⚠️ 以下所有内容总结都来自于 大语言模型的能力,如有错误,仅供参考,谨慎使用
🔴 请注意:千万不要用于严肃的学术场景,只能用于论文阅读前的初筛!
💗 如果您觉得我们的项目对您有帮助 ChatPaperFree ,还请您给我们一些鼓励!⭐️ HuggingFace免费体验
2025-04-09 更新
DanceMosaic: High-Fidelity Dance Generation with Multimodal Editability
Authors:Foram Niravbhai Shah, Parshwa Shah, Muhammad Usama Saleem, Ekkasit Pinyoanuntapong, Pu Wang, Hongfei Xue, Ahmed Helmy
Recent advances in dance generation have enabled automatic synthesis of 3D dance motions. However, existing methods still struggle to produce high-fidelity dance sequences that simultaneously deliver exceptional realism, precise dance-music synchronization, high motion diversity, and physical plausibility. Moreover, existing methods lack the flexibility to edit dance sequences according to diverse guidance signals, such as musical prompts, pose constraints, action labels, and genre descriptions, significantly restricting their creative utility and adaptability. Unlike the existing approaches, DanceMosaic enables fast and high-fidelity dance generation, while allowing multimodal motion editing. Specifically, we propose a multimodal masked motion model that fuses the text-to-motion model with music and pose adapters to learn probabilistic mapping from diverse guidance signals to high-quality dance motion sequences via progressive generative masking training. To further enhance the motion generation quality, we propose multimodal classifier-free guidance and inference-time optimization mechanism that further enforce the alignment between the generated motions and the multimodal guidance. Extensive experiments demonstrate that our method establishes a new state-of-the-art performance in dance generation, significantly advancing the quality and editability achieved by existing approaches.
舞蹈生成领域的最新进展已经实现了3D舞蹈动作的自动合成。然而,现有方法仍然难以生成高保真度的舞蹈序列,难以同时实现卓越的真实性、精确的舞蹈音乐同步、高度的动作多样性和物理可行性。此外,现有方法缺乏根据多种指导信号编辑舞蹈序列的灵活性,如音乐提示、姿势约束、动作标签和风格描述,这极大地限制了其创意实用性和适应性。与现有方法不同,DanceMosaic能够实现快速和高保真的舞蹈生成,同时支持多种模式的动作编辑。具体来说,我们提出了一种多模式掩模运动模型,该模型将文本到运动模型与音乐和姿势适配器融合,通过渐进的生成掩模训练,学习从多种指导信号到高质量舞蹈运动序列的概率映射。为了进一步提高运动生成质量,我们提出了多模式无分类器引导和推理时间优化机制,进一步强化了生成动作与多模式指导之间的对齐。大量实验表明,我们的方法在舞蹈生成方面达到了新的最先进的性能,显著提高了现有方法所实现的质量和可编辑性。
论文及项目相关链接
Summary
本文介绍了DanceMosaic在舞蹈生成领域的最新进展。该方法能够快速地生成高保真度的舞蹈序列,并允许多模态运动编辑。通过提出一种多模态掩模运动模型,融合了文本到运动的模型,与音乐和姿态适配器相结合,通过渐进的生成掩模训练,学习从多种指导信号到高质量舞蹈运动序列的概率映射。为进一步提高运动生成质量,还提出了多模态无分类器指导和推理时间优化机制,进一步强化生成运动与多模态指导之间的对齐。实验证明,该方法在舞蹈生成方面达到了新的技术水平,显著提高了现有方法的品质和可编辑性。
Key Takeaways
- DanceMosaic实现了快速且高保真度的舞蹈生成。
- 现有方法在生成同时满足真实感、精确的音乐同步、高运动多样性和物理可行性的舞蹈序列时仍有困难。
- DanceMosaic通过多模态掩模运动模型融合文本到运动的模型、音乐和姿态适配器。
- 该方法通过渐进的生成掩模训练学习概率映射,从多样的指导信号到高质量的舞蹈运动序列。
- 多模态无分类器指导和推理时间优化机制用于提高运动生成质量,并强化生成运动与多模态指导之间的对齐。
- DanceMosaic方法允许根据多种指导信号(如音乐提示、姿态约束、动作标签和风格描述)进行舞蹈序列的编辑,增加了其创造性和适应性。
点此查看论文截图
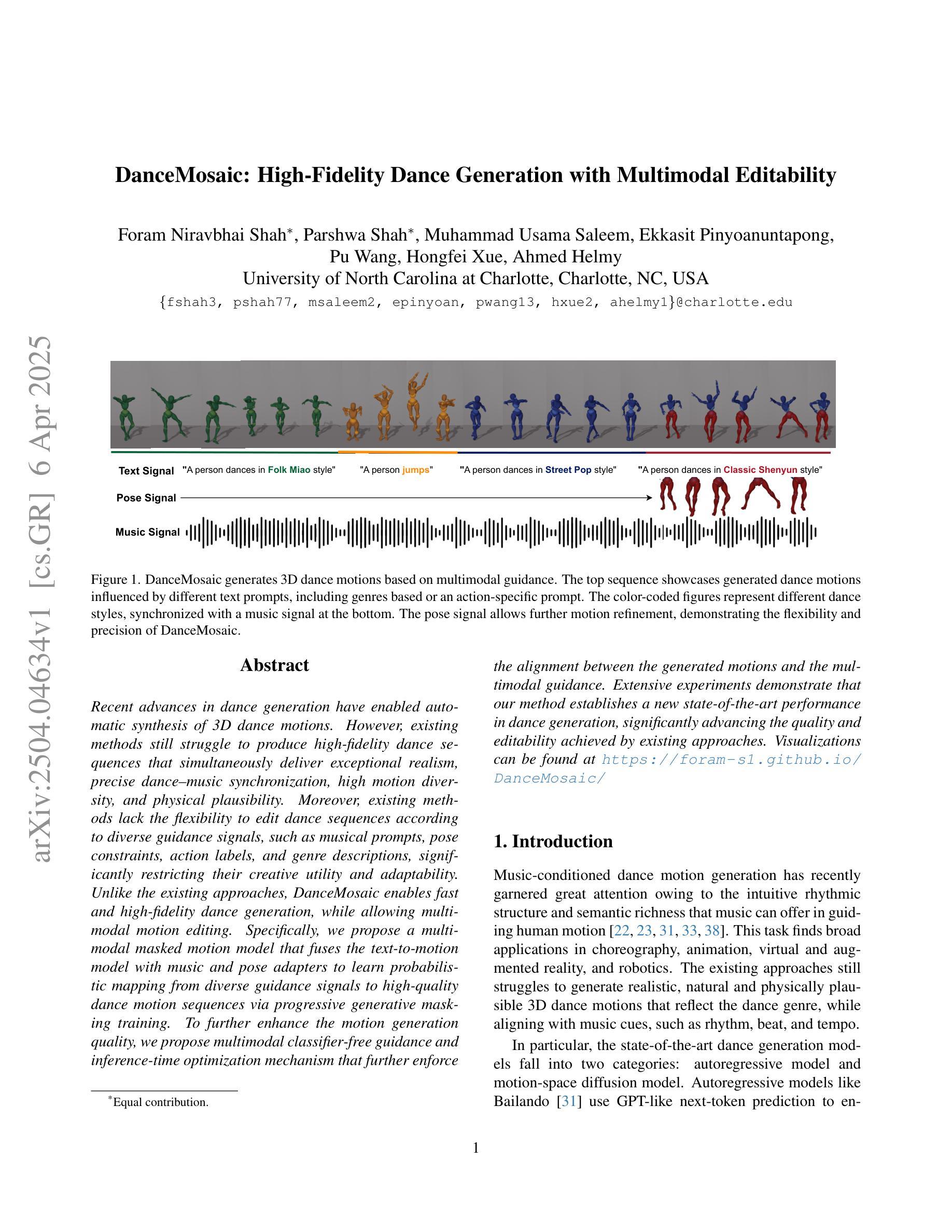
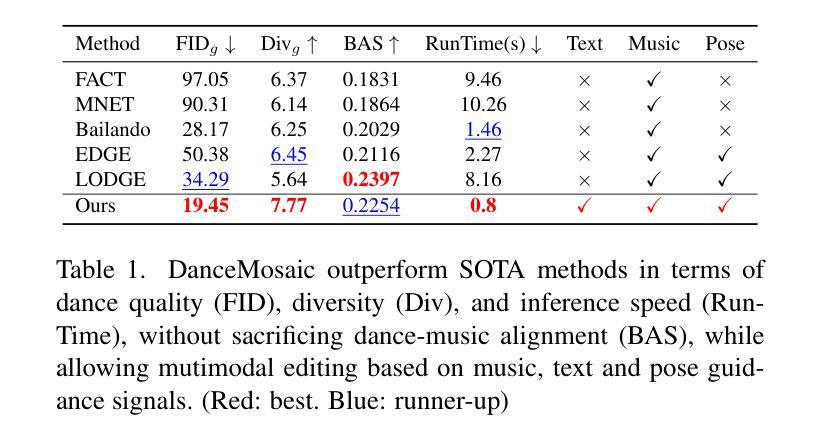
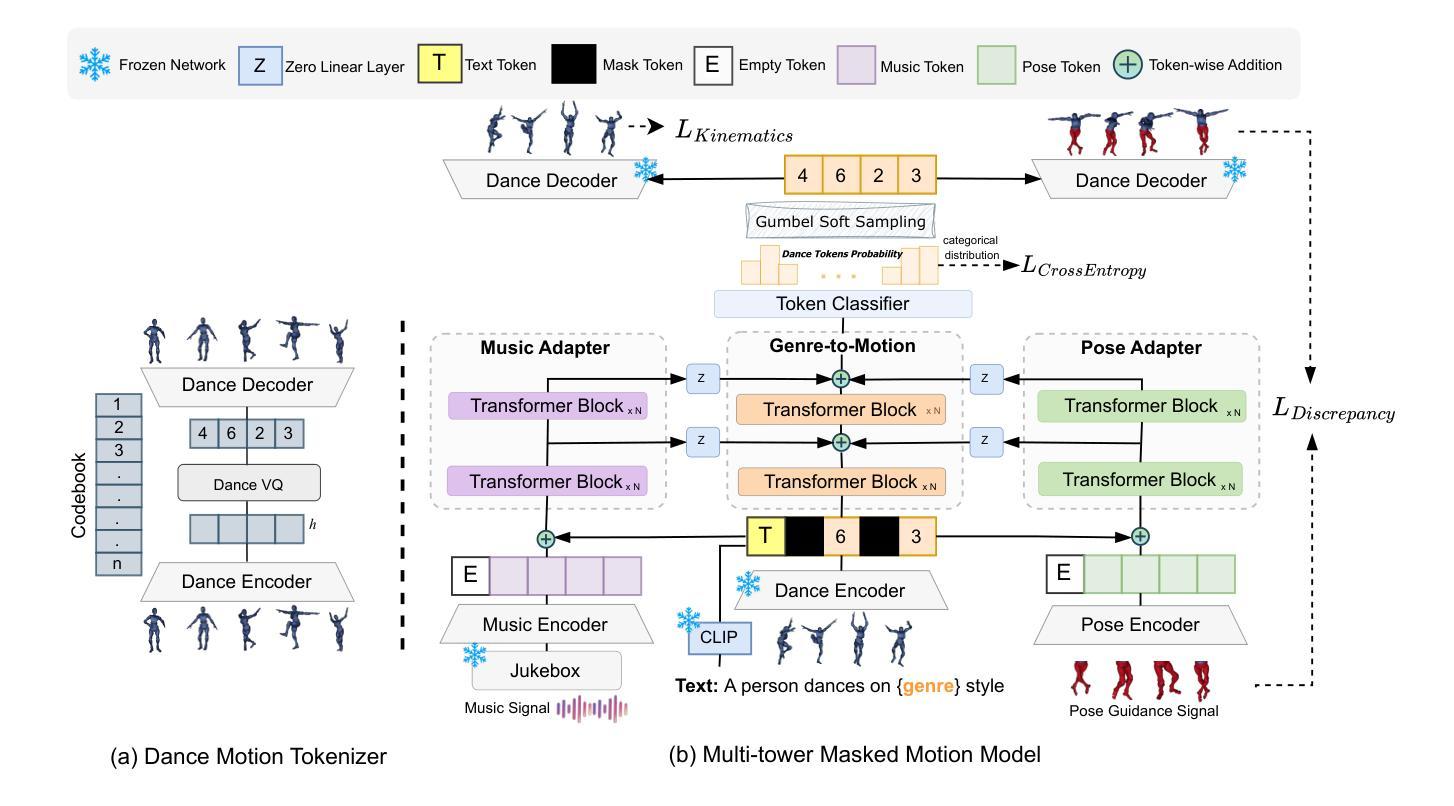
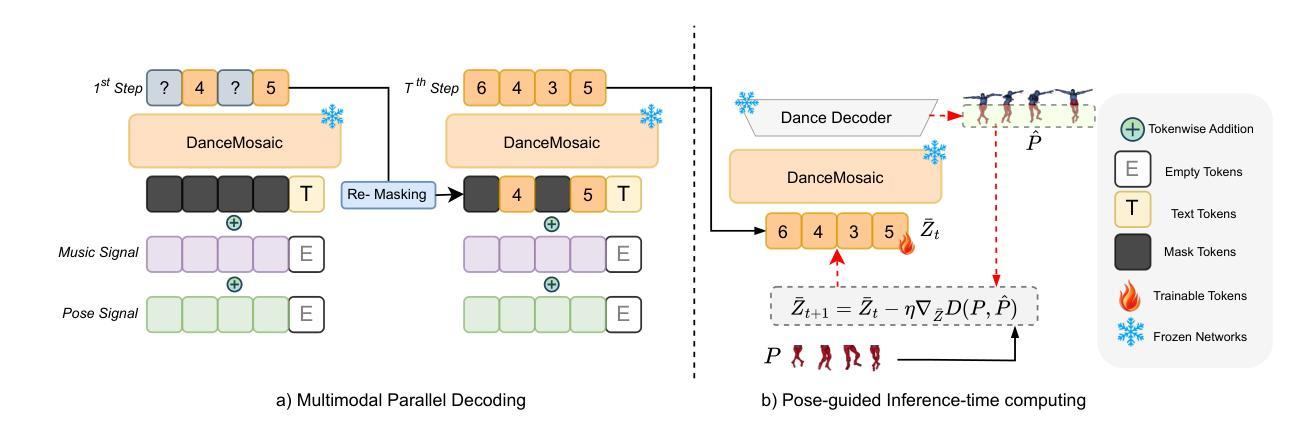
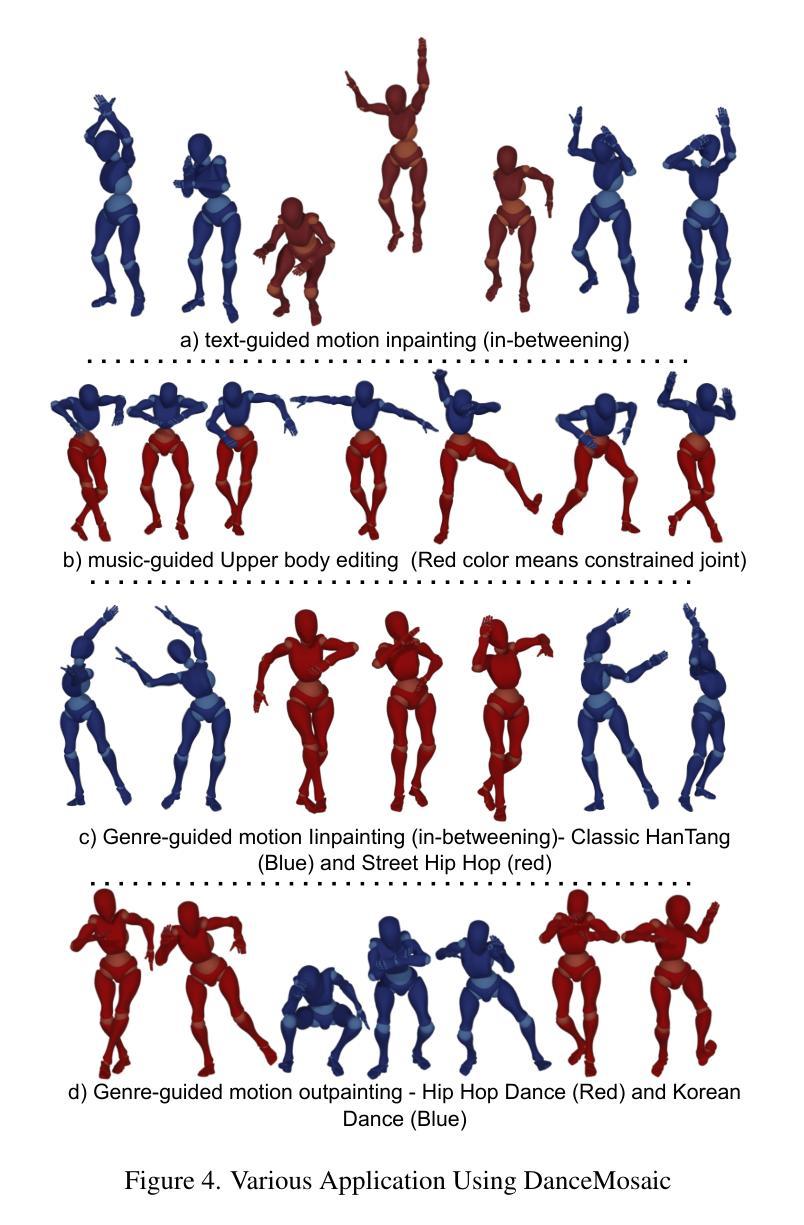