⚠️ 以下所有内容总结都来自于 大语言模型的能力,如有错误,仅供参考,谨慎使用
🔴 请注意:千万不要用于严肃的学术场景,只能用于论文阅读前的初筛!
💗 如果您觉得我们的项目对您有帮助 ChatPaperFree ,还请您给我们一些鼓励!⭐️ HuggingFace免费体验
2025-04-09 更新
FluentLip: A Phonemes-Based Two-stage Approach for Audio-Driven Lip Synthesis with Optical Flow Consistency
Authors:Shiyan Liu, Rui Qu, Yan Jin
Generating consecutive images of lip movements that align with a given speech in audio-driven lip synthesis is a challenging task. While previous studies have made strides in synchronization and visual quality, lip intelligibility and video fluency remain persistent challenges. This work proposes FluentLip, a two-stage approach for audio-driven lip synthesis, incorporating three featured strategies. To improve lip synchronization and intelligibility, we integrate a phoneme extractor and encoder to generate a fusion of audio and phoneme information for multimodal learning. Additionally, we employ optical flow consistency loss to ensure natural transitions between image frames. Furthermore, we incorporate a diffusion chain during the training of Generative Adversarial Networks (GANs) to improve both stability and efficiency. We evaluate our proposed FluentLip through extensive experiments, comparing it with five state-of-the-art (SOTA) approaches across five metrics, including a proposed metric called Phoneme Error Rate (PER) that evaluates lip pose intelligibility and video fluency. The experimental results demonstrate that our FluentLip approach is highly competitive, achieving significant improvements in smoothness and naturalness. In particular, it outperforms these SOTA approaches by approximately $\textbf{16.3%}$ in Fr'echet Inception Distance (FID) and $\textbf{35.2%}$ in PER.
生成与给定语音相匹配的连续唇动图像在音频驱动唇形合成中是一项具有挑战性的任务。尽管之前的研究在同步和视觉质量方面取得了进展,但唇形的清晰度和视频的流畅度仍然是持久的挑战。本研究提出了FluentLip,这是一种用于音频驱动的唇形合成的两阶段方法,结合了三种特征策略。为了改善唇形同步和清晰度,我们整合了音素提取器和编码器,以生成用于多模式学习的音频和音素信息融合。此外,我们采用光流一致性损失来确保图像帧之间的自然过渡。此外,我们在生成对抗网络(GANs)的训练过程中引入了扩散链,以提高稳定性和效率。我们通过大量实验评估了所提出的FluentLip,将其与五种最先进的方法在五个指标上进行比较,包括一个名为音素错误率(PER)的提出指标,该指标评估唇形姿势的清晰度和视频的流畅度。实验结果证明,我们的FluentLip方法具有很强的竞争力,在平滑度和自然度方面实现了显著改进。特别是,它在Fréchet Inception Distance(FID)上比这些最先进的方法高出约**16.3%,在PER上高出35.2%**。
论文及项目相关链接
Summary
本文提出一种名为FluentLip的两阶段音频驱动唇动合成方法,旨在解决连续图像生成中与给定语音相匹配的唇动合成挑战。方法包括利用音频和音素信息的融合进行多模态学习,以提高唇同步和可识别度;采用光流一致性损失确保图像帧之间的自然过渡;在生成对抗网络(GANs)的训练过程中引入扩散链,以提高稳定性和效率。实验结果表明,FluentLip在平滑性和自然性方面表现出高度竞争力,相较于其他先进方法,在Fréchet Inception Distance (FID)和提出的用于评估唇姿态可识别度和视频流畅度的音素错误率(PER)上取得了显著改进。
Key Takeaways
- FluentLip是一种两阶段的音频驱动唇动合成方法,旨在解决唇动图像的连续生成与音频中语音的匹配问题。
- 通过整合音素提取器和编码器,生成音频和音素信息的融合,进行多模态学习,提高唇同步和可识别度。
- 采用光流一致性损失,确保图像帧之间的自然过渡。
- 在GANs的训练中引入扩散链,提高稳定性和效率。
- 实验结果展示FluentLip在平滑性和自然性方面的优势。
- 与其他先进方法相比,FluentLip在FID和PER指标上取得了显著改进。
点此查看论文截图
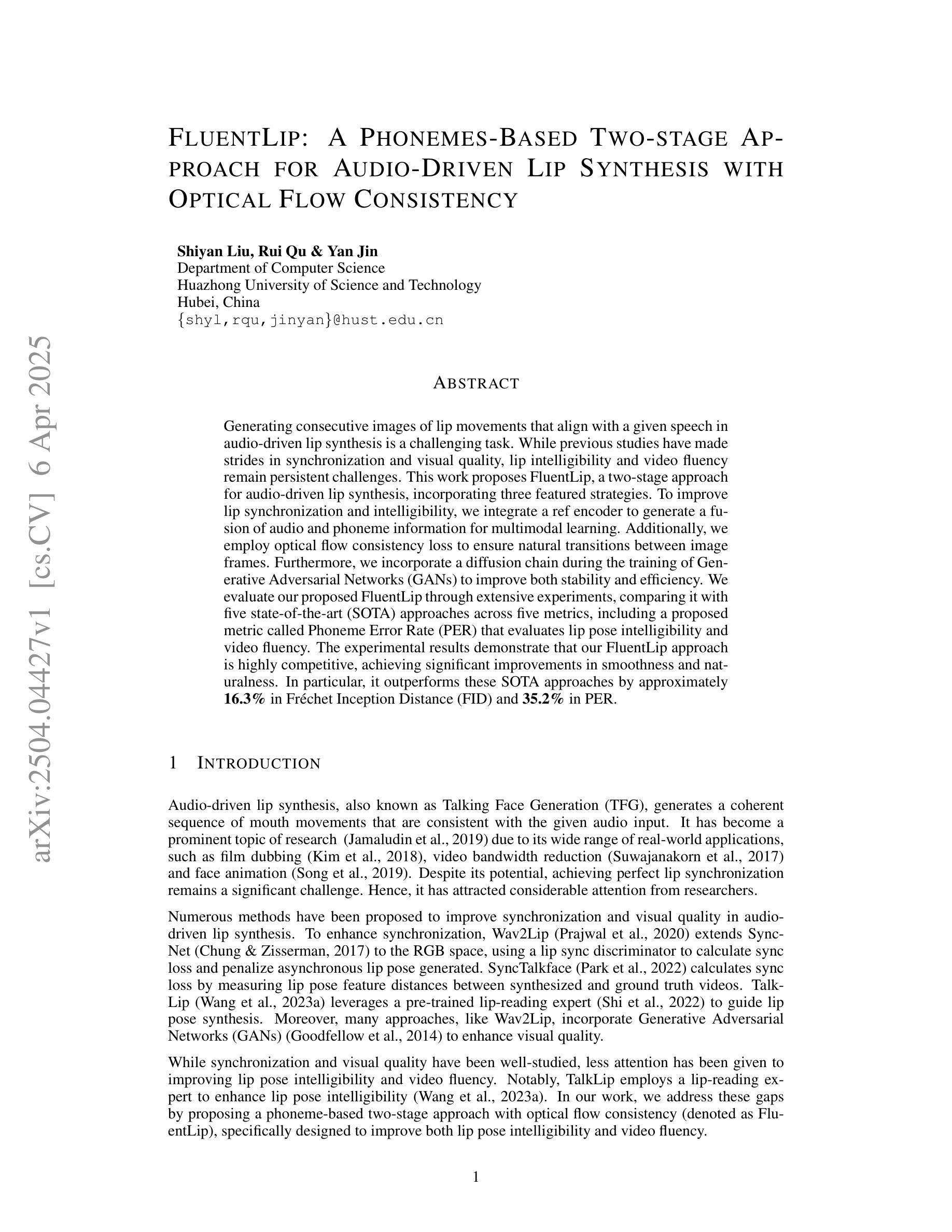
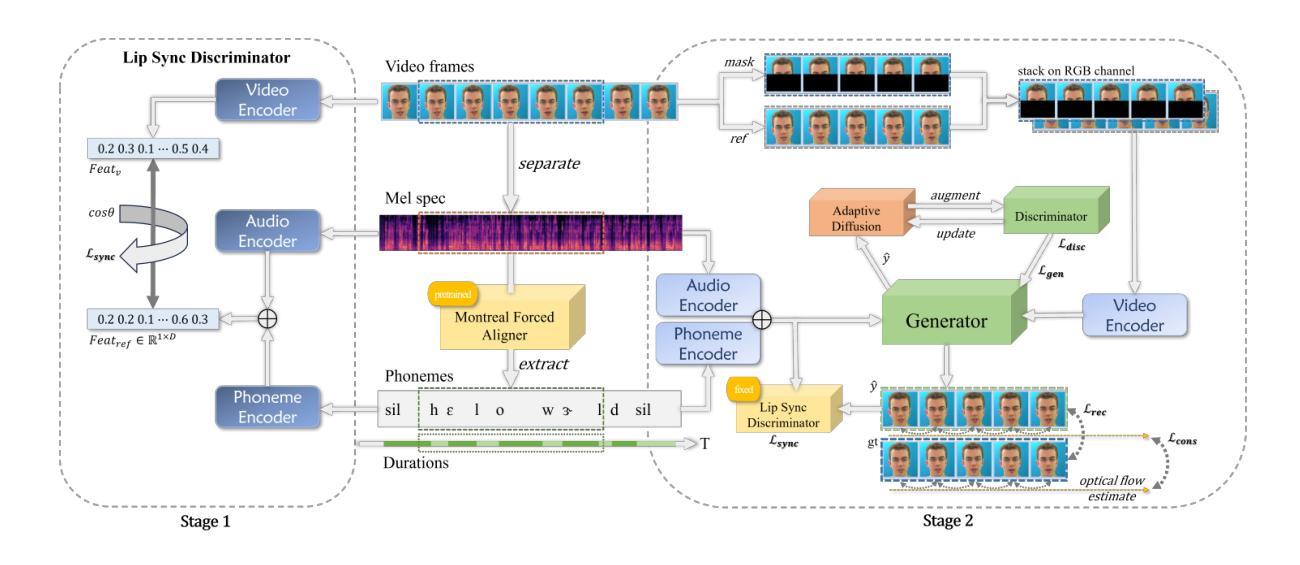
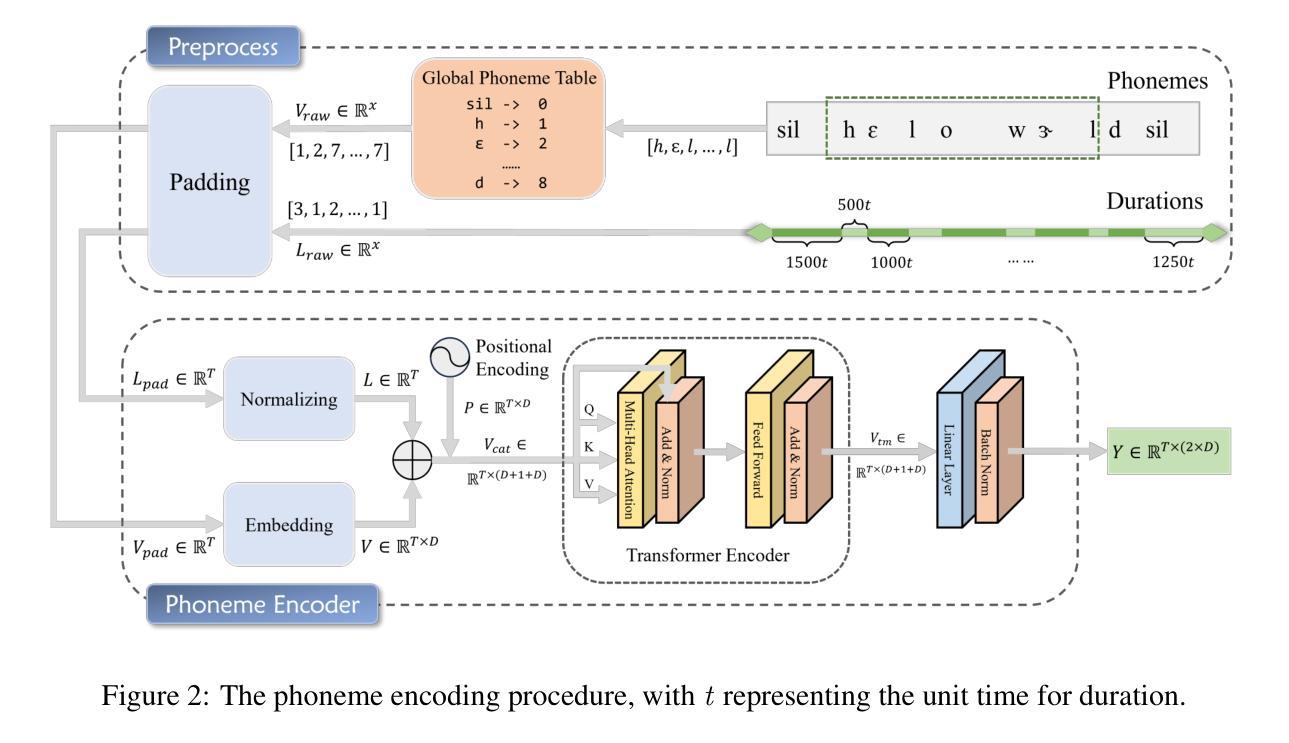
AnomalyHybrid: A Domain-agnostic Generative Framework for General Anomaly Detection
Authors:Ying Zhao
Anomaly generation is an effective way to mitigate data scarcity for anomaly detection task. Most existing works shine at industrial anomaly generation with multiple specialists or large generative models, rarely generalizing to anomalies in other applications. In this paper, we present AnomalyHybrid, a domain-agnostic framework designed to generate authentic and diverse anomalies simply by combining the reference and target images. AnomalyHybrid is a Generative Adversarial Network(GAN)-based framework having two decoders that integrate the appearance of reference image into the depth and edge structures of target image respectively. With the help of depth decoders, AnomalyHybrid achieves authentic generation especially for the anomalies with depth values changing, such a s protrusion and dent. More, it relaxes the fine granularity structural control of the edge decoder and brings more diversity. Without using annotations, AnomalyHybrid is easily trained with sets of color, depth and edge of same images having different augmentations. Extensive experiments carried on HeliconiusButterfly, MVTecAD and MVTec3D datasets demonstrate that AnomalyHybrid surpasses the GAN-based state-of-the-art on anomaly generation and its downstream anomaly classification, detection and segmentation tasks. On MVTecAD dataset, AnomalyHybrid achieves 2.06/0.32 IS/LPIPS for anomaly generation, 52.6 Acc for anomaly classification with ResNet34, 97.3/72.9 AP for image/pixel-level anomaly detection with a simple UNet.
异常生成是缓解异常检测任务中数据稀缺问题的有效方法。大多数现有工作擅长于使用多个专家或大型生成模型进行工业异常生成,很少推广到其他应用的异常。在本文中,我们提出了AnomalyHybrid,这是一个基于领域的框架,旨在通过结合参考图像和目标图像来生成真实且多样化的异常值。AnomalyHybrid是一个基于生成对抗网络(GAN)的框架,具有两个解码器,分别将参考图像的外观集成到目标图像的深度和边缘结构中。借助深度解码器,AnomalyHybrid实现了真实生成,尤其是对于深度值变化的异常值,如凸起和凹陷。此外,它放宽了边缘解码器的精细粒度结构控制并带来了更多样性。无需使用注释,AnomalyHybrid可以很容易地使用具有不同增强的同一图像的颜色、深度和边缘集合进行训练。在HeliconiusButterfly、MVTecAD和MVTec3D数据集上进行的广泛实验表明,AnomalyHybrid在异常生成及其下游异常分类、检测和分割任务上超过了基于GAN的最新技术。在MVTecAD数据集上,AnomalyHybrid在异常生成方面达到了2.06/0.32的IS/LPIPS指标,使用ResNet34进行异常分类的准确率为52.6%,使用简单的UNet进行图像/像素级异常检测的AP为97.3/72.9。
论文及项目相关链接
PDF Accepted to CVPR 2025 workshop on Harnessing Generative Models for Synthetic Visual Datasets (SyntaGen)
Summary
本文提出一种名为AnomalyHybrid的通用异常生成框架,通过结合参考图像和目标图像,生成真实且多样的异常图像。该框架基于生成对抗网络(GAN),包含两个解码器,分别将参考图像的外观融入目标图像的深度和边缘结构。AnomalyHybrid能在不使用注释的情况下,通过不同增强方式的图像集进行训练,实现真实异常的生成,特别是在深度值变化的异常上表现突出,如凸起和凹陷等。在HeliconiusButterfly、MVTecAD和MVTec3D数据集上的实验表明,AnomalyHybrid在异常生成及其下游的异常分类、检测和分割任务上超越了基于GAN的最新技术。
Key Takeaways
Anomaly generation is an effective way to mitigate data scarcity for anomaly detection tasks.
异常生成是缓解异常检测任务中数据稀缺问题的有效方法。AnomalyHybrid是一个基于GAN的通用异常生成框架,能够通过结合参考图像和目标图像生成真实且多样的异常图像。
AnomalyHybrid框架可生成真实的多元异常图像,它基于GAN技术并整合参考图像。AnomalyHybrid包含两个解码器,分别处理参考图像的外观与目标图像的深度和边缘结构。
AnomalyHybrid具有两个解码器,一个处理参考图像的外观信息融入目标图像中,另一个处理深度和边缘结构信息。
点此查看论文截图
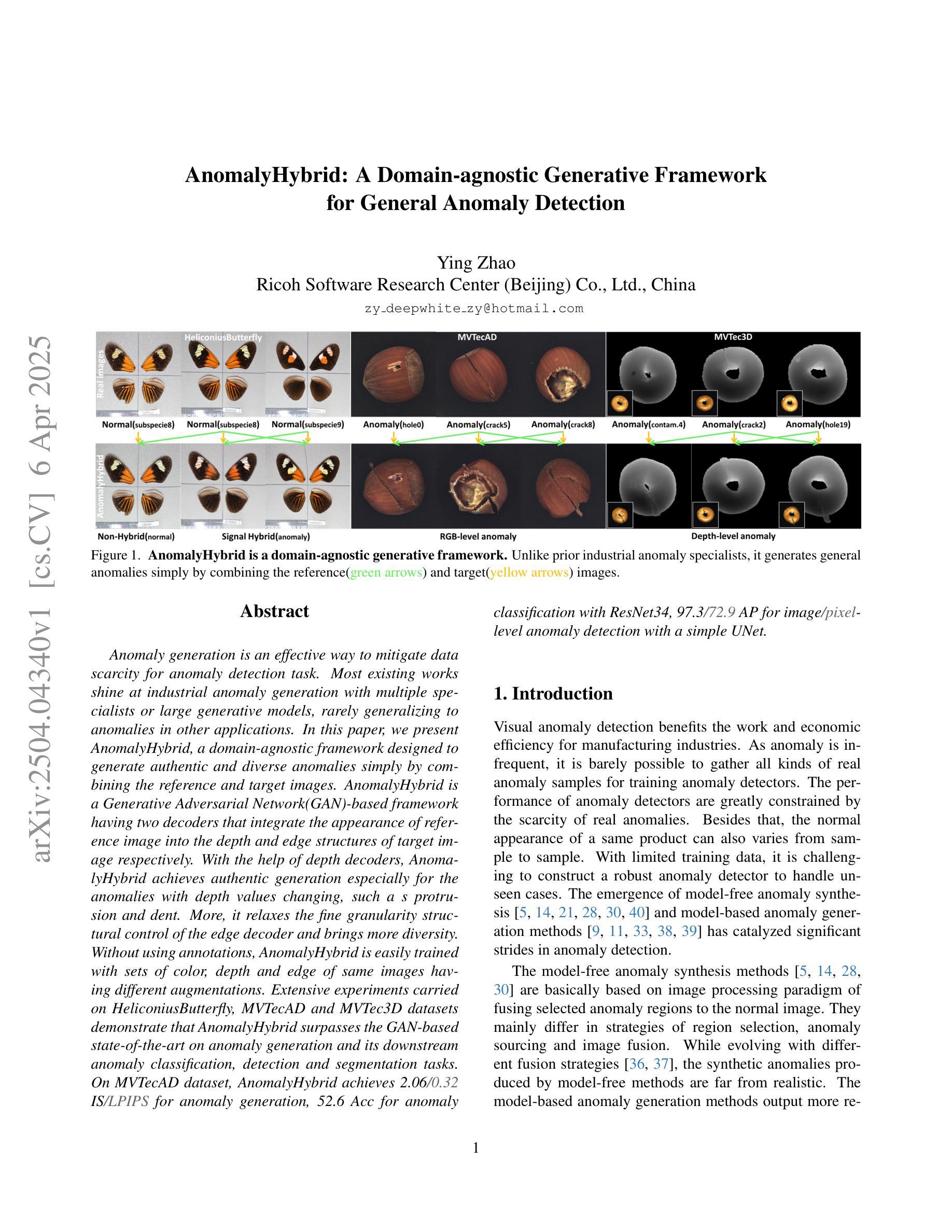
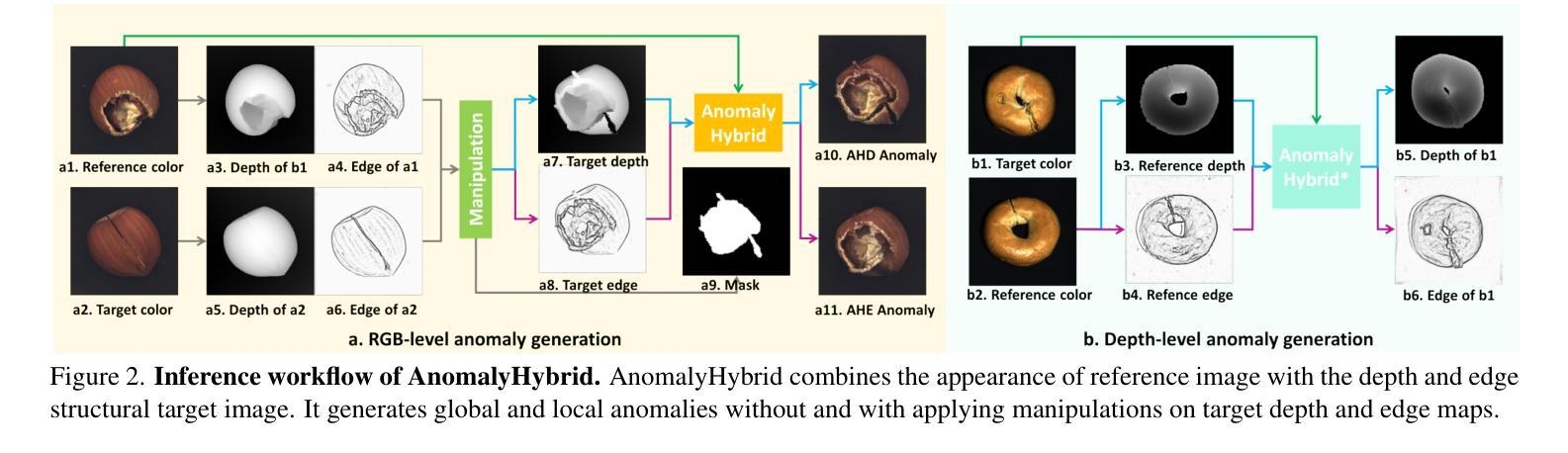
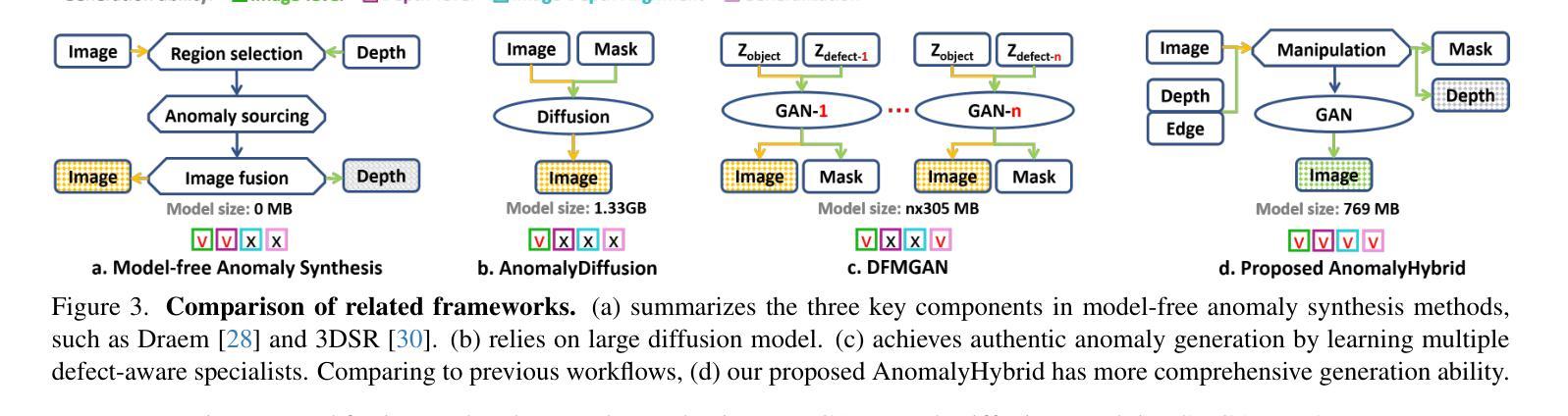
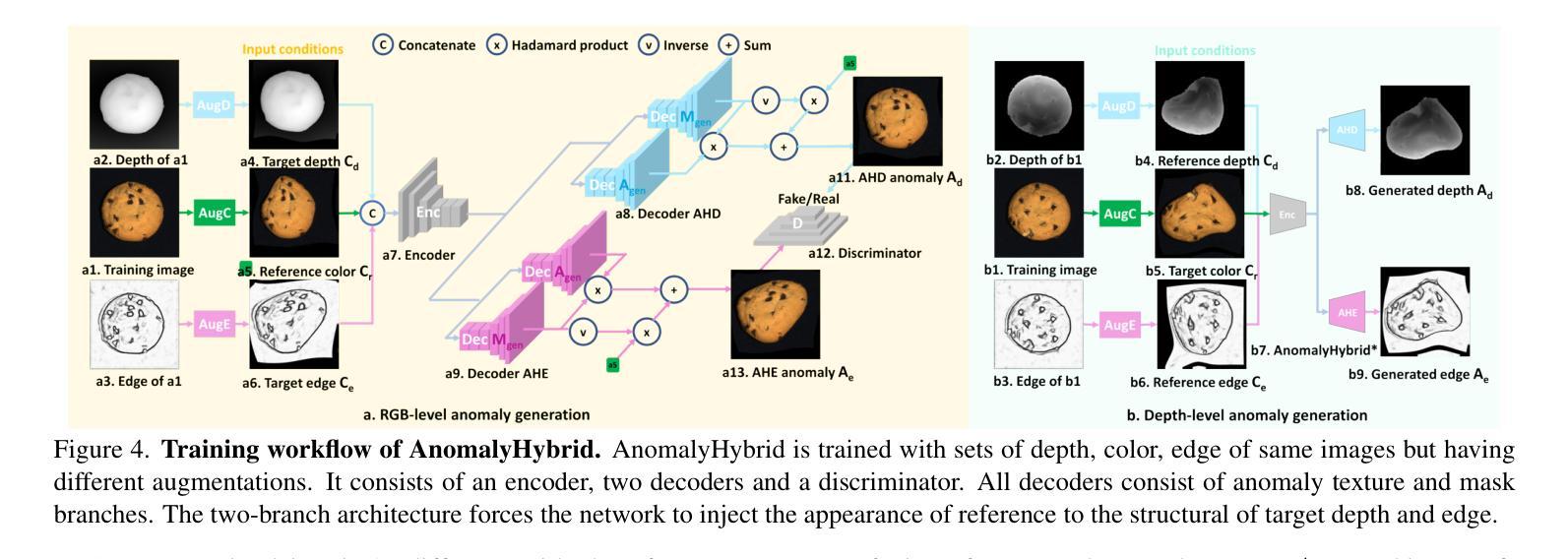
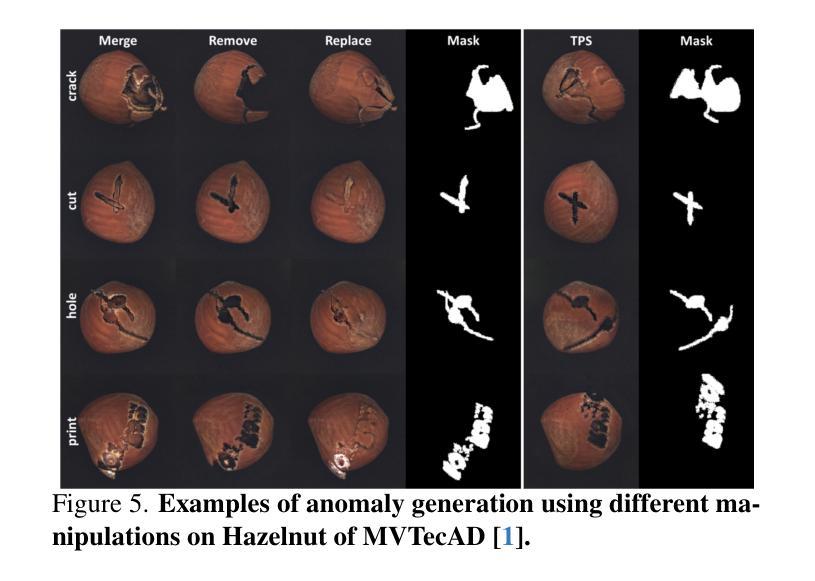
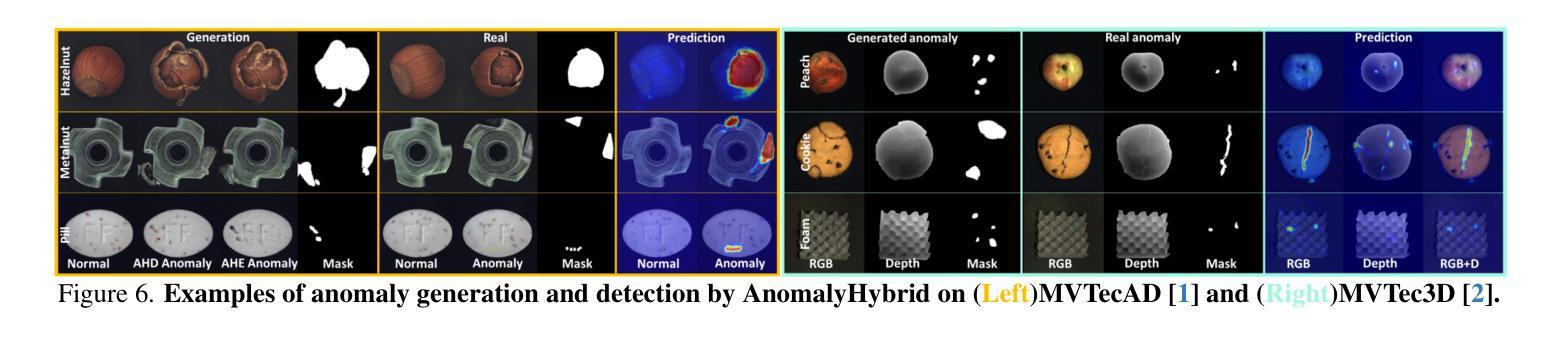
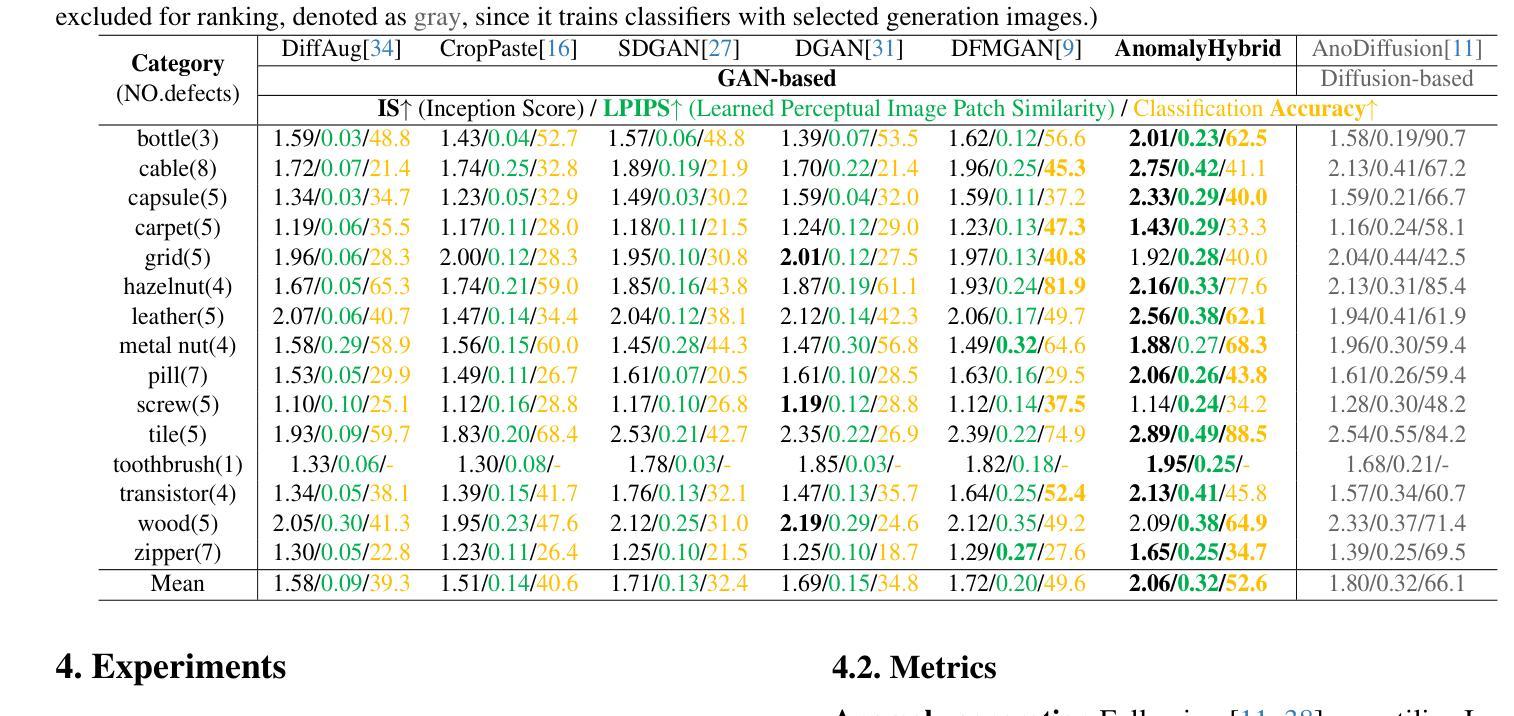
A Hybrid Wavelet-Fourier Method for Next-Generation Conditional Diffusion Models
Authors:Andrew Kiruluta, Andreas Lemos
We present a novel generative modeling framework,Wavelet-Fourier-Diffusion, which adapts the diffusion paradigm to hybrid frequency representations in order to synthesize high-quality, high-fidelity images with improved spatial localization. In contrast to conventional diffusion models that rely exclusively on additive noise in pixel space, our approach leverages a multi-transform that combines wavelet sub-band decomposition with partial Fourier steps. This strategy progressively degrades and then reconstructs images in a hybrid spectral domain during the forward and reverse diffusion processes. By supplementing traditional Fourier-based analysis with the spatial localization capabilities of wavelets, our model can capture both global structures and fine-grained features more effectively. We further extend the approach to conditional image generation by integrating embeddings or conditional features via cross-attention. Experimental evaluations on CIFAR-10, CelebA-HQ, and a conditional ImageNet subset illustrate that our method achieves competitive or superior performance relative to baseline diffusion models and state-of-the-art GANs, as measured by Fr'echet Inception Distance (FID) and Inception Score (IS). We also show how the hybrid frequency-based representation improves control over global coherence and fine texture synthesis, paving the way for new directions in multi-scale generative modeling.
我们提出了一种新型生成建模框架——Wavelet-Fourier-Diffusion,该框架将扩散范式适应于混合频率表示,以合成具有改进的空间定位的高质量、高保真图像。与传统的仅依赖于像素空间中的添加性噪声的扩散模型不同,我们的方法利用了一种多转换技术,结合了小波子带分解和部分傅里叶步骤。该策略在正向和反向扩散过程中在混合光谱域中逐步降解然后重建图像。通过结合传统的基于傅里的分析与小波的空间定位能力,我们的模型可以更有效地捕获全局结构和精细特征。我们通过集成嵌入或通过交叉注意力添加条件特征,进一步将该方法扩展到条件图像生成。在CIFAR-10、CelebA-HQ和条件ImageNet子集上的实验评估表明,我们的方法在Férchet Inception Distance(FID)和Inception Score(IS)方面相对于基线扩散模型和最新GAN达到了具有竞争力的或更优越的性能。我们还展示了基于混合频率的表示如何改善全局一致性和精细纹理合成的控制,为多角度生成建模开辟了新的方向。
论文及项目相关链接
Summary
本文提出了一种新颖的生成模型框架——Wavelet-Fourier-Diffusion,该框架结合了扩散模型与混合频率表示技术,旨在合成高质量、高保真度的图像,并改进空间定位能力。与传统的仅依赖像素空间添加噪声的扩散模型不同,该模型采用多变换融合技术,结合小波子带分解和部分傅里叶步骤。在正向和反向扩散过程中,该策略在混合光谱域中逐步降解并重建图像。通过结合传统的基于傅里叶的分析与小波的空间定位能力,该模型可以更有效地捕捉全局结构和精细特征。此外,通过嵌入或条件特征通过交叉注意力进行集成,将该方法扩展到条件图像生成。在CIFAR-10、CelebA-HQ和条件ImageNet子集上的实验评估表明,该方法相对于基线扩散模型和最先进的GANs具有竞争性或优越性,如以Fréchet Inception Distance(FID)和Inception Score(IS)衡量。同时,混合频率表示技术提高了全局一致性和精细纹理合成的控制性,为跨尺度生成建模开辟了新方向。
Key Takeaways
- 提出了Wavelet-Fourier-Diffusion生成模型框架,结合了扩散模型与混合频率表示技术。
- 框架采用多变换融合技术,结合小波子带分解和部分傅里叶步骤,以提高图像合成的质量。
- 在正向和反向扩散过程中,模型在混合光谱域中逐步降解并重建图像。
- 结合传统的基于傅里叶的分析与小波的空间定位能力,更有效地捕捉全局结构和精细特征。
- 通过嵌入或条件特征通过交叉注意力集成,实现条件图像生成。
- 在多个数据集上的实验评估表明,该方法的性能具有竞争性或优越性。
点此查看论文截图
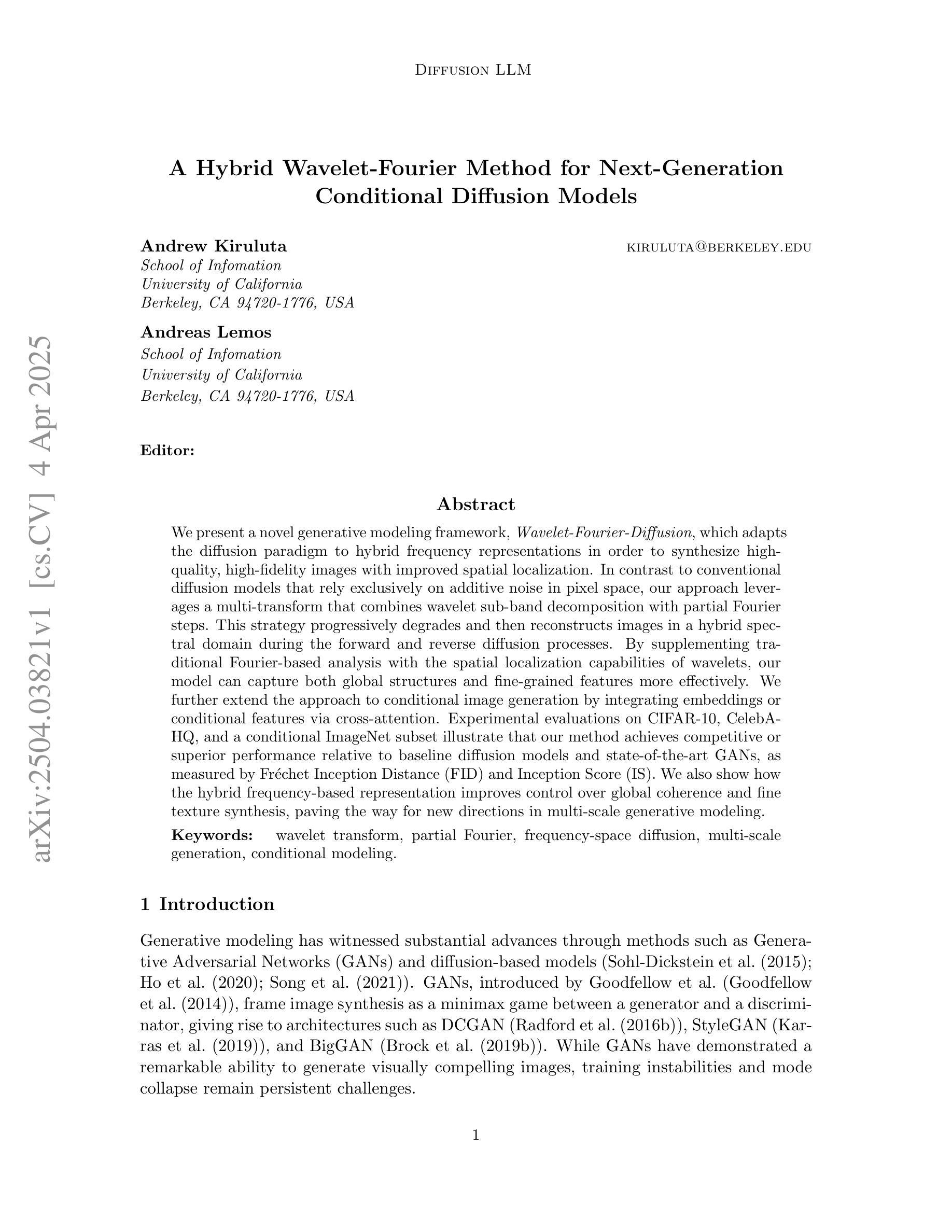
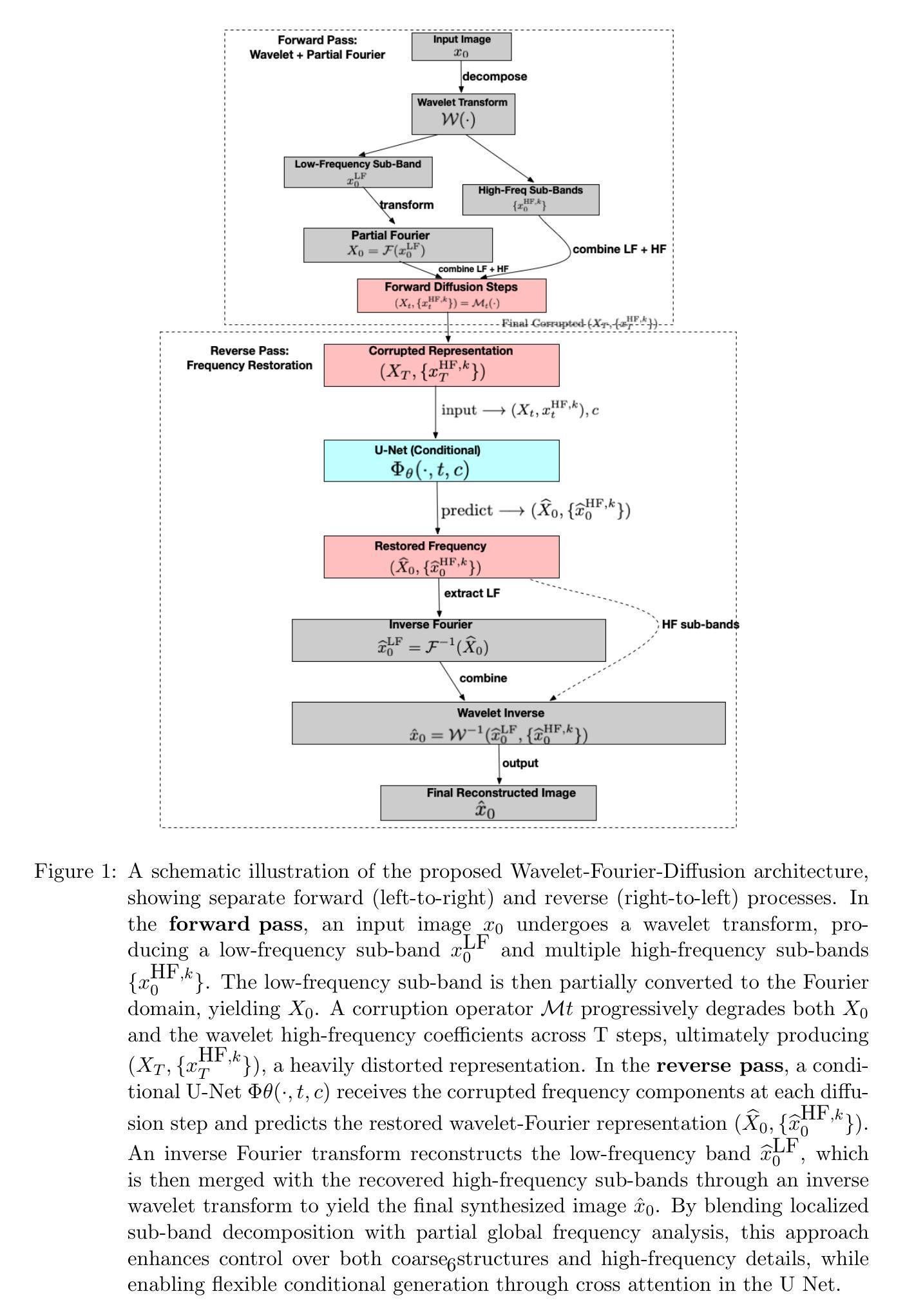