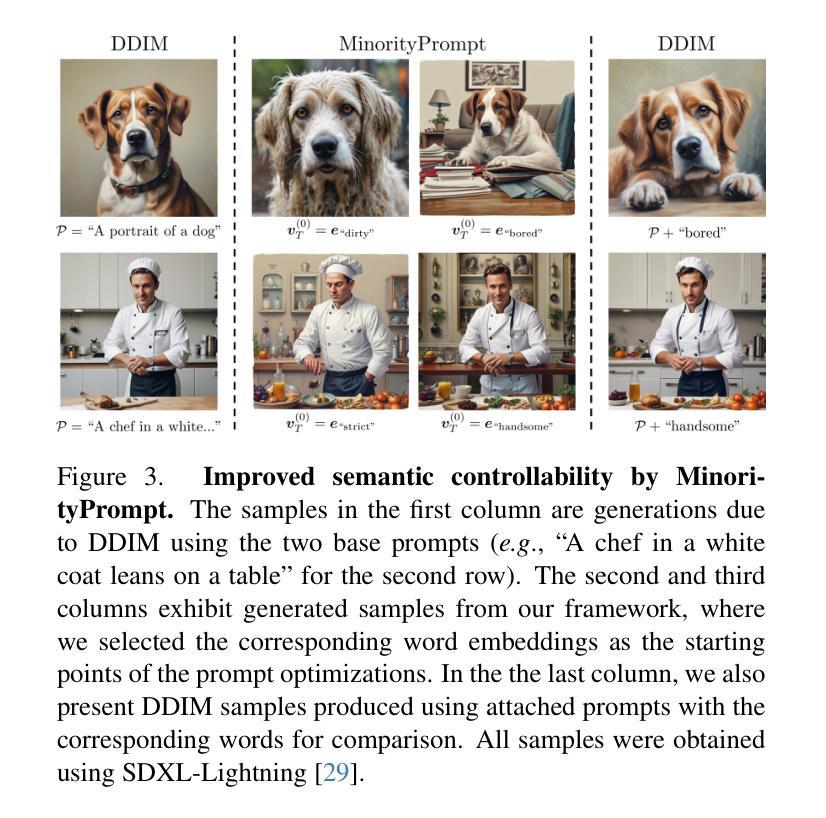
ReviveDiff: A Universal Diffusion Model for Restoring Images in Adverse Weather Conditions
Authors:Wenfeng Huang, Guoan Xu, Wenjing Jia, Stuart Perry, Guangwei Gao
Images captured in challenging environments–such as nighttime, smoke, rainy weather, and underwater–often suffer from significant degradation, resulting in a substantial loss of visual quality. The effective restoration of these degraded images is critical for the subsequent vision tasks. While many existing approaches have successfully incorporated specific priors for individual tasks, these tailored solutions limit their applicability to other degradations. In this work, we propose a universal network architecture, dubbed ``ReviveDiff’’, which can address various degradations and bring images back to life by enhancing and restoring their quality. Our approach is inspired by the observation that, unlike degradation caused by movement or electronic issues, quality degradation under adverse conditions primarily stems from natural media (such as fog, water, and low luminance), which generally preserves the original structures of objects. To restore the quality of such images, we leveraged the latest advancements in diffusion models and developed ReviveDiff to restore image quality from both macro and micro levels across some key factors determining image quality, such as sharpness, distortion, noise level, dynamic range, and color accuracy. We rigorously evaluated ReviveDiff on seven benchmark datasets covering five types of degrading conditions: Rainy, Underwater, Low-light, Smoke, and Nighttime Hazy. Our experimental results demonstrate that ReviveDiff outperforms the state-of-the-art methods both quantitatively and visually.
在具有挑战性的环境中捕捉的图像,如夜间、烟雾、雨天和水下环境,常常会出现显著的退化,导致视觉质量的大量损失。这些退化图像的有效恢复对后续的视觉任务至关重要。虽然许多现有方法已经成功地结合了针对各个任务的特定先验知识,但这些定制解决方案限制了它们在处理其他退化问题时的适用性。在这项工作中,我们提出了一种名为“ReviveDiff”的通用网络架构,它可以解决各种退化问题,并通过增强和恢复图像质量使图像恢复生机。我们的方法受到以下观察结果的启发:与运动或电子问题引起的退化不同,恶劣条件下的质量退化主要源于自然媒介(如雾、水和低亮度),这些媒介通常保留了物体的原始结构。为了恢复此类图像的质量,我们利用扩散模型的最新进展,并开发了ReviveDiff来从宏观和微观层面恢复图像质量,涉及决定图像质量的关键因素,如清晰度、失真、噪声水平、动态范围和色彩准确性。我们在七个基准数据集上对ReviveDiff进行了严格评估,涵盖了五种退化条件:雨天、水下、低光、烟雾和夜间朦胧。我们的实验结果表明,ReviveDiff在定量和视觉上均优于最新方法。
论文及项目相关链接
Summary
本文提出了一种名为“ReviveDiff”的通用网络架构,该架构能够应对各种图像退化问题,并在恶劣条件下恢复图像质量。通过对扩散模型的最新进展加以利用,ReviveDiff能够从宏观和微观层面恢复图像质量,涵盖决定图像质量的关键因素,如清晰度、失真、噪声水平、动态范围和色彩准确性。在七个基准数据集上的实验结果表明,ReviveDiff在五种退化条件下均优于现有方法。
Key Takeaways
- 图像在恶劣环境(如夜间、烟雾、雨天、水下等)中捕捉时,会遭受显著退化,导致视觉质量损失。
- 有效恢复这些退化图像对于后续视觉任务至关重要。
- 现有方法通常针对特定任务融入特定先验知识,但这种方法限制了它们在处理其他退化问题时的适用性。
- 提出的“ReviveDiff”网络架构旨在解决各种退化问题,使图像恢复生机。
- ReviveDiff利用扩散模型的最新进展,能够从宏观和微观层面恢复图像质量。
- ReviveDiff在多个基准数据集上进行了严格评估,覆盖多种退化条件,包括雨天、水下、低光、烟雾和夜间雾霾。
点此查看论文截图
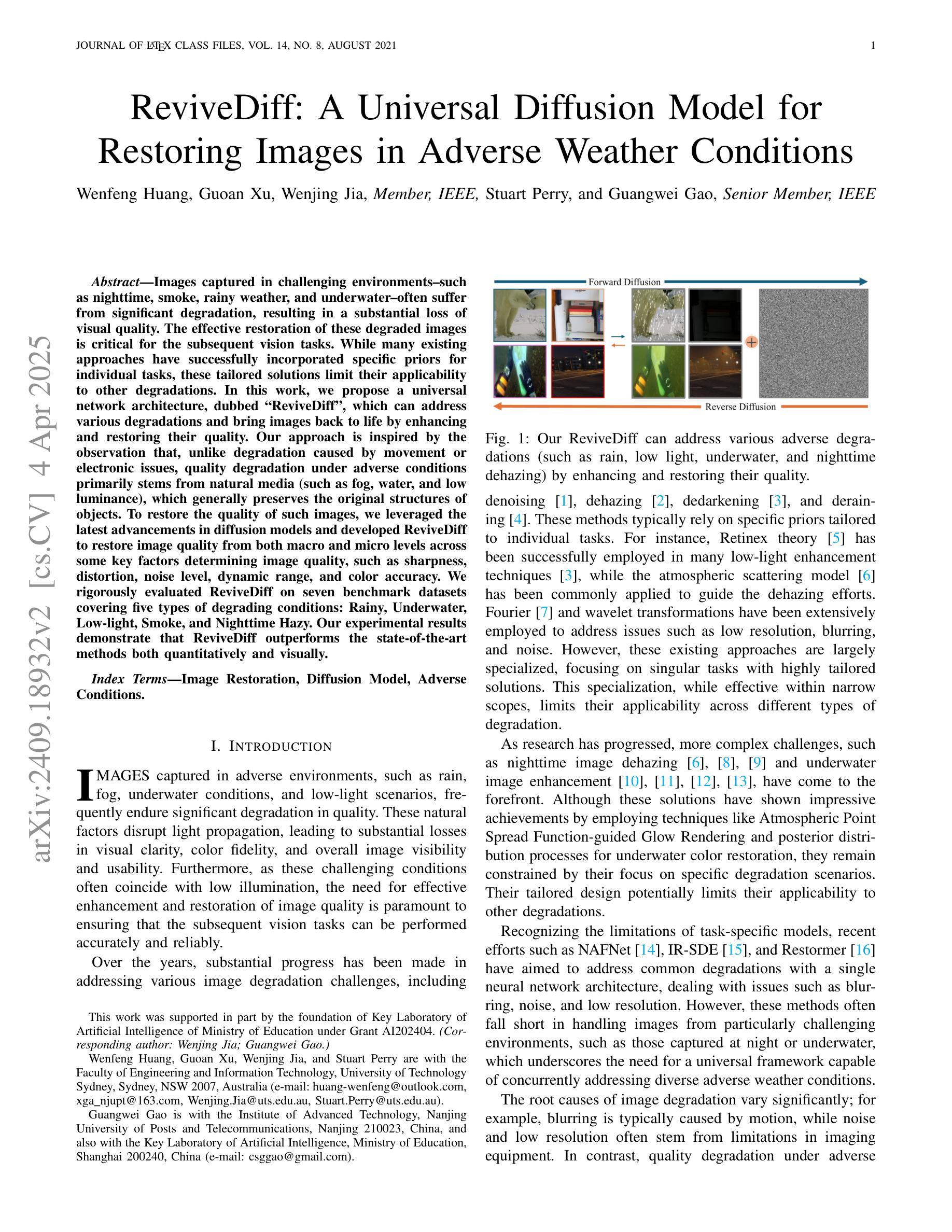
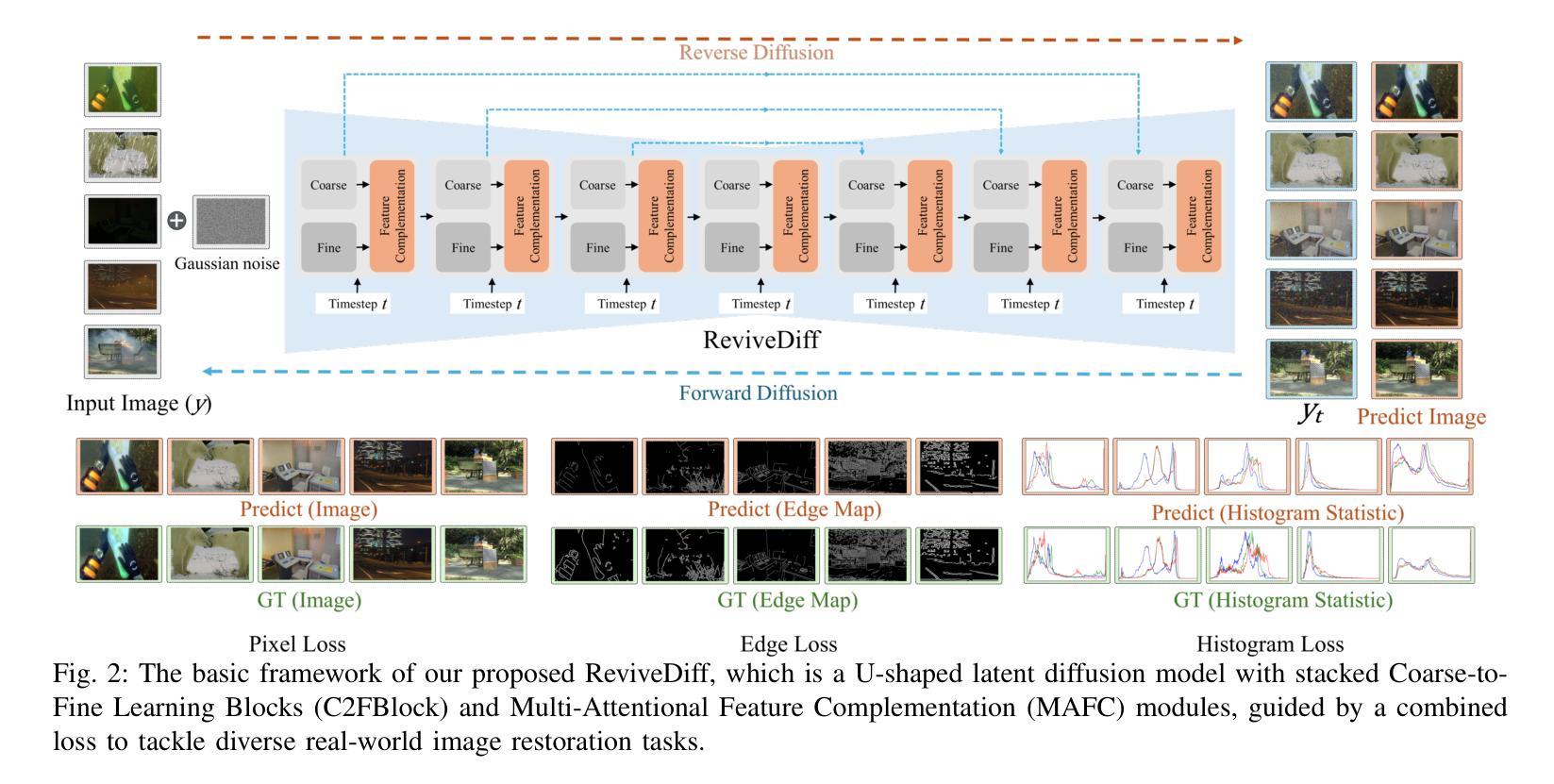
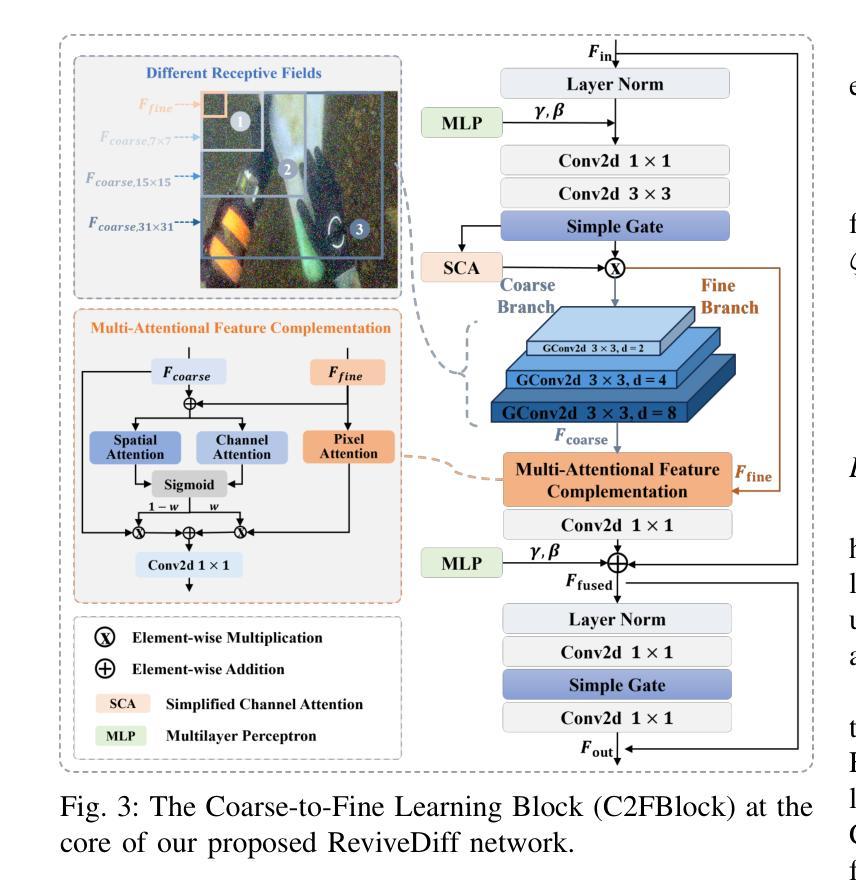
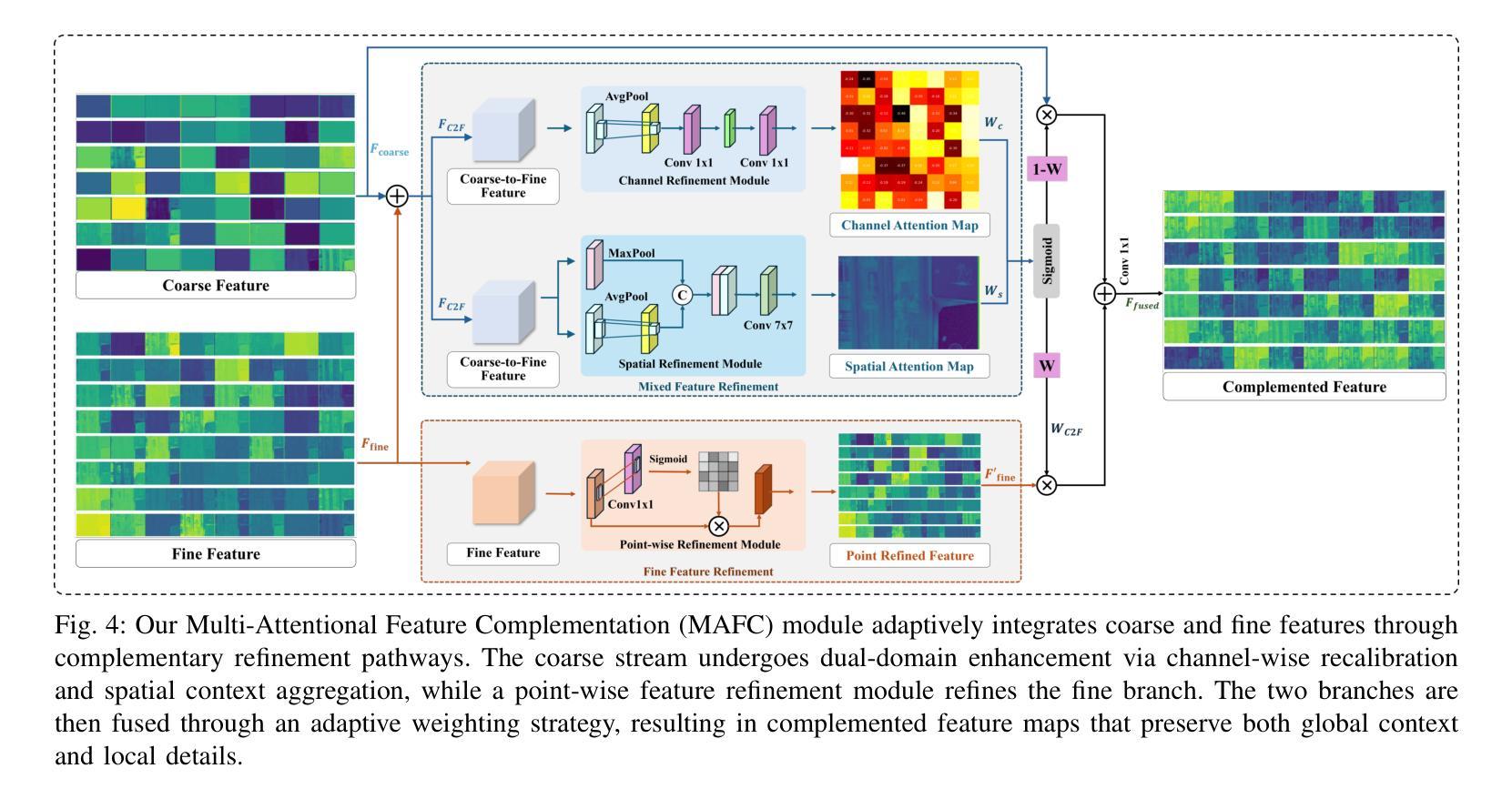
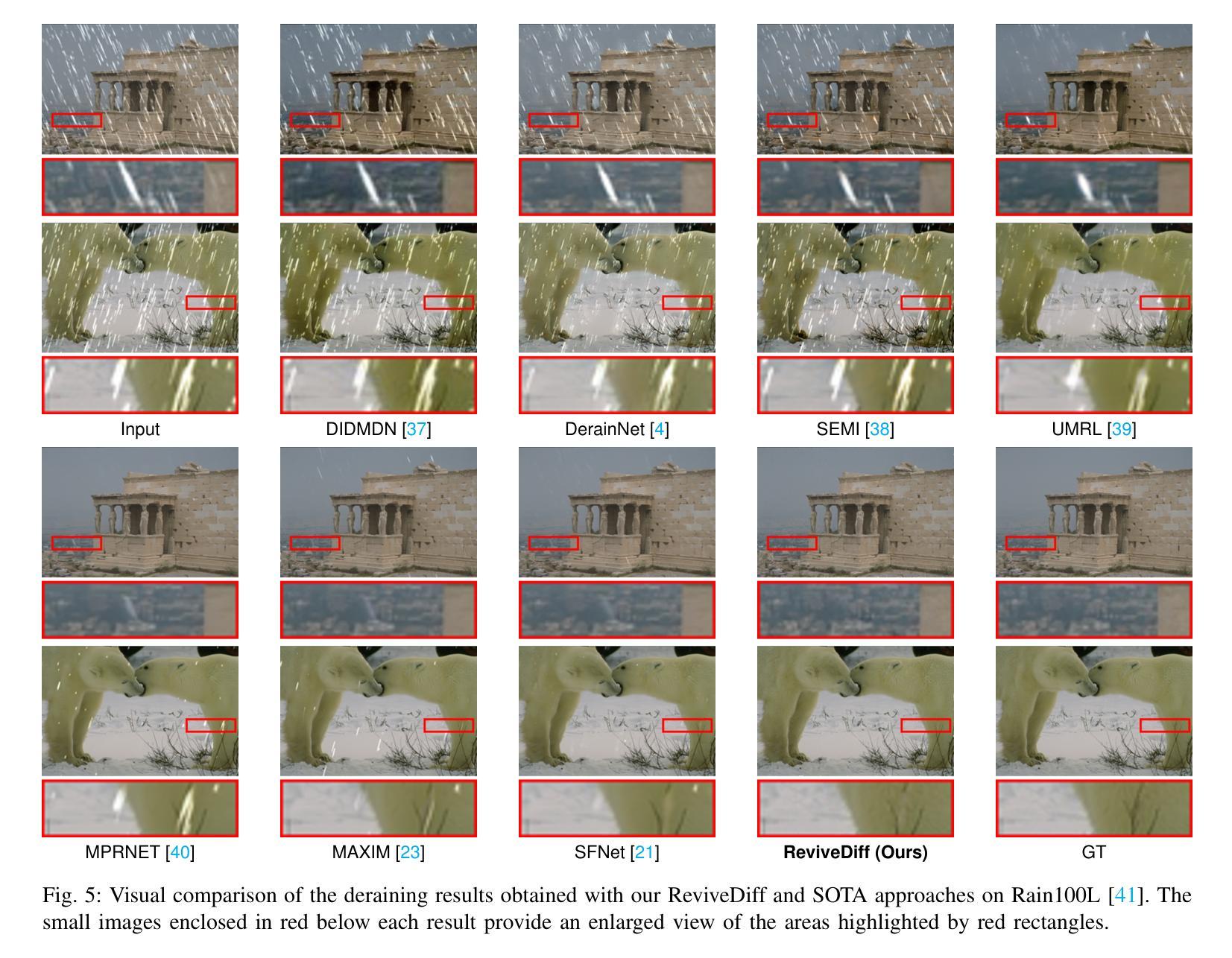