⚠️ 以下所有内容总结都来自于 大语言模型的能力,如有错误,仅供参考,谨慎使用
🔴 请注意:千万不要用于严肃的学术场景,只能用于论文阅读前的初筛!
💗 如果您觉得我们的项目对您有帮助 ChatPaperFree ,还请您给我们一些鼓励!⭐️ HuggingFace免费体验
2025-04-09 更新
Semantic Contextualization of Face Forgery: A New Definition, Dataset, and Detection Method
Authors:Mian Zou, Baosheng Yu, Yibing Zhan, Siwei Lyu, Kede Ma
In recent years, deep learning has greatly streamlined the process of manipulating photographic face images. Aware of the potential dangers, researchers have developed various tools to spot these counterfeits. Yet, none asks the fundamental question: What digital manipulations make a real photographic face image fake, while others do not? In this paper, we put face forgery in a semantic context and define that computational methods that alter semantic face attributes to exceed human discrimination thresholds are sources of face forgery. Following our definition, we construct a large face forgery image dataset, where each image is associated with a set of labels organized in a hierarchical graph. Our dataset enables two new testing protocols to probe the generalizability of face forgery detectors. Moreover, we propose a semantics-oriented face forgery detection method that captures label relations and prioritizes the primary task (i.e., real or fake face detection). We show that the proposed dataset successfully exposes the weaknesses of current detectors as the test set and consistently improves their generalizability as the training set. Additionally, we demonstrate the superiority of our semantics-oriented method over traditional binary and multi-class classification-based detectors.
近年来,深度学习极大地简化了操作摄影面部图像的过程。研究人员意识到潜在的危险,已经开发出各种工具来识别这些伪造品。然而,没有人提出根本问题:什么样的数字操作会使真实的摄影面部图像变得虚假,而其他操作则不会?在本文中,我们将面部伪造置于语义背景下,并定义改变语义面部属性并超过人类辨别阈值的计算方法是面部伪造的来源。根据我们的定义,我们构建了一个大型面部伪造图像数据集,每个图像都与一组标签相关联,并按层次图组织。我们的数据集使两种新的测试协议成为可能,以检验面部伪造检测器的泛化能力。此外,我们提出了一种面向语义的面部伪造检测方法,该方法可以捕获标签关系并优先主要任务(即真实或虚假面部检测)。我们表明,所提出的数据集作为测试集成功暴露了当前检测器的弱点,作为训练集则始终提高了它们的泛化能力。此外,我们证明了与基于传统二元分类和多类分类的检测器相比,我们的面向语义的方法具有优越性。
论文及项目相关链接
Summary
人脸识别技术日益成熟,使得操纵照片中的面部图像变得更为便捷。尽管存在检测伪造人脸的工具,但缺乏从语义角度探讨哪些数字操作会使真实的人脸图像变得不真实的问题。本文首次定义了语义人脸属性超过人类辨别阈值的计算方法是伪造人脸的来源。基于此定义,构建了一个大型人脸伪造图像数据集,并推出两种测试协议以检验人脸伪造检测器的泛化能力。同时提出了一种面向语义的人脸伪造检测方法,该方法能够捕捉标签关系并优先进行真实或伪造人脸检测的主要任务。实验表明,所构建数据集有效地暴露了现有检测器的弱点并提升了其泛化能力。与传统基于二进制和多类分类的检测器相比,本文的语义方法表现出卓越的性能。
Key Takeaways
- 探讨了当前人脸图像处理技术的潜在危险及缺乏从语义角度研究的问题。
- 定义了语义人脸属性超过人类辨别阈值的计算方法是伪造人脸的来源。
- 构建了一个大型人脸伪造图像数据集,附带层次化标签图。
- 提出两种测试协议以评估人脸伪造检测器的泛化能力。
- 提出了一种面向语义的人脸伪造检测方法,可捕捉标签关系并优先执行主要任务。
- 所构建数据集提高了现有检测器的泛化能力并暴露其弱点。
点此查看论文截图
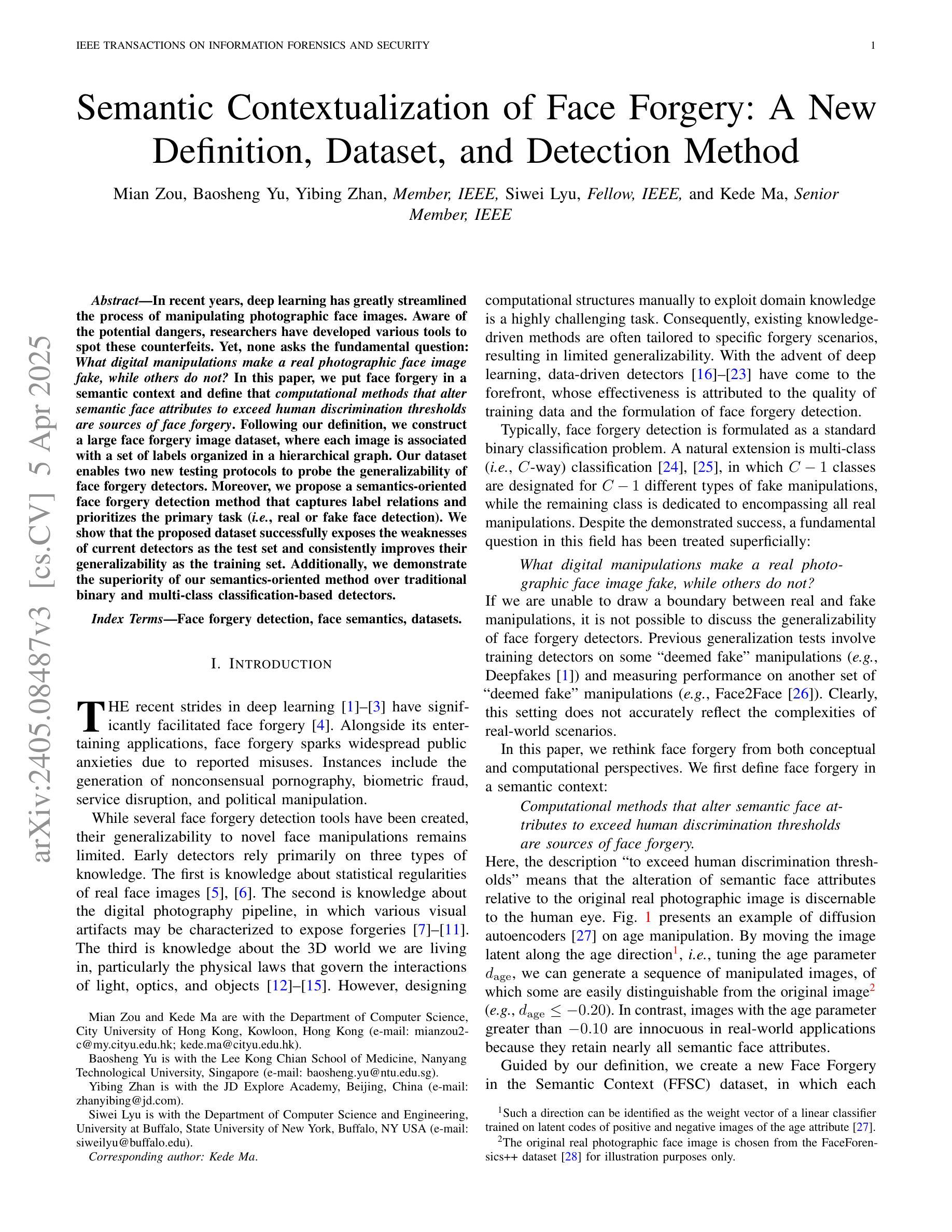
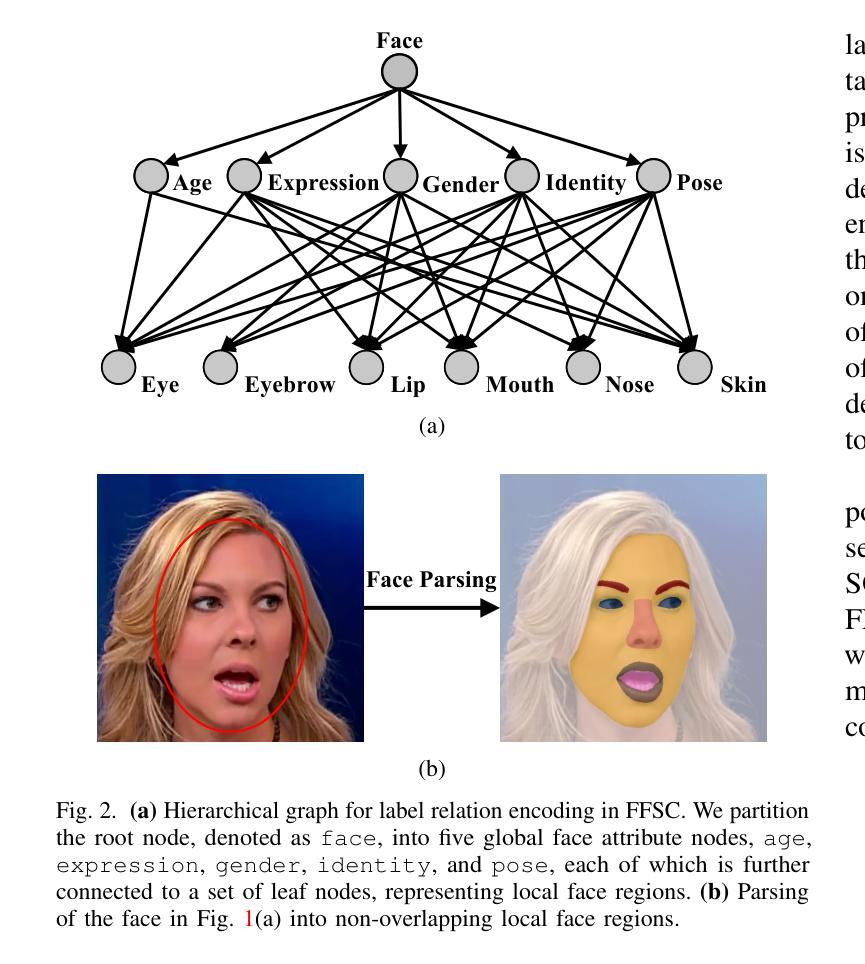
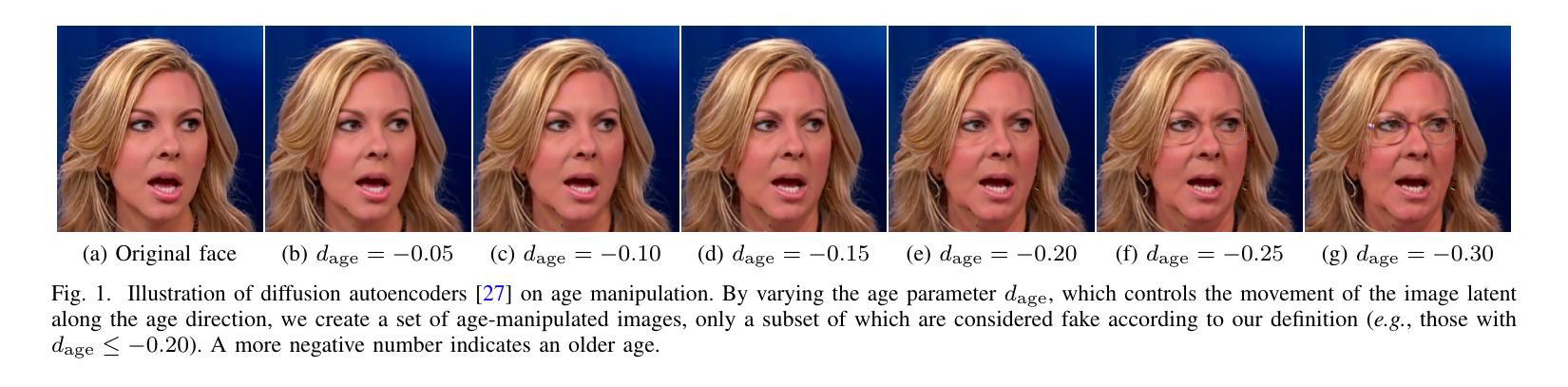
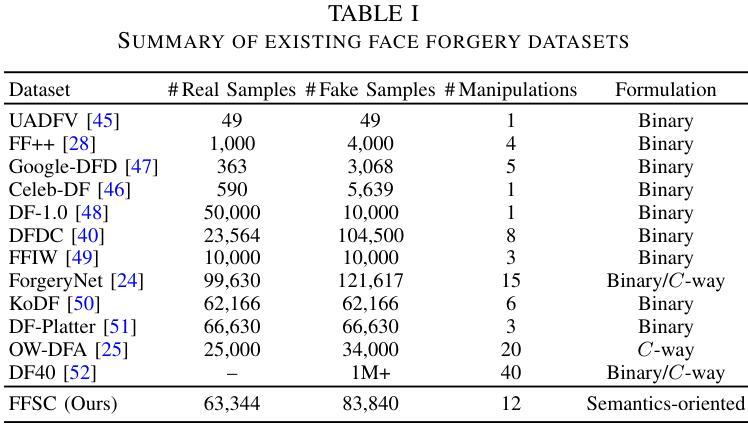
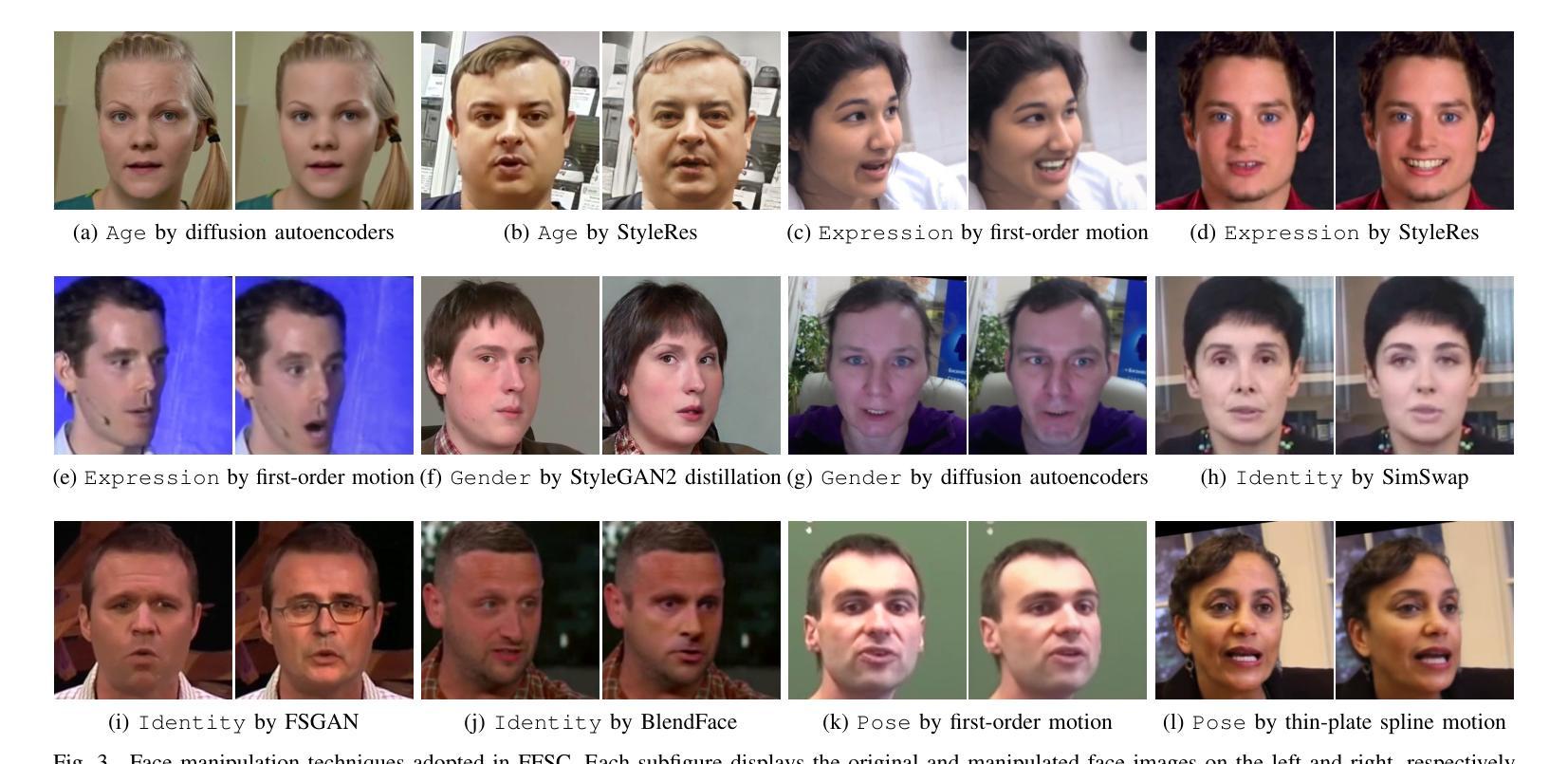
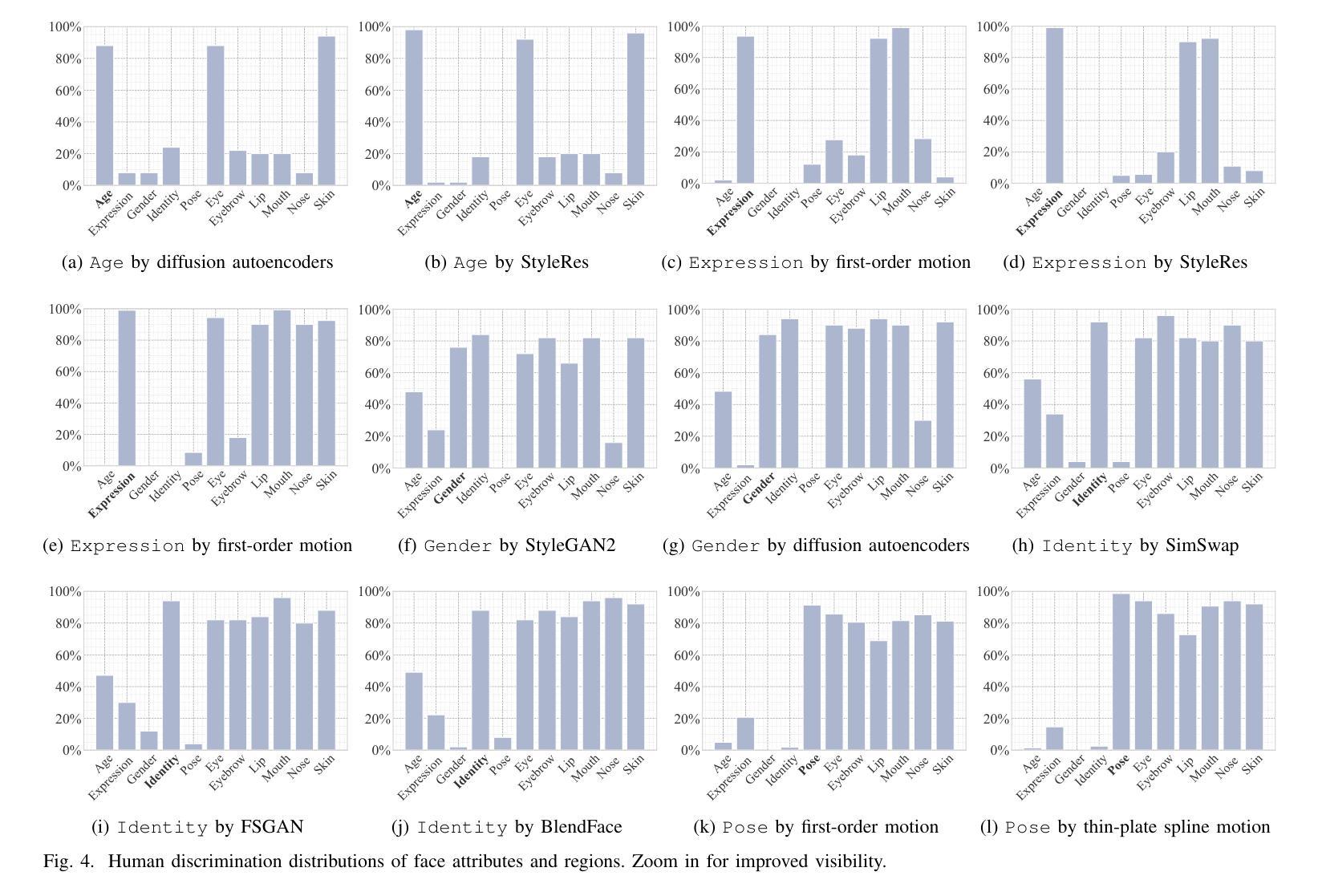