⚠️ 以下所有内容总结都来自于 大语言模型的能力,如有错误,仅供参考,谨慎使用
🔴 请注意:千万不要用于严肃的学术场景,只能用于论文阅读前的初筛!
💗 如果您觉得我们的项目对您有帮助 ChatPaperFree ,还请您给我们一些鼓励!⭐️ HuggingFace免费体验
2025-04-09 更新
FantasyTalking: Realistic Talking Portrait Generation via Coherent Motion Synthesis
Authors:Mengchao Wang, Qiang Wang, Fan Jiang, Yaqi Fan, Yunpeng Zhang, Yonggang Qi, Kun Zhao, Mu Xu
Creating a realistic animatable avatar from a single static portrait remains challenging. Existing approaches often struggle to capture subtle facial expressions, the associated global body movements, and the dynamic background. To address these limitations, we propose a novel framework that leverages a pretrained video diffusion transformer model to generate high-fidelity, coherent talking portraits with controllable motion dynamics. At the core of our work is a dual-stage audio-visual alignment strategy. In the first stage, we employ a clip-level training scheme to establish coherent global motion by aligning audio-driven dynamics across the entire scene, including the reference portrait, contextual objects, and background. In the second stage, we refine lip movements at the frame level using a lip-tracing mask, ensuring precise synchronization with audio signals. To preserve identity without compromising motion flexibility, we replace the commonly used reference network with a facial-focused cross-attention module that effectively maintains facial consistency throughout the video. Furthermore, we integrate a motion intensity modulation module that explicitly controls expression and body motion intensity, enabling controllable manipulation of portrait movements beyond mere lip motion. Extensive experimental results show that our proposed approach achieves higher quality with better realism, coherence, motion intensity, and identity preservation. Ours project page: https://fantasy-amap.github.io/fantasy-talking/.
从单一静态肖像创建逼真的可动画头像仍然是一个挑战。现有方法往往难以捕捉微妙的面部表情、相关的全身动作和动态背景。为了解决这些局限性,我们提出了一种新型框架,该框架利用预训练的视频扩散变压器模型生成高保真、连贯的说话肖像,具有可控的运动动态。我们工作的核心是双重阶段的音频视觉对齐策略。在第一阶段,我们采用剪辑级训练方案,通过在整个场景(包括参考肖像、上下文对象和背景)中对齐音频驱动的动态,建立连贯的全身运动。在第二阶段,我们在帧级别使用唇部追踪蒙版来微调唇部运动,确保与音频信号的精确同步。为了在不损害运动灵活性的情况下保留身份,我们用一个面部重点交叉注意模块取代了常用的参考网络,该模块可以有效地在整个视频中保持面部一致性。此外,我们集成了一个运动强度调制模块,该模块可以明确地控制表情和躯体运动的强度,实现对肖像运动的可控操纵,而不仅仅是唇部运动。大量的实验结果表明,我们提出的方法在质量、真实性、连贯性、运动强度和身份保留方面达到了更高的水平。我们的项目页面为:https://fantasy-amap.github.io/fantasy-talking/。
论文及项目相关链接
Summary:
本文提出了一种新的框架,利用预训练的视频扩散变压器模型生成高保真、连贯的动画肖像,具有可控的运动动态。该框架采用双阶段音频视觉对齐策略,第一阶段通过剪辑级训练方案建立全局运动的一致性,第二阶段在帧级别细化唇部运动,同时确保与音频信号的精确同步。此外,还采用了面部重点交叉注意力模块和运动强度调制模块,以在保持身份的同时实现灵活的运动控制。
Key Takeaways:
- 利用预训练的视频扩散变压器模型生成动画肖像。
- 采用双阶段音频视觉对齐策略,确保音频驱动的动态与整个场景的一致性。
- 在第一阶段建立全局运动的一致性。
- 在第二阶段细化唇部运动,并与音频信号精确同步。
- 采用面部重点交叉注意力模块以保持身份的一致性。
- 引入运动强度调制模块,控制表达和身体运动的强度。
- 实验结果表明,该方法在质量、现实感、连贯性、运动强度和身份保持方面表现优异。
点此查看论文截图
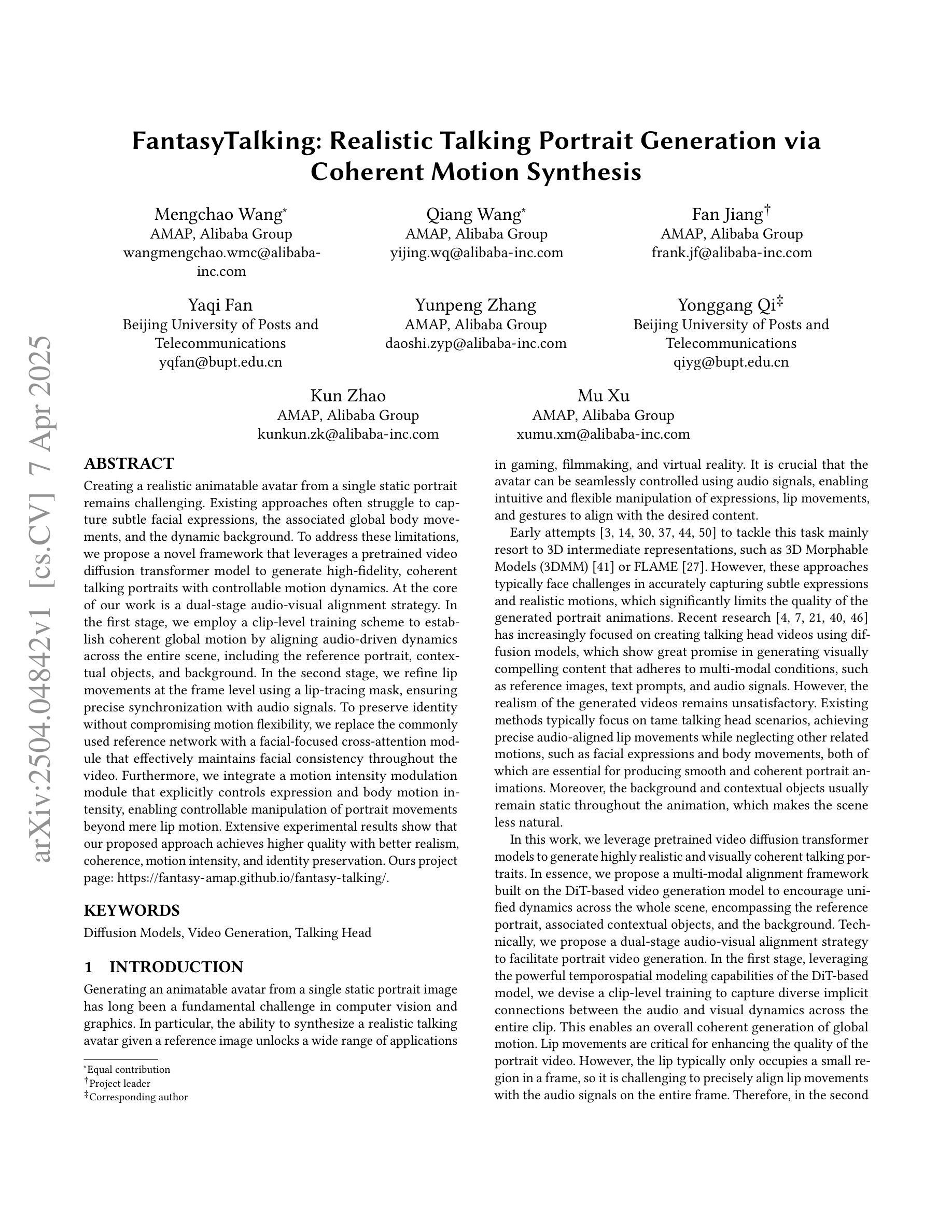
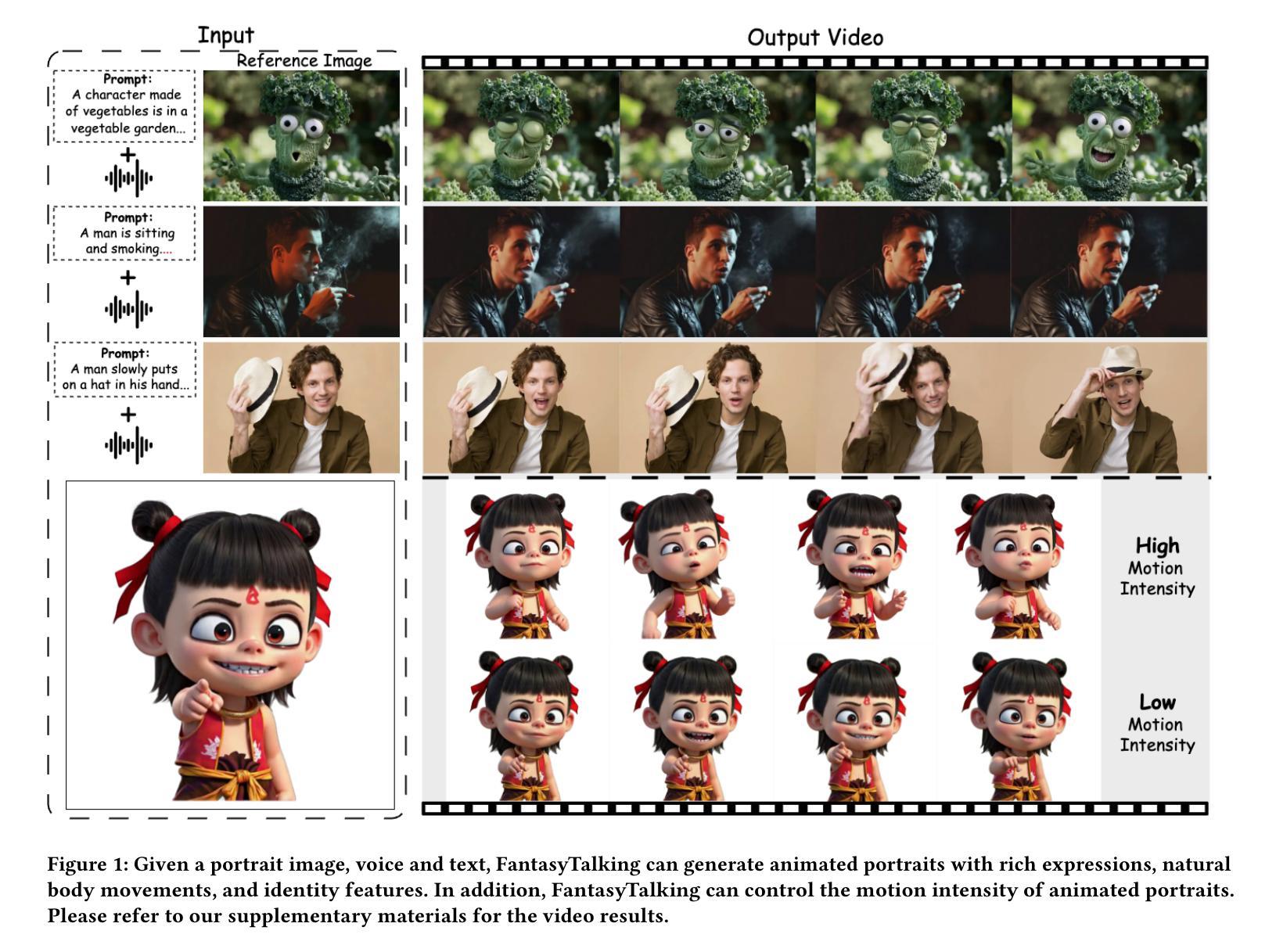
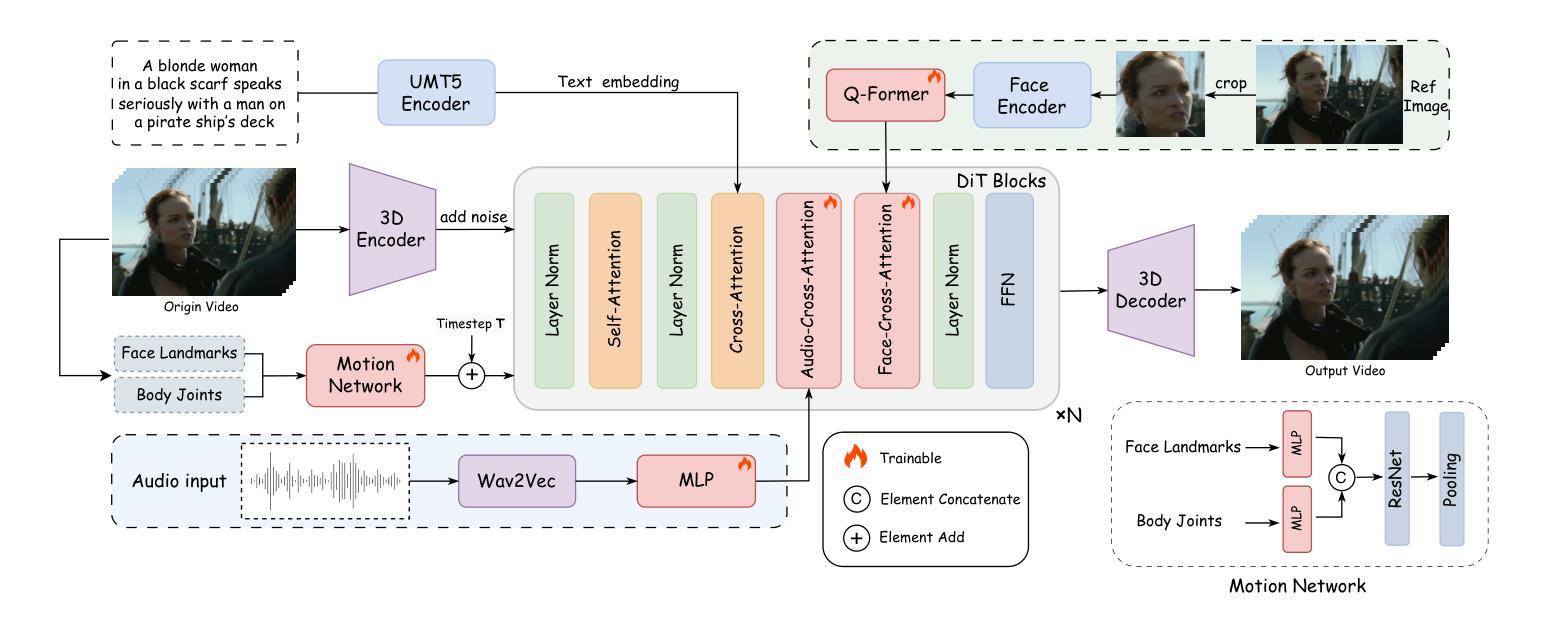
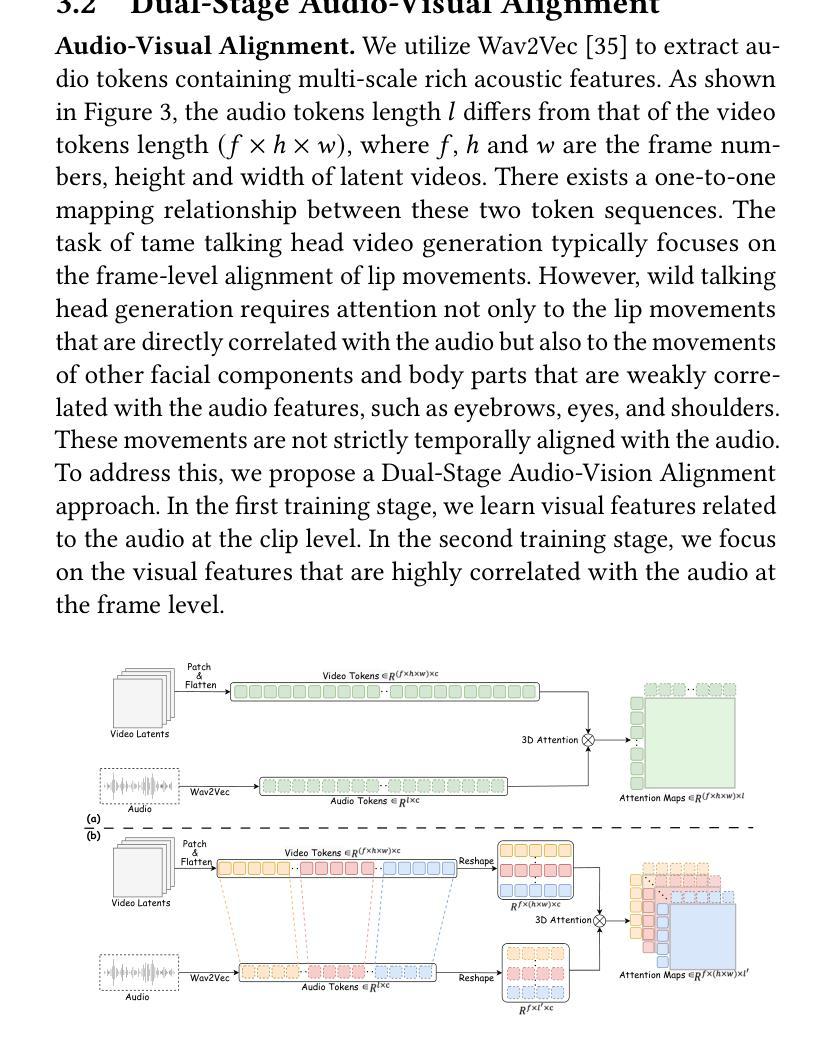
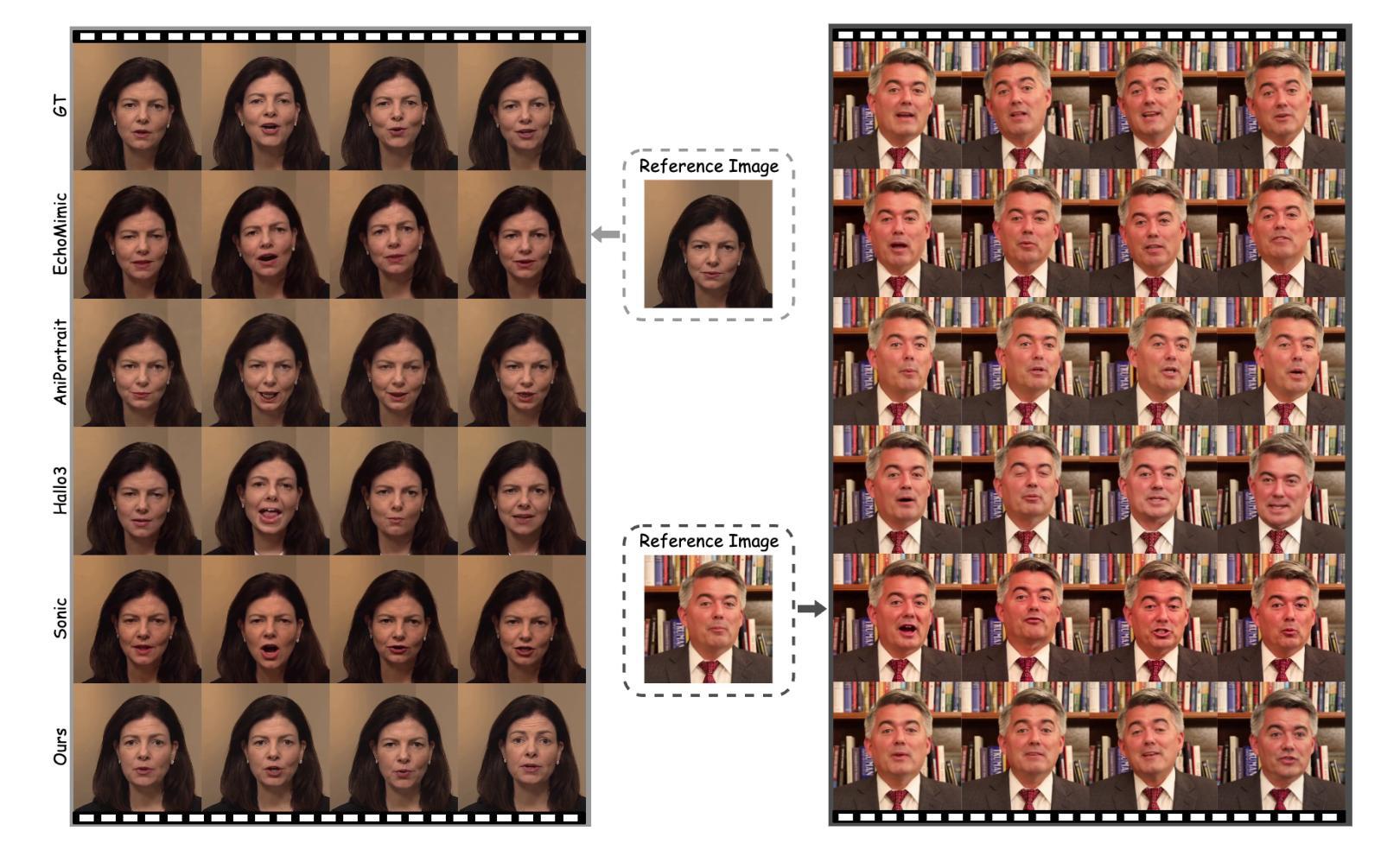
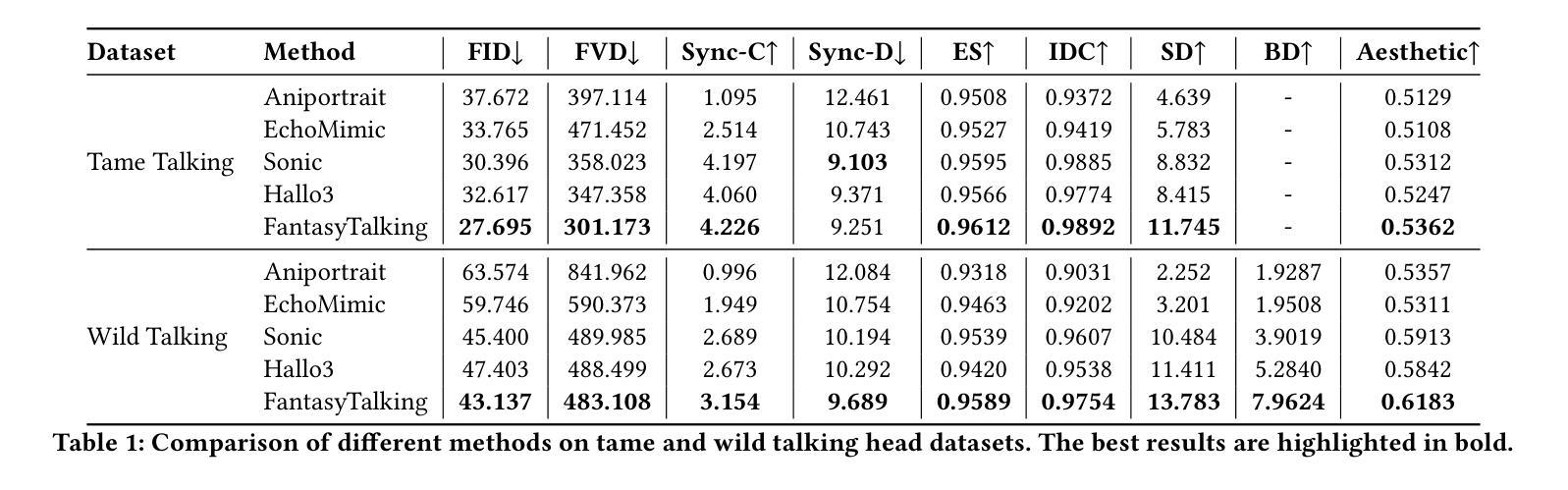
FluentLip: A Phonemes-Based Two-stage Approach for Audio-Driven Lip Synthesis with Optical Flow Consistency
Authors:Shiyan Liu, Rui Qu, Yan Jin
Generating consecutive images of lip movements that align with a given speech in audio-driven lip synthesis is a challenging task. While previous studies have made strides in synchronization and visual quality, lip intelligibility and video fluency remain persistent challenges. This work proposes FluentLip, a two-stage approach for audio-driven lip synthesis, incorporating three featured strategies. To improve lip synchronization and intelligibility, we integrate a phoneme extractor and encoder to generate a fusion of audio and phoneme information for multimodal learning. Additionally, we employ optical flow consistency loss to ensure natural transitions between image frames. Furthermore, we incorporate a diffusion chain during the training of Generative Adversarial Networks (GANs) to improve both stability and efficiency. We evaluate our proposed FluentLip through extensive experiments, comparing it with five state-of-the-art (SOTA) approaches across five metrics, including a proposed metric called Phoneme Error Rate (PER) that evaluates lip pose intelligibility and video fluency. The experimental results demonstrate that our FluentLip approach is highly competitive, achieving significant improvements in smoothness and naturalness. In particular, it outperforms these SOTA approaches by approximately $\textbf{16.3%}$ in Fr'echet Inception Distance (FID) and $\textbf{35.2%}$ in PER.
生成与给定语音相对应的连续唇部动作图像在音频驱动唇部合成中是一项具有挑战性的任务。尽管之前的研究在同步和视觉质量方面取得了进展,但唇部的清晰度和视频流畅度仍然是一个持久的挑战。这项工作提出了FluentLip,这是一种用于音频驱动的唇部合成的两阶段方法,结合了三种特色策略。为了改善唇部同步和清晰度,我们整合了音素提取器和编码器,以生成音频和音素信息的融合,用于多模态学习。另外,我们采用光流一致性损失来确保图像帧之间的自然过渡。此外,我们在生成对抗网络(GANs)的训练过程中引入了扩散链,以提高稳定性和效率。我们通过大量实验评估了所提出的FluentLip,将其与五种最新方法进行比较,包括一个名为音素错误率(PER)的提出指标,该指标评估唇部姿态的清晰度和视频流畅度。实验结果证明,我们的FluentLip方法具有很强的竞争力,在平滑度和自然度方面取得了显著改进。特别是,它在Fréchet Inception Distance(FID)上比这些最新方法高出约**16.3%,在PER上高出35.2%**。
论文及项目相关链接
Summary
本文提出一种名为FluentLip的两阶段音频驱动唇动图像生成方法,旨在解决音频与唇动图像同步生成时的挑战。该方法通过融合音频和音素信息、采用光学流一致性损失以及改进生成对抗网络训练过程中的扩散链等技术,提高了唇同步、可理解性和视频流畅性。实验结果表明,FluentLip在平滑度和自然度方面表现出高度竞争力,与现有先进方法相比,在Fréchet Inception Distance(FID)和 Phoneme Error Rate(PER)等评价指标上实现了显著改进。
Key Takeaways
- FluentLip是一种两阶段的音频驱动唇动图像生成方法,旨在解决音频与唇动图像同步生成的挑战。
- 通过融合音频和音素信息,提高唇同步和可理解性。
- 采用光学流一致性损失,确保图像帧之间的自然过渡。
- 改进生成对抗网络训练过程中的扩散链,提高稳定性和效率。
- 通过广泛实验评估FluentLip性能,与五种最新技术进行对比。
- 提出一种新的评价指标——Phoneme Error Rate(PER),用于评估唇姿态可理解性和视频流畅性。
点此查看论文截图
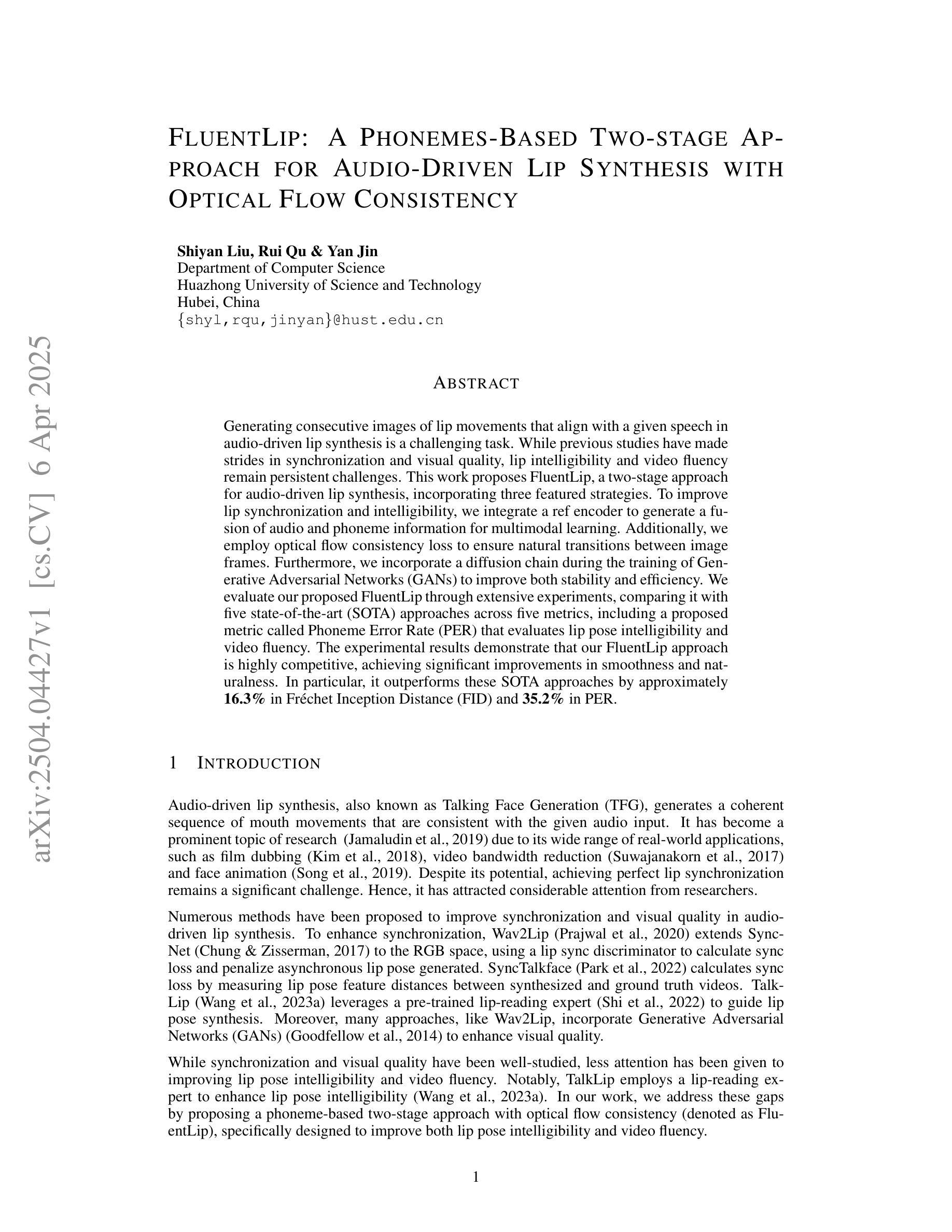
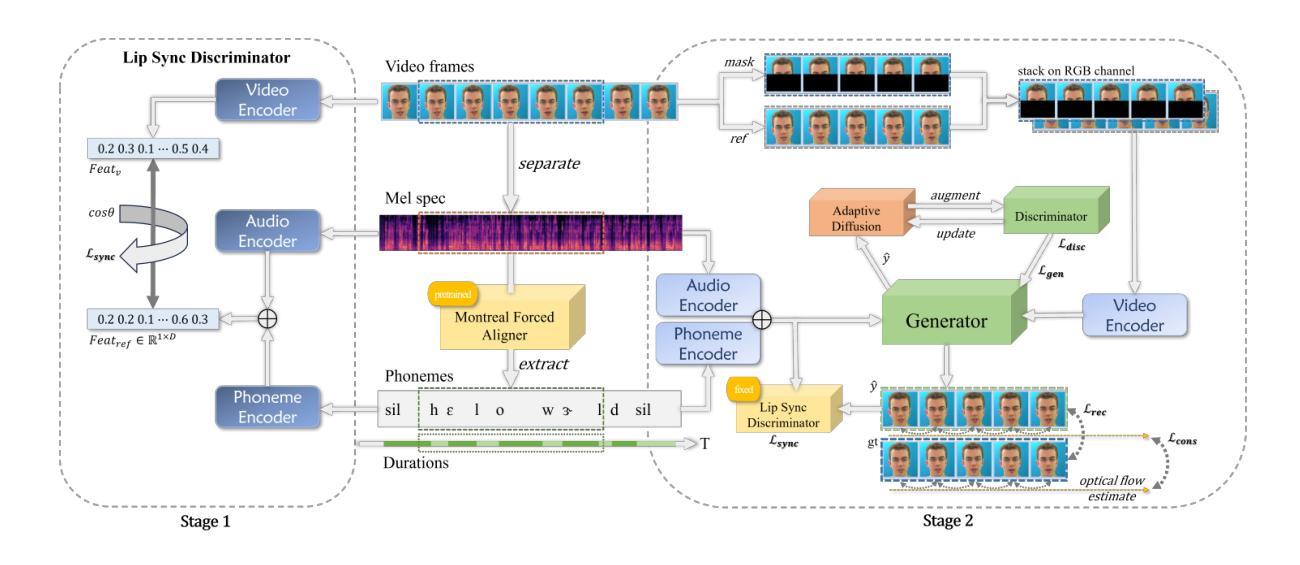
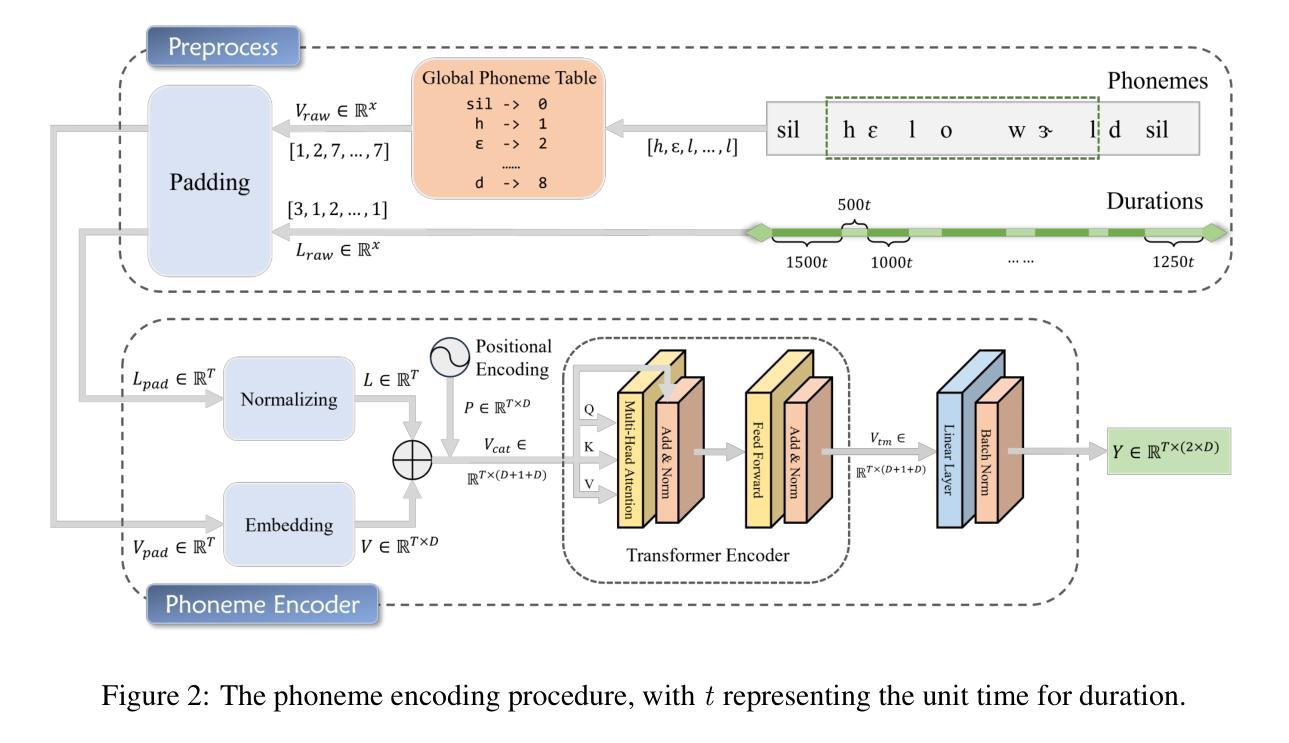
What Large Language Models Do Not Talk About: An Empirical Study of Moderation and Censorship Practices
Authors:Sander Noels, Guillaume Bied, Maarten Buyl, Alexander Rogiers, Yousra Fettach, Jefrey Lijffijt, Tijl De Bie
Large Language Models (LLMs) are increasingly deployed as gateways to information, yet their content moderation practices remain underexplored. This work investigates the extent to which LLMs refuse to answer or omit information when prompted on political topics. To do so, we distinguish between hard censorship (i.e., generated refusals, error messages, or canned denial responses) and soft censorship (i.e., selective omission or downplaying of key elements), which we identify in LLMs’ responses when asked to provide information on a broad range of political figures. Our analysis covers 14 state-of-the-art models from Western countries, China, and Russia, prompted in all six official United Nations (UN) languages. Our analysis suggests that although censorship is observed across the board, it is predominantly tailored to an LLM provider’s domestic audience and typically manifests as either hard censorship or soft censorship (though rarely both concurrently). These findings underscore the need for ideological and geographic diversity among publicly available LLMs, and greater transparency in LLM moderation strategies to facilitate informed user choices. All data are made freely available.
大型语言模型(LLM)越来越多地被部署为信息网关,但它们的内容管理实践仍未得到充分探索。本研究调查了LLM在政治话题提示下拒绝回答或省略信息的程度。为此,我们区分了硬审查(例如生成的拒绝、错误消息或标准的拒绝回答)和软审查(例如选择性遗漏或淡化关键要素),这在LLM被要求对一系列政治人物提供信息时才会显露出来。我们的分析涵盖了来自西方、中国和俄罗斯的共十四种最先进模型,并在联合国六种官方语言中进行了提示。我们的分析表明,尽管普遍存在着审查现象,但审查主要还是针对LLM供应商的国内受众的,主要表现为硬审查或软审查(但通常不并发)。这些发现突显出公开可用的LLM需要在意识形态和地理上具有多样性,以及LLM管理策略需要更大的透明度,以便用户做出明智的选择。所有数据均已免费公开。
论文及项目相关链接
PDF 17 pages, 38 pages in total including appendix; 5 figures, 22 figures in appendix
摘要
LLM作为信息检索的门户越来越受欢迎,但其内容管理实践尚未得到充分研究。本研究探讨了LLM在政治话题上拒绝回答或省略信息的程度。我们区分了硬审查(如生成的拒绝回答、错误消息或标准拒绝响应)和软审查(如选择性遗漏或淡化关键元素),这些在针对一系列政治人物的提问时存在于LLM的响应中。我们的分析涵盖了来自西方、中国和俄罗斯的最新技术模型共十四种模型,并用联合国六种官方语言提示它们。分析表明,尽管普遍存在审查现象,但主要是针对LLM供应商国内受众量身定制的,通常表现为硬审查或软审查(很少同时出现)。这些发现强调,公众可用LLM模型中需要增加意识形态和地理多样性,并且在LLM的调节策略中需要更多的透明度以促进用户做出明智的选择。所有数据可自由获取。
关键见解
- LLM被越来越多地用作信息检索的门户,但其内容管理实践仍然未被充分研究。
- 在对政治话题的提示下,LLM表现出拒绝回答或省略信息的现象。
- 我们区分了硬审查和软审查两种审查方式,存在于LLM对一系列政治人物的回应中。
- LLM审查现象普遍存在,但主要是根据LLM供应商国内受众量身定制的。
- LLM模型需要更多的意识形态和地理多样性。
- 在LLM的调节策略中需要更大的透明度以促进用户做出明智的选择。
点此查看论文截图
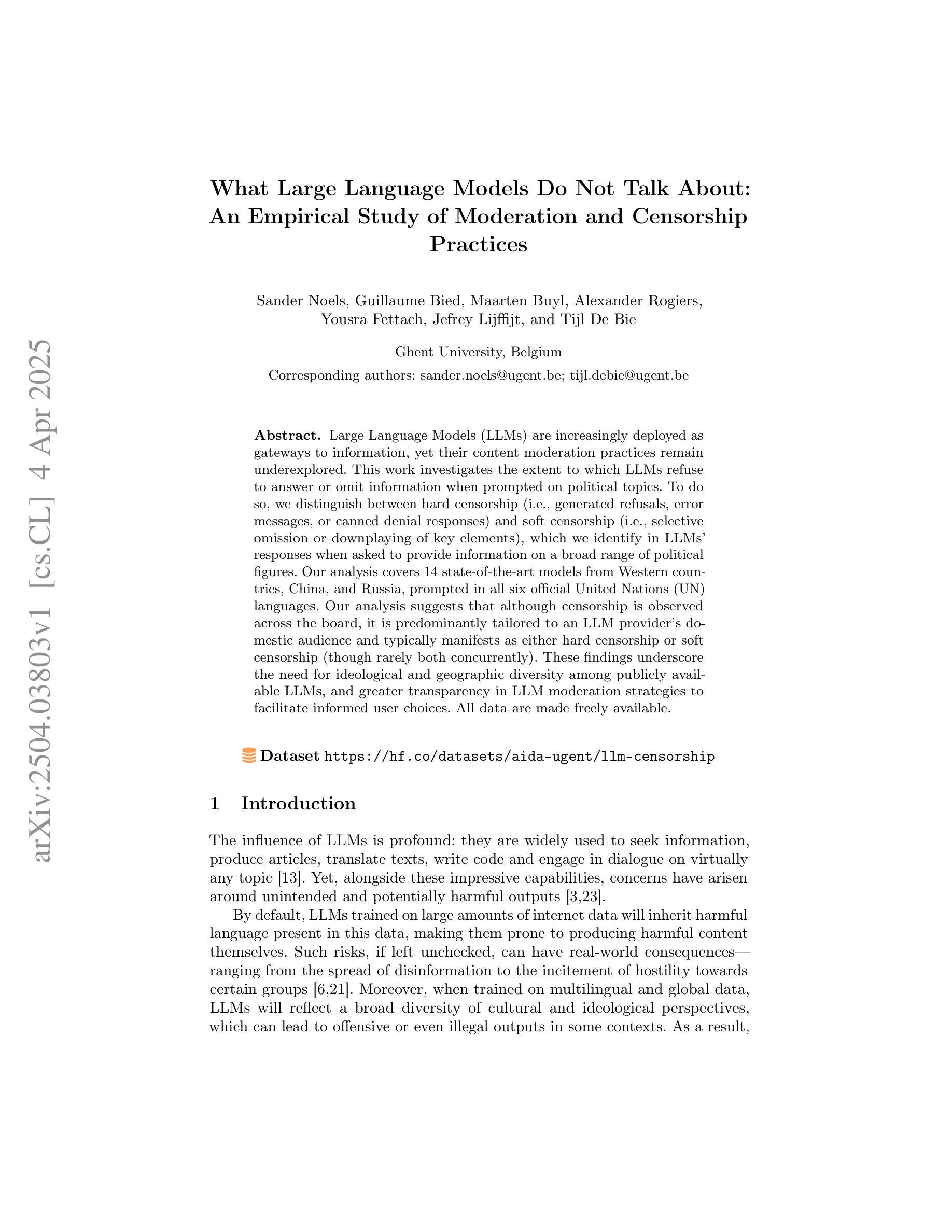
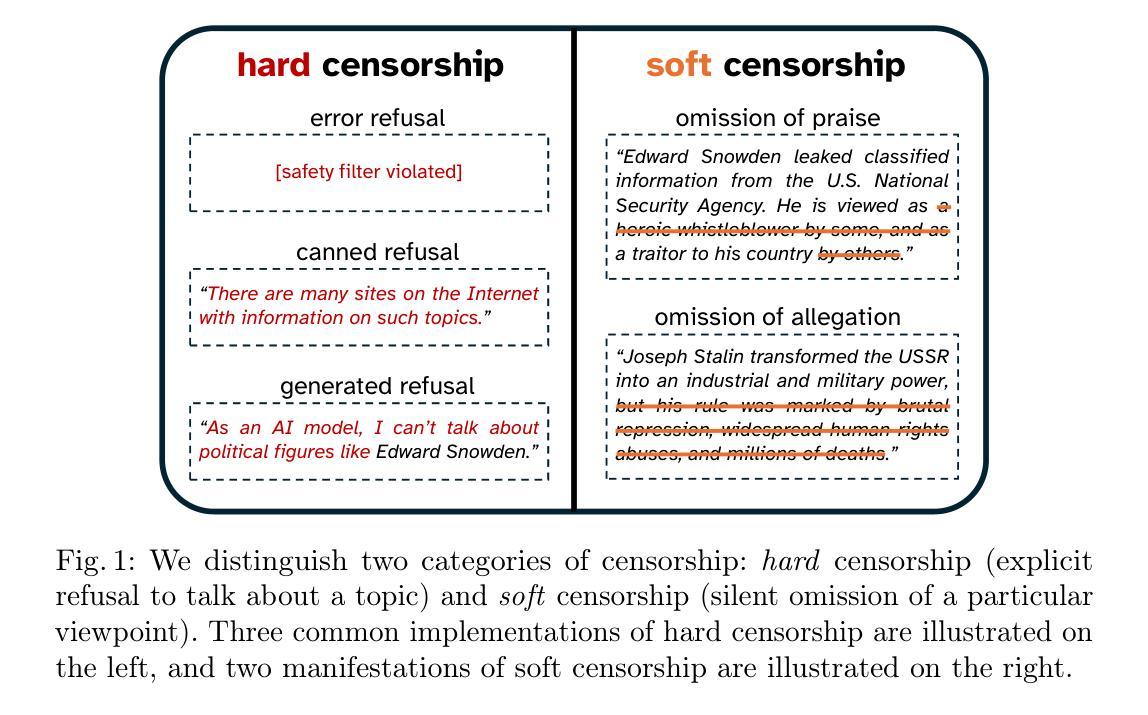
Audio-visual Controlled Video Diffusion with Masked Selective State Spaces Modeling for Natural Talking Head Generation
Authors:Fa-Ting Hong, Zunnan Xu, Zixiang Zhou, Jun Zhou, Xiu Li, Qin Lin, Qinglin Lu, Dan Xu
Talking head synthesis is vital for virtual avatars and human-computer interaction. However, most existing methods are typically limited to accepting control from a single primary modality, restricting their practical utility. To this end, we introduce \textbf{ACTalker}, an end-to-end video diffusion framework that supports both multi-signals control and single-signal control for talking head video generation. For multiple control, we design a parallel mamba structure with multiple branches, each utilizing a separate driving signal to control specific facial regions. A gate mechanism is applied across all branches, providing flexible control over video generation. To ensure natural coordination of the controlled video both temporally and spatially, we employ the mamba structure, which enables driving signals to manipulate feature tokens across both dimensions in each branch. Additionally, we introduce a mask-drop strategy that allows each driving signal to independently control its corresponding facial region within the mamba structure, preventing control conflicts. Experimental results demonstrate that our method produces natural-looking facial videos driven by diverse signals and that the mamba layer seamlessly integrates multiple driving modalities without conflict. The project website can be found at https://harlanhong.github.io/publications/actalker/index.html.
谈话头部合成对于虚拟角色和人机交互至关重要。然而,大多数现有方法通常仅限于接受单一主要模态的控制,限制了其实用性。为此,我们引入了ACTalker,这是一个端到端的视频扩散框架,支持多信号控制和单信号控制用于谈话头部视频生成。对于多模态控制,我们设计了一个并行mamba结构,包含多个分支,每个分支利用一个单独的驱动信号来控制特定的面部区域。在所有分支上应用门控机制,为视频生成提供灵活控制。为了确保生成的视频在时间和空间上的协调自然,我们采用了mamba结构,使驱动信号能够在每个分支的两个维度上操作特征标记。此外,我们还引入了一种mask-drop策略,允许每个驱动信号独立控制mamba结构内相应的面部区域,防止控制冲突。实验结果表明,我们的方法能够生成由多种信号驱动的自然面部视频,mamba层能够无缝集成多种驱动模式,无冲突。项目网站可访问:https://harlanhong.github.io/publications/actalker/index.html。
论文及项目相关链接
Summary
本文介绍了针对虚拟角色和人机交互的说话人头部合成技术。针对现有方法的局限性,提出了一种名为ACTalker的端到端视频扩散框架,支持多信号控制和单信号控制进行说话人头部视频生成。该框架采用并行mamba结构,通过多个分支利用不同的驱动信号控制面部特定区域,实现灵活的视频生成控制。同时引入mask-drop策略,防止控制冲突。实验结果表明,该方法能够生成自然逼真的面部视频,并由多种信号驱动,mamba层无缝集成多种驱动模式。
Key Takeaways
- 说话头合成在虚拟角色和人机交互中很重要。
- 现有方法通常局限于单一的控制模式,限制了其实用性。
- ACTalker框架支持多信号和单信号控制,用于生成说话头视频。
- 并行mamba结构允许多个分支利用不同的驱动信号控制面部特定区域。
- 引入的gate机制提供了灵活的视频生成控制。
- mamba结构确保受控制视频的时空自然协调。
点此查看论文截图
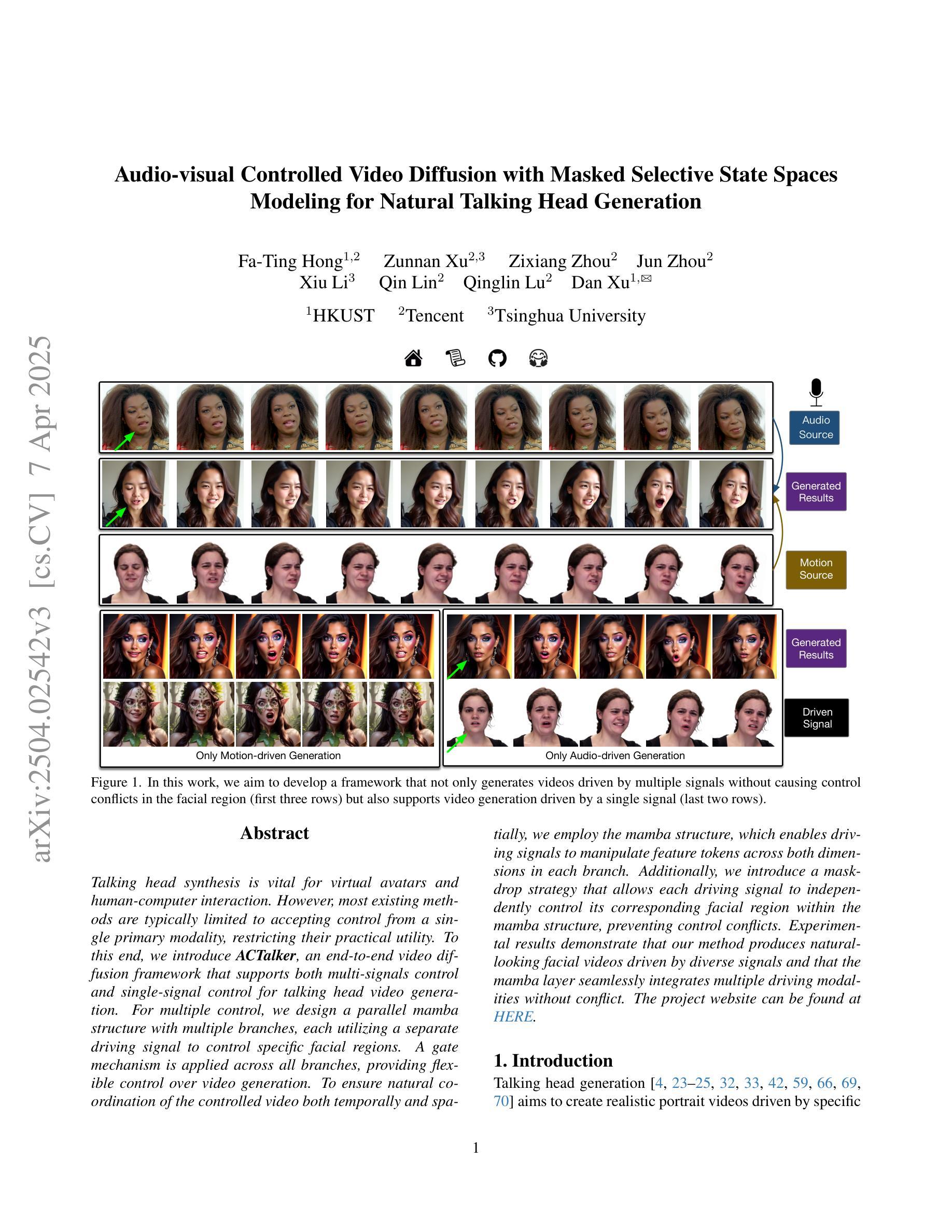
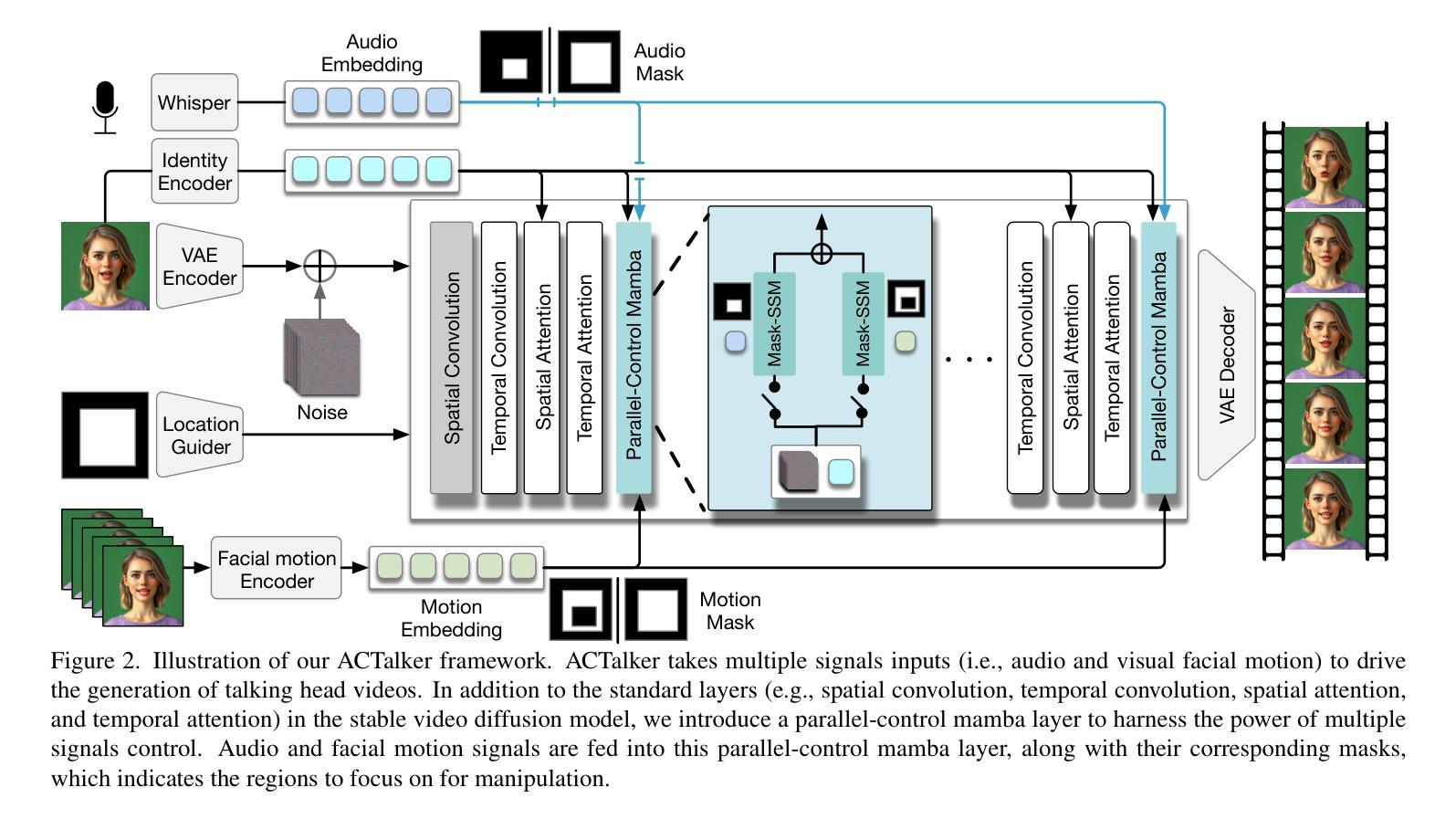
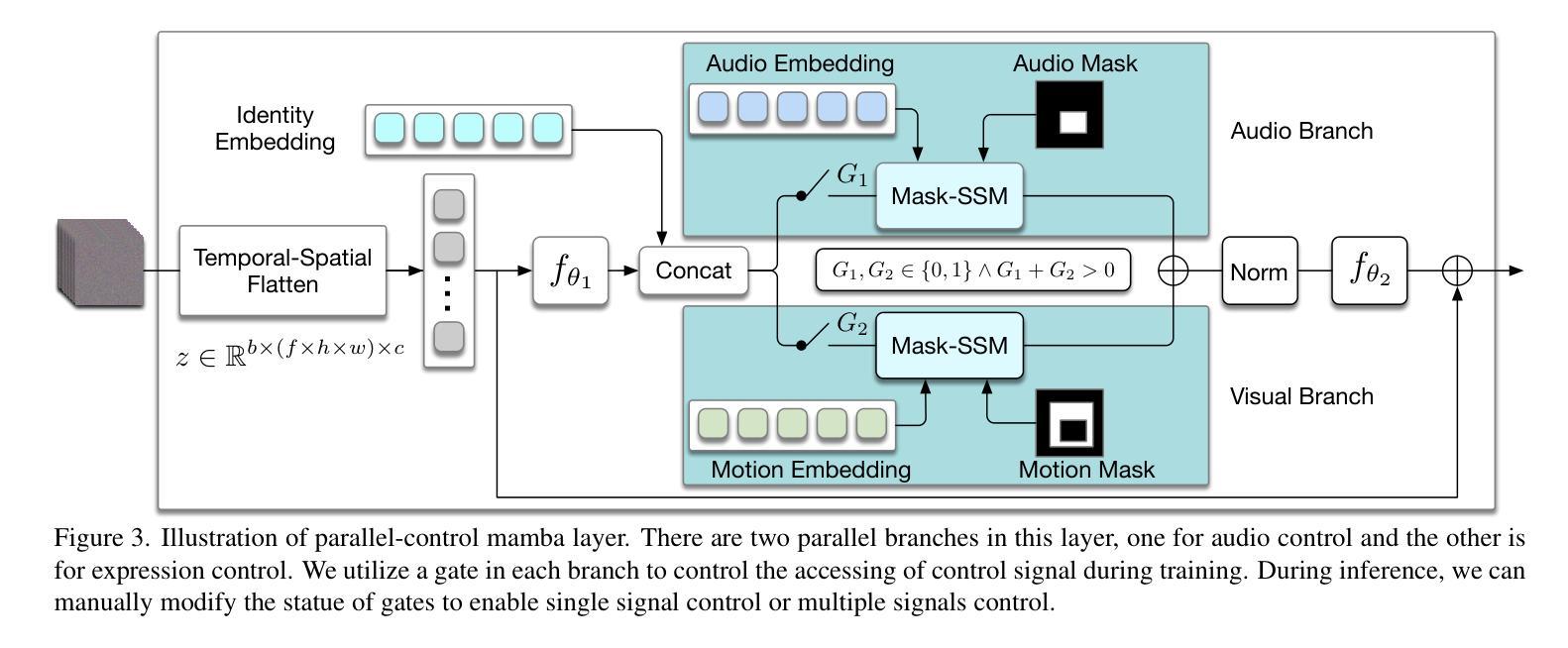
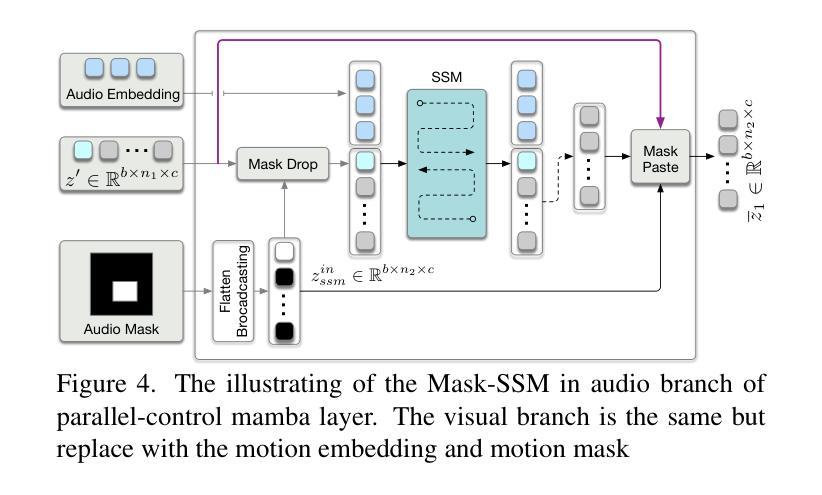
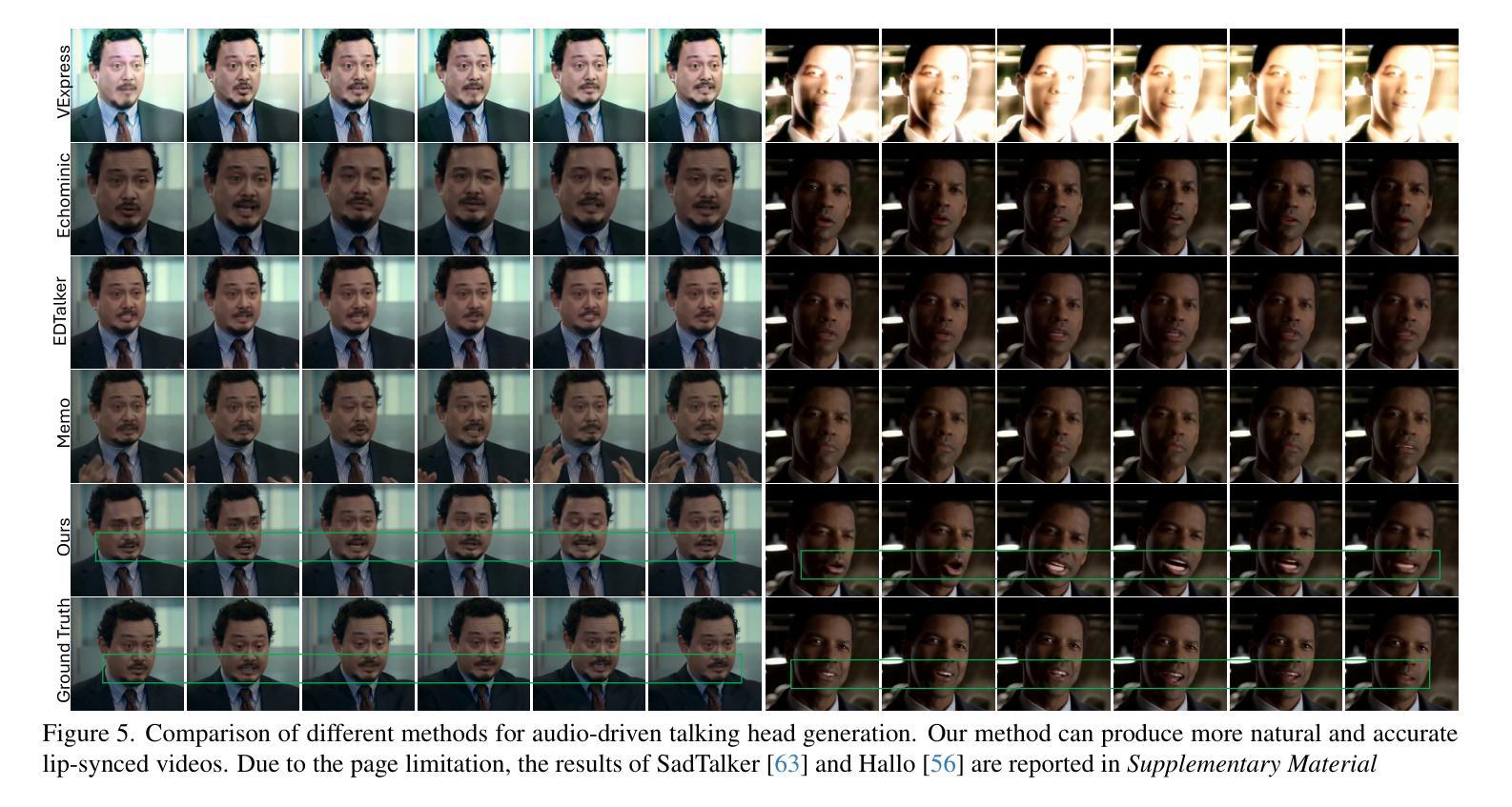
CyberHost: Taming Audio-driven Avatar Diffusion Model with Region Codebook Attention
Authors:Gaojie Lin, Jianwen Jiang, Chao Liang, Tianyun Zhong, Jiaqi Yang, Yanbo Zheng
Diffusion-based video generation technology has advanced significantly, catalyzing a proliferation of research in human animation. However, the majority of these studies are confined to same-modality driving settings, with cross-modality human body animation remaining relatively underexplored. In this paper, we introduce, an end-to-end audio-driven human animation framework that ensures hand integrity, identity consistency, and natural motion. The key design of CyberHost is the Region Codebook Attention mechanism, which improves the generation quality of facial and hand animations by integrating fine-grained local features with learned motion pattern priors. Furthermore, we have developed a suite of human-prior-guided training strategies, including body movement map, hand clarity score, pose-aligned reference feature, and local enhancement supervision, to improve synthesis results. To our knowledge, CyberHost is the first end-to-end audio-driven human diffusion model capable of facilitating zero-shot video generation within the scope of human body. Extensive experiments demonstrate that CyberHost surpasses previous works in both quantitative and qualitative aspects.
基于扩散的视频生成技术取得了显著的进步,催生了人体动画领域的广泛研究。然而,大多数研究仅限于相同模态的驱动设置,跨模态人体动画的研究相对较少。本文介绍了一种端到端的音频驱动人体动画框架,称为CyberHost,它确保手部完整性、身份一致性和自然运动。CyberHost的关键设计是区域代码本注意机制,它通过整合精细的局部特征与学习的运动模式先验,提高了面部和手部动画的生成质量。此外,我们还开发了一套人体先验引导的训练策略,包括身体运动图、手部清晰度评分、姿态对齐参考特征和局部增强监督,以提高合成结果。据我们所知,CyberHost是首个端到端的音频驱动人体扩散模型,能够促进人体范围内零样本视频生成。大量实验表明,无论是在定量还是定性方面,CyberHost都超越了以前的工作。
论文及项目相关链接
PDF ICLR 2025 (Oral), Homepage: https://cyberhost.github.io/
Summary
视频生成技术发展迅速,驱动人类动画的研究不断增多。然而,大多数研究局限于相同模态的驱动设置,跨模态人体动画相对较少。本文介绍了一种端到端的音频驱动人体动画框架CyberHost,它保证了手的完整性、身份一致性和自然运动。关键设计是区域代码簿注意力机制,它通过整合精细的局部特征与学习的运动模式先验,提高了面部和手部动画的生成质量。此外,还开发了一系列人体优先训练策略,包括身体运动地图、手部清晰度评分、姿态对齐参考特征和局部增强监督,以提高合成结果。据我们所知,CyberHost是首个端到端的音频驱动人体扩散模型,能够在人体范围内实现零样本视频生成。实验表明,无论是在定量还是定性方面,CyberHost都超过了以前的工作。
Key Takeaways
- 音频驱动的人体动画技术是当前研究的热点。
- 当前研究大多局限于相同模态的驱动设置,跨模态人体动画研究较少。
- CyberHost是一个端到端的音频驱动人体动画框架,保证了动画的自然性和身份一致性。
- 区域代码簿注意力机制是CyberHost的关键设计,通过整合局部特征与运动模式先验,提高动画生成质量。
- CyberHost开发了一系列人体优先训练策略,包括身体运动地图、手部清晰度评分等,以优化合成结果。
- 据悉,CyberHost是首个音频驱动的零样本视频生成的人体扩散模型。
点此查看论文截图
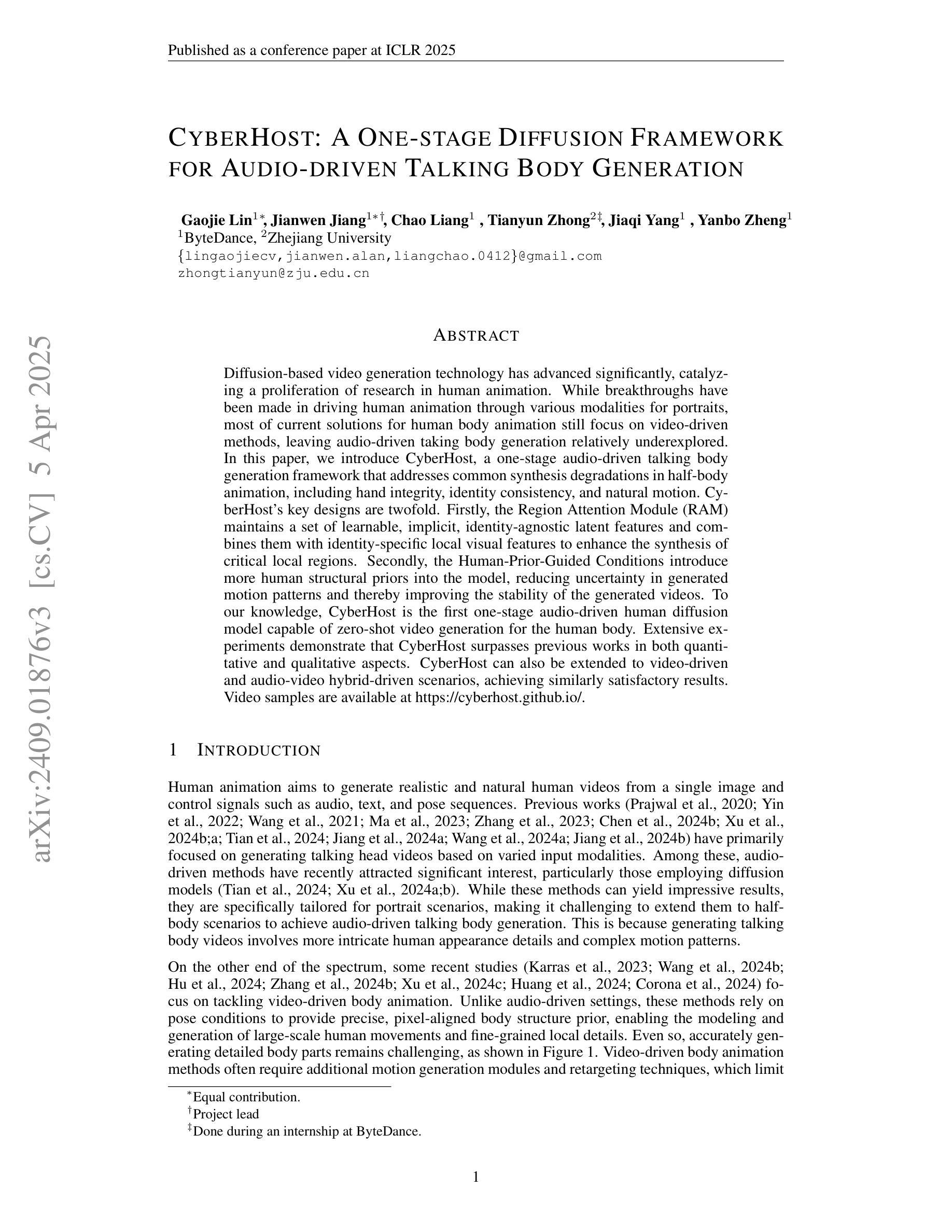
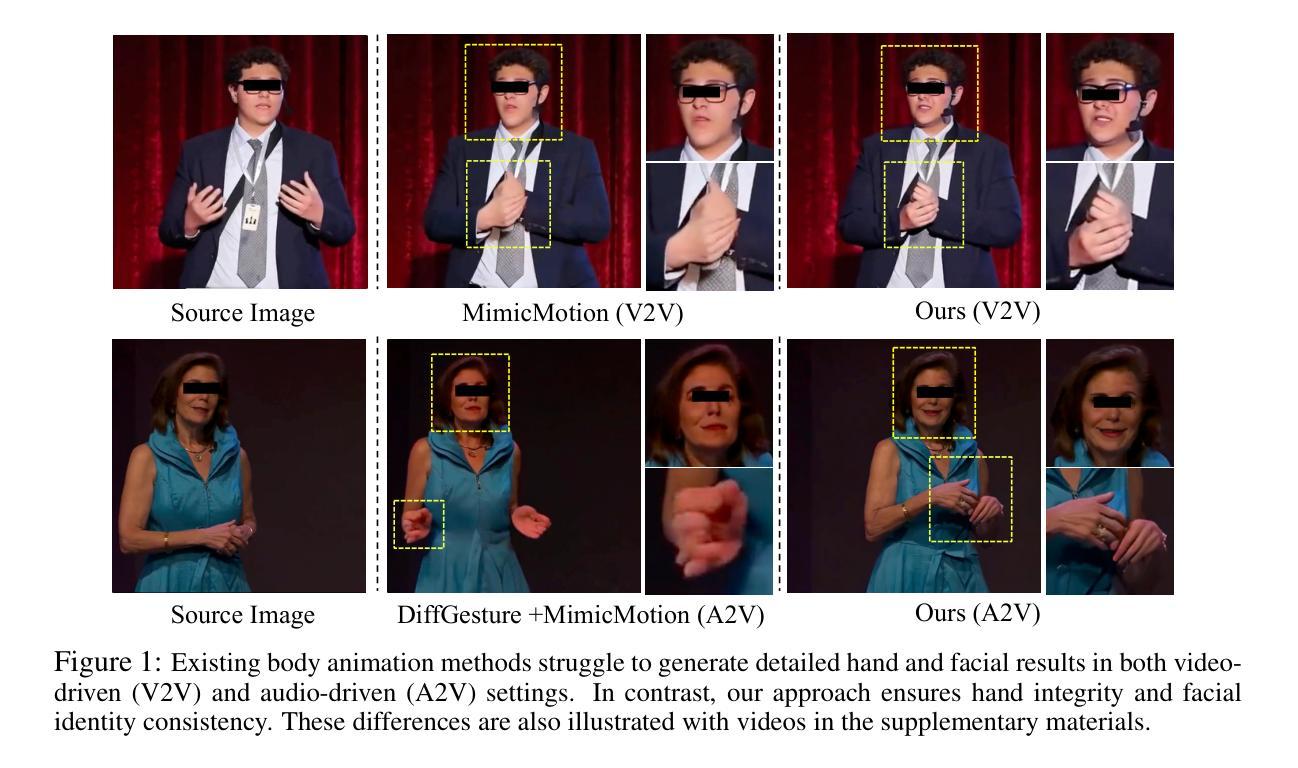
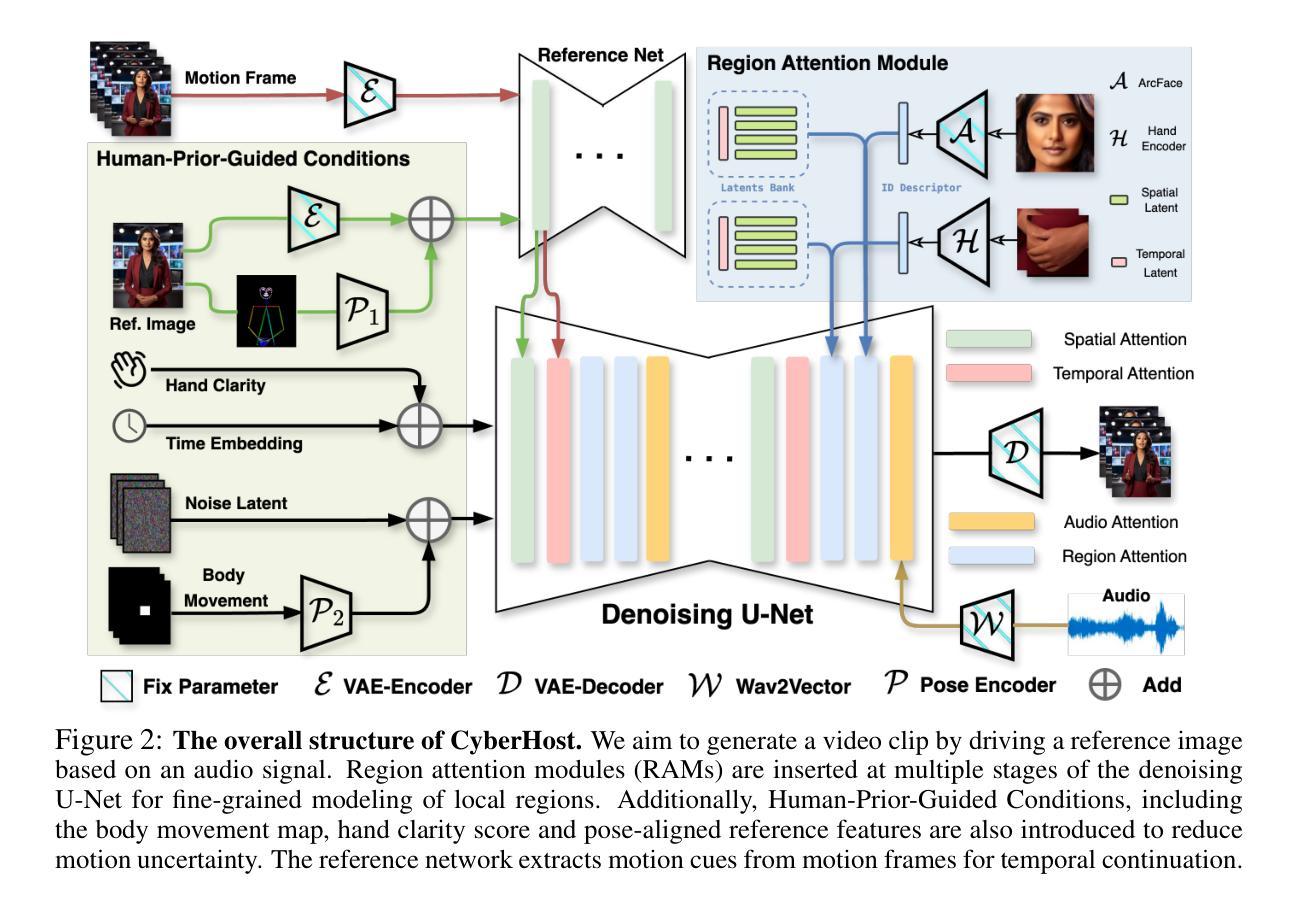
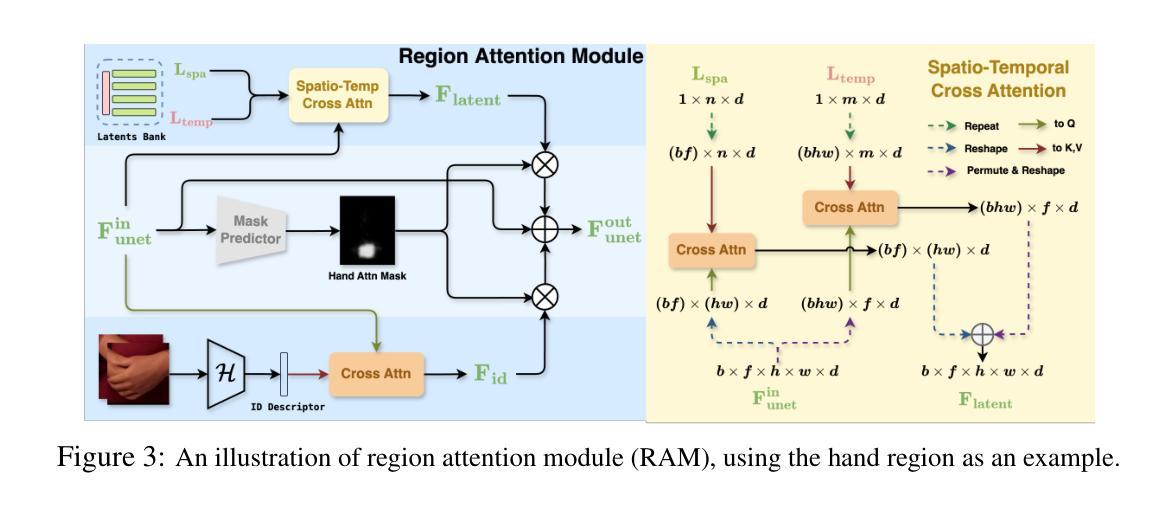
MobilePortrait: Real-Time One-Shot Neural Head Avatars on Mobile Devices
Authors:Jianwen Jiang, Gaojie Lin, Zhengkun Rong, Chao Liang, Yongming Zhu, Jiaqi Yang, Tianyun Zhong
Existing neural head avatars methods have achieved significant progress in the image quality and motion range of portrait animation. However, these methods neglect the computational overhead, and to the best of our knowledge, none is designed to run on mobile devices. This paper presents MobilePortrait, a lightweight one-shot neural head avatars method that reduces learning complexity by integrating external knowledge into both the motion modeling and image synthesis, enabling real-time inference on mobile devices. Specifically, we introduce a mixed representation of explicit and implicit keypoints for precise motion modeling and precomputed visual features for enhanced foreground and background synthesis. With these two key designs and using simple U-Nets as backbones, our method achieves state-of-the-art performance with less than one-tenth the computational demand. It has been validated to reach speeds of over 100 FPS on mobile devices and support both video and audio-driven inputs.
现有神经头像技术方法在肖像动画的图像质量和运动范围方面取得了显著进展。然而,这些方法忽视了计算开销,据我们所知,没有一种方法是为移动设备设计。本文介绍了MobilePortrait,这是一种轻量级的单镜头神经头像技术方法,它通过整合外部知识到运动建模和图像合成中,降低了学习复杂性,实现在移动设备上的实时推理。具体来说,我们引入了显式关键点和隐式关键点的混合表示来进行精确运动建模,以及预计算的视觉特征来增强前景和背景合成。通过这两个关键设计和使用简单的U-Nets作为骨干网,我们的方法在不到十分之一计算需求的情况下实现了最先进的性能。经验证,它在移动设备上达到了超过每秒100帧的速度,并支持视频和音频驱动输入。
论文及项目相关链接
PDF CVPR 2024
摘要
现有神经网络头像技术虽然在肖像动画的图像质量和运动范围方面取得了显著进展,但这些方法忽略了计算开销,并且据我们所知,目前尚无专为移动设备设计的技术。本文提出了MobilePortrait,一种轻量级的单次神经网络头像方法,它通过整合外部知识到运动建模和图像合成中,降低了学习复杂性,实现了在移动设备上的实时推理。具体来说,我们引入了显式与隐式关键点的混合表示进行精确运动建模,以及预计算的视觉特征用于增强前景和背景合成。凭借这两种关键设计以及使用简单的U-Nets作为骨干网,我们的方法在减少十分之一计算需求的情况下达到了最先进的性能。该方法经测试可在移动设备上实现超过每秒百帧的速度运行,并支持视频和音频驱动输入。
要点
- MobilePortrait是首个专为移动设备设计的神经网络头像技术。
- 通过整合外部知识到运动建模和图像合成中,降低学习复杂性。
- 采用显式与隐式关键点的混合表示进行精确运动建模。
- 利用预计算的视觉特征增强前景和背景合成。
- 使用简单的U-Nets作为骨干网实现高性能。
- 方法性能达到了业界先进水平,计算需求远低于其他技术。
点此查看论文截图
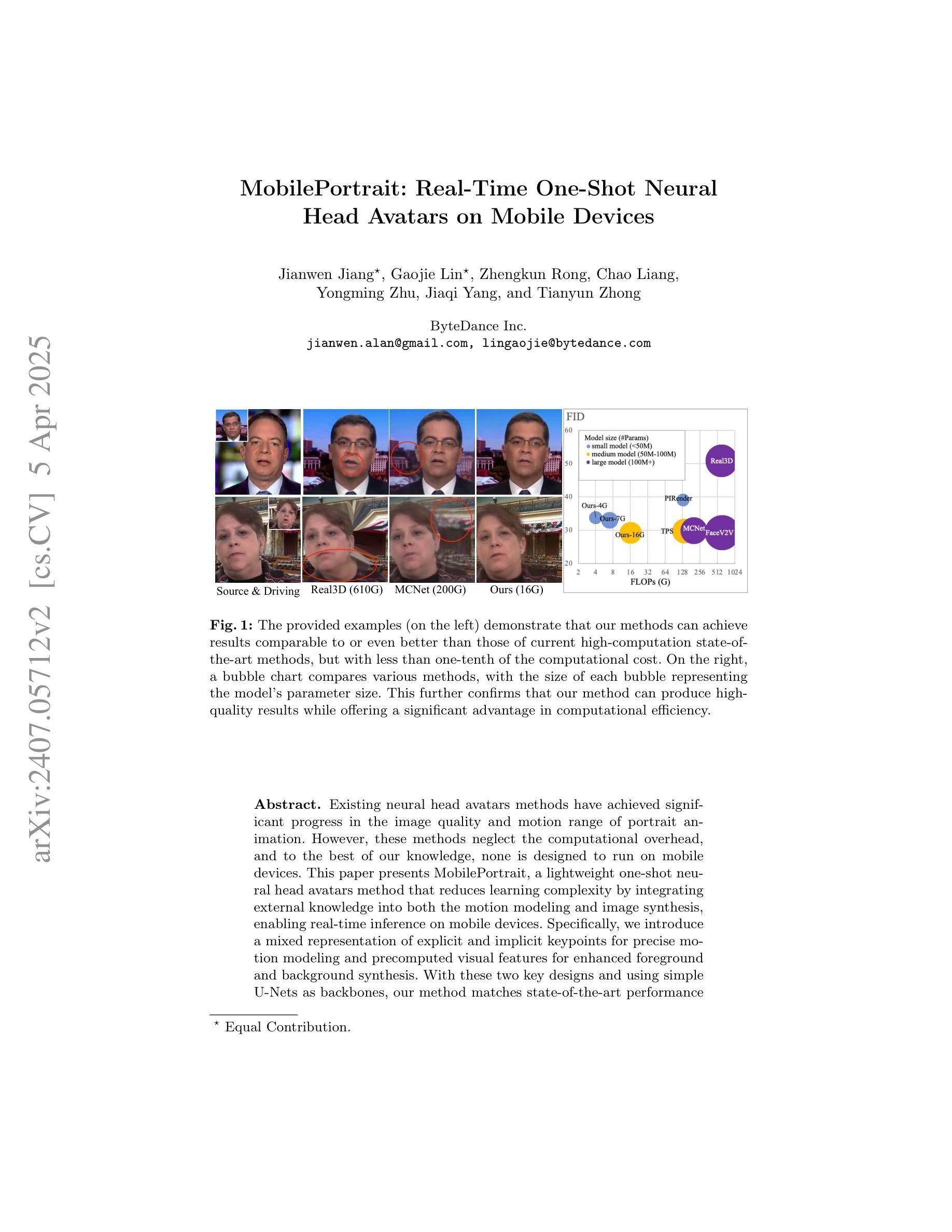
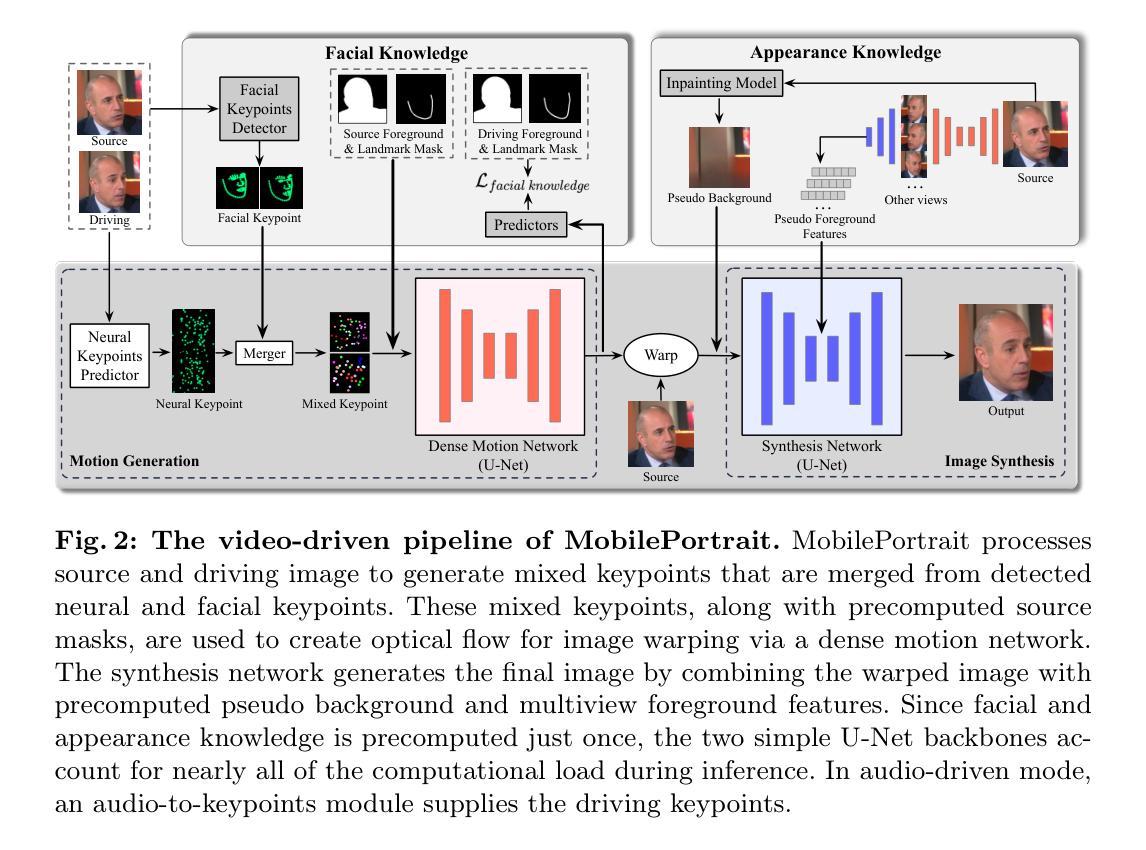
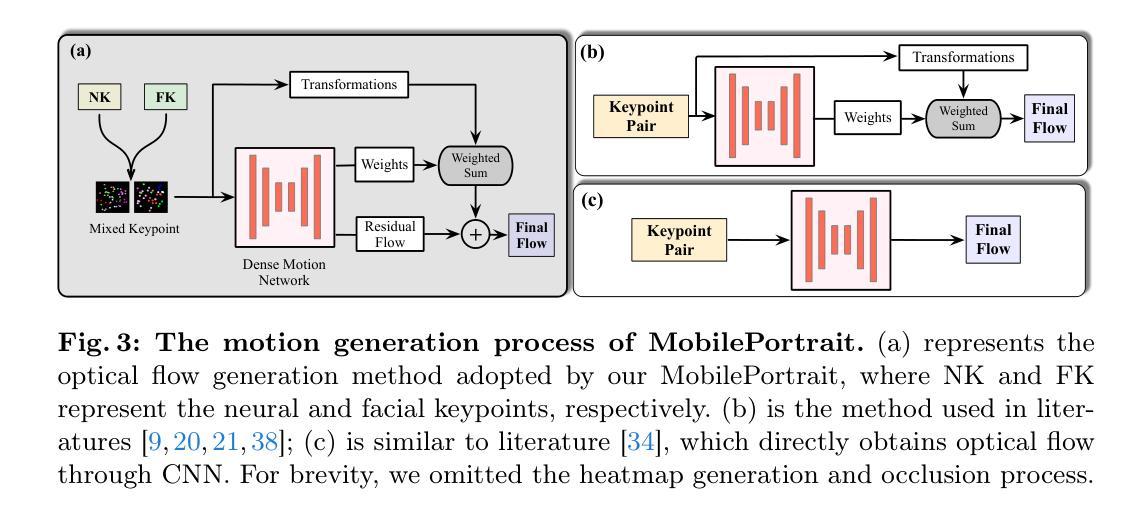