⚠️ 以下所有内容总结都来自于 大语言模型的能力,如有错误,仅供参考,谨慎使用
🔴 请注意:千万不要用于严肃的学术场景,只能用于论文阅读前的初筛!
💗 如果您觉得我们的项目对您有帮助 ChatPaperFree ,还请您给我们一些鼓励!⭐️ HuggingFace免费体验
2025-04-08 更新
Shape My Moves: Text-Driven Shape-Aware Synthesis of Human Motions
Authors:Ting-Hsuan Liao, Yi Zhou, Yu Shen, Chun-Hao Paul Huang, Saayan Mitra, Jia-Bin Huang, Uttaran Bhattacharya
We explore how body shapes influence human motion synthesis, an aspect often overlooked in existing text-to-motion generation methods due to the ease of learning a homogenized, canonical body shape. However, this homogenization can distort the natural correlations between different body shapes and their motion dynamics. Our method addresses this gap by generating body-shape-aware human motions from natural language prompts. We utilize a finite scalar quantization-based variational autoencoder (FSQ-VAE) to quantize motion into discrete tokens and then leverage continuous body shape information to de-quantize these tokens back into continuous, detailed motion. Additionally, we harness the capabilities of a pretrained language model to predict both continuous shape parameters and motion tokens, facilitating the synthesis of text-aligned motions and decoding them into shape-aware motions. We evaluate our method quantitatively and qualitatively, and also conduct a comprehensive perceptual study to demonstrate its efficacy in generating shape-aware motions.
我们探讨了身体形状如何影响人类动作合成。在现有的文本到动作生成方法中,由于学习同质化、规范化的身体形状较为容易,因此往往忽视了身体形状对动作合成的影响这一方面。然而,这种同质化可能会扭曲不同身体形状与其运动动力学之间的自然关联。我们的方法通过从自然语言提示生成感知身体形状的人类运动来弥补这一空白。我们使用基于有限标量量化的变分自编码器(FSQ-VAE)将运动量化成离散标记,然后利用连续的身体形状信息对这些标记进行反量化,以恢复为连续、详细的运动。此外,我们还利用预训练的语言模型来预测连续的形态参数和运动标记,从而促进与文本对齐的动作合成,并将它们解码为感知形态的运。我们进行了定量和定性的评估,并进行了全面的感知研究,以证明其在生成感知形态运动方面的有效性。
论文及项目相关链接
PDF CVPR 2025. Project page: https://shape-move.github.io
Summary
本文探讨了身体形状如何影响人类运动合成。现有文本到运动生成方法往往忽略了身体形状的影响,易于学习同质化的、规范的身体形状,这可能会扭曲不同身体形状与其运动动态之间的自然关联。本研究通过从自然语言提示生成感知身体形状的人类运动来解决这一差距。使用基于有限标量量化的变分自动编码器(FSQ-VAE)将运动量化成离散符号,并利用连续身体形状信息对符号进行去量化,恢复成详细运动。结合预训练的语言模型预测连续形状参数和运动符号,促进文本对齐运动的合成,并将其解码为感知形状的运动。本研究通过定量、定性和感知研究评估了方法的有效性在生成感知形状的运动方面。
Key Takeaways
- 本文强调了身体形状对人类运动合成的影响,指出现有方法忽略了这一点,导致学习到的运动缺乏多样性。
- 提出了一种基于有限标量量化的变分自动编码器(FSQ-VAE)的新方法,能够将运动量化成离散符号。
- 引入连续身体形状信息,对量化后的符号进行去量化,以恢复详细的运动信息。
- 利用预训练的语言模型预测连续形状参数和运动符号,实现了文本与运动的对齐合成。
- 方法能够生成感知身体形状的运动,即运动与身体形状之间的自然关联得到保留。
- 通过定量、定性和感知研究对方法进行了全面评估,证明了其有效性。
- 该方法有望为文本到运动生成领域带来新的研究方向,特别是在考虑身体多样性方面。
点此查看论文截图
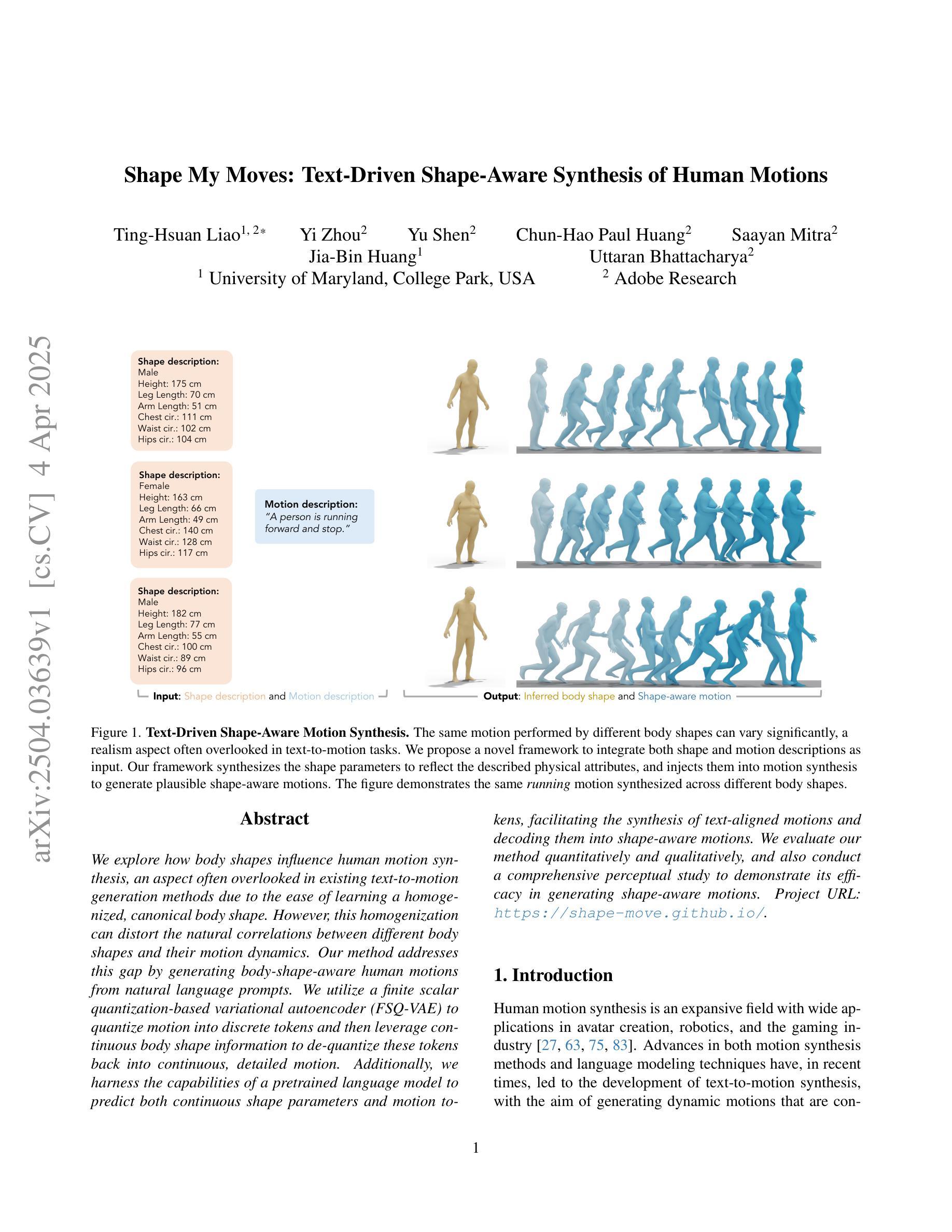
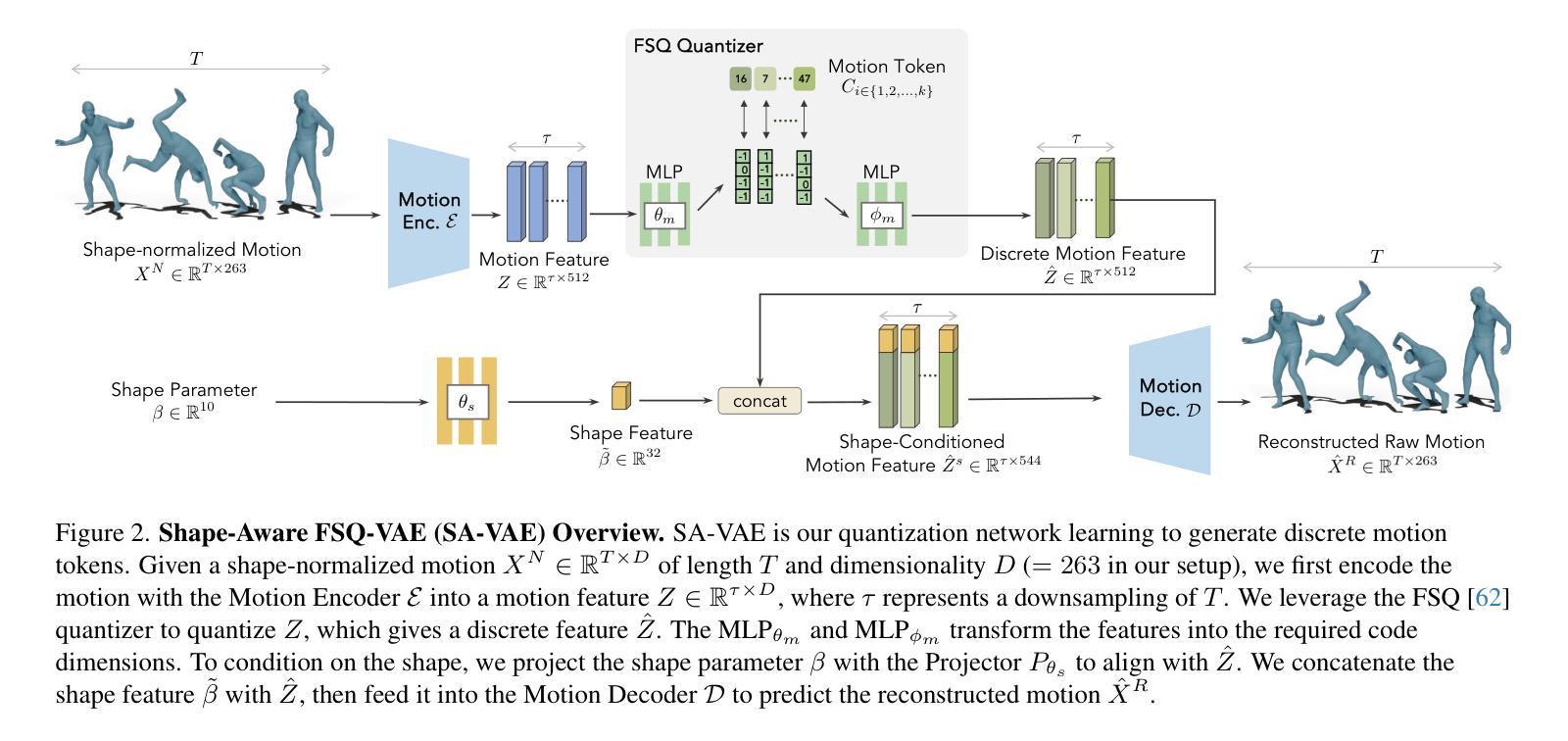
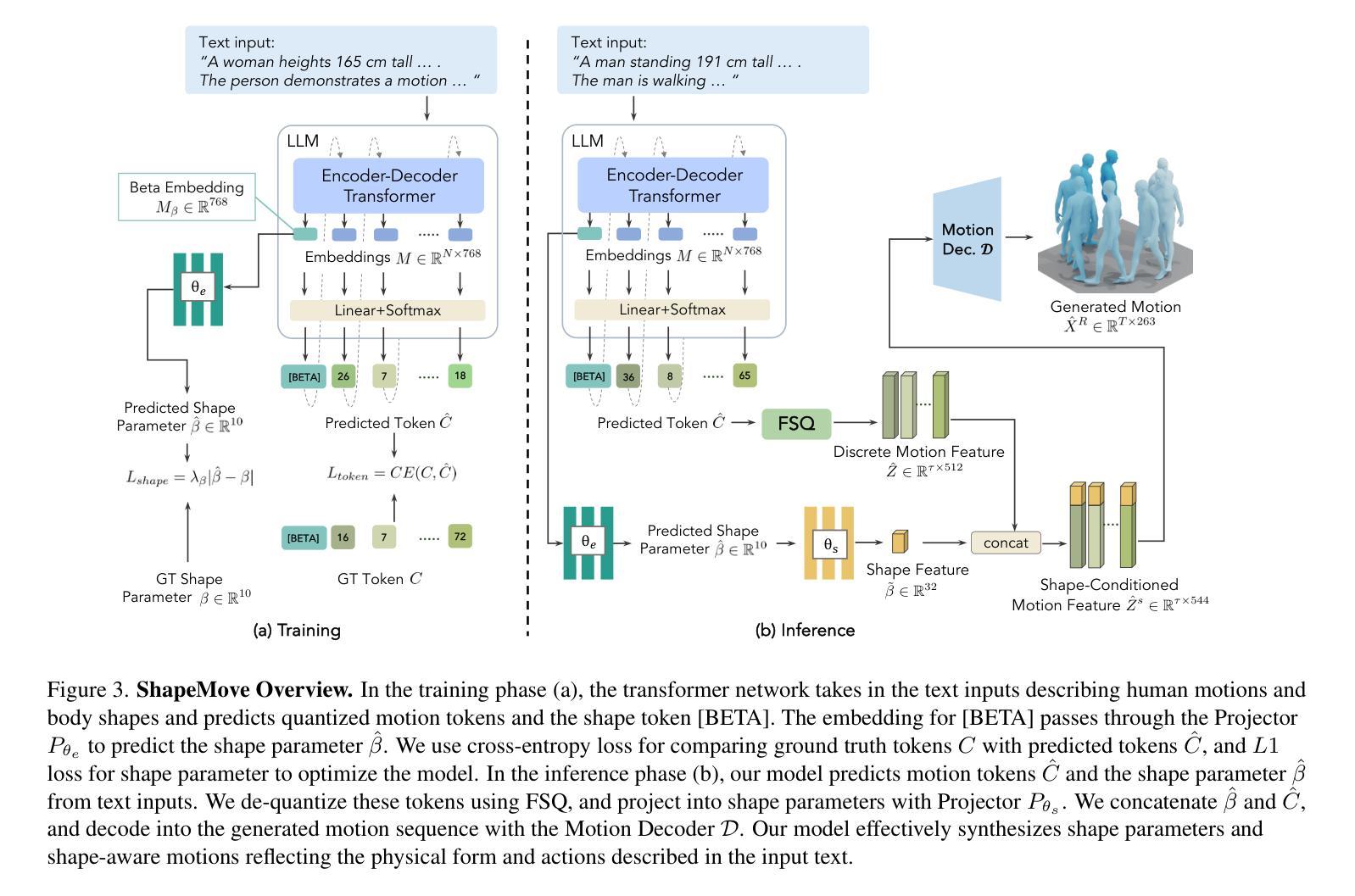
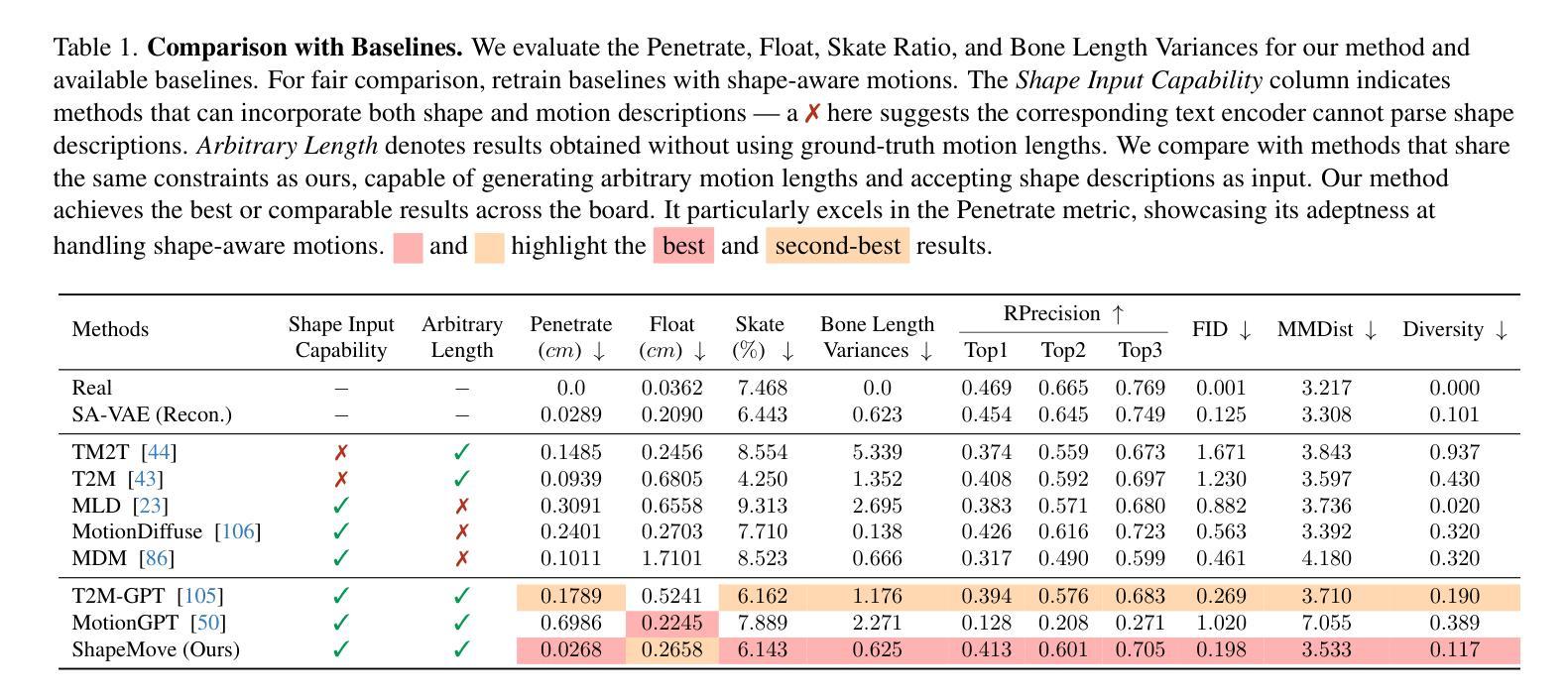
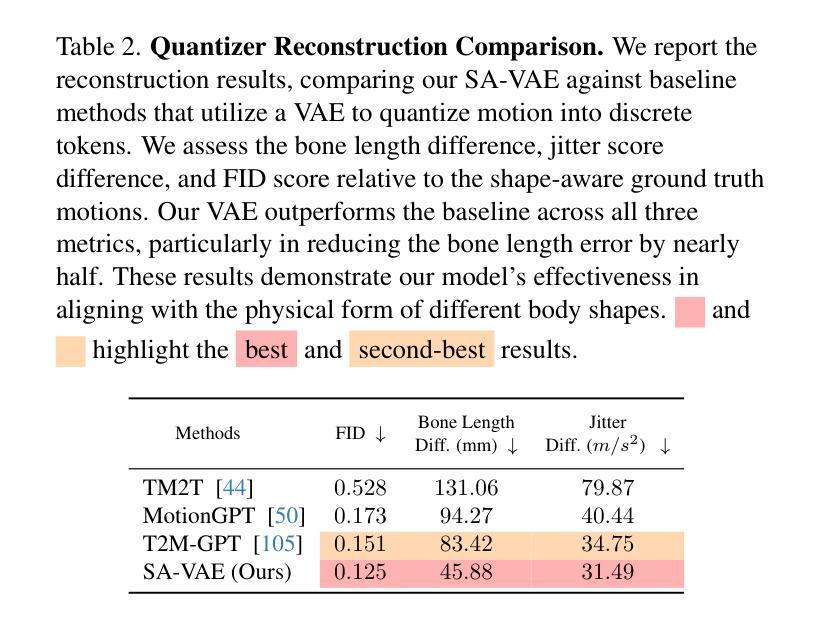