⚠️ 以下所有内容总结都来自于 大语言模型的能力,如有错误,仅供参考,谨慎使用
🔴 请注意:千万不要用于严肃的学术场景,只能用于论文阅读前的初筛!
💗 如果您觉得我们的项目对您有帮助 ChatPaperFree ,还请您给我们一些鼓励!⭐️ HuggingFace免费体验
2025-04-10 更新
When Less Is More: A Sparse Facial Motion Structure For Listening Motion Learning
Authors:Tri Tung Nguyen Nguyen, Quang Tien Dam, Dinh Tuan Tran, Joo-Ho Lee
Effective human behavior modeling is critical for successful human-robot interaction. Current state-of-the-art approaches for predicting listening head behavior during dyadic conversations employ continuous-to-discrete representations, where continuous facial motion sequence is converted into discrete latent tokens. However, non-verbal facial motion presents unique challenges owing to its temporal variance and multi-modal nature. State-of-the-art discrete motion token representation struggles to capture underlying non-verbal facial patterns making training the listening head inefficient with low-fidelity generated motion. This study proposes a novel method for representing and predicting non-verbal facial motion by encoding long sequences into a sparse sequence of keyframes and transition frames. By identifying crucial motion steps and interpolating intermediate frames, our method preserves the temporal structure of motion while enhancing instance-wise diversity during the learning process. Additionally, we apply this novel sparse representation to the task of listening head prediction, demonstrating its contribution to improving the explanation of facial motion patterns.
有效的人类行为建模对于成功的人机交互至关重要。当前最先进的预测二元对话中倾听头部行为的方法采用连续到离散表示,将连续的面部运动序列转换为离散潜在符号。然而,非言语面部运动由于其时间上的变化和多种模态的特性而呈现出独特的挑战。最先进的离散运动符号表示法难以捕捉潜在的非言语面部模式,使得训练倾听头部低效且生成的运动保真度低。本研究提出了一种新的表示和预测非言语面部运动的方法,通过将长序列编码为关键帧和过渡帧的稀疏序列。通过识别关键的运动步骤并插入中间帧,我们的方法可以在学习过程中保持运动的时序结构,同时提高实例级的多样性。此外,我们将这种新型稀疏表示应用于预测头部运动的任务中,证明了它对解释面部运动模式的贡献。
论文及项目相关链接
Summary
本文强调有效的人类行为建模对于成功的人机交互至关重要。当前预测对话中倾听头部行为的最先进方法采用连续到离散表示,将连续面部运动序列转换为离散潜在符号。然而,非言语面部运动因其时间上的变化和多种模式而具有独特挑战。当前离散运动符号表示法难以捕捉潜在的非言语面部模式,使得训练倾听头部时效率较低,产生的运动逼真度较低。本研究提出了一种新的非言语面部运动表示和预测方法,通过将长序列编码为关键帧和过渡帧的稀疏序列来识别关键运动步骤并推断中间帧,该方法在保留运动的时序结构的同时,增强了学习过程中的个体差异性。此外,我们将这种新型稀疏表示应用于预测头部运动的任务中,证明了它对解释面部运动模式的贡献。
Key Takeaways
- 有效的人类行为建模对于实现成功的人机交互至关重要。
- 当前预测倾听头部行为的方法主要使用连续到离散的表示方式。
- 非言语面部运动具有独特挑战,表现在其时间上的变化和多种模式上。
- 当前离散运动符号表示法难以捕捉潜在的非言语面部模式。
- 本研究提出了一种新的非言语面部运动表示方法,通过编码长序列为稀疏序列来识别关键运动步骤并推断中间帧。
- 该方法在保留运动的时序结构的同时,增强了学习过程中的个体差异性。
点此查看论文截图
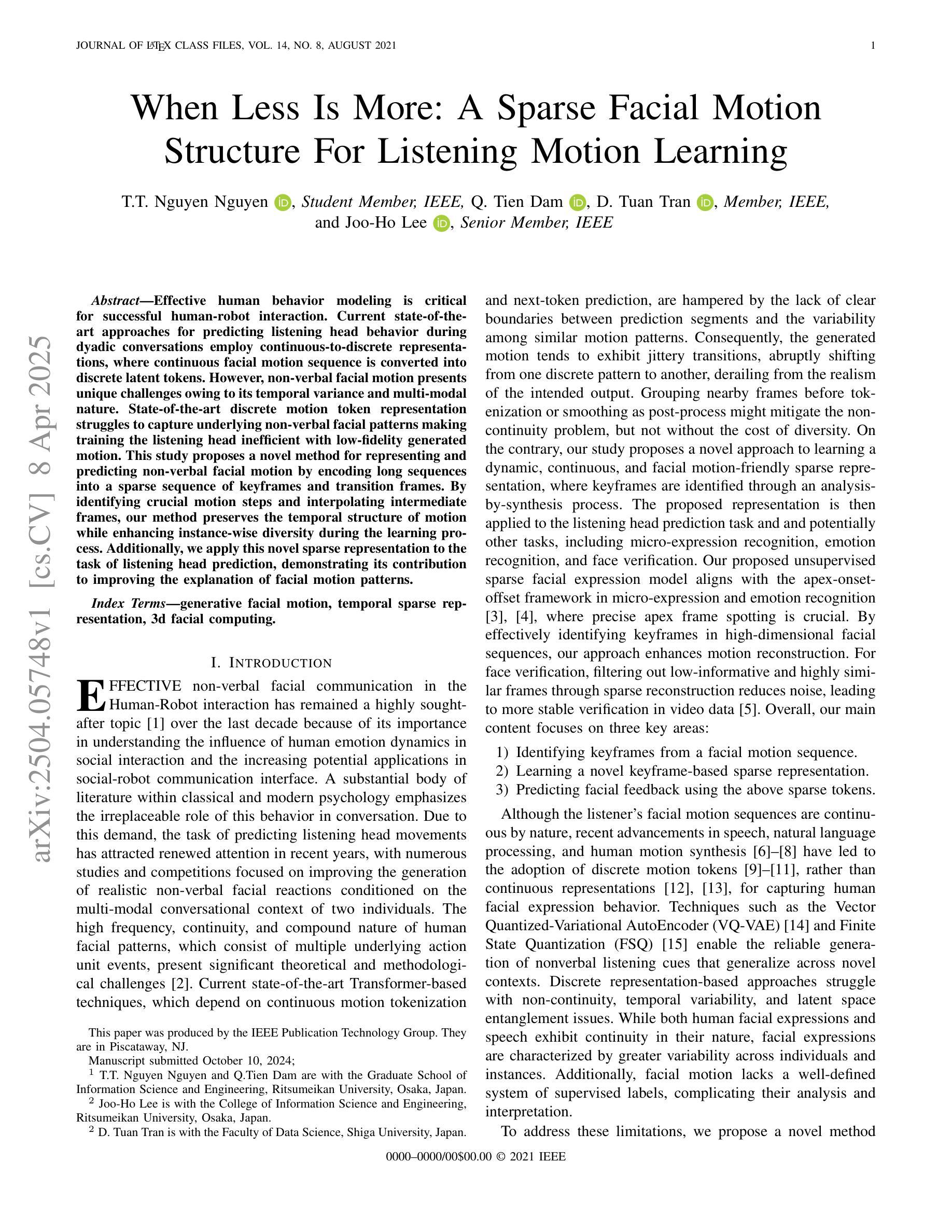
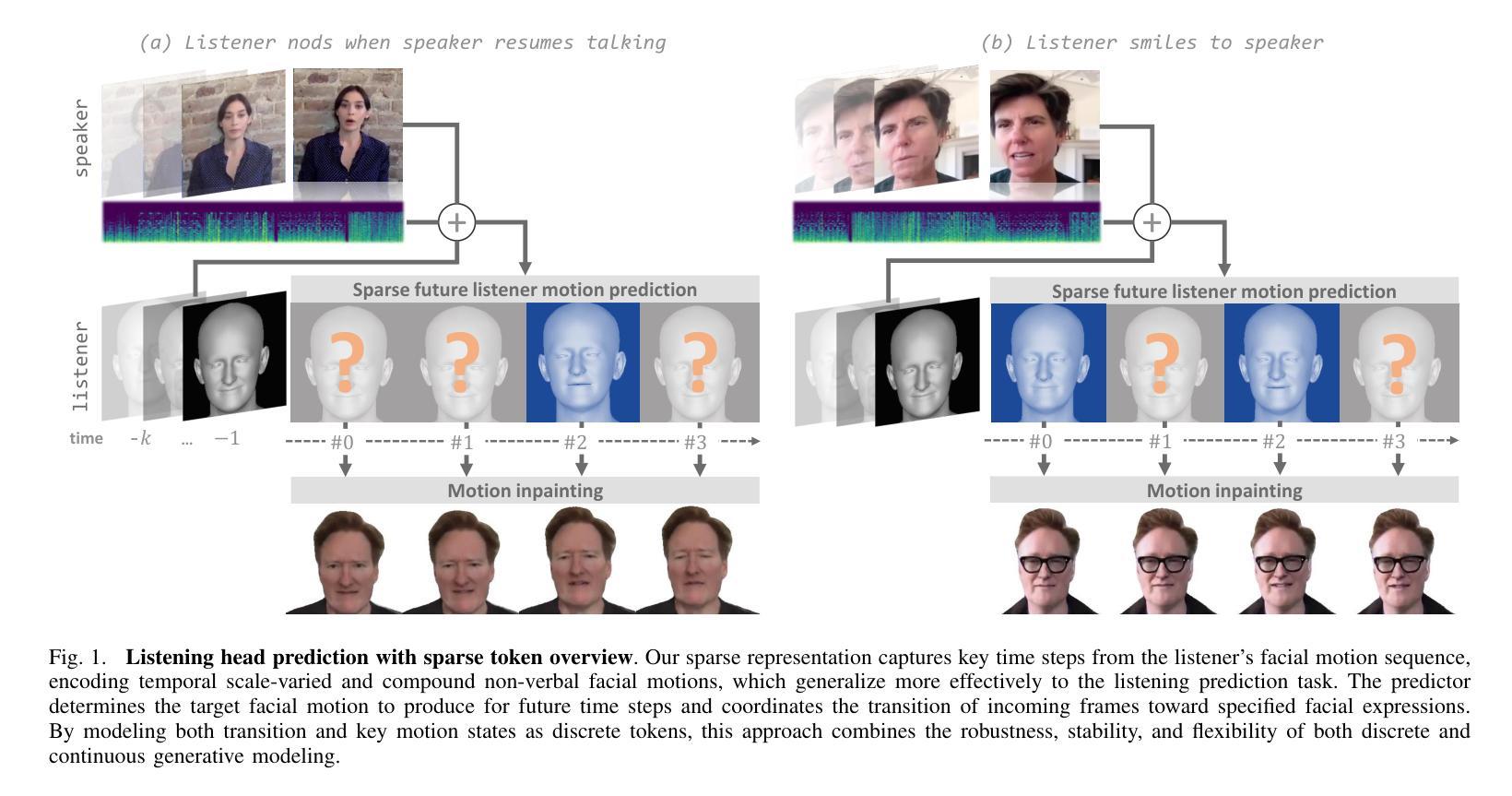
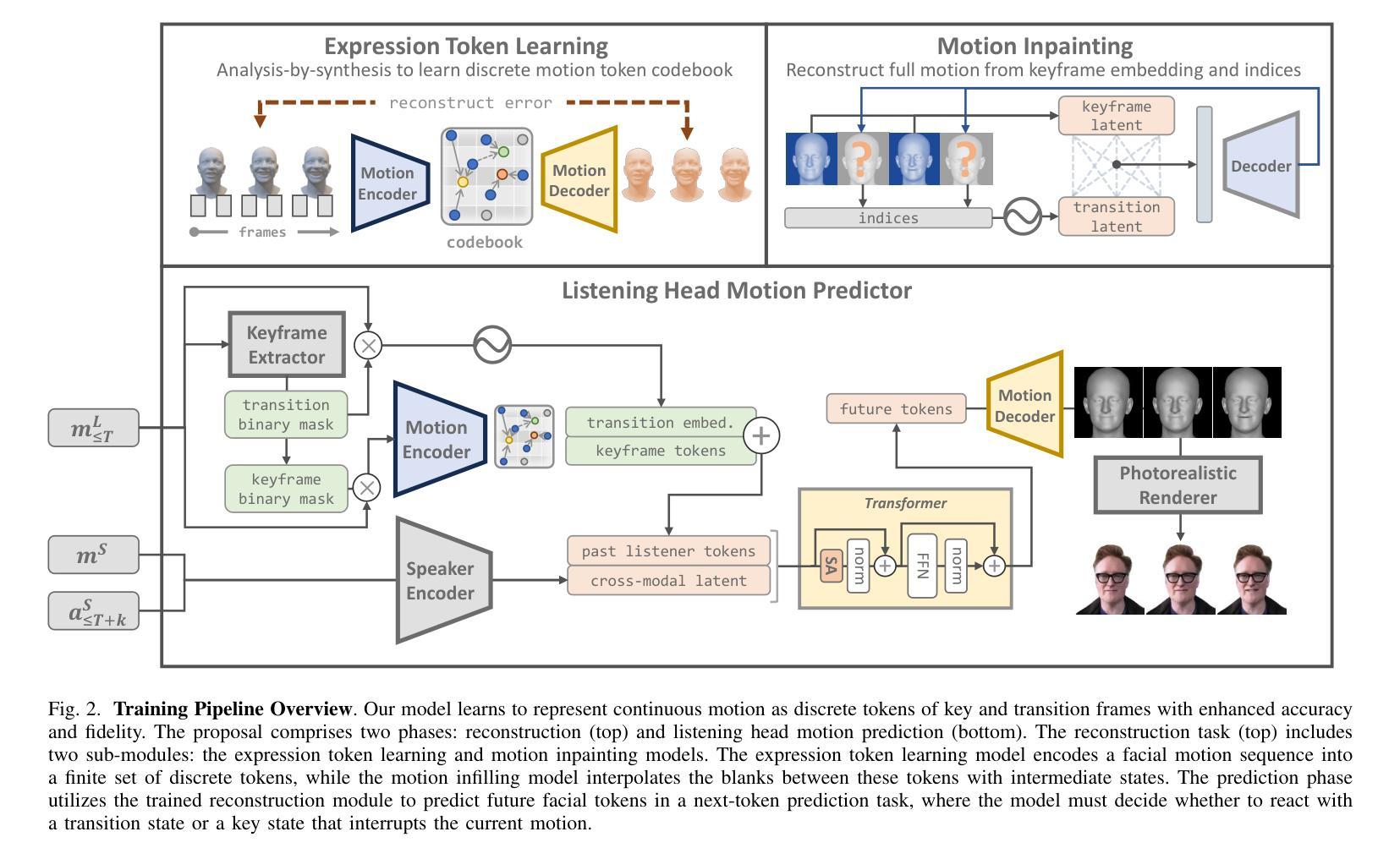
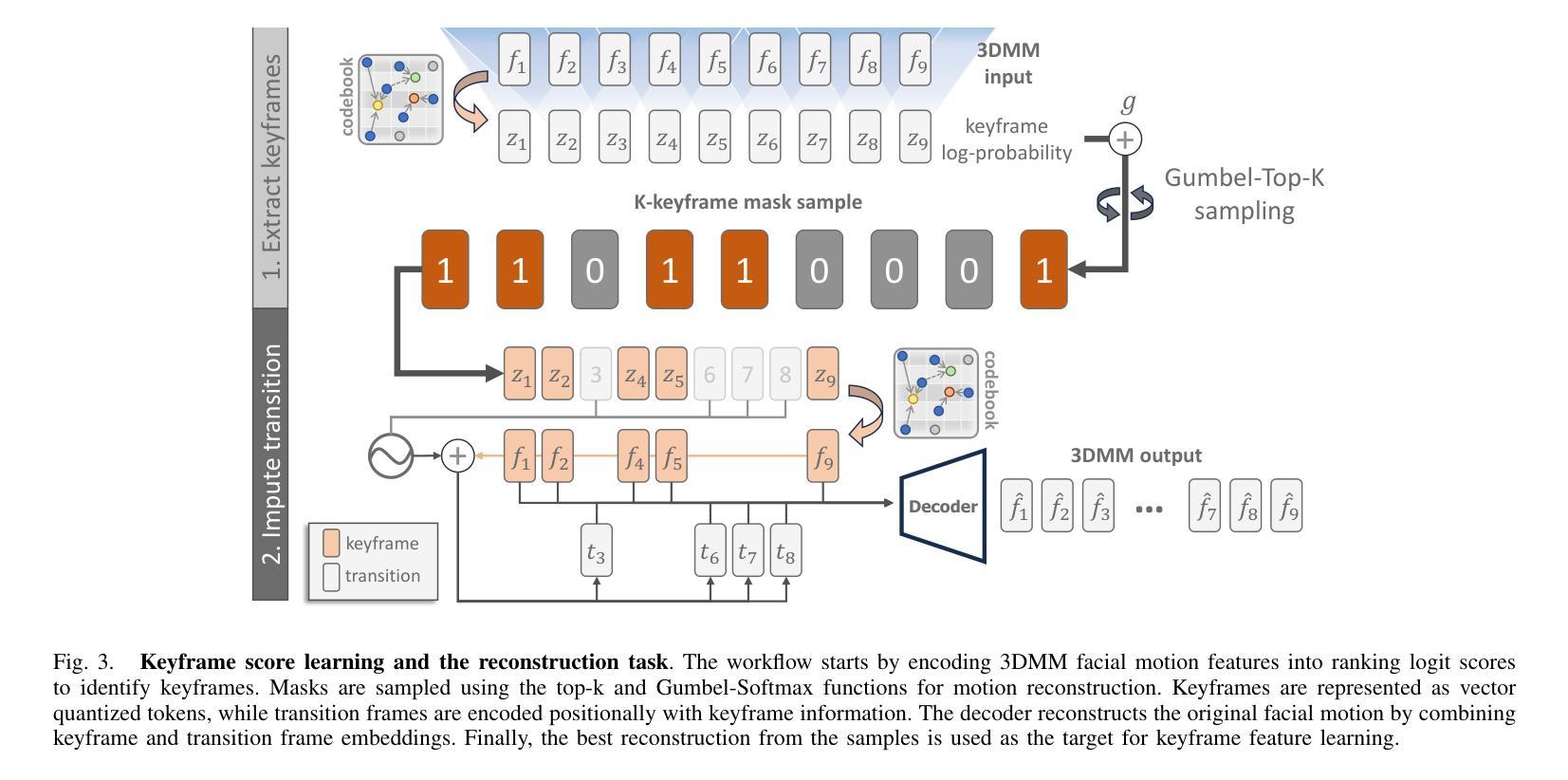
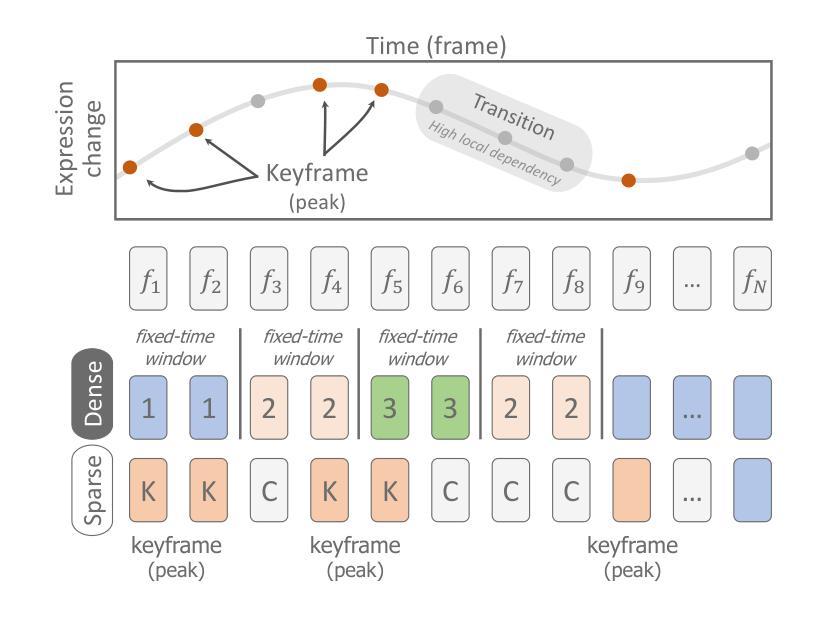
Local-Cloud Inference Offloading for LLMs in Multi-Modal, Multi-Task, Multi-Dialogue Settings
Authors:Liangqi Yuan, Dong-Jun Han, Shiqiang Wang, Christopher G. Brinton
Compared to traditional machine learning models, recent large language models (LLMs) can exhibit multi-task-solving capabilities through multiple dialogues and multi-modal data sources. These unique characteristics of LLMs, together with their large model size, make their deployment more challenging. Specifically, (i) deploying LLMs on local devices faces computational, memory, and energy resource issues, while (ii) deploying them in the cloud cannot guarantee real-time service and incurs communication/usage costs. In this paper, we design TMO, a local-cloud LLM inference system with Three-M Offloading: Multi-modal, Multi-task, and Multi-dialogue. TMO incorporates (i) a lightweight local LLM that can process simple tasks at high speed and (ii) a large-scale cloud LLM that can handle multi-modal data sources. We develop a resource-constrained reinforcement learning (RCRL) strategy for TMO that optimizes the inference location (i.e., local vs. cloud) and multi-modal data sources to use for each task/dialogue, aiming to maximize the long-term reward (response quality, latency, and usage cost) while adhering to resource constraints. We also contribute M4A1, a new dataset we curated that contains reward and cost metrics across multiple modality, task, dialogue, and LLM configurations, enabling evaluation of offloading decisions. We demonstrate the effectiveness of TMO compared to several exploration-decision and LLM-as-Agent baselines, showing significant improvements in latency, cost, and response quality.
与传统机器学习模型相比,最近的大型语言模型(LLM)能够通过多种对话和多模态数据源展现出多任务解决能力。LLM的这些独特特点以及它们的大型模型规模,使得其部署更具挑战性。具体而言,(i)在本地设备上部署LLM面临计算、内存和能源资源问题,而(ii)在云端部署它们则无法保证实时服务并会产生通信/使用成本。在本文中,我们设计了TMO,一个具有三种卸载功能的本地云LLM推理系统:多模态、多任务和多对话。TMO结合了(i)一个轻量级的本地LLM,可以高速处理简单任务,以及(ii)一个可以处理多模态数据源的大规模云LLM。我们为TMO开发了一种资源受限强化学习(RCRL)策略,该策略可以优化推理位置(即本地与云之间)以及针对每个任务/对话要使用的多模态数据源,旨在最大化长期奖励(响应质量、延迟和使用成本),同时遵守资源限制。我们还贡献了M4A1数据集,这是我们整理的一个新数据集,其中包含跨多个模态、任务、对话和LLM配置下的奖励和成本指标,能够对卸载决策进行评估。我们证明了与多种探索决策和LLM代理基线相比,TMO的有效性,在延迟、成本和质量方面显示出显着改进。
论文及项目相关链接
Summary
近期的大型语言模型(LLM)具备多任务解决能力和多模态数据源处理能力。然而,部署这些模型面临计算、内存和能源资源挑战。为此,本文设计了TMO系统,结合本地和云端推理,实现多模态、多任务和多对话的负载转移。TMO采用轻量化本地LLM处理简单任务,而大规模云端LLM处理多模态数据源。通过资源约束强化学习策略优化推理位置和数据源使用,旨在实现长期奖励最大化。此外,本文还贡献了新的数据集M4A1,用于评估负载转移决策的效果。实验表明,TMO相较于其他探索决策和LLM-as-Agent基线方案,在延迟、成本和响应质量方面有明显改进。
Key Takeaways
- 大型语言模型(LLM)具备多任务解决能力和多模态数据源处理能力。
- LLMs的部署面临计算、内存和能源资源挑战,本地和云端部署各有优缺点。
- TMO系统结合本地和云端推理,实现多模态、多任务和多对话的负载转移。
- TMO采用轻量化本地LLM处理简单任务,大规模云端LLM处理复杂任务。
- 通过资源约束强化学习策略优化推理位置和数据源使用。
- 贡献了新的数据集M4A1,用于评估负载转移决策的效果。
点此查看论文截图
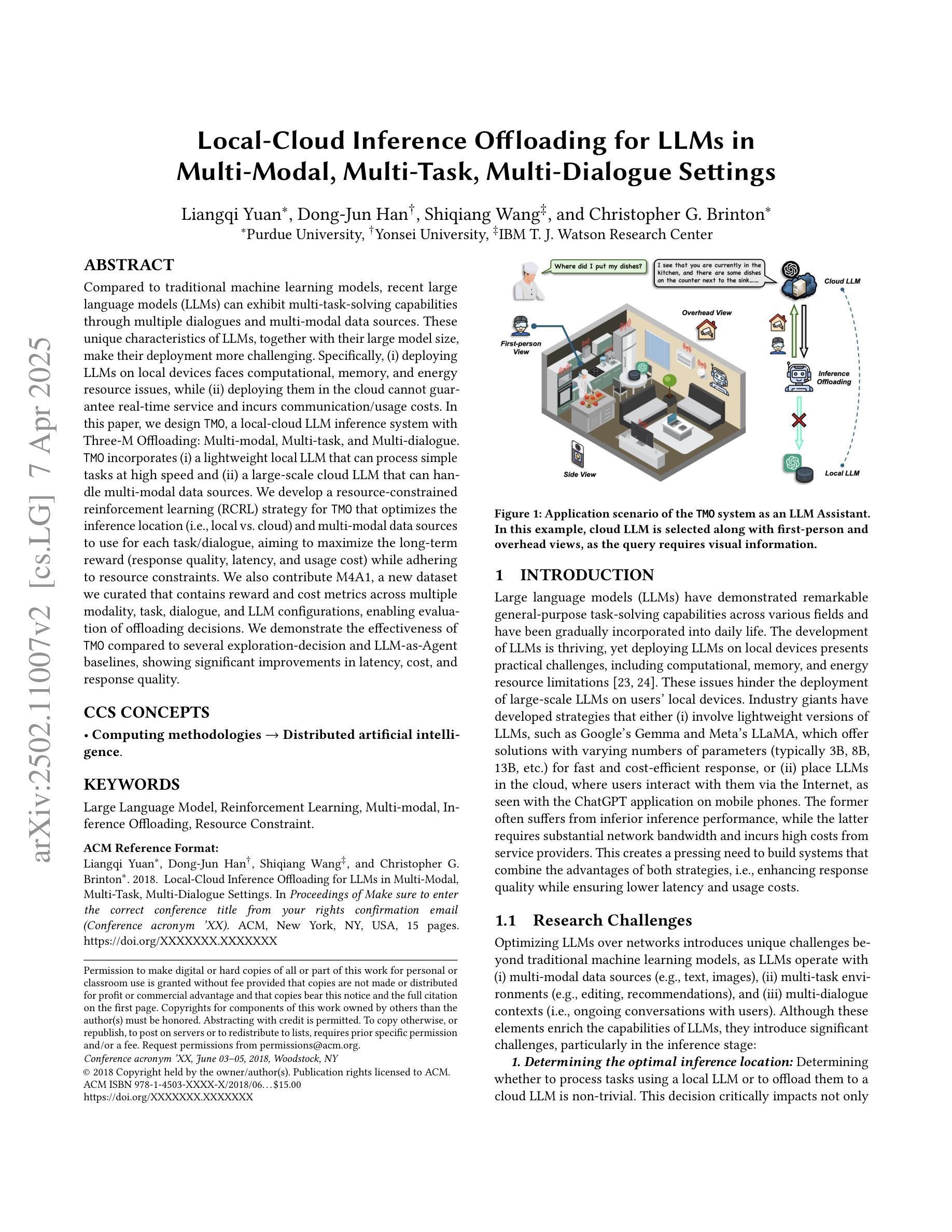
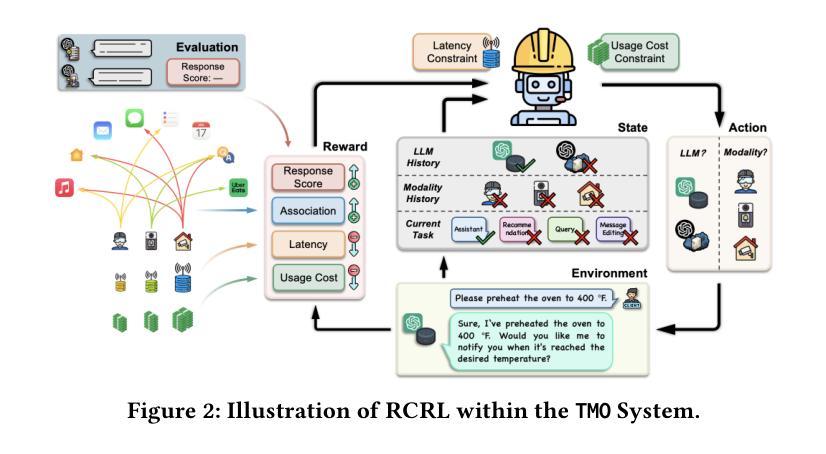