⚠️ 以下所有内容总结都来自于 大语言模型的能力,如有错误,仅供参考,谨慎使用
🔴 请注意:千万不要用于严肃的学术场景,只能用于论文阅读前的初筛!
💗 如果您觉得我们的项目对您有帮助 ChatPaperFree ,还请您给我们一些鼓励!⭐️ HuggingFace免费体验
2025-04-10 更新
MobilePortrait: Real-Time One-Shot Neural Head Avatars on Mobile Devices
Authors:Jianwen Jiang, Gaojie Lin, Zhengkun Rong, Chao Liang, Yongming Zhu, Jiaqi Yang, Tianyun Zhong
Existing neural head avatars methods have achieved significant progress in the image quality and motion range of portrait animation. However, these methods neglect the computational overhead, and to the best of our knowledge, none is designed to run on mobile devices. This paper presents MobilePortrait, a lightweight one-shot neural head avatars method that reduces learning complexity by integrating external knowledge into both the motion modeling and image synthesis, enabling real-time inference on mobile devices. Specifically, we introduce a mixed representation of explicit and implicit keypoints for precise motion modeling and precomputed visual features for enhanced foreground and background synthesis. With these two key designs and using simple U-Nets as backbones, our method achieves state-of-the-art performance with less than one-tenth the computational demand. It has been validated to reach speeds of over 100 FPS on mobile devices and support both video and audio-driven inputs.
现有的神经头像方法已经在肖像动画的图像质量和运动范围方面取得了显著进展。然而,这些方法忽视了计算开销,而且据我们所知,没有设计用于移动设备运行的方法。本文介绍了MobilePortrait,这是一种轻量级的单镜头神经头像方法,它通过整合外部知识到运动建模和图像合成中,降低了学习复杂性,实现在移动设备上的实时推理。具体来说,我们引入了显式关键点和隐式关键点的混合表示来进行精确运动建模,以及预计算的视觉特征来增强前景和背景合成。通过这两个关键设计和使用简单的U-Nets作为骨干网,我们的方法在不到十分之一计算需求的情况下达到了最先进的性能。经验证,它在移动设备上的速度超过100帧/秒,并支持视频和音频驱动输入。
论文及项目相关链接
PDF CVPR 2025
Summary
基于移动设备的轻量级单镜头神经网络头部头像技术。通过整合外部知识到运动建模和图像合成中,降低学习复杂度,实现移动设备上的实时推理。采用明确的隐关键点混合表示,提高运动建模精度;利用预计算视觉特征增强前景和背景合成。使用简单的U-Nets作为骨干网,该方法在计算需求不到十分之一的情况下实现了业界领先性能,在移动设备上速度超过每秒100帧,支持视频和音频驱动输入。
Key Takeaways
- 现有神经网络头像技术在图像质量和运动范围方面取得了显著进展,但忽视了计算开销,且没有设计用于移动设备的方法。
- MobilePortrait是一个轻量级的单镜头神经网络头像方法,旨在降低学习复杂度并适应移动设备。
- MobilePortrait通过整合外部知识到运动建模和图像合成中来实现其高效性能。
- 采用混合表示法,包括明确的隐关键点,以提高运动建模的精确度。
- 利用预计算的视觉特征增强前景和背景的合成。
- 使用简单的U-Nets作为骨干网,实现了高性能,同时减少了计算需求。
点此查看论文截图
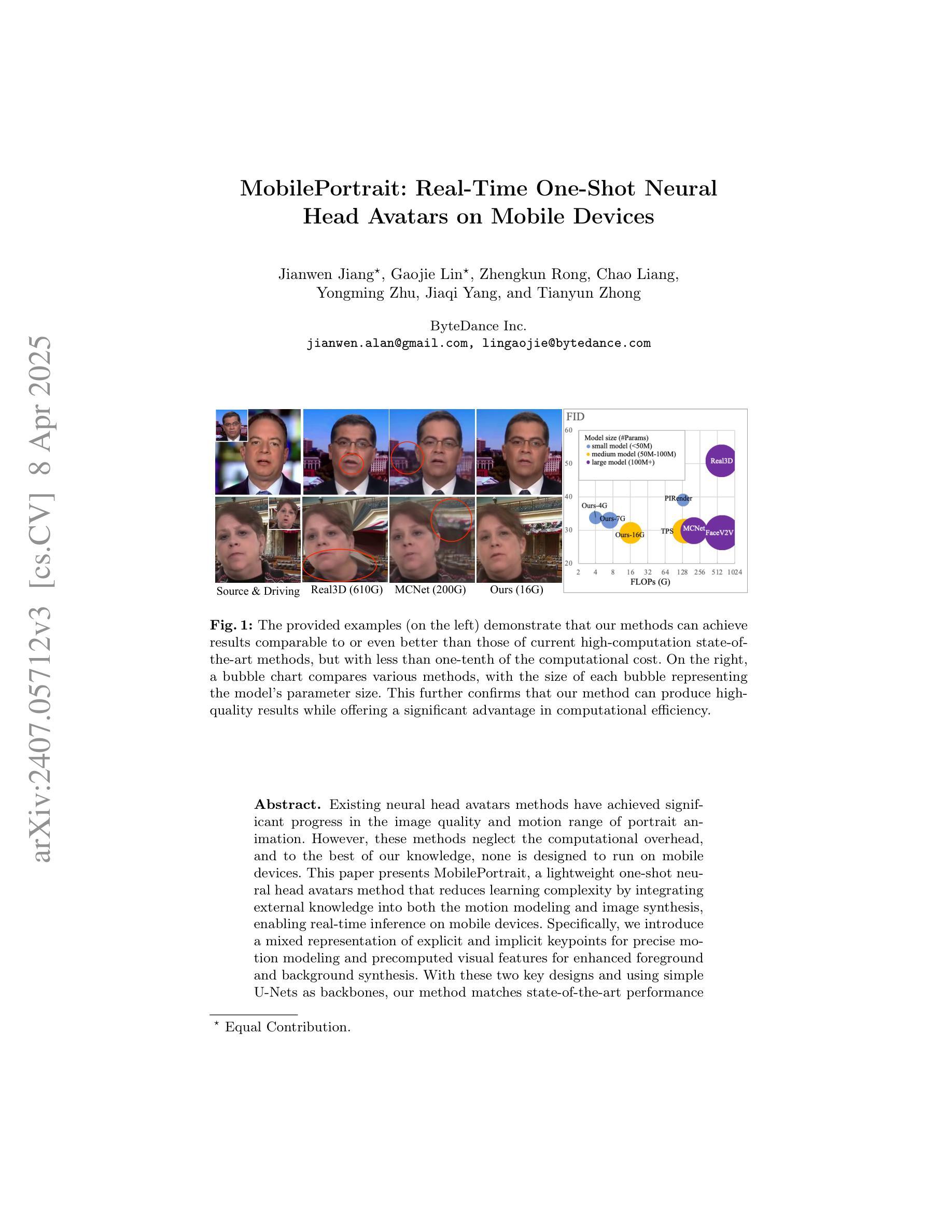
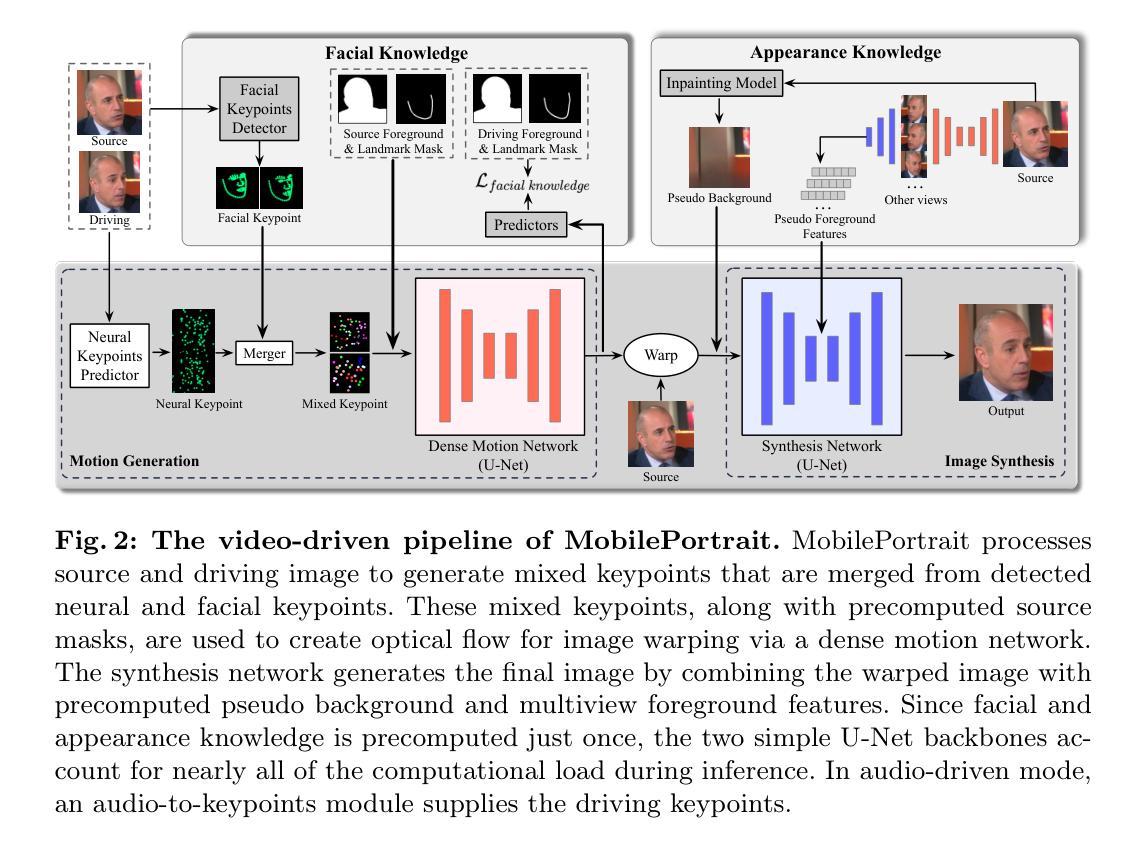
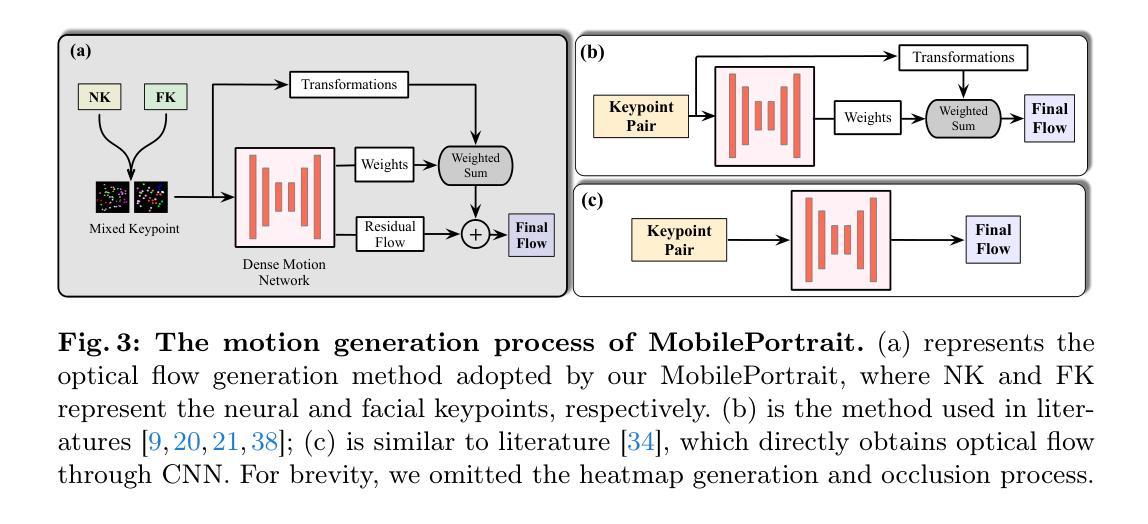