⚠️ 以下所有内容总结都来自于 大语言模型的能力,如有错误,仅供参考,谨慎使用
🔴 请注意:千万不要用于严肃的学术场景,只能用于论文阅读前的初筛!
💗 如果您觉得我们的项目对您有帮助 ChatPaperFree ,还请您给我们一些鼓励!⭐️ HuggingFace免费体验
2025-04-10 更新
AVENet: Disentangling Features by Approximating Average Features for Voice Conversion
Authors:Wenyu Wang, Yiquan Zhou, Jihua Zhu, Hongwu Ding, Jiacheng Xu, Shihao Li
Voice conversion (VC) has made progress in feature disentanglement, but it is still difficult to balance timbre and content information. This paper evaluates the pre-trained model features commonly used in voice conversion, and proposes an innovative method for disentangling speech feature representations. Specifically, we first propose an ideal content feature, referred to as the average feature, which is calculated by averaging the features within frame-level aligned parallel speech (FAPS) data. For generating FAPS data, we utilize a technique that involves freezing the duration predictor in a Text-to-Speech system and manipulating speaker embedding. To fit the average feature on traditional VC datasets, we then design the AVENet to take features as input and generate closely matching average features. Experiments are conducted on the performance of AVENet-extracted features within a VC system. The experimental results demonstrate its superiority over multiple current speech feature disentangling methods. These findings affirm the effectiveness of our disentanglement approach.
语音转换(VC)在特征分离方面已经取得了一定的进展,但仍难以平衡音色和内容信息的平衡。本文对语音转换中常用的预训练模型特征进行了评估,提出了一种创新的语音特征表示分离方法。具体来说,我们首先提出了理想的内容特征,称为平均特征,它是通过计算帧级对齐并行语音(FAPS)数据内的特征平均值来得到的。为了生成FAPS数据,我们采用了一种技术,即在文本到语音系统中冻结持续时间预测器并操作说话者嵌入。为了在传统的VC数据集上适应平均特征,然后我们设计了AVENet,以特征作为输入并生成紧密匹配的平均特征。在VC系统中对AVENet提取的特征的性能进行了实验。实验结果表明,其在多个当前的语音特征分离方法中具有优越性。这些发现证实了我们分离方法的有效性。
论文及项目相关链接
PDF Accepted by ICME 2025
Summary
本文探讨了语音转换中的特征解耦问题,评估了常用于语音转换的预训练模型特征,并提出了一种新的语音特征表示解耦方法。通过计算帧级对齐并行语音数据的平均特征,实现了理想的内容特征提取。同时,设计了一个名为AVENet的模型,能够在传统语音转换数据集上拟合这些平均特征,并在实验上验证了其性能优于多种现有的语音特征解耦方法。
Key Takeaways
- 语音转换中仍存在平衡音色和内容信息的难题。
- 评估了常用的预训练模型特征在语音转换中的应用。
- 提出了一种新的理想内容特征提取方法,即计算帧级对齐并行语音数据的平均特征。
- 通过冻结文本到语音系统的时长预测器并操作说话者嵌入来生成帧级对齐并行语音数据。
- 设计了AVENet模型,能够在传统语音转换数据集上拟合平均特征。
- 实验证明了AVENet提取的特征在语音转换系统中的性能优于多种现有方法。
点此查看论文截图
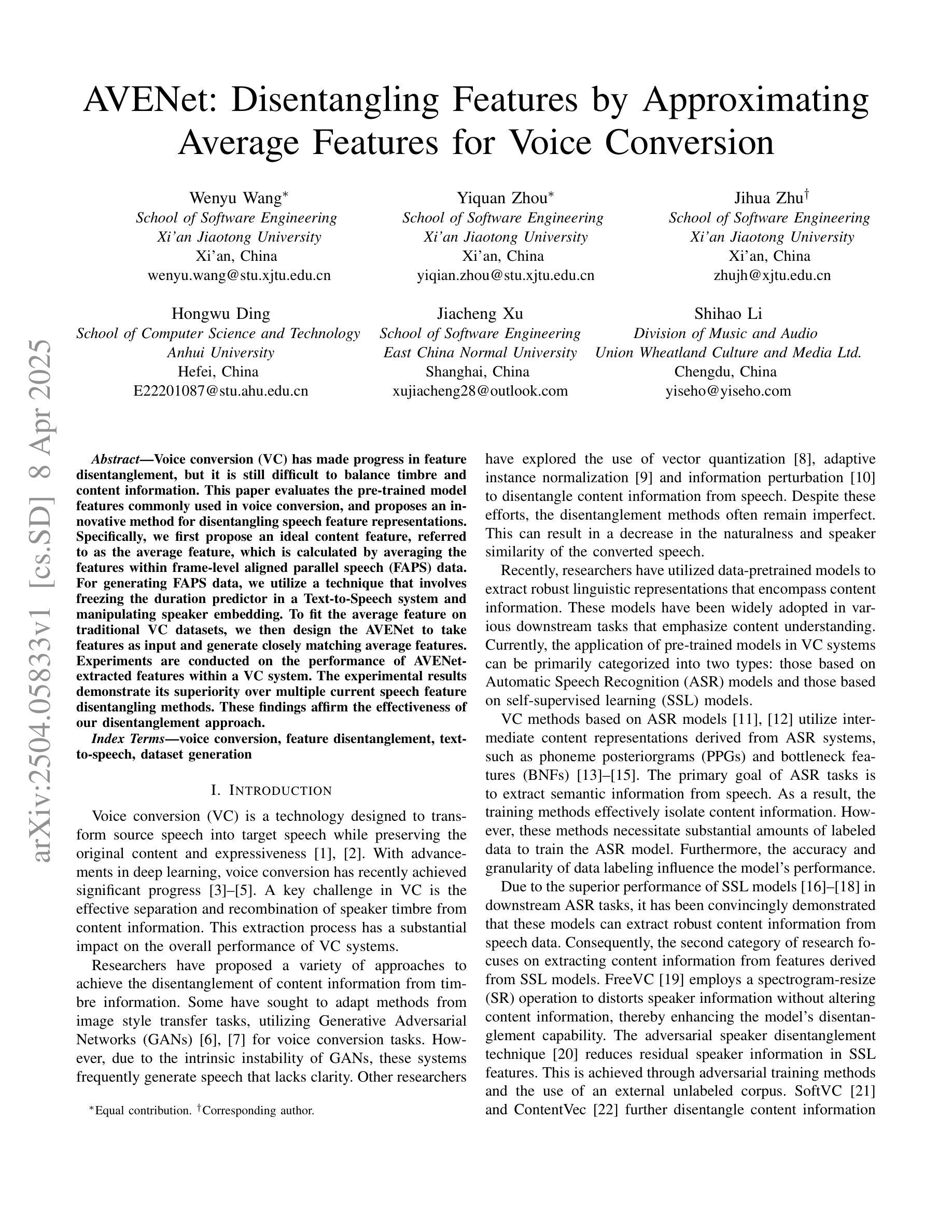
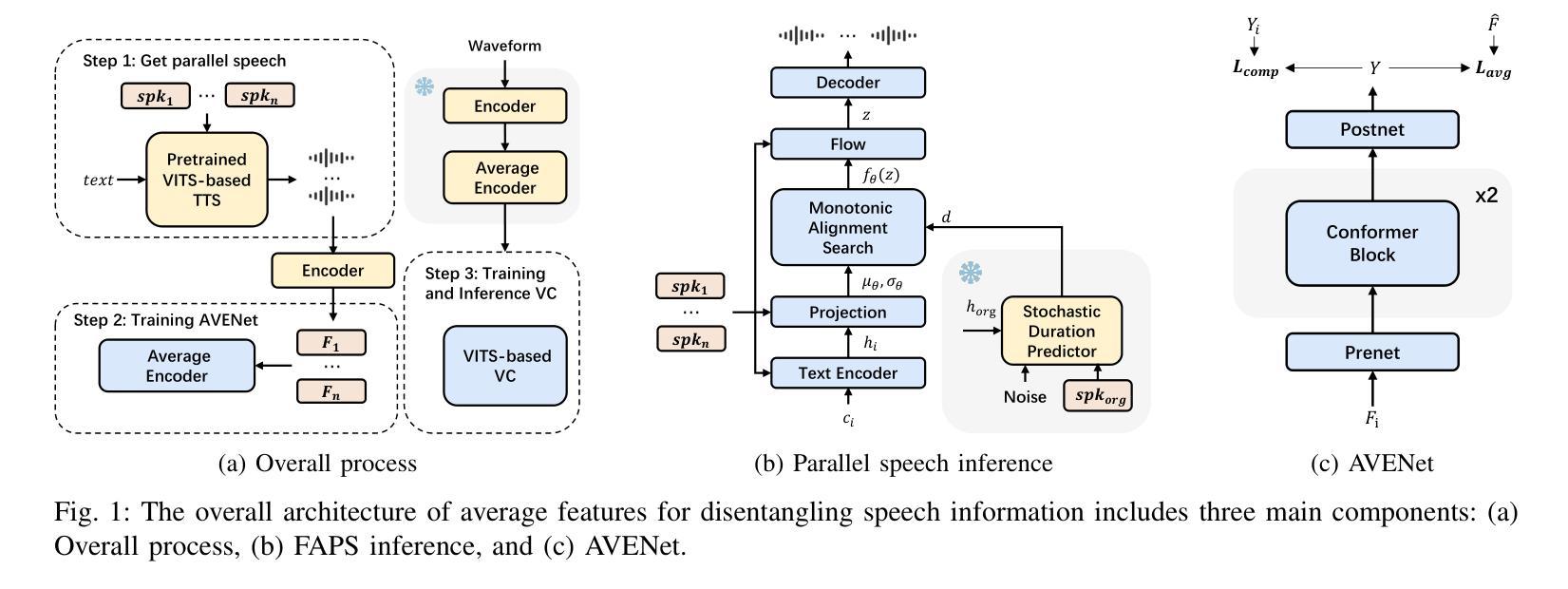
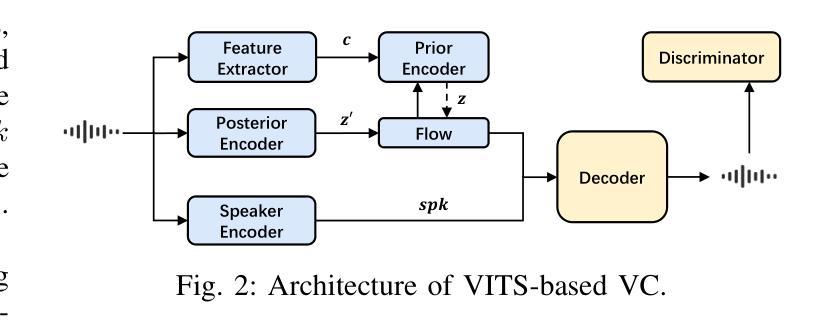
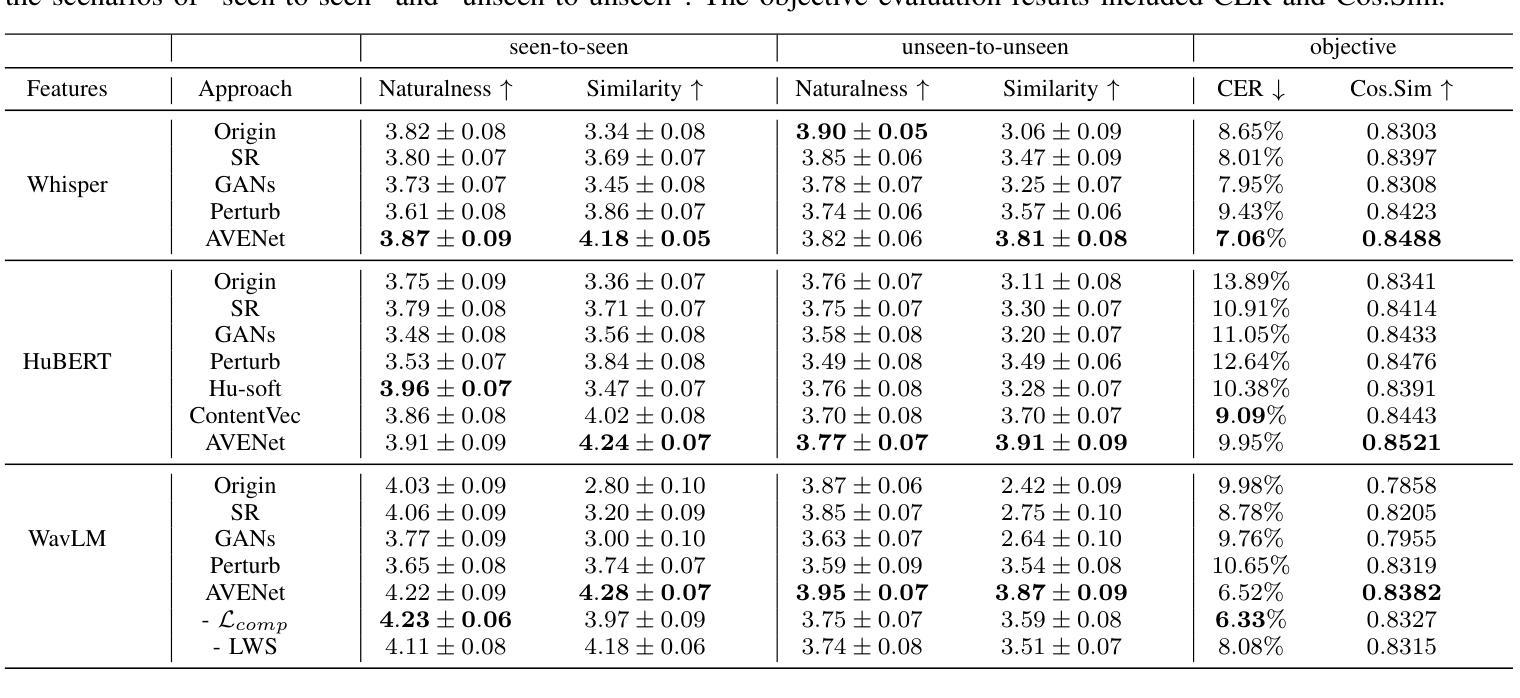
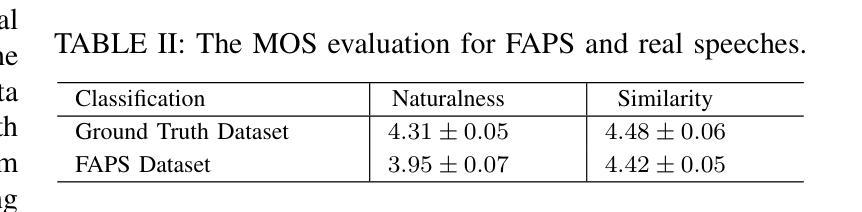
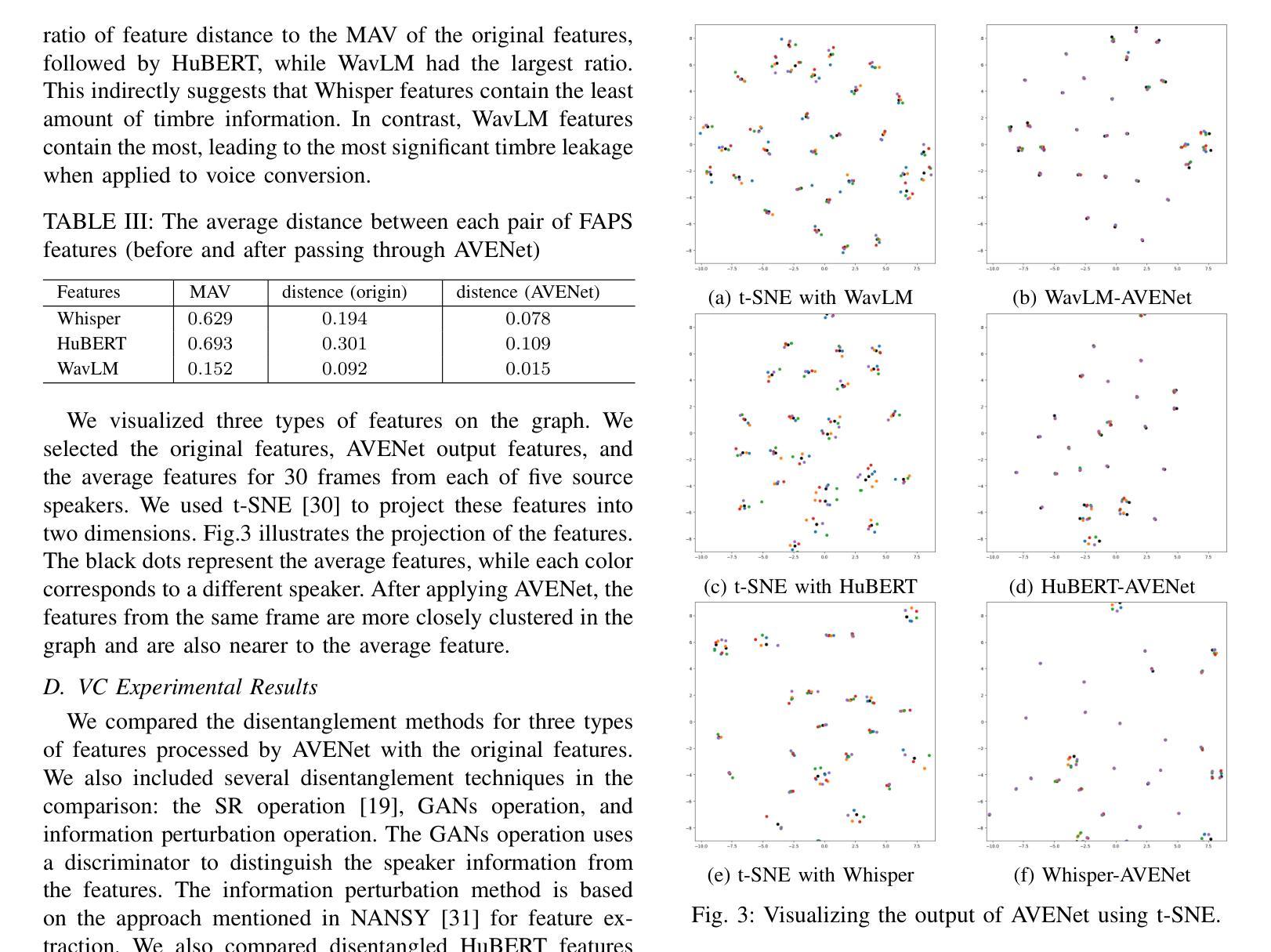