⚠️ 以下所有内容总结都来自于 大语言模型的能力,如有错误,仅供参考,谨慎使用
🔴 请注意:千万不要用于严肃的学术场景,只能用于论文阅读前的初筛!
💗 如果您觉得我们的项目对您有帮助 ChatPaperFree ,还请您给我们一些鼓励!⭐️ HuggingFace免费体验
2025-04-10 更新
Flash Sculptor: Modular 3D Worlds from Objects
Authors:Yujia Hu, Songhua Liu, Xingyi Yang, Xinchao Wang
Existing text-to-3D and image-to-3D models often struggle with complex scenes involving multiple objects and intricate interactions. Although some recent attempts have explored such compositional scenarios, they still require an extensive process of optimizing the entire layout, which is highly cumbersome if not infeasible at all. To overcome these challenges, we propose Flash Sculptor in this paper, a simple yet effective framework for compositional 3D scene/object reconstruction from a single image. At the heart of Flash Sculptor lies a divide-and-conquer strategy, which decouples compositional scene reconstruction into a sequence of sub-tasks, including handling the appearance, rotation, scale, and translation of each individual instance. Specifically, for rotation, we introduce a coarse-to-fine scheme that brings the best of both worlds–efficiency and accuracy–while for translation, we develop an outlier-removal-based algorithm that ensures robust and precise parameters in a single step, without any iterative optimization. Extensive experiments demonstrate that Flash Sculptor achieves at least a 3 times speedup over existing compositional 3D methods, while setting new benchmarks in compositional 3D reconstruction performance. Codes are available at https://github.com/YujiaHu1109/Flash-Sculptor.
现有的文本到3D和图像到3D模型通常在处理涉及多个对象和复杂交互的复杂场景时面临挑战。尽管最近的一些尝试已经探索了这种组合场景,但它们仍然需要优化整个布局的大量过程,这在某些情况下几乎不可行。为了克服这些挑战,我们在本文中提出了Flash Sculptor,这是一个简单有效的框架,可以从单张图像进行组合3D场景/对象重建。Flash Sculptor的核心在于一种分而治之的策略,它将组合场景重建解耦为一系列子任务,包括处理每个实例的外观、旋转、比例和平移。具体来说,对于旋转,我们引入了一种由粗到精的方案,结合了效率和准确性;对于平移,我们开发了一种基于异常值去除的算法,确保在单步中即可获得稳健且精确的参数,无需任何迭代优化。大量实验表明,Flash Sculptor与现有的组合3D方法相比,至少实现了3倍的速度提升,同时在组合3D重建性能上达到了新的基准。相关代码已发布至:https://github.com/YujiaHu1109/Flash-Sculptor。
论文及项目相关链接
Summary
本文提出了一种名为Flash Sculptor的框架,用于从单张图像进行场景/对象的3D重建。它采用分而治之的策略,将复杂的场景重建分解为多个子任务,包括处理每个实例的外观、旋转、尺度和平移。Flash Sculptor实现了高效的旋转粗到细方案,并开发了一种基于异常值去除的算法,确保在单步中精确稳健地计算平移参数,无需任何迭代优化。实验表明,Flash Sculptor在合成3D方法上的速度至少提升了三倍,同时设定了新的合成3D重建性能基准。
Key Takeaways
- Flash Sculptor是一个用于从单张图像进行场景/对象3D重建的框架。
- 它采用分而治之的策略处理复杂的场景重建。
- Flash Sculptor将场景重建分解为多个子任务,包括处理每个实例的外观、旋转、尺度和平移。
- 提出了高效的旋转粗到细方案。
- 开发了一种基于异常值去除的算法,确保精确稳健地计算平移参数。
- 实验表明,Flash Sculptor在合成3D方法上的速度有显著提升。
点此查看论文截图
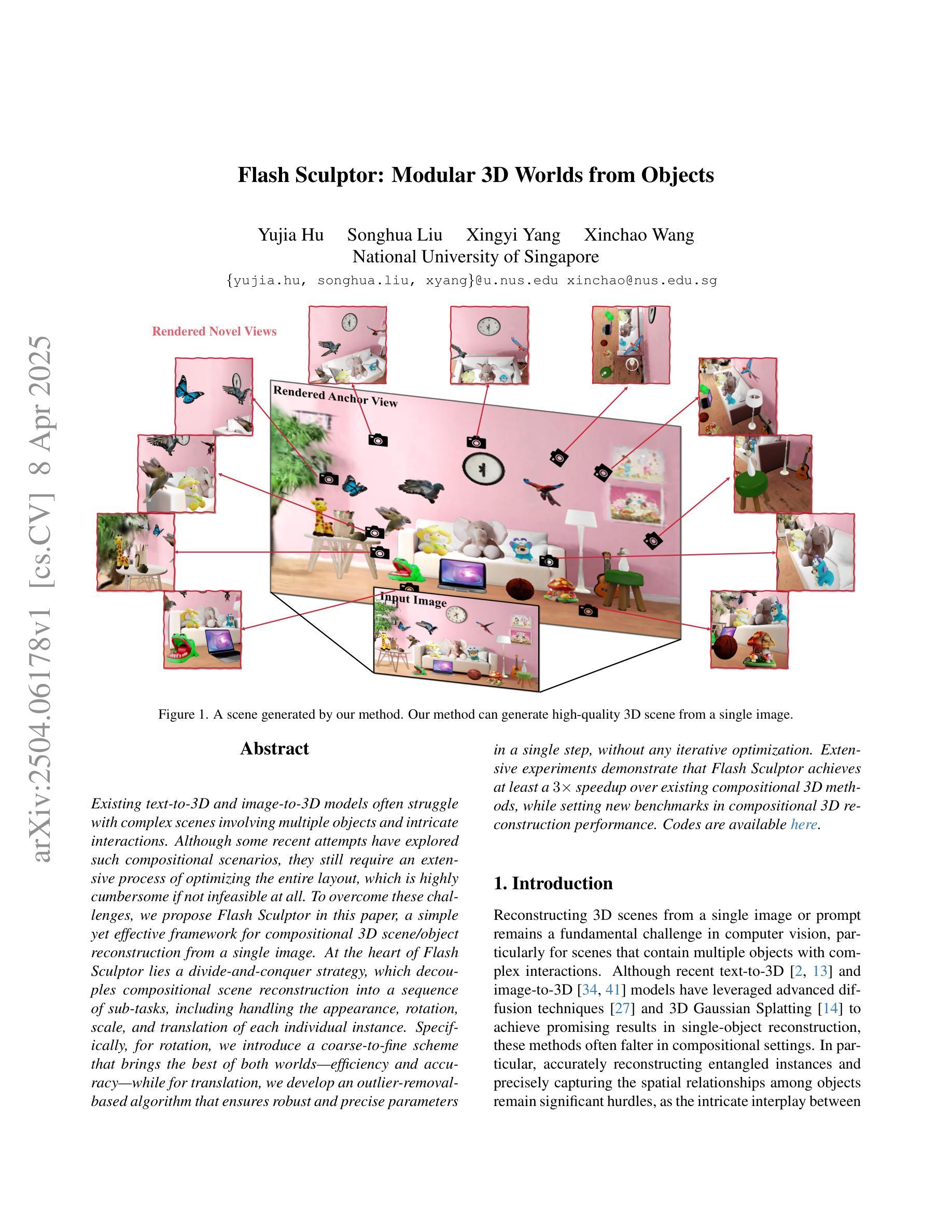
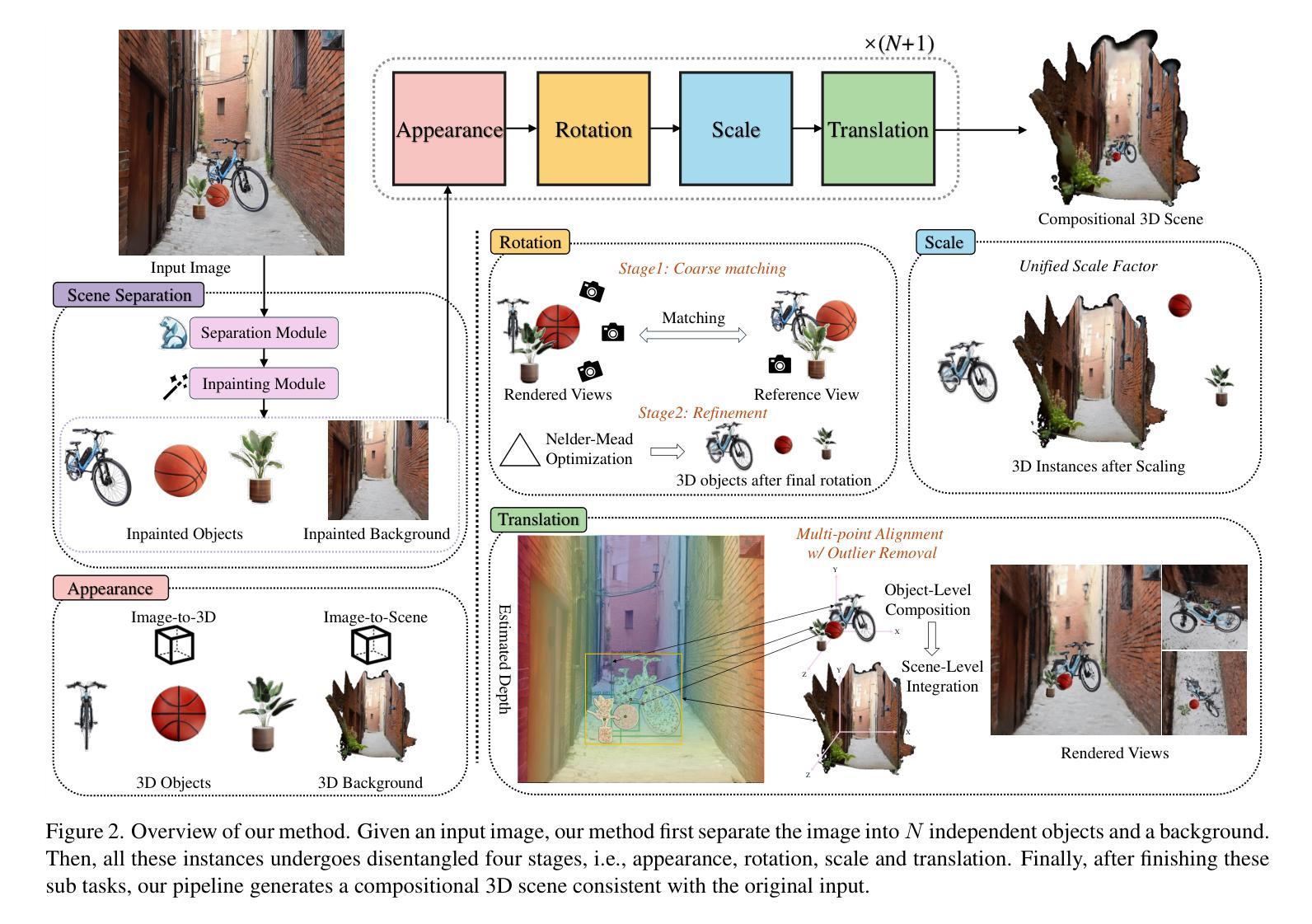
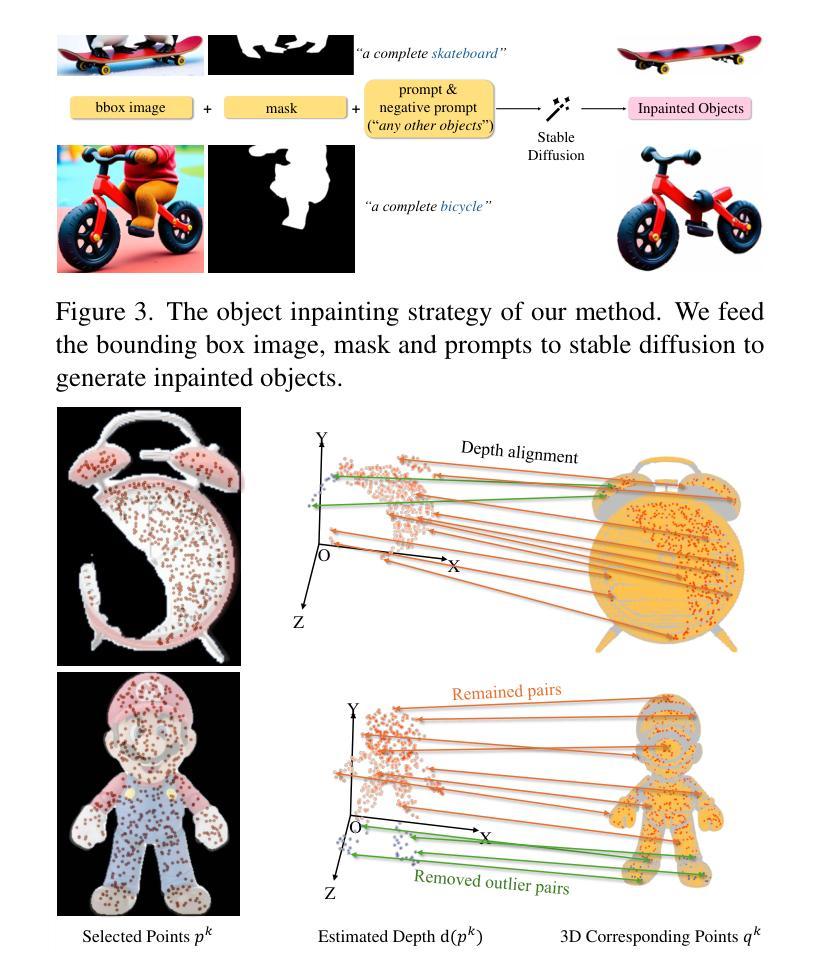
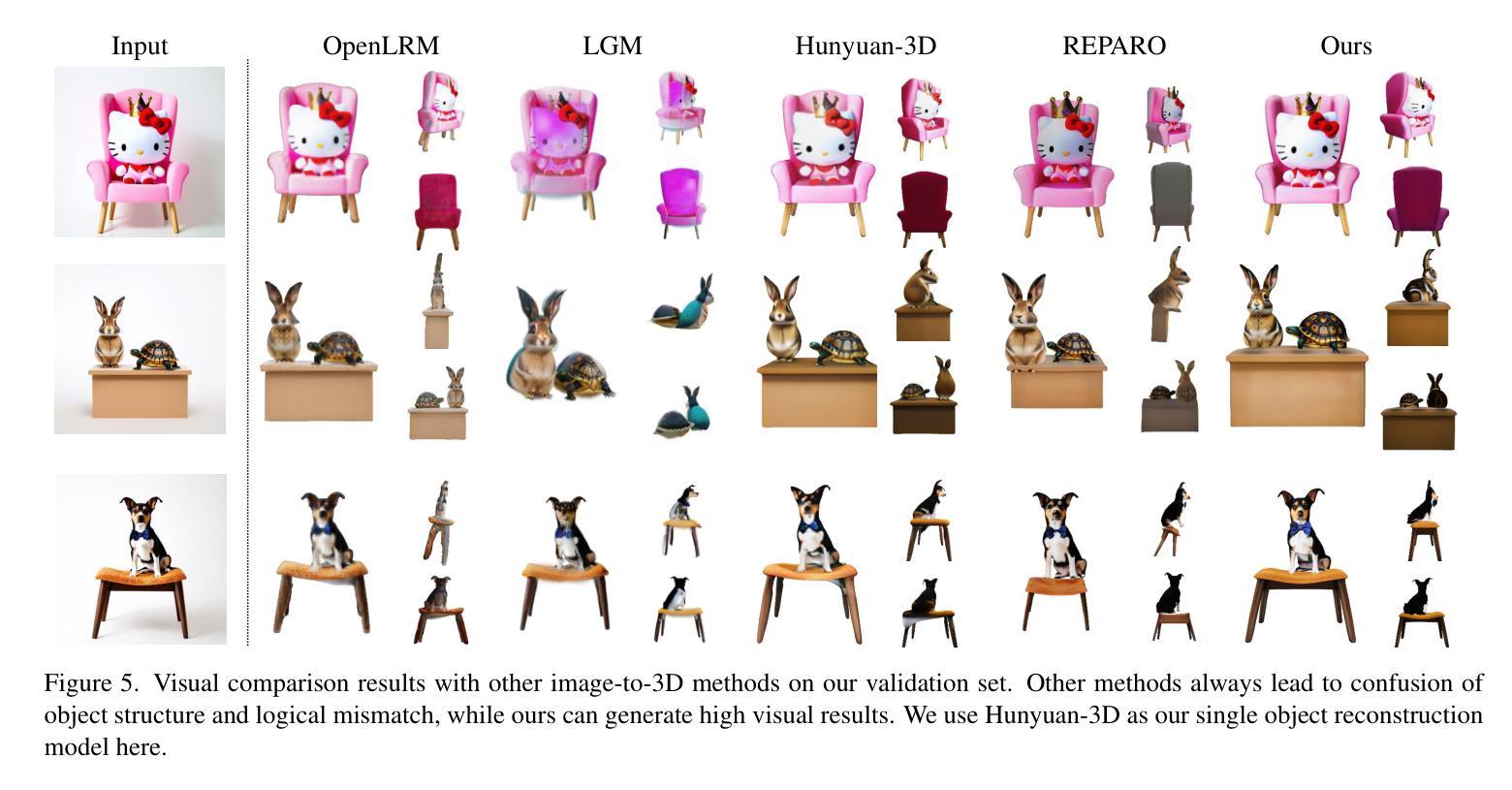
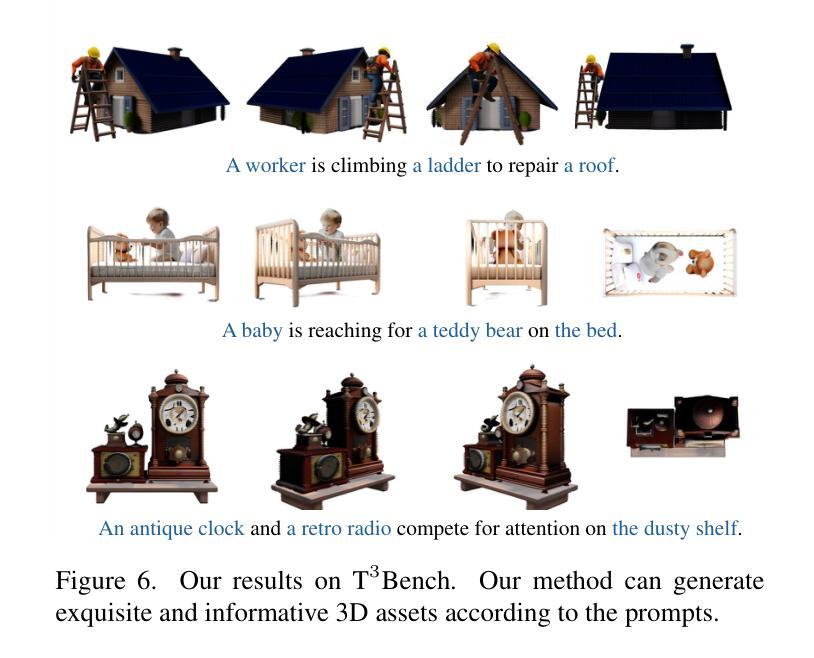
Highest Probability Density Conformal Regions
Authors:Max Sampson, Kung-Sik Chan
This paper proposes a new method for finding the highest predictive density set or region, within the heteroscedastic regression framework. This framework enjoys the property that any highest predictive density set is a translation of some scalar multiple of a highest density set for the standardized regression error, with the same prediction accuracy. The proposed method leverages this property to efficiently compute conformal prediction regions, using signed conformal inference, kernel density estimation, in conjunction with any conditional mean, and scale estimators. While most conformal prediction methods output prediction intervals, this method adapts to the target. When the target is multi-modal, the proposed method outputs an approximation of the smallest multi-modal set. When the target is uni-modal, the proposed method outputs an approximation of the smallest interval. Under mild regularity conditions, we show that these conformal prediction sets are asymptotically close to the true smallest prediction sets. Because of the conformal guarantee, even in finite sample sizes the method has guaranteed coverage. With simulations and a real data analysis we demonstrate that the proposed method is better than existing methods when the target is multi-modal, and gives similar results when the target is uni-modal. Supplementary materials, including proofs and additional images, are available online.
本文提出了一种新的方法,用于在异方差回归框架内找到最高预测密度集或区域。该框架具有这样的属性:任何最高预测密度集都是标准化回归误差最高密度集的某种标量倍的平移,并且具有相同的预测精度。所提出的方法利用这一属性,结合符号化顺应推理、核密度估计以及任何条件均值和尺度估计,有效地计算顺应预测区域。虽然大多数顺应预测方法输出预测区间,但该方法适应于目标。当目标是多模态时,所提出的方法输出最小多模态集的近似值。当目标是单模态时,该方法输出最小区间的近似值。在温和的正则条件下,我们证明这些顺应预测集最终接近真正的最小预测集。由于顺应性保证,即使在有限样本量下,该方法也具有保证的覆盖范围。通过模拟和真实数据分析,我们证明当目标为多模态时,所提出的方法优于现有方法,而当目标为单模态时,所提出的方法给出了类似的结果。补充材料,包括证明和额外图像,均可在网上找到。
论文及项目相关链接
Summary
新一代异方差回归框架下的预测密度集寻找方法。该方法利用最高预测密度集的性质,通过符号化保形推断、核密度估计以及任何条件均值和尺度估计,有效地计算保形预测区域。该方法可适应目标模式,无论是多模态还是单模态,并输出相应的最小预测集。在温和的正则条件下,该方法的预测集渐近接近真实最小预测集。即使样本量有限,该方法也具有保形覆盖的保证。模拟和真实数据分析表明,当目标为多模态时,该方法优于现有方法;当目标为单模态时,该方法的结果相似。
Key Takeaways
- 新方法在异方差回归框架下寻找最高预测密度集。
- 方法利用最高预测密度集的性质进行计算。
- 通过符号化保形推断等技术实现有效计算。
- 方法可适应目标的多模态和单模态特性。
- 输出相应的最小预测集,无论是多模态还是单模态目标。
- 在温和条件下,预测集渐近接近真实最小预测集。
- 方法具有保形覆盖的保证,并通过模拟和真实数据分析验证其有效性。
点此查看论文截图
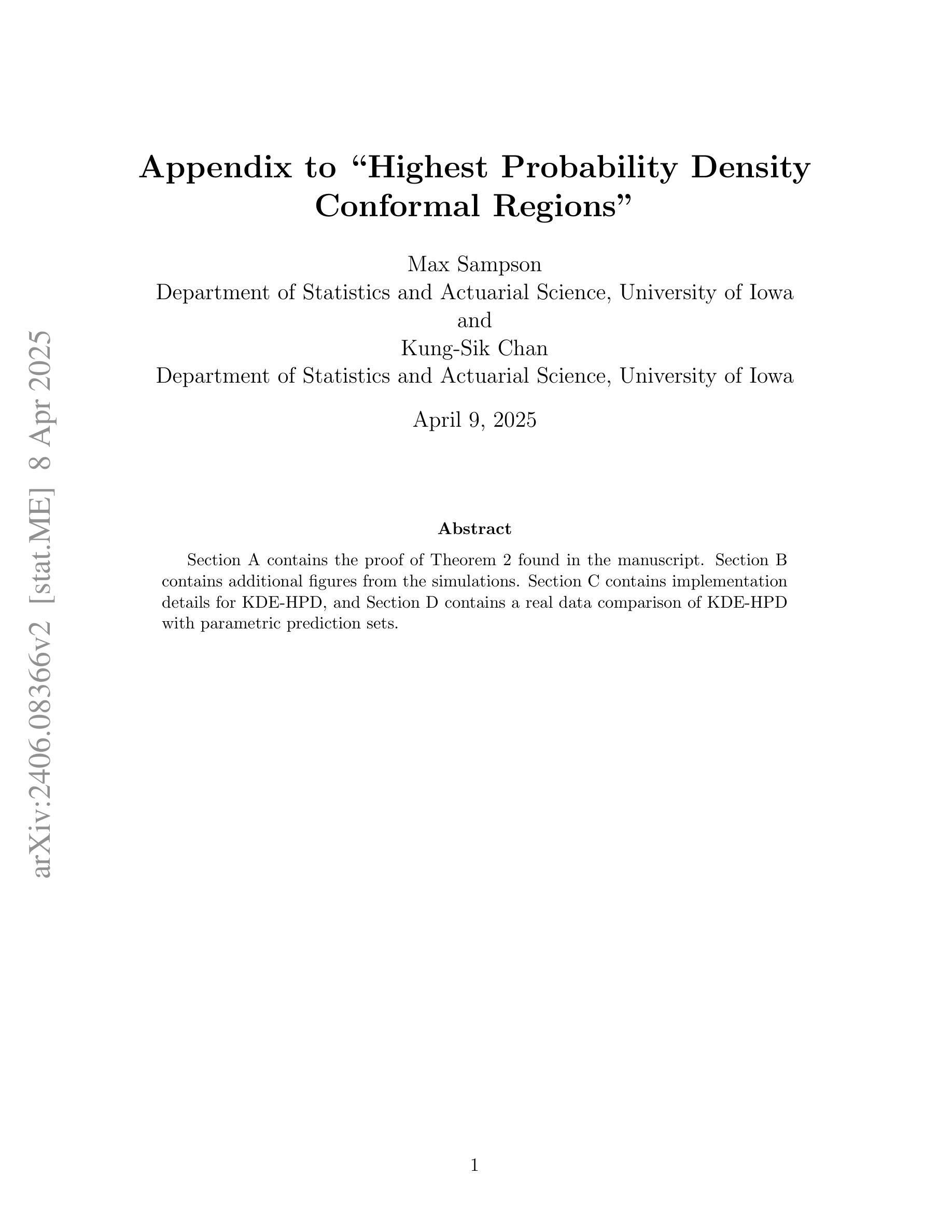