⚠️ 以下所有内容总结都来自于 大语言模型的能力,如有错误,仅供参考,谨慎使用
🔴 请注意:千万不要用于严肃的学术场景,只能用于论文阅读前的初筛!
💗 如果您觉得我们的项目对您有帮助 ChatPaperFree ,还请您给我们一些鼓励!⭐️ HuggingFace免费体验
2025-04-11 更新
Distilling Textual Priors from LLM to Efficient Image Fusion
Authors:Ran Zhang, Xuanhua He, Ke Cao, Liu Liu, Li Zhang, Man Zhou, Jie Zhang
Multi-modality image fusion aims to synthesize a single, comprehensive image from multiple source inputs. Traditional approaches, such as CNNs and GANs, offer efficiency but struggle to handle low-quality or complex inputs. Recent advances in text-guided methods leverage large model priors to overcome these limitations, but at the cost of significant computational overhead, both in memory and inference time. To address this challenge, we propose a novel framework for distilling large model priors, eliminating the need for text guidance during inference while dramatically reducing model size. Our framework utilizes a teacher-student architecture, where the teacher network incorporates large model priors and transfers this knowledge to a smaller student network via a tailored distillation process. Additionally, we introduce spatial-channel cross-fusion module to enhance the model’s ability to leverage textual priors across both spatial and channel dimensions. Our method achieves a favorable trade-off between computational efficiency and fusion quality. The distilled network, requiring only 10% of the parameters and inference time of the teacher network, retains 90% of its performance and outperforms existing SOTA methods. Extensive experiments demonstrate the effectiveness of our approach. The implementation will be made publicly available as an open-source resource.
多模态图像融合旨在从多个源输入中合成单一、全面的图像。传统方法,如卷积神经网络和生成对抗网络,虽然效率较高,但在处理低质量或复杂输入时遇到困难。最近的文本引导方法利用大型模型先验来克服这些限制,但同时在内存和推理时间方面产生了巨大的计算开销。为了解决这一挑战,我们提出了一种提炼大型模型先验的新型框架,无需在推理过程中使用文本引导,同时显著减小了模型大小。我们的框架采用师徒架构,其中教师网络融入大型模型先验,并通过定制的提炼过程将这些知识转移到较小的学生网络。此外,我们引入了空间通道交叉融合模块,以提高模型在空间和通道维度上利用文本先验的能力。我们的方法在计算效率和融合质量之间实现了有利的权衡。经过提炼的网络仅需教师网络的10%参数和推理时间,即可保留90%的性能表现并超越现有的最先进方法。大量实验证明了我们方法的有效性。该实现将作为开源资源公开发布。
论文及项目相关链接
Summary
本文提出了一种新型的多模态图像融合框架,该框架通过蒸馏大型模型先验知识,解决了传统方法在处理低质量或复杂输入时的局限性,同时避免了文本引导带来的计算开销。通过教师-学生架构,教师网络融入大型模型先验知识,并通过定制的蒸馏过程将这些知识转移到较小的学生网络。此外,引入空间通道交叉融合模块,增强模型在空间和通道维度上利用文本先验的能力。该方法在计算效率和融合质量之间取得了良好的平衡。蒸馏网络仅需教师网络的10%参数和推理时间,就能保留90%的性能,并优于现有最先进的方法。
Key Takeaways
- 提出了一种新型多模态图像融合框架,解决了传统方法在处理低质量或复杂输入时的局限性。
- 通过教师-学生架构,实现了大型模型先验知识的蒸馏,避免了文本引导带来的计算开销。
- 引入了空间通道交叉融合模块,增强了模型利用文本先验的能力。
- 蒸馏网络性能优异,仅需教师网络的10%参数和推理时间,就能保留90%的性能。
- 该方法在计算效率和融合质量之间取得了良好的平衡。
- 进行了广泛的实验,证明了该方法的有效性。
点此查看论文截图
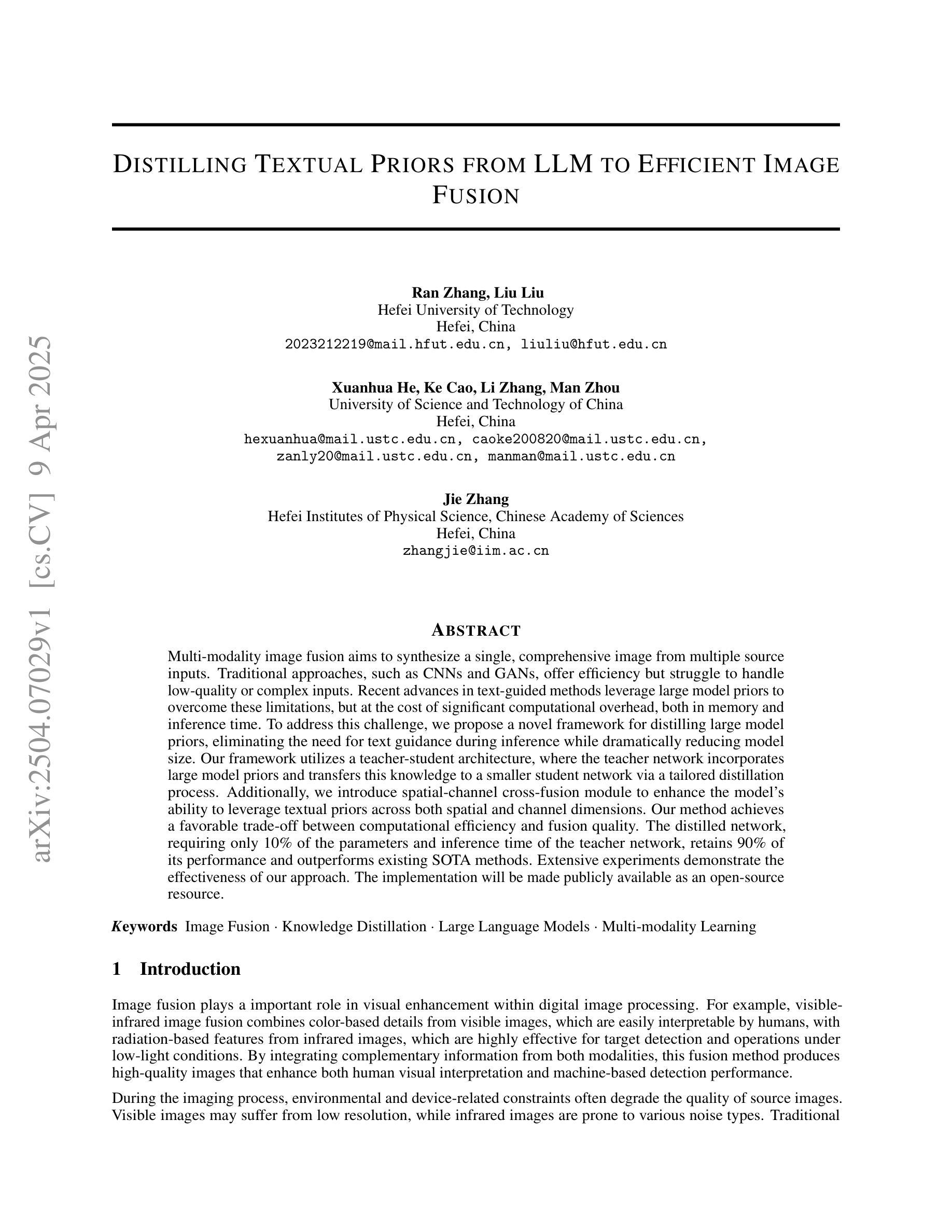
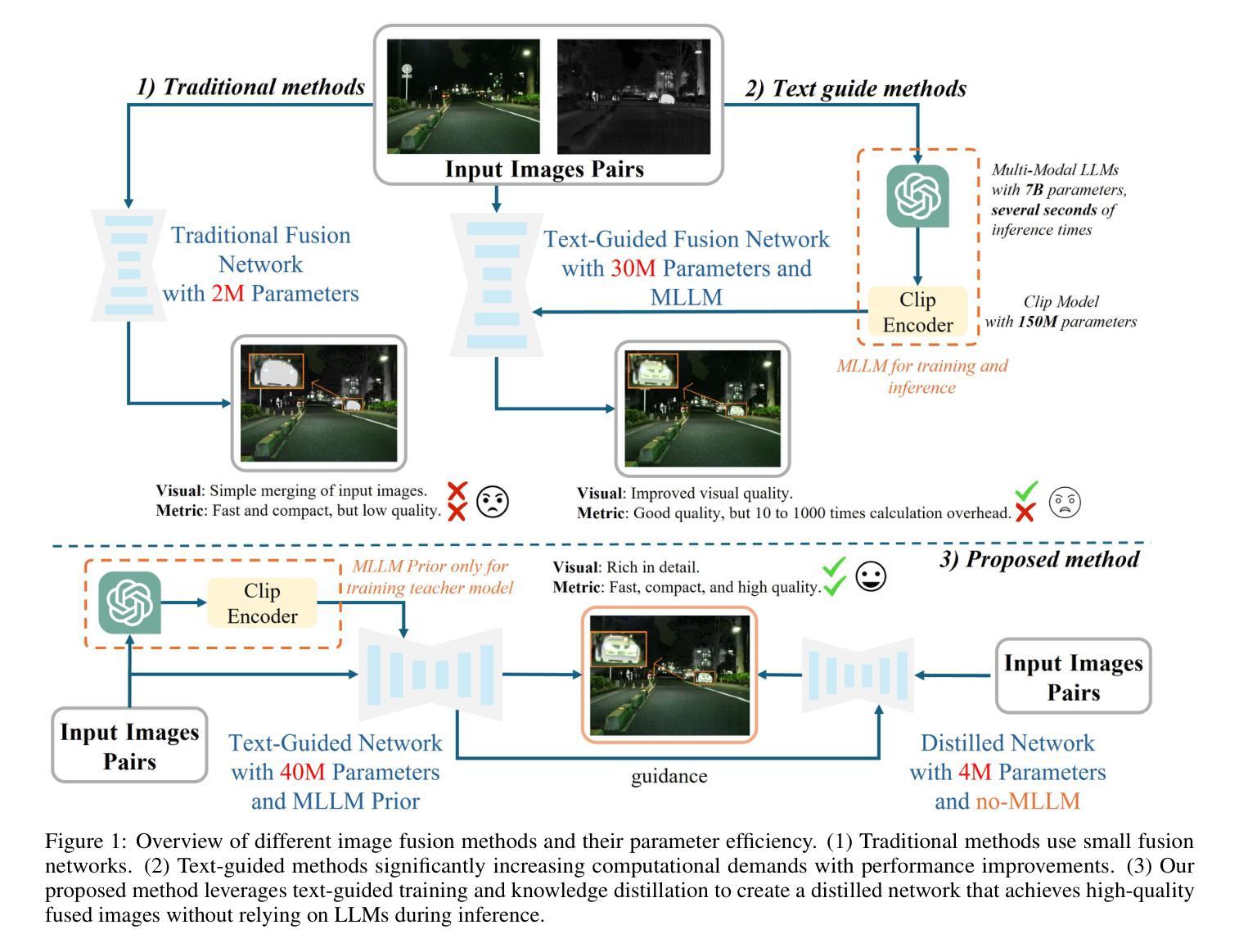
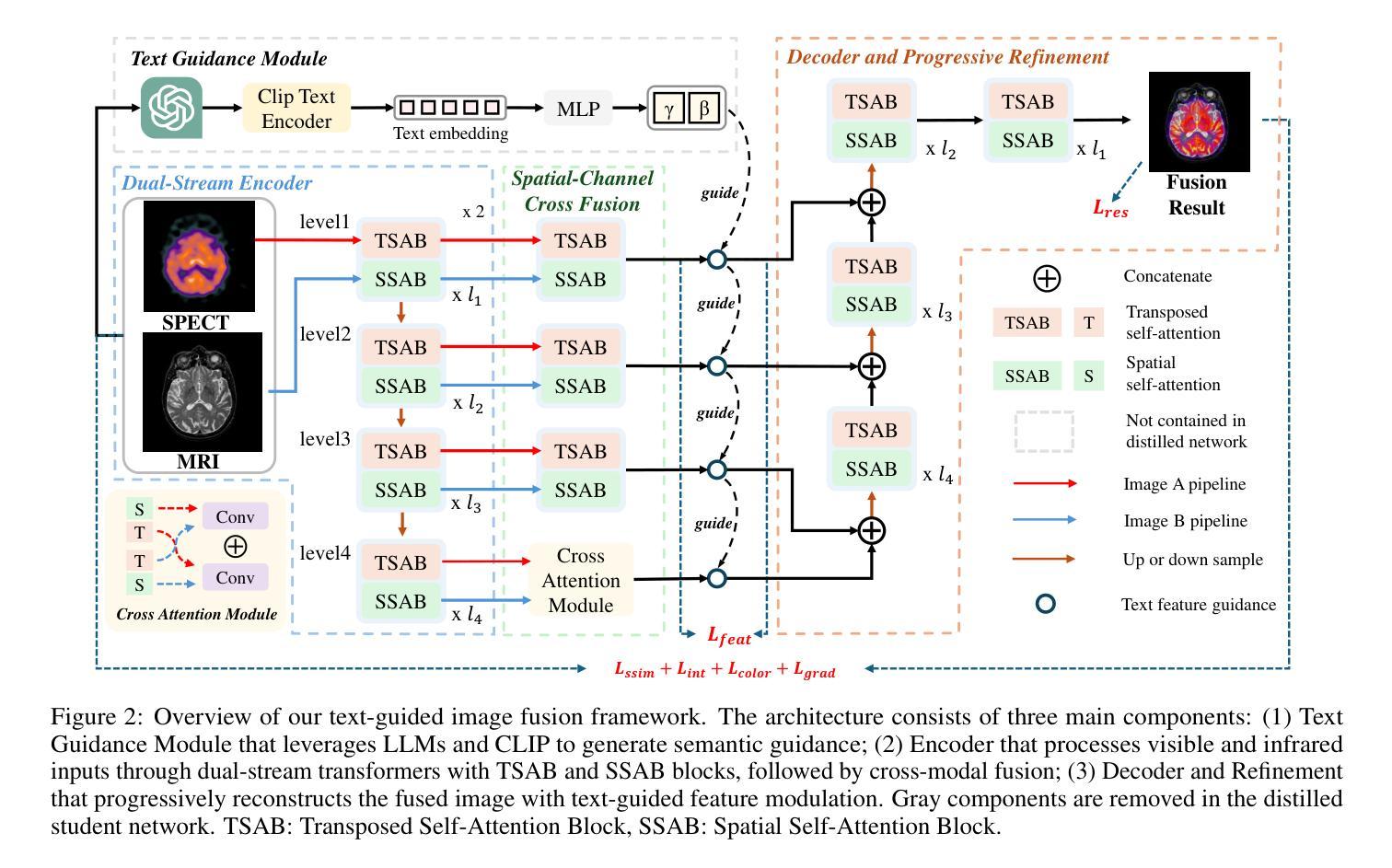
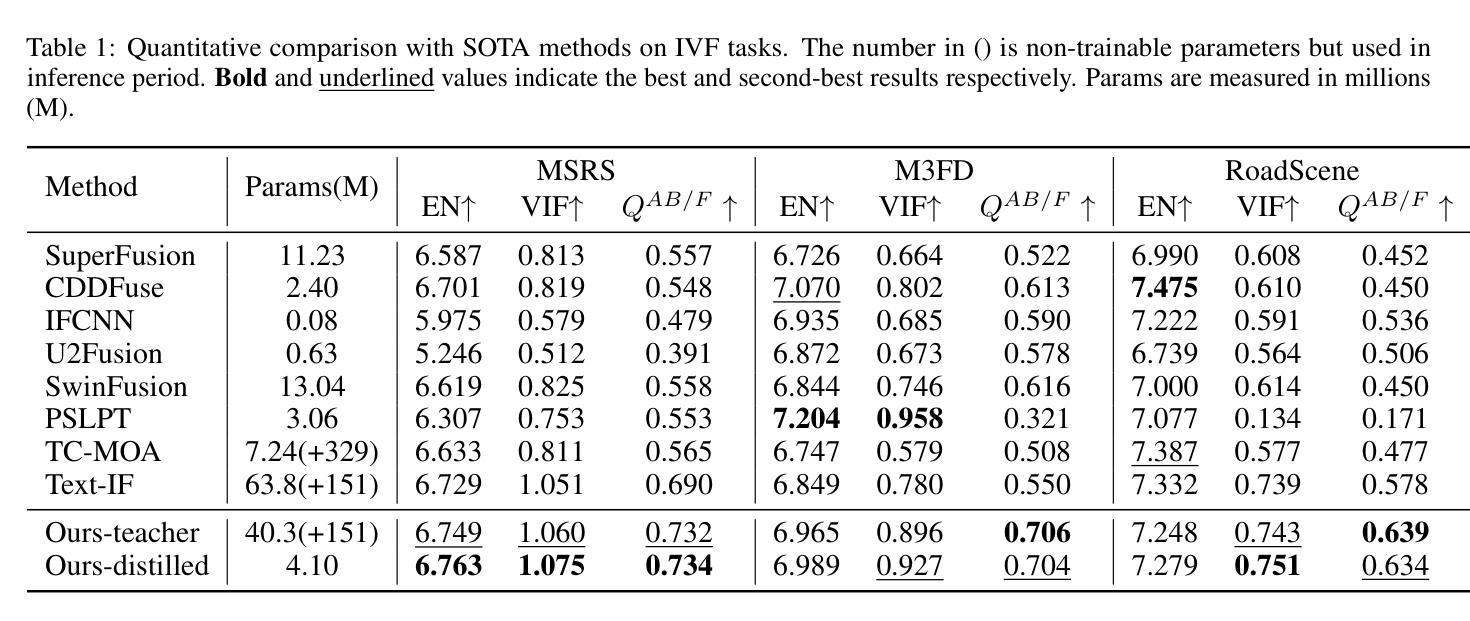