⚠️ 以下所有内容总结都来自于 大语言模型的能力,如有错误,仅供参考,谨慎使用
🔴 请注意:千万不要用于严肃的学术场景,只能用于论文阅读前的初筛!
💗 如果您觉得我们的项目对您有帮助 ChatPaperFree ,还请您给我们一些鼓励!⭐️ HuggingFace免费体验
2025-04-09 更新
InstructionBench: An Instructional Video Understanding Benchmark
Authors:Haiwan Wei, Yitian Yuan, Xiaohan Lan, Wei Ke, Lin Ma
Despite progress in video large language models (Video-LLMs), research on instructional video understanding, crucial for enhancing access to instructional content, remains insufficient. To address this, we introduce InstructionBench, an Instructional video understanding Benchmark, which challenges models’ advanced temporal reasoning within instructional videos characterized by their strict step-by-step flow. Employing GPT-4, we formulate Q&A pairs in open-ended and multiple-choice formats to assess both Coarse-Grained event-level and Fine-Grained object-level reasoning. Our filtering strategies exclude questions answerable purely by common-sense knowledge, focusing on visual perception and analysis when evaluating Video-LLM models. The benchmark finally contains 5k questions across over 700 videos. We evaluate the latest Video-LLMs on our InstructionBench, finding that closed-source models outperform open-source ones. However, even the best model, GPT-4o, achieves only 53.42% accuracy, indicating significant gaps in temporal reasoning. To advance the field, we also develop a comprehensive instructional video dataset with over 19k Q&A pairs from nearly 2.5k videos, using an automated data generation framework, thereby enriching the community’s research resources.
尽管视频大型语言模型(Video-LLMs)领域已经取得了一定的进展,但对于教学视频理解的研究,对于增强教学内容的可访问性至关重要,这方面的研究仍然不足。为了解决这个问题,我们引入了InstructionBench,这是一个教学视频理解基准测试,它挑战了模型在教学视频中的高级时间推理能力,这些视频以严格的逐步流程为特征。我们利用GPT-4,以开放式和多项选择题的形式制定问答对,评估粗粒度事件级和细粒度对象级的推理。我们的过滤策略排除了仅凭常识知识就能回答的问题,在评估Video-LLM模型时侧重于视觉感知和分析。该基准测试最终包含超过700个视频的5000个问题。我们在InstructionBench上评估了最新的Video-LLMs,发现封闭源模型的表现优于开源模型。然而,即使是最好的模型GPT-4o,其准确率也只有53.42%,表明在时间推理方面仍存在巨大差距。为了推动该领域的发展,我们还使用自动化数据生成框架,从近2.5k个视频中构建了包含超过1.9万个问答对的教学视频数据集,从而丰富了社区的研究资源。
论文及项目相关链接
Summary
本文介绍了针对教学视频理解的基准测试——InstructionBench。该基准测试强调教学视频的逐步流程特点,要求模型具备高级时间推理能力。利用GPT-4,形成问答对,以开放题和选择题形式评估粗粒度事件级和细粒度对象级的推理。评估视频大型语言模型时,排除仅依赖常识知识回答的问题,专注于视觉感知和分析。基准测试包含超过700个视频的5000个问题。对最新的视频大型语言模型进行评估,发现封闭源模型表现优于开源模型,但最佳模型GPT-4o准确率仅为53.42%,显示时间推理存在显著差距。为推进该领域发展,使用自动化数据生成框架,从近2.5k视频中开发出包含超过1.9万问答对的综合教学视频数据集。
Key Takeaways
- 教学视频理解研究对于增强教学内容访问至关重要,但仍显不足。
- IntroductionBench基准测试旨在评估模型在教学视频中的时间推理能力。
- GPT-4被用于形成问答对,涵盖粗粒度事件级和细粒度对象级的推理。
- 在评估视频大型语言模型时,重点考察视觉感知和分析能力。
- 基准测试包含大量问题,涵盖超过700个视频。
- 封闭源模型在教学视频理解方面表现优于开源模型。
点此查看论文截图
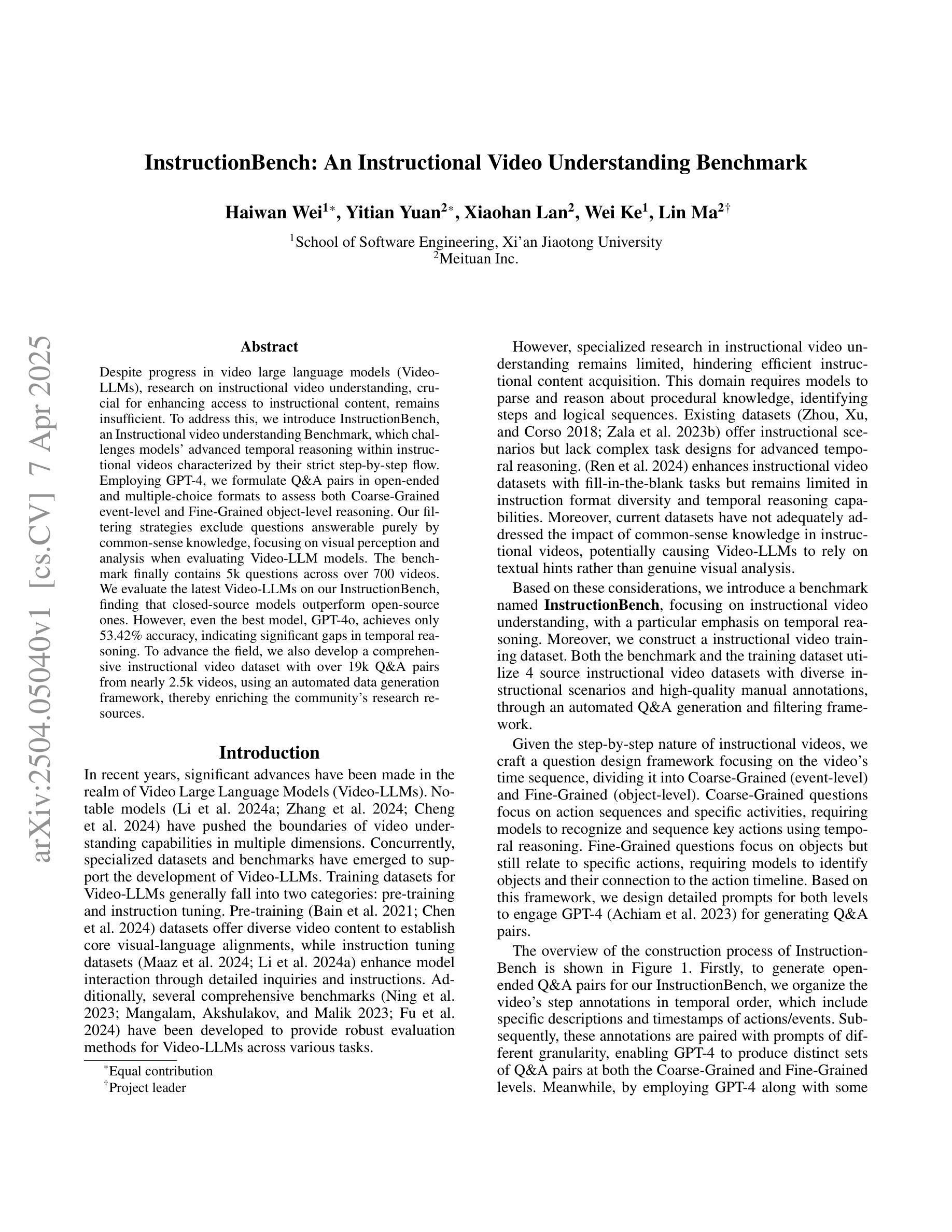
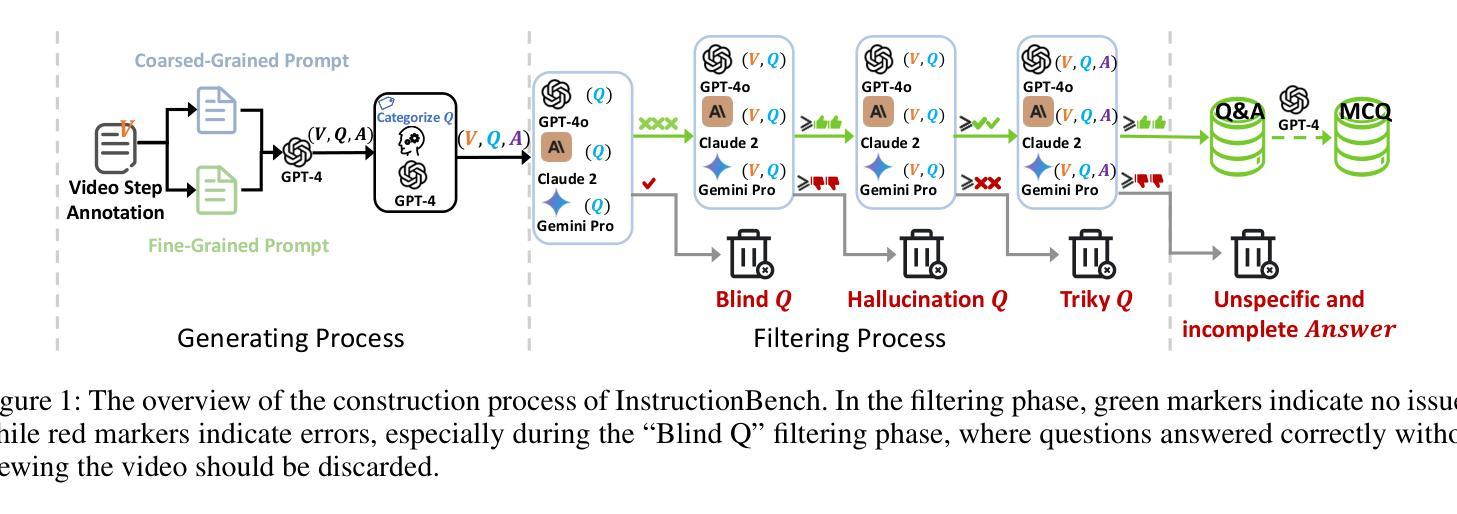
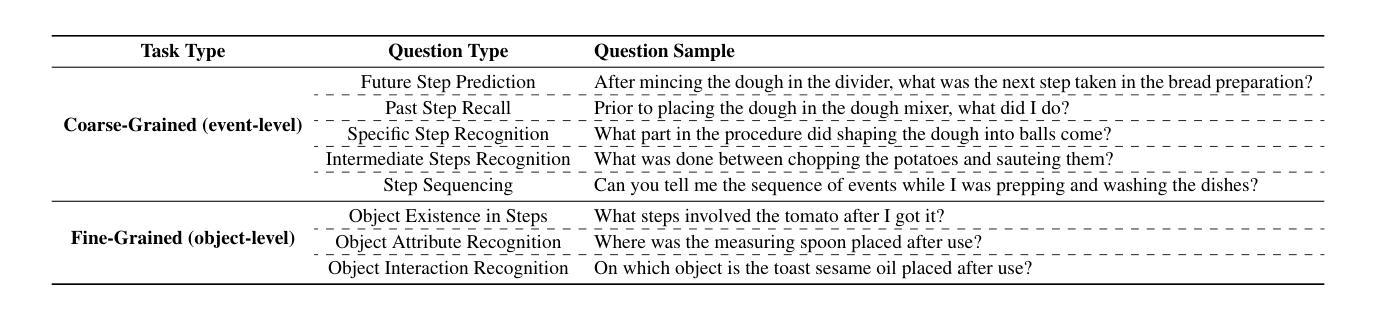
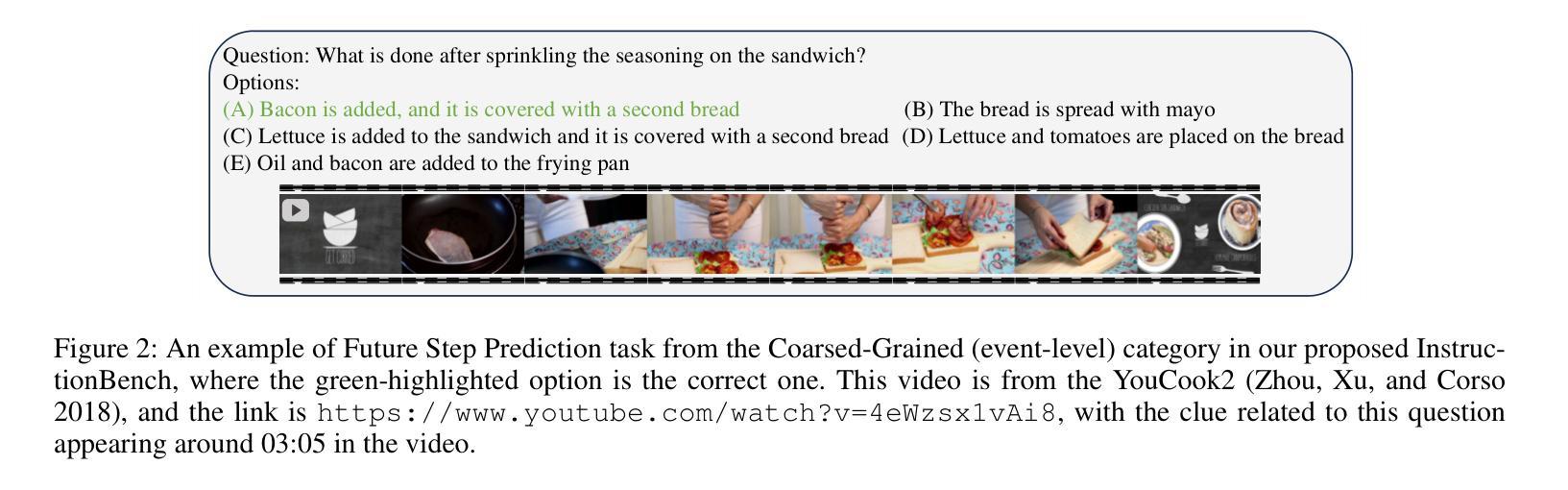
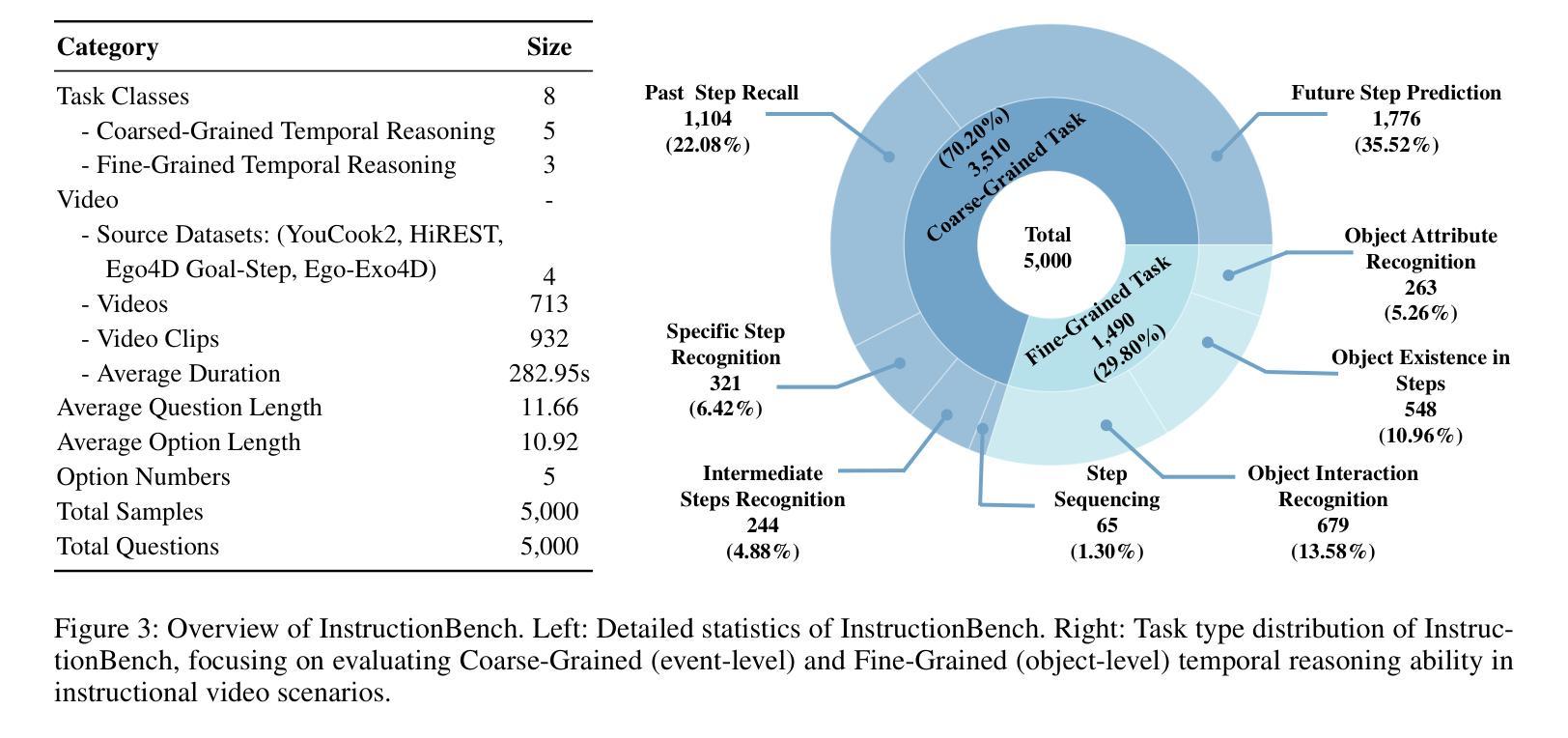
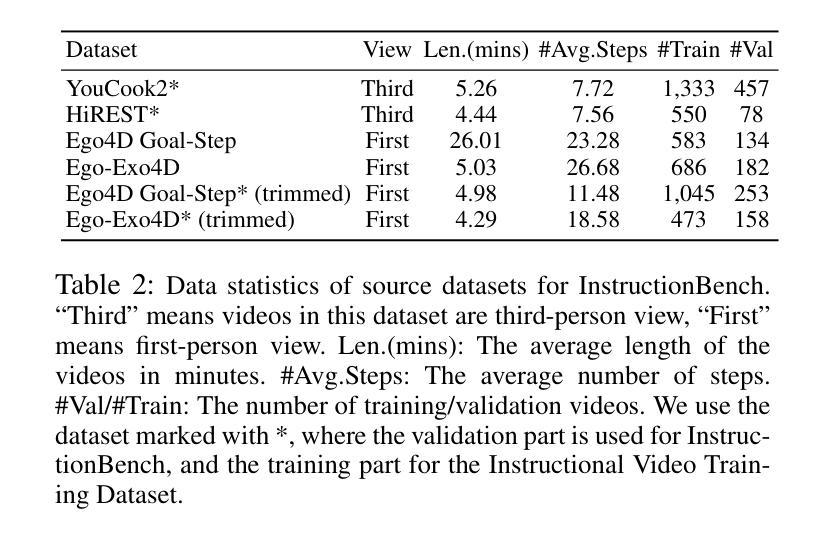
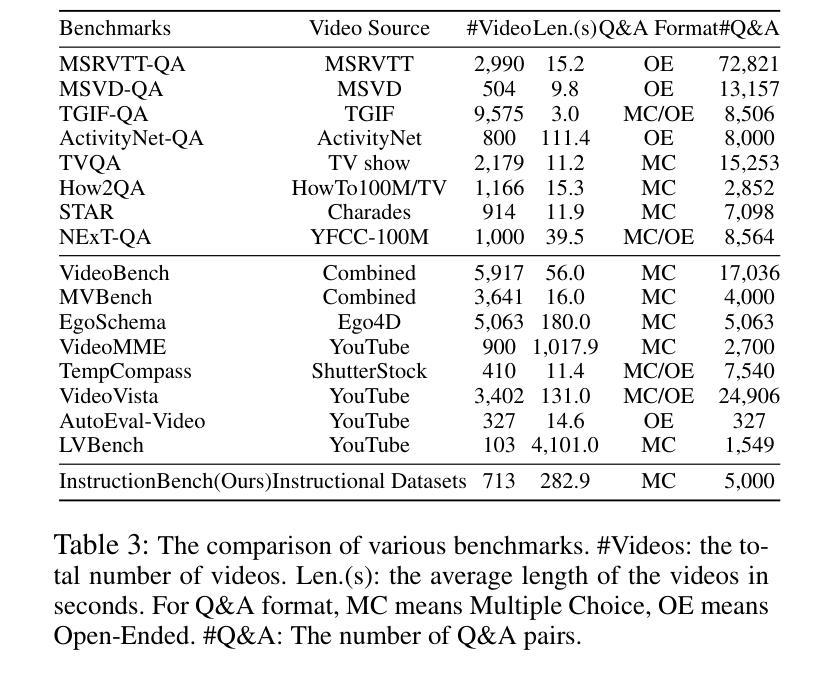
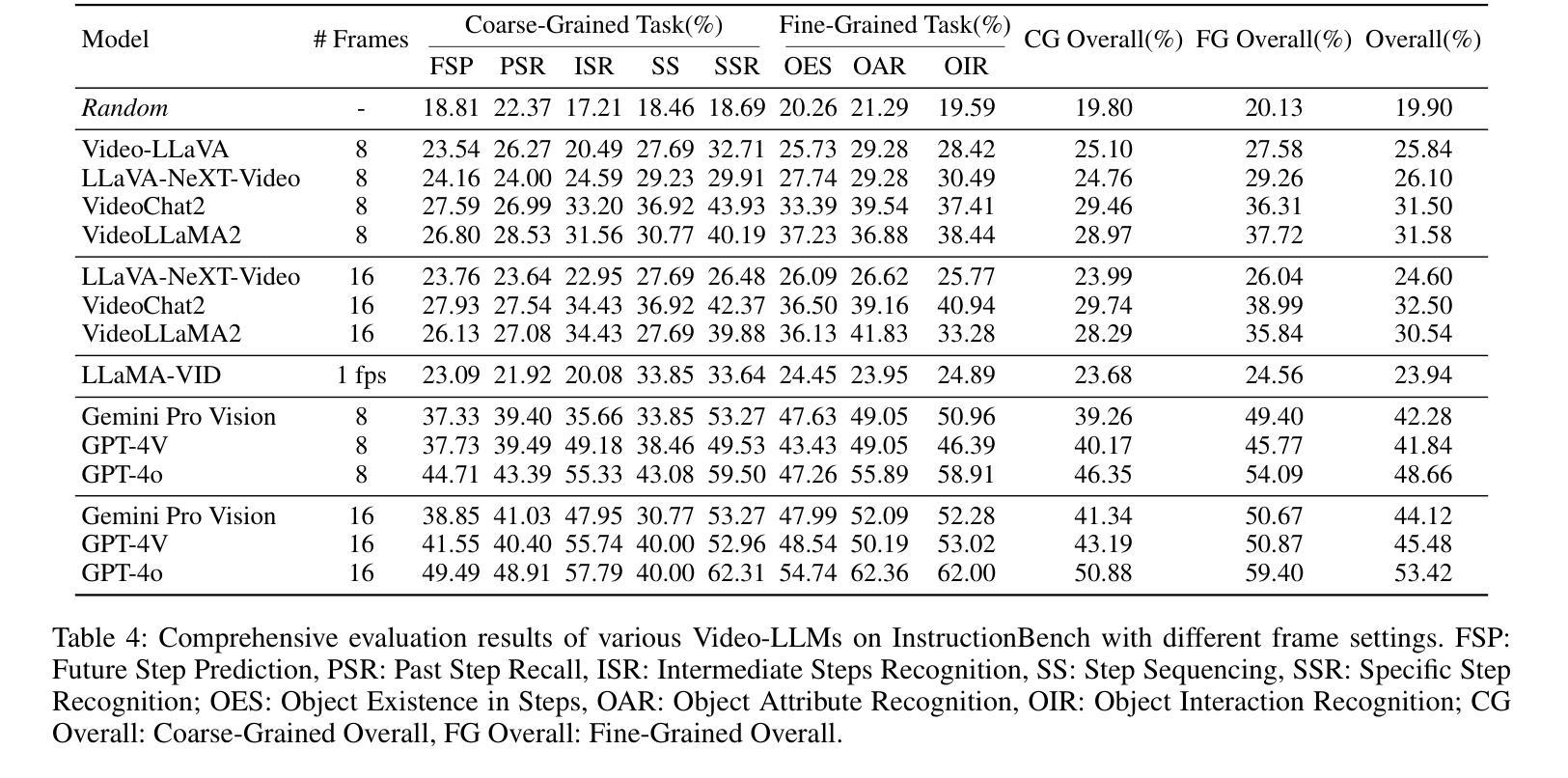
VideoAgent2: Enhancing the LLM-Based Agent System for Long-Form Video Understanding by Uncertainty-Aware CoT
Authors:Zhuo Zhi, Qiangqiang Wu, Minghe shen, Wenbo Li, Yinchuan Li, Kun Shao, Kaiwen Zhou
Long video understanding has emerged as an increasingly important yet challenging task in computer vision. Agent-based approaches are gaining popularity for processing long videos, as they can handle extended sequences and integrate various tools to capture fine-grained information. However, existing methods still face several challenges: (1) they often rely solely on the reasoning ability of large language models (LLMs) without dedicated mechanisms to enhance reasoning in long video scenarios; and (2) they remain vulnerable to errors or noise from external tools. To address these issues, we propose a specialized chain-of-thought (CoT) process tailored for long video analysis. Our proposed CoT with plan-adjust mode enables the LLM to incrementally plan and adapt its information-gathering strategy. We further incorporate heuristic uncertainty estimation of both the LLM and external tools to guide the CoT process. This allows the LLM to assess the reliability of newly collected information, refine its collection strategy, and make more robust decisions when synthesizing final answers. Empirical experiments show that our uncertainty-aware CoT effectively mitigates noise from external tools, leading to more reliable outputs. We implement our approach in a system called VideoAgent2, which also includes additional modules such as general context acquisition and specialized tool design. Evaluation on three dedicated long video benchmarks (and their subsets) demonstrates that VideoAgent2 outperforms the previous state-of-the-art agent-based method, VideoAgent, by an average of 13.1% and achieves leading performance among all zero-shot approaches
长视频理解在计算机视觉领域是一个越来越重要且具有挑战性的任务。基于代理的方法在处理长视频时越来越受欢迎,因为它们可以处理扩展序列并集成各种工具来捕获精细信息。然而,现有方法仍面临一些挑战:(1)它们通常仅依赖大型语言模型的推理能力,而没有专门的机制来增强长视频场景中的推理;(2)它们仍然容易受到外部工具的错误或噪声的影响。为了解决这些问题,我们提出了一种专门针对长视频分析设计的思考链(CoT)过程。我们提出的带有计划调整模式的CoT使LLM能够逐步规划并适应其信息收集策略。我们进一步结合了LLM和外部工具的自启发不确定性估计来引导CoT过程。这使得LLM能够评估新收集信息的可靠性,调整其收集策略,并在合成最终答案时做出更稳健的决策。经验实验表明,我们具有不确定性感知的CoT有效地减轻了来自外部工具的噪声,从而产生了更可靠的输出。我们在名为VideoAgent2的系统中实现了我们的方法,该系统还包括通用上下文获取和专用工具设计等其他模块。在三个专用的长视频基准测试(及其子集)上的评估表明,VideoAgent2比之前的最新代理方法VideoAgent平均高出13.1%,在所有零样本方法中表现领先。
论文及项目相关链接
Summary
针对长视频理解的挑战,提出了一种基于chain-of-thought(CoT)的流程优化方案。结合计划调整模式和不确定性评估机制,增强了大型语言模型在长视频场景中的推理能力,并提高了系统的稳健性。在多个长视频基准测试上,VideoAgent2系统表现出卓越性能,相较于上一代系统有显著提升。
Key Takeaways
1. 长视频理解在计算机视觉中是一项重要且具挑战性的任务。
2. 基于agent的方法处理长视频时能够处理扩展序列并集成各种工具以捕获精细信息。
3. 现有方法依赖大型语言模型的推理能力,但在长视频场景中缺乏专门的增强推理机制。
4. 提出的CoT流程具有计划调整模式,使大型语言模型能够逐步规划并适应其信息收集策略。
5. 结合启发式不确定性评估机制,指导CoT流程,使大型语言模型能够评估新收集信息的可靠性,优化信息收集策略,并做出更稳健的决策。
6. 实证实验表明,不确定性感知的CoT有效减轻了来自外部工具的噪声,产生了更可靠的结果。
点此查看论文截图
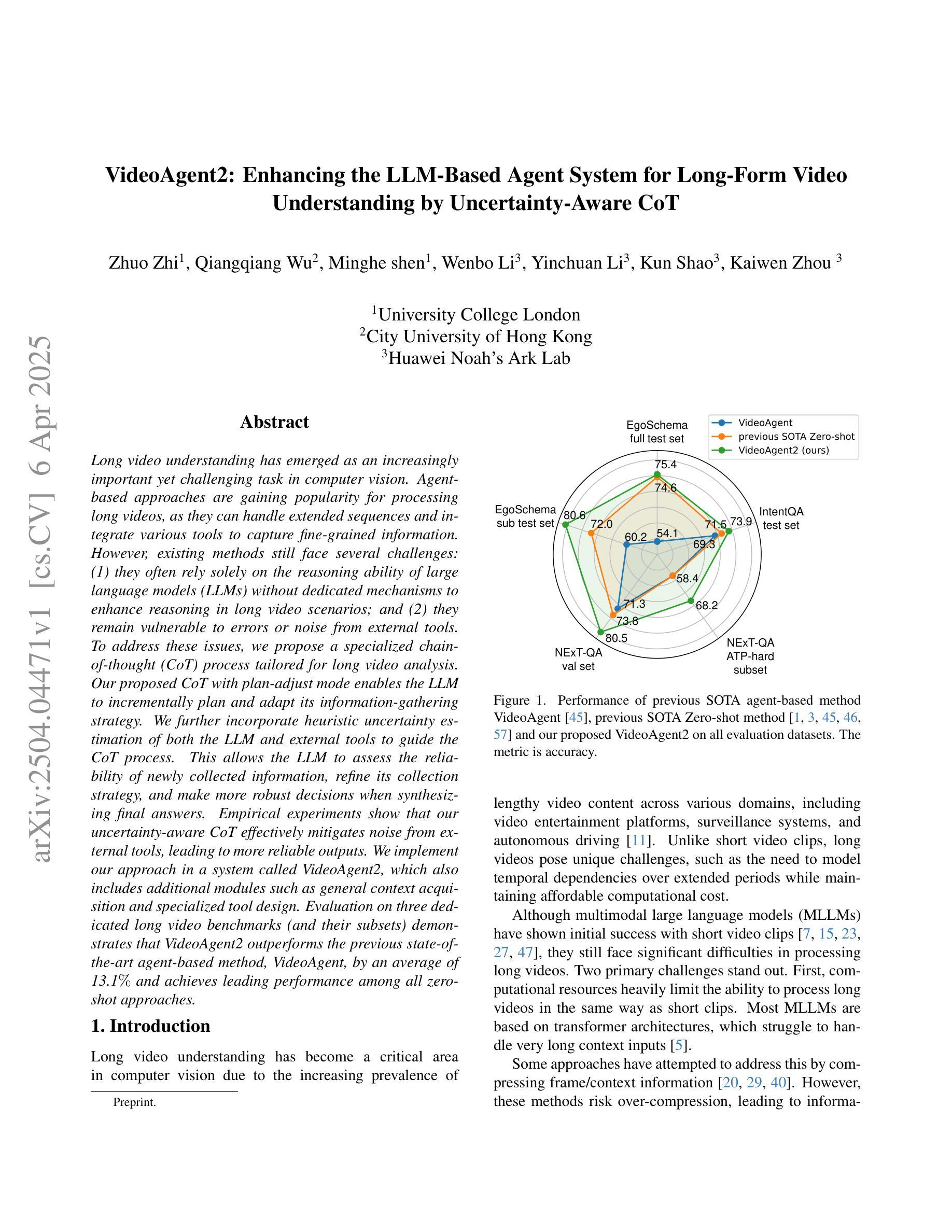
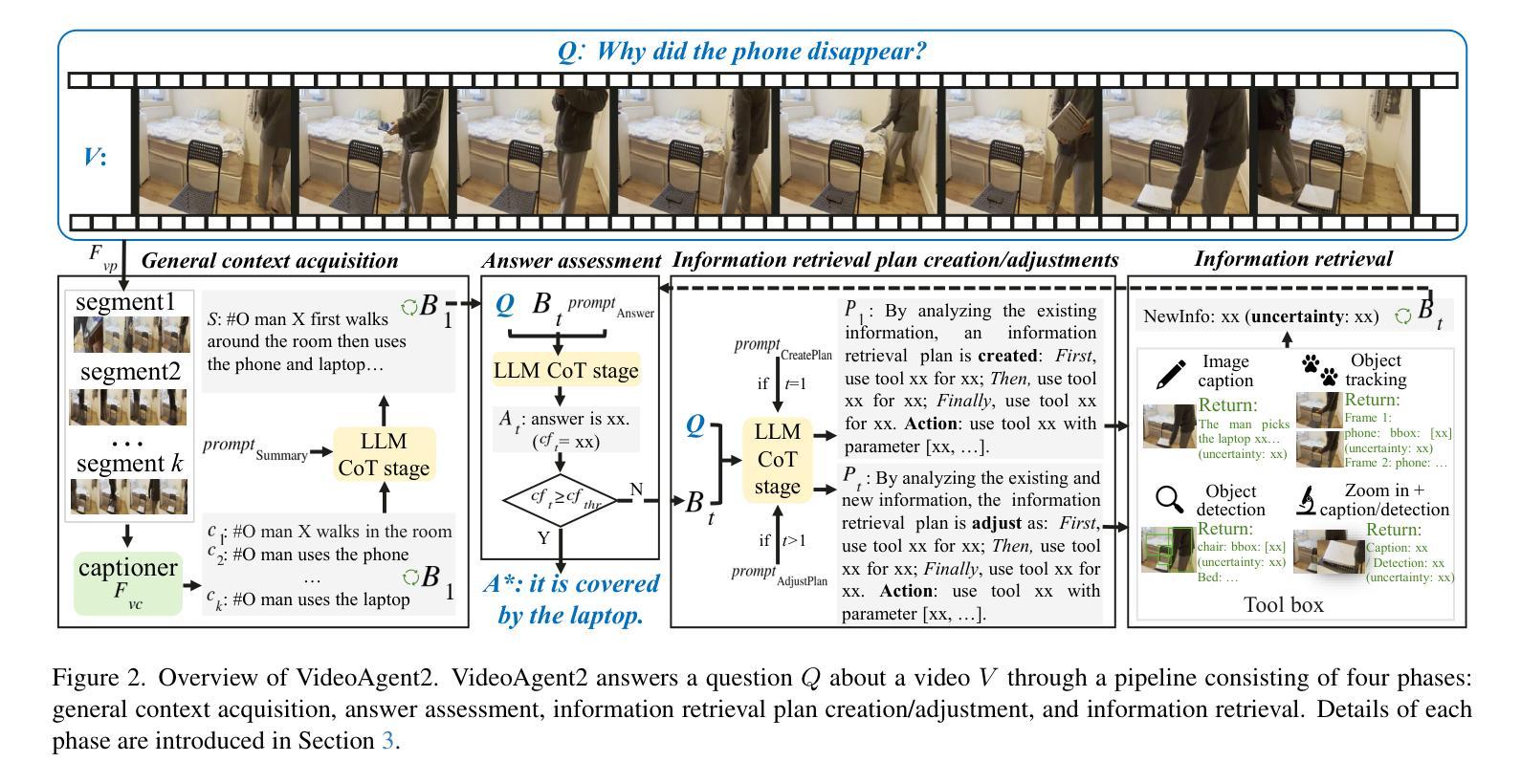
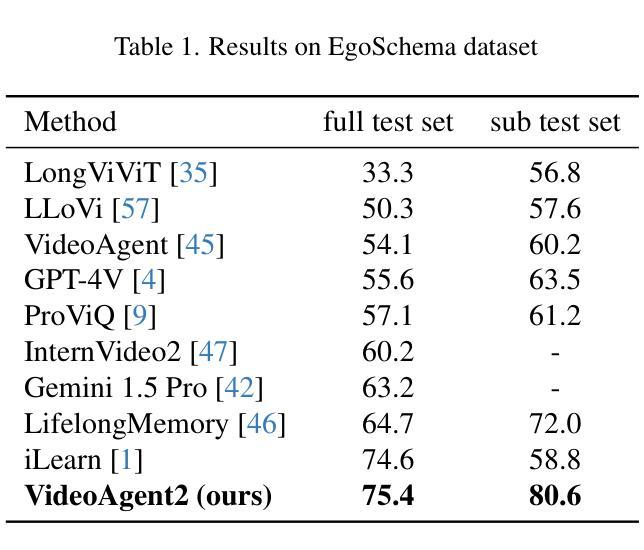
Re-thinking Temporal Search for Long-Form Video Understanding
Authors:Jinhui Ye, Zihan Wang, Haosen Sun, Keshigeyan Chandrasegaran, Zane Durante, Cristobal Eyzaguirre, Yonatan Bisk, Juan Carlos Niebles, Ehsan Adeli, Li Fei-Fei, Jiajun Wu, Manling Li
Efficiently understanding long-form videos remains a significant challenge in computer vision. In this work, we revisit temporal search paradigms for long-form video understanding and address a fundamental issue pertaining to all state-of-the-art (SOTA) long-context vision-language models (VLMs). Our contributions are twofold: First, we frame temporal search as a Long Video Haystack problem: finding a minimal set of relevant frames (e.g., one to five) from tens of thousands based on specific queries. Upon this formulation, we introduce LV-Haystack, the first dataset with 480 hours of videos, 15,092 human-annotated instances for both training and evaluation aiming to improve temporal search quality and efficiency. Results on LV-Haystack highlight a significant research gap in temporal search capabilities, with current SOTA search methods only achieving 2.1% temporal F1 score on the Longvideobench subset. Next, inspired by visual search in images, we propose a lightweight temporal search framework, T* that reframes costly temporal search as spatial search. T* leverages powerful visual localization techniques commonly used in images and introduces an adaptive zooming-in mechanism that operates across both temporal and spatial dimensions. Extensive experiments show that integrating T* with existing methods significantly improves SOTA long-form video understanding. Under an inference budget of 32 frames, T* improves GPT-4o’s performance from 50.5% to 53.1% and LLaVA-OneVision-OV-72B’s performance from 56.5% to 62.4% on the Longvideobench XL subset. Our code, benchmark, and models are provided in the Supplementary material.
对于计算机视觉而言,高效理解长视频仍然是一个巨大的挑战。在这项工作中,我们重新审视了长视频理解的时序搜索范式,并针对所有先进的(SOTA)长上下文视觉语言模型(VLMs)解决了一个基本问题。我们的贡献有两方面:首先,我们将时序搜索构建为长视频堆检索问题:从数万个相关帧中找出少量(例如一到五个)与特定查询相关的帧。基于此构想,我们推出了LV-Haystack数据集,该数据集包含480小时的视频和针对训练和评估的15,092个人工标注实例,旨在提高时序搜索的质量和效率。在LV-Haystack上的结果显示了时序搜索能力存在显著的研究差距,当前最先进的搜索方法仅在Longvideobench子集上实现了2.1%的时间F1分数。接下来,受图像视觉搜索的启发,我们提出了一种轻量级的时序搜索框架T,它将昂贵的时序搜索重新定位为空间搜索。T利用图像中常用的强大视觉定位技术,并引入了一种自适应缩放机制,该机制在时间和空间维度上运行。大量实验表明,将T与现有方法相结合,可以显著提高SOTA长视频理解的效果。在推理预算为32帧的条件下,T将GPT-4o的性能从50.5%提高到53.1%,将LLaVA-OneVision-OV-72B的性能从56.5%提高到62.4%,这是在Longvideobench XL子集上的结果。我们的代码、基准测试和模型都已在补充材料提供。
论文及项目相关链接
PDF Accepted by CVPR 2025; A real-world long video needle-in-haystack benchmark; long-video QA with human ref frames
摘要
本文重新审视了长视频理解的时序搜索范式,并针对所有最先进的长期上下文视觉语言模型(VLMs)的一个根本问题进行了深入研究。文章贡献主要体现在两个方面:首先,将时序搜索问题比作从海量的长视频中筛选出一小部分关键帧的问题(例如从数十万帧中只选择一到五帧),并据此推出了LV-Haystack数据集,包含480小时的视频和用于训练和评估的15,092个人工标注实例。研究结果表明,当前最先进的搜索方法仅在Longvideobench子集上实现2.1%的时间F1得分,存在显著的研究差距。其次,文章提出了一个轻量级的时序搜索框架T,将昂贵的时序搜索重新定位为空间搜索。T利用图像中常用的视觉定位技术,并引入自适应缩放机制,在时间和空域维度上运行。实验表明,将T与现有方法相结合,可显著提高当前最先进的长期视频理解效果。在推理预算为32帧的条件下,T将GPT-4o的性能从50.5%提高到53.1%,LLaVA-OneVision-OV-72B的性能从56.5%提高到62.4%。
关键见解
- 长视频理解中的时序搜索仍然存在显著挑战,尤其是从海量的视频中筛选出关键帧的问题。
- LV-Haystack数据集的推出为改善时序搜索质量和效率提供了重要资源。
- 当前最先进的搜索方法在Longvideobench子集上的表现不佳,仅为2.1%的时间F1得分,表明存在巨大的研究空间。
- 提出的轻量级时序搜索框架T*,将复杂的时序搜索问题简化为空间搜索问题。
- T*利用视觉定位技术和自适应缩放机制,在时间和空域上操作,提高了搜索效率。
- 结合T*,现有方法的性能得到显著提高,特别是在推理预算有限的情况下。
- 文章提供了代码、基准测试和模型,为未来的研究提供了基础。
点此查看论文截图
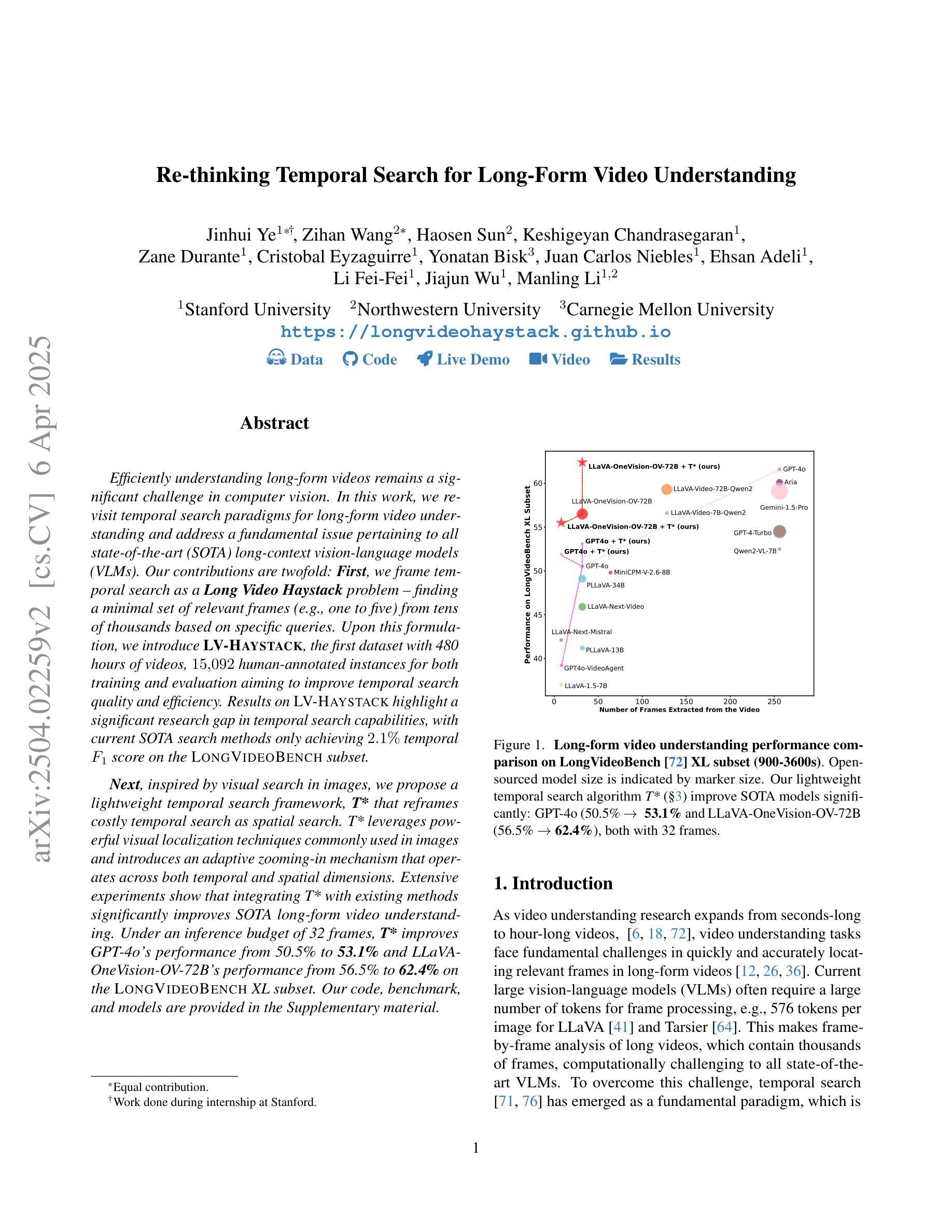
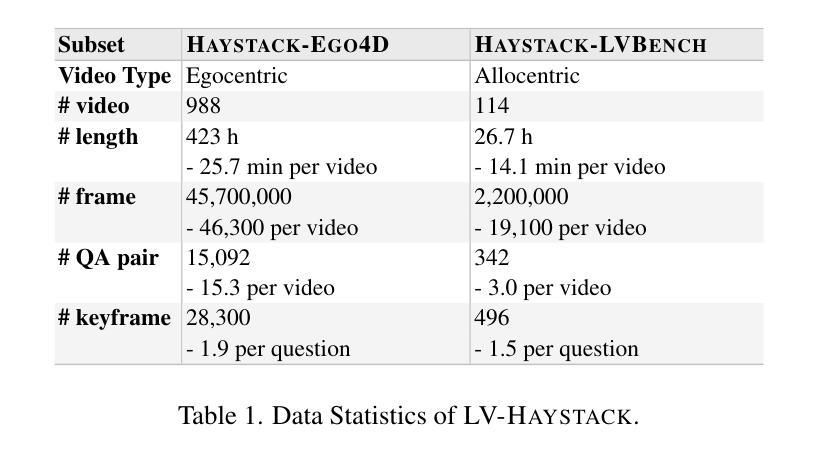
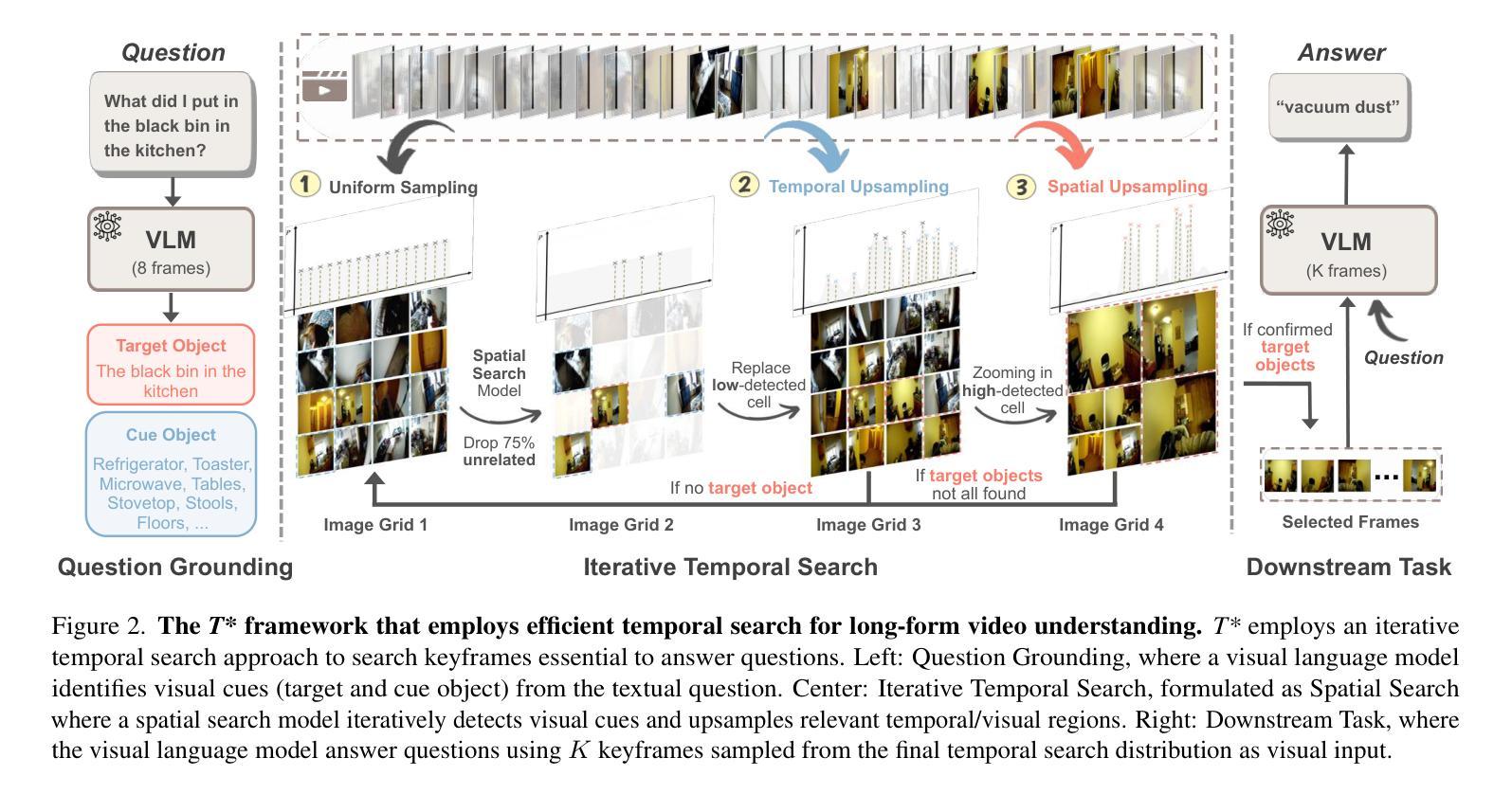
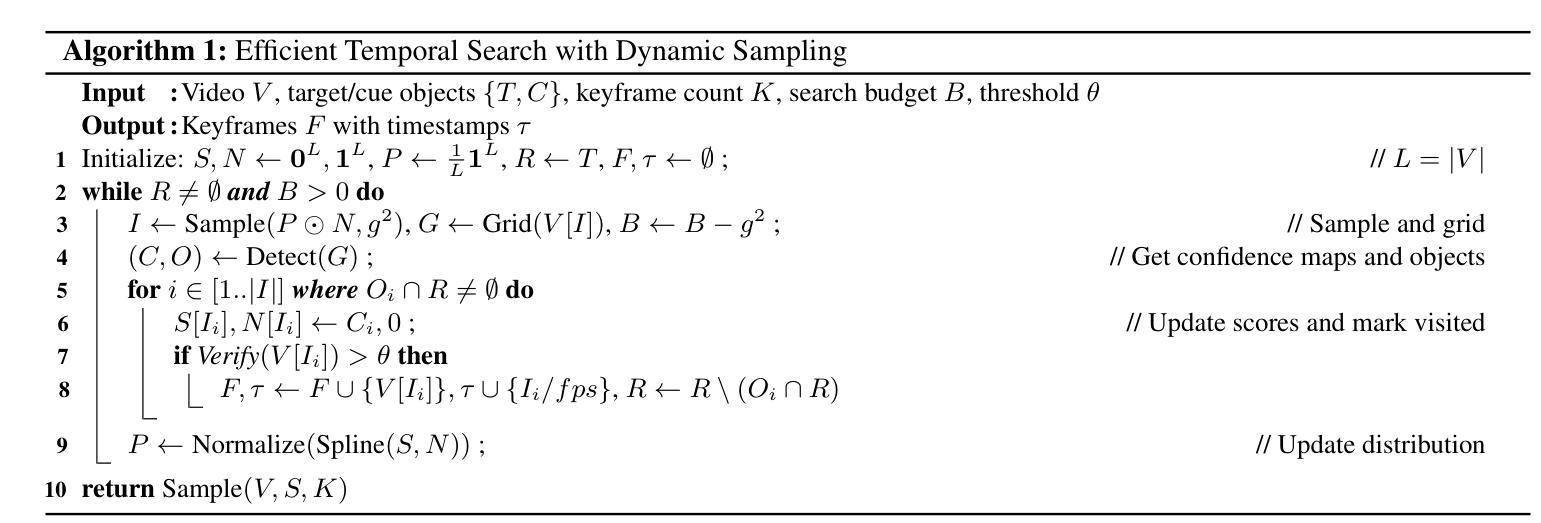
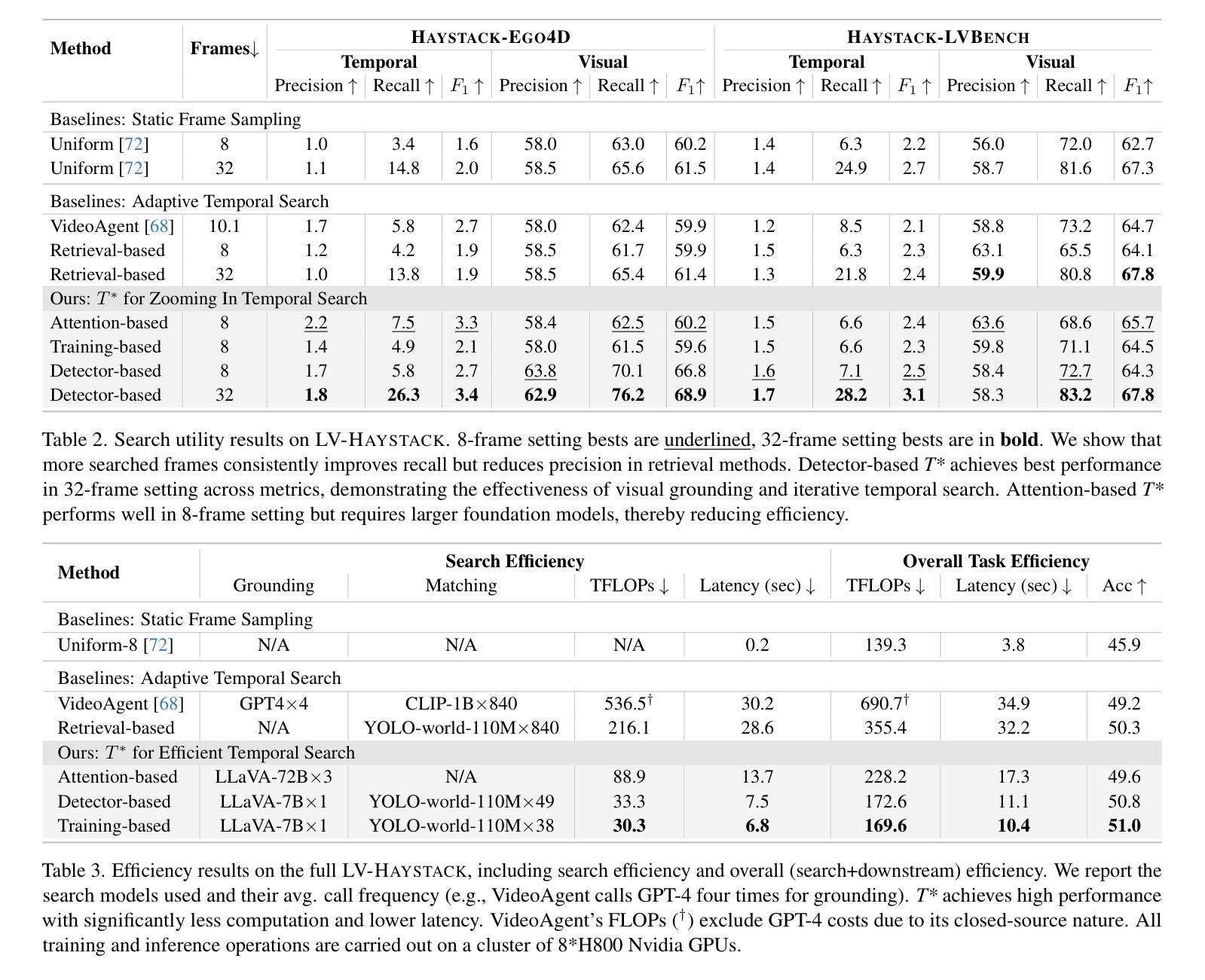
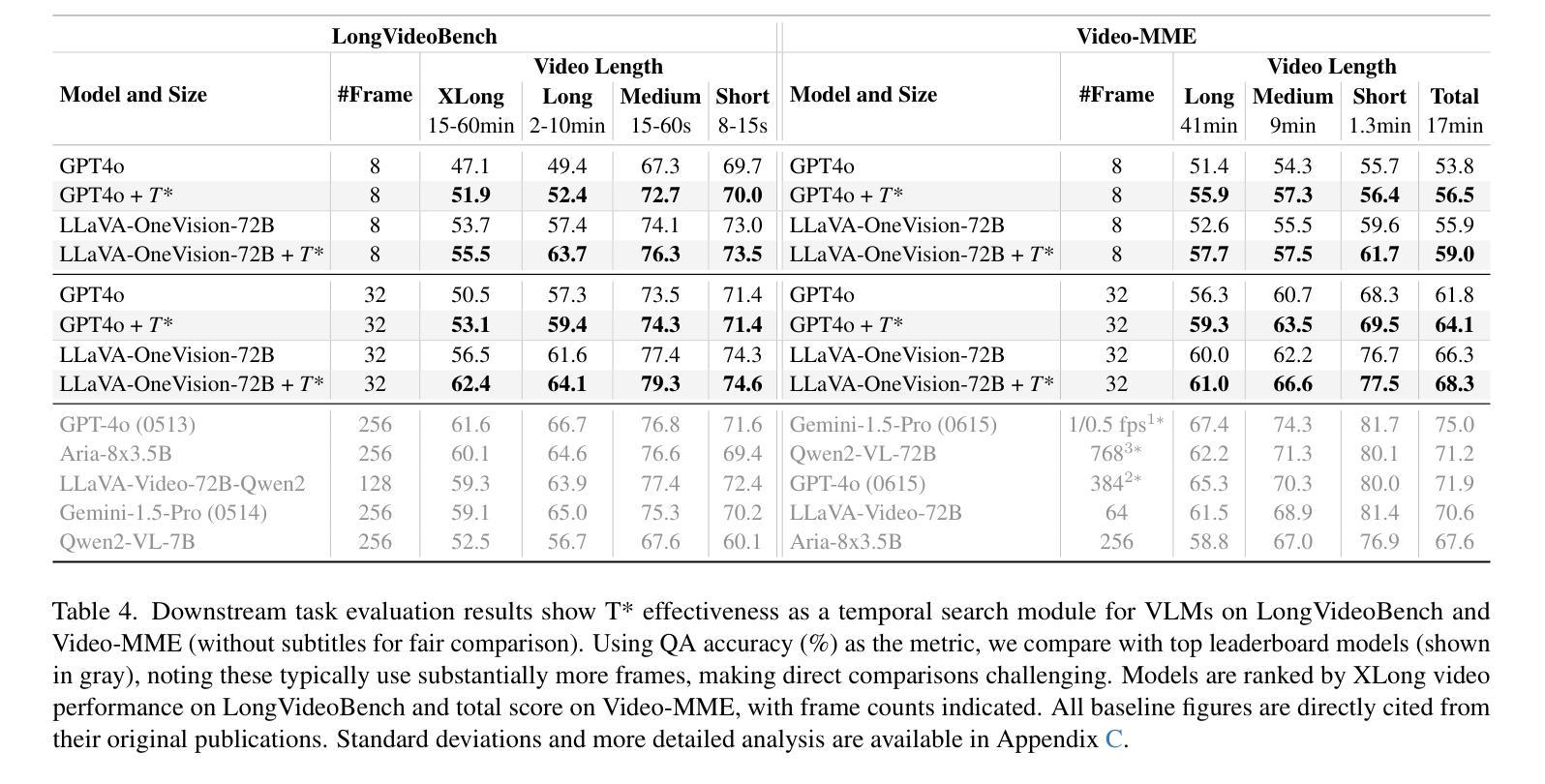
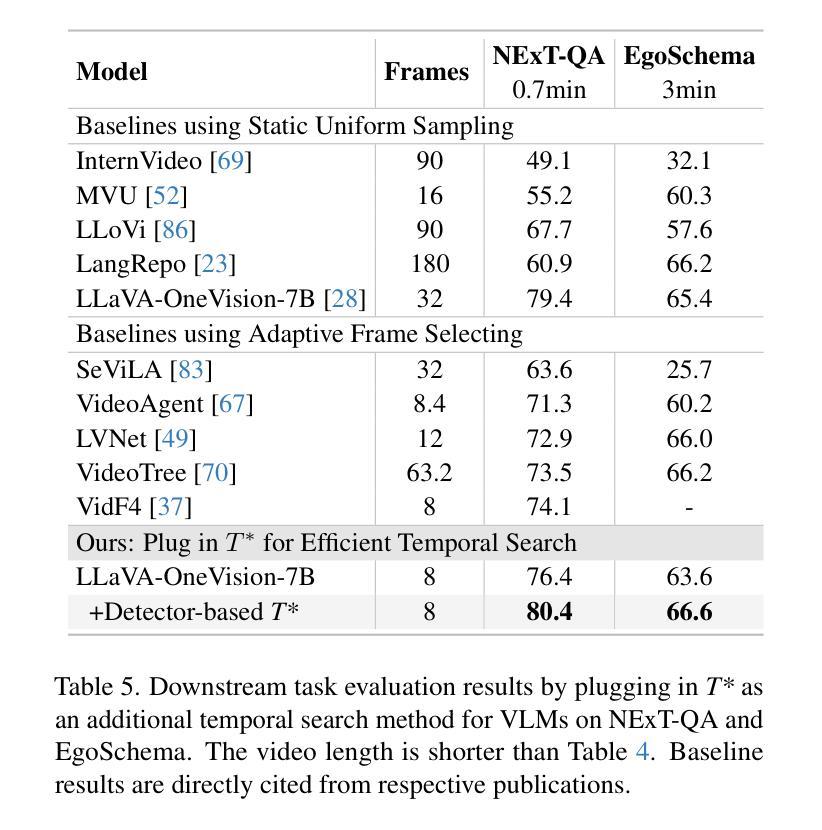