⚠️ 以下所有内容总结都来自于 大语言模型的能力,如有错误,仅供参考,谨慎使用
🔴 请注意:千万不要用于严肃的学术场景,只能用于论文阅读前的初筛!
💗 如果您觉得我们的项目对您有帮助 ChatPaperFree ,还请您给我们一些鼓励!⭐️ HuggingFace免费体验
2025-04-08 更新
OnRL-RAG: Real-Time Personalized Mental Health Dialogue System
Authors:Ahsan Bilal, Beiyu Lin, Mehdi Zaeifi
Large language models (LLMs) have been widely used for various tasks and applications. However, LLMs and fine-tuning are limited to the pre-trained data. For example, ChatGPT’s world knowledge until 2021 can be outdated or inaccurate. To enhance the capabilities of LLMs, Retrieval-Augmented Generation (RAG), is proposed to augment LLMs with additional, new, latest details and information to LLMs. While RAG offers the correct information, it may not best present it, especially to different population groups with personalizations. Reinforcement Learning from Human Feedback (RLHF) adapts to user needs by aligning model responses with human preference through feedback loops. In real-life applications, such as mental health problems, a dynamic and feedback-based model would continuously adapt to new information and offer personalized assistance due to complex factors fluctuating in a daily environment. Thus, we propose an Online Reinforcement Learning-based Retrieval-Augmented Generation (OnRL-RAG) system to detect and personalize the responding systems to mental health problems, such as stress, anxiety, and depression. We use an open-source dataset collected from 2028 College Students with 28 survey questions for each student to demonstrate the performance of our proposed system with the existing systems. Our system achieves superior performance compared to standard RAG and simple LLM via GPT-4o, GPT-4o-mini, Gemini-1.5, and GPT-3.5. This work would open up the possibilities of real-life applications of LLMs for personalized services in the everyday environment. The results will also help researchers in the fields of sociology, psychology, and neuroscience to align their theories more closely with the actual human daily environment.
大型语言模型(LLMs)已广泛应用于各种任务和应用。然而,LLMs和微调都受限于预训练数据。例如,ChatGPT截至2021年的世界知识可能已过时或不准确。为了增强LLMs的功能,提出了检索增强生成(RAG)来向LLMs添加额外、最新、详细的资讯。虽然RAG提供了正确的信息,但它可能无法以最佳方式呈现,特别是对于具有个性化的不同人群。强化学习通过人类反馈(RLHF)适应用户需求,通过反馈循环使模型响应与人类偏好保持一致。在现实生活应用中,例如在心理健康问题方面,一个动态且基于反馈的模型将不断适应新信息并提供个性化援助,因为日常环境中的复杂因素在不断波动。因此,我们提出了基于在线强化学习的检索增强生成(OnRL-RAG)系统,用于检测和个性化应对心理健康问题,如压力、焦虑和抑郁。我们使用从28名大学生收集的开源数据集进行演示,每项评估包含有对每个学生进行的的多个问卷调查问题来验证我们的系统与现有系统的表现。相较于标准的RAG和简单的LLM(如GPT-4o、GPT-4o-mini、Gemini-1.5和GPT-3.5),我们的系统表现卓越。这项工作将为LLMs在个性化服务方面的现实生活应用开辟可能性,特别是在日常环境中。此外,该研究还将有助于社会学、心理学和神经科学领域的研究人员将他们的理论更紧密地与实际的日常人类环境相结合。
论文及项目相关链接
Summary
大语言模型广泛应用于各种任务和应用,但其知识更新受限于预训练数据。为提高大语言模型的能力,提出了基于检索的增强生成技术(RAG)。然而,RAG在呈现信息方面可能存在不足,尤其对于不同人群的个人化需求。因此,结合强化学习人类反馈(RLHF)的技术应运而生,能够根据实际用户需求调整模型响应。针对真实世界应用如心理健康问题,我们提出了基于在线强化学习的检索增强生成系统(OnRL-RAG),用于检测和个性化应对压力、焦虑和抑郁等精神健康问题。使用从大学生收集的开源数据集进行演示,该系统相较于其他现有系统表现出卓越性能。此研究开启了语言模型在个性化服务中的实际应用可能性,并有助于社会学、心理学和神经科学领域的研究者更好地将理论与实际相结合。
Key Takeaways
- 大语言模型(LLMs)广泛应用于各种任务和应用,但受限于预训练数据的时效性。
- 检索增强生成技术(RAG)旨在提高LLMs的能力,提供最新的详细信息。
- RAG在信息呈现方面可能存在不足,不能满足不同人群的个人化需求。
- 强化学习人类反馈(RLHF)技术能够根据实际用户需求调整模型响应。
- 针对精神健康问题如压力、焦虑和抑郁,提出了基于在线强化学习的检索增强生成系统(OnRL-RAG)。
- OnRL-RAG系统使用开源数据集进行验证,相较于其他系统展现出卓越性能。
点此查看论文截图
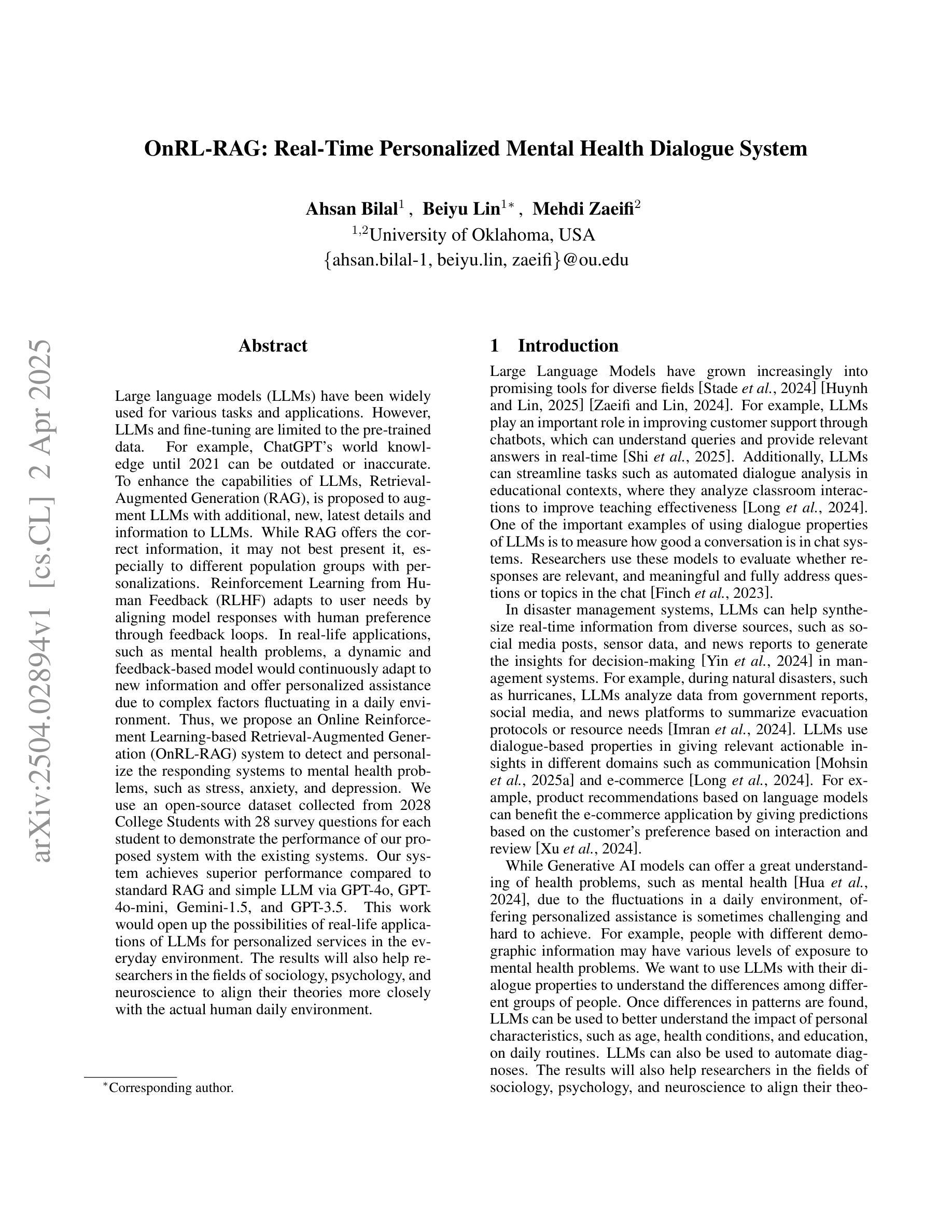
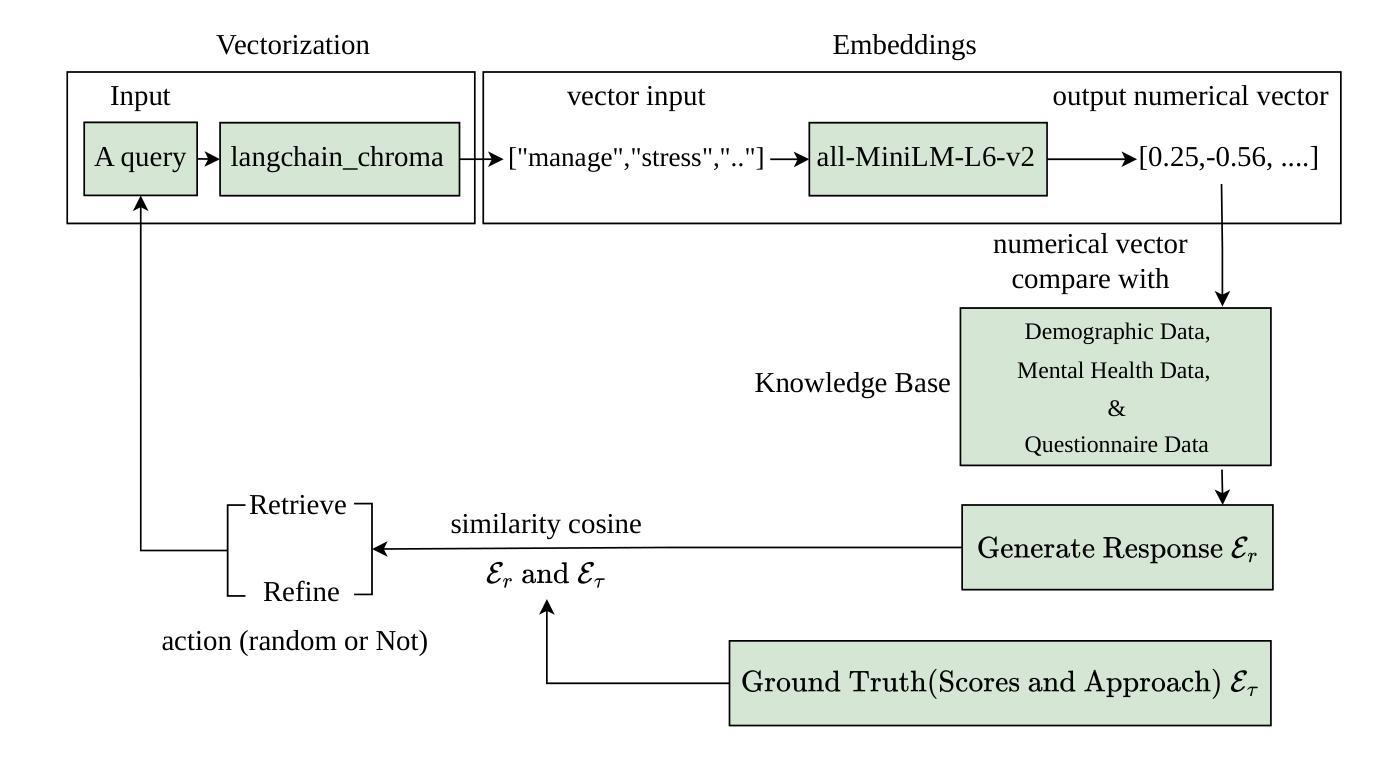
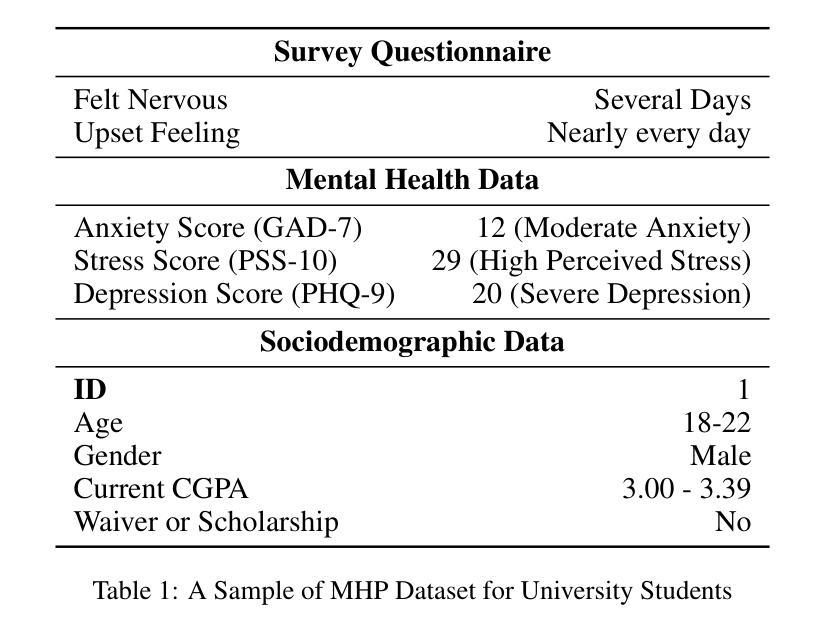