⚠️ 以下所有内容总结都来自于 大语言模型的能力,如有错误,仅供参考,谨慎使用
🔴 请注意:千万不要用于严肃的学术场景,只能用于论文阅读前的初筛!
💗 如果您觉得我们的项目对您有帮助 ChatPaperFree ,还请您给我们一些鼓励!⭐️ HuggingFace免费体验
2025-04-12 更新
GenEAva: Generating Cartoon Avatars with Fine-Grained Facial Expressions from Realistic Diffusion-based Faces
Authors:Hao Yu, Rupayan Mallick, Margrit Betke, Sarah Adel Bargal
Cartoon avatars have been widely used in various applications, including social media, online tutoring, and gaming. However, existing cartoon avatar datasets and generation methods struggle to present highly expressive avatars with fine-grained facial expressions and are often inspired from real-world identities, raising privacy concerns. To address these challenges, we propose a novel framework, GenEAva, for generating high-quality cartoon avatars with fine-grained facial expressions. Our approach fine-tunes a state-of-the-art text-to-image diffusion model to synthesize highly detailed and expressive facial expressions. We then incorporate a stylization model that transforms these realistic faces into cartoon avatars while preserving both identity and expression. Leveraging this framework, we introduce the first expressive cartoon avatar dataset, GenEAva 1.0, specifically designed to capture 135 fine-grained facial expressions, featuring 13,230 expressive cartoon avatars with a balanced distribution across genders, racial groups, and age ranges. We demonstrate that our fine-tuned model generates more expressive faces than the state-of-the-art text-to-image diffusion model SDXL. We also verify that the cartoon avatars generated by our framework do not include memorized identities from fine-tuning data. The proposed framework and dataset provide a diverse and expressive benchmark for future research in cartoon avatar generation.
卡通头像已广泛应用于各种应用程序,包括社交媒体、在线教学和游戏。然而,现有的卡通头像数据集和生成方法在呈现具有高度表情和细腻面部表情的头像方面存在困难,并且它们通常从真实身份中获得灵感,这引发了隐私担忧。为了解决这些挑战,我们提出了一种新型框架GenEAva,用于生成具有细腻面部表情的高质量卡通头像。我们的方法微调了最先进的文本到图像扩散模型,以合成高度详细和富有表现力的面部表情。然后,我们融入了一个风格化模型,将这些逼真的脸庞转化为卡通头像,同时保留身份和表情。借助这一框架,我们推出了首个富有表现力的卡通头像数据集GenEAva 1.0,专门设计用于捕捉135种细腻面部表情,包含13,230个富有表现力的卡通头像,在性别、种族和年龄范围方面平衡分布。我们证明,我们的微调模型比最先进的文本到图像扩散模型SDXL生成的面孔更具表现力。我们还验证了由我们框架生成的卡通头像不包括微调数据中的记忆身份。所提出的框架和数据集为卡通头像生成的未来研究提供了多样化和富有表现力的基准。
论文及项目相关链接
Summary
基于文本生成卡通头像的技术挑战,包括表情表达不足和隐私问题等,研究者提出了一种新型框架GenEAva。该框架结合了先进的文本到图像扩散模型和风格化模型,能够生成高质量、表情丰富的卡通头像数据集GenEAva 1.0。该数据集包含不同性别、种族和年龄分布的卡通头像,且不会出现身份泄露问题。研究证明,该框架生成的卡通头像表情更丰富,并且不包含训练数据中的身份信息。该框架和数据集为未来的卡通头像生成研究提供了多样化和丰富的基准。
Key Takeaways
- GenEAva框架结合了先进的文本到图像扩散模型和风格化模型,用于生成高质量、表情丰富的卡通头像。
- GenEAva 1.0数据集包含不同性别、种族和年龄分布的卡通头像,旨在捕捉面部表情的细节差异。
- GenEAva框架生成的卡通头像表情更丰富,超越了现有的技术。
- 该框架生成的卡通头像不会泄露训练数据中的身份信息,避免了隐私问题。
- 该框架和数据集为未来的卡通头像生成研究提供了多样化和丰富的基准。
点此查看论文截图
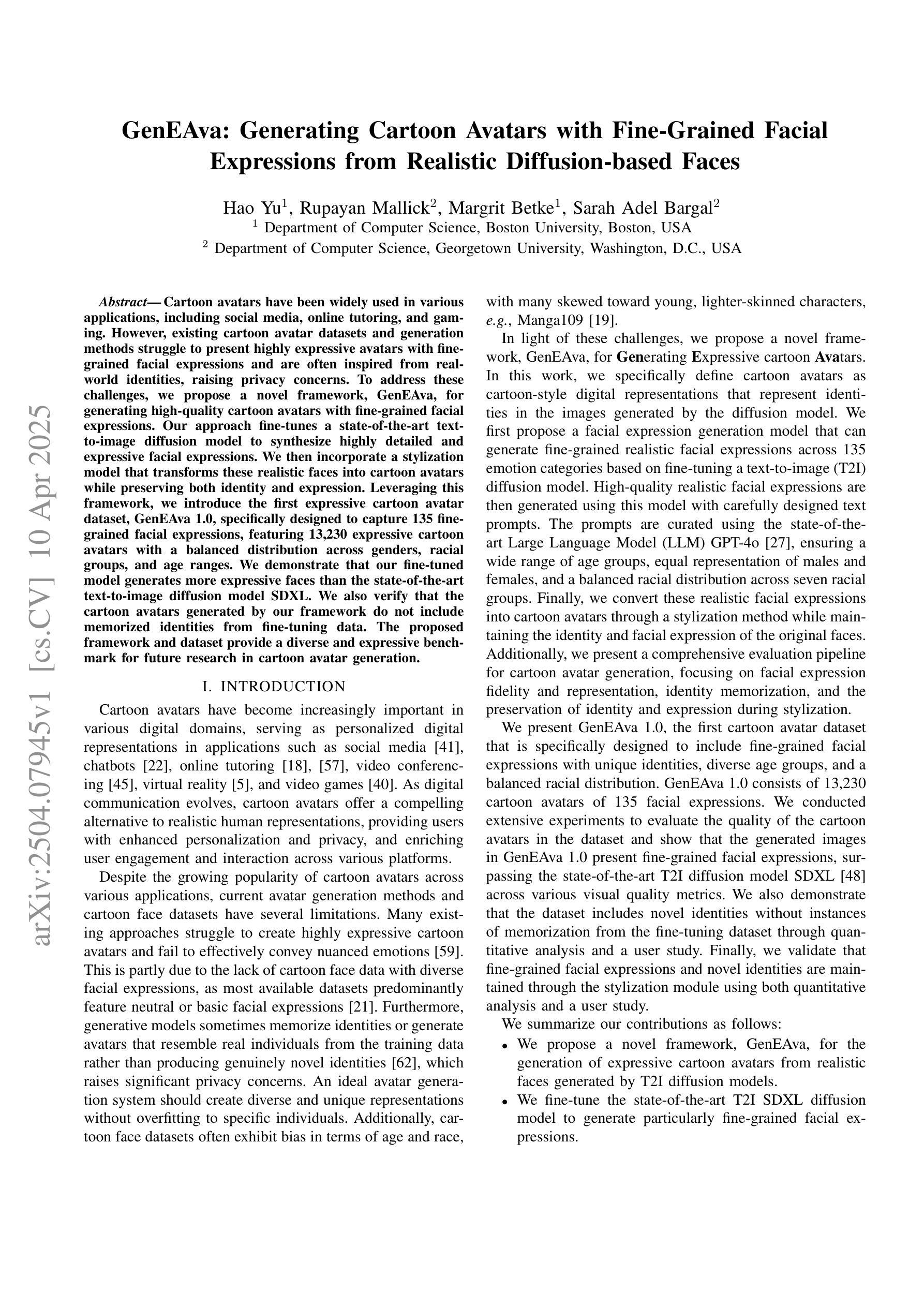
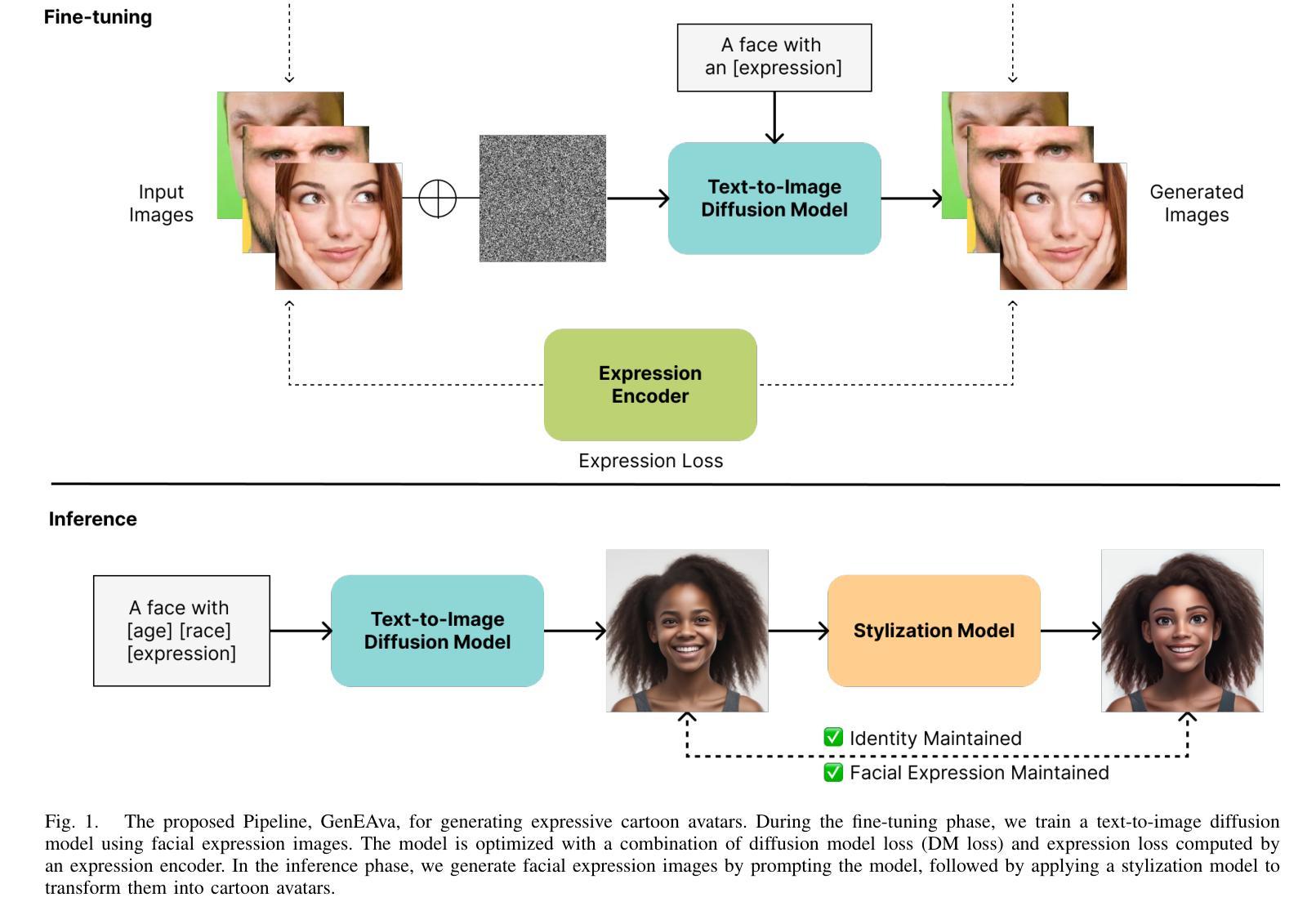
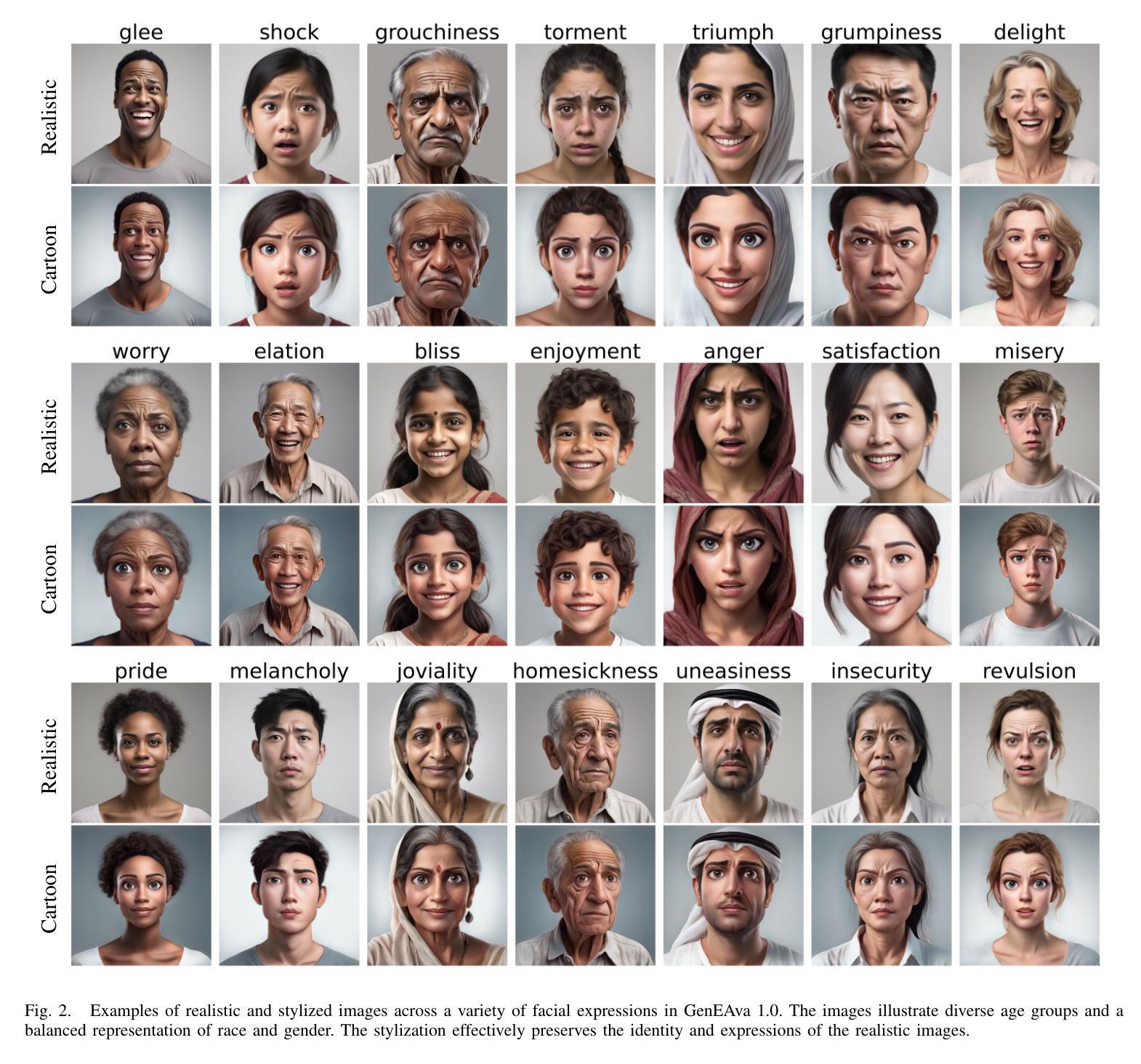
GIGA: Generalizable Sparse Image-driven Gaussian Avatars
Authors:Anton Zubekhin, Heming Zhu, Paulo Gotardo, Thabo Beeler, Marc Habermann, Christian Theobalt
Driving a high-quality and photorealistic full-body human avatar, from only a few RGB cameras, is a challenging problem that has become increasingly relevant with emerging virtual reality technologies. To democratize such technology, a promising solution may be a generalizable method that takes sparse multi-view images of an unseen person and then generates photoreal free-view renderings of such identity. However, the current state of the art is not scalable to very large datasets and, thus, lacks in diversity and photorealism. To address this problem, we propose a novel, generalizable full-body model for rendering photoreal humans in free viewpoint, as driven by sparse multi-view video. For the first time in literature, our model can scale up training to thousands of subjects while maintaining high photorealism. At the core, we introduce a MultiHeadUNet architecture, which takes sparse multi-view images in texture space as input and predicts Gaussian primitives represented as 2D texels on top of a human body mesh. Importantly, we represent sparse-view image information, body shape, and the Gaussian parameters in 2D so that we can design a deep and scalable architecture entirely based on 2D convolutions and attention mechanisms. At test time, our method synthesizes an articulated 3D Gaussian-based avatar from as few as four input views and a tracked body template for unseen identities. Our method excels over prior works by a significant margin in terms of cross-subject generalization capability as well as photorealism.
通过少数RGB摄像头实现高质量、真实感十足的全人身人物虚拟化身是一个颇具挑战性的问题,但随着虚拟现实技术的兴起,这个问题变得越来越重要。为了使这种技术走向大众化,一种可行的解决方案是采用通用方法,即对未见过的个体进行稀疏的多视角图像拍摄,然后生成此类身份的真实感自由视角渲染。然而,目前的技术并不能扩展到大规模数据集,因此在多样性和真实感方面存在不足。为了解决这个问题,我们提出了一种新型的通用全身模型,用于在自由视角下渲染真实感的人物形象,由稀疏的多视角视频驱动。我们的模型能够扩展到成千上万的主题进行训练,同时保持高度真实感,这在文献中是首次。我们的核心方法是引入MultiHeadUNet架构,它以稀疏多视角图像纹理空间作为输入,预测高斯基本单位,以人体网格上的二维纹理元素表示。重要的是,我们以二维方式表示稀疏视图图像信息、身体形态和二维高斯参数,这样我们就可以设计一个深度可伸缩的架构,完全基于二维卷积和注意力机制。在测试阶段,我们的方法能够从最多四个视角和跟踪的身体模板中合成基于高斯模型的灵活三维虚拟化身,用于未见过的身份。我们的方法在跨主题推广能力和真实感方面都大大超过了以前的工作。
论文及项目相关链接
PDF 14 pages, 10 figures, project page: https://vcai.mpi-inf.mpg.de/projects/GIGA
Summary
本文提出一种新型通用全身模型,用于在稀疏多视角视频驱动下渲染逼真人类。该模型采用MultiHeadUNet架构,能够在数千个主题上进行训练的同时保持高逼真度。通过输入稀疏的多视角图像纹理空间,预测基于高斯原语表示的二维人体网格上的texels。此方法能在测试时仅从四个视角和跟踪的身体模板合成具有艺术性的三维高斯基础化身,并在跨主题推广能力和逼真度上大大超过先前的工作。
Key Takeaways
- 提出一种新型通用全身模型,用于从稀疏多视角图像渲染逼真人类。
- 采用MultiHeadUNet架构,实现大规模数据集上的高逼真度训练。
- 输入稀疏的多视角图像纹理空间,预测二维人体网格上的texels,采用高斯原语表示。
- 模型能够从少数视角合成具有艺术性的三维高斯基础化身。
- 模型在跨主题推广能力和逼真度上显著优于先前的工作。
- 利用注意力机制和二维卷积设计深度可伸缩架构。
点此查看论文截图
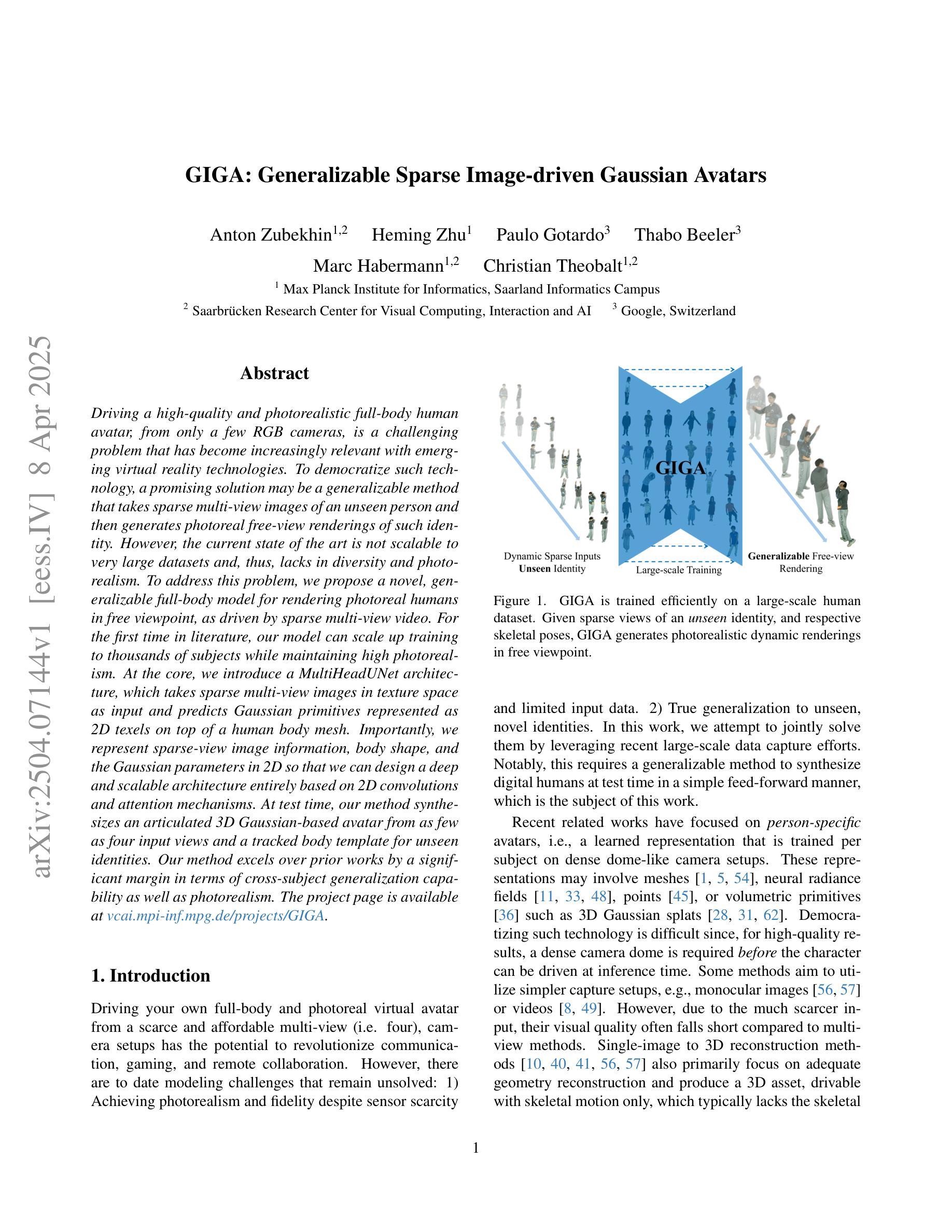
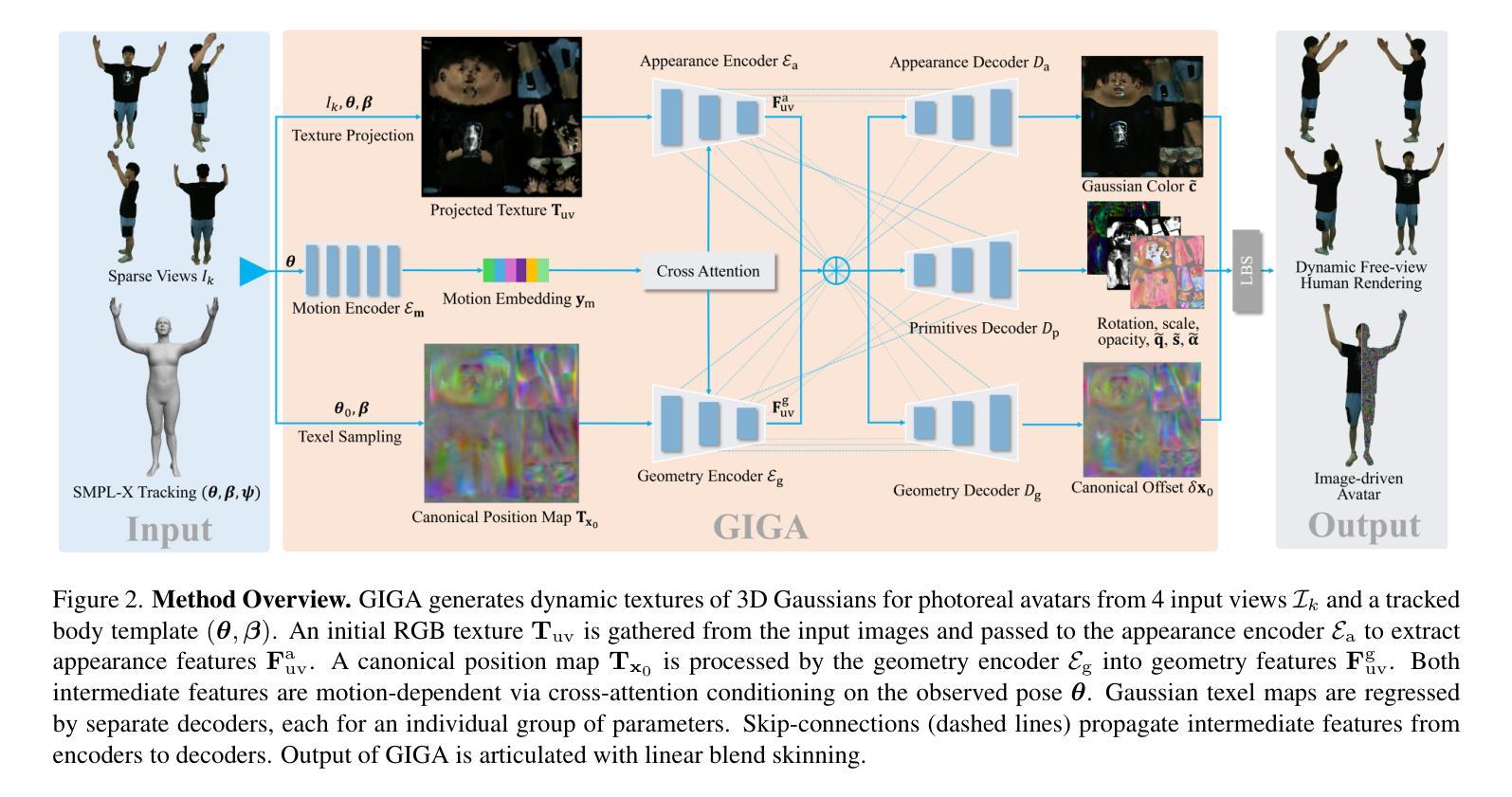
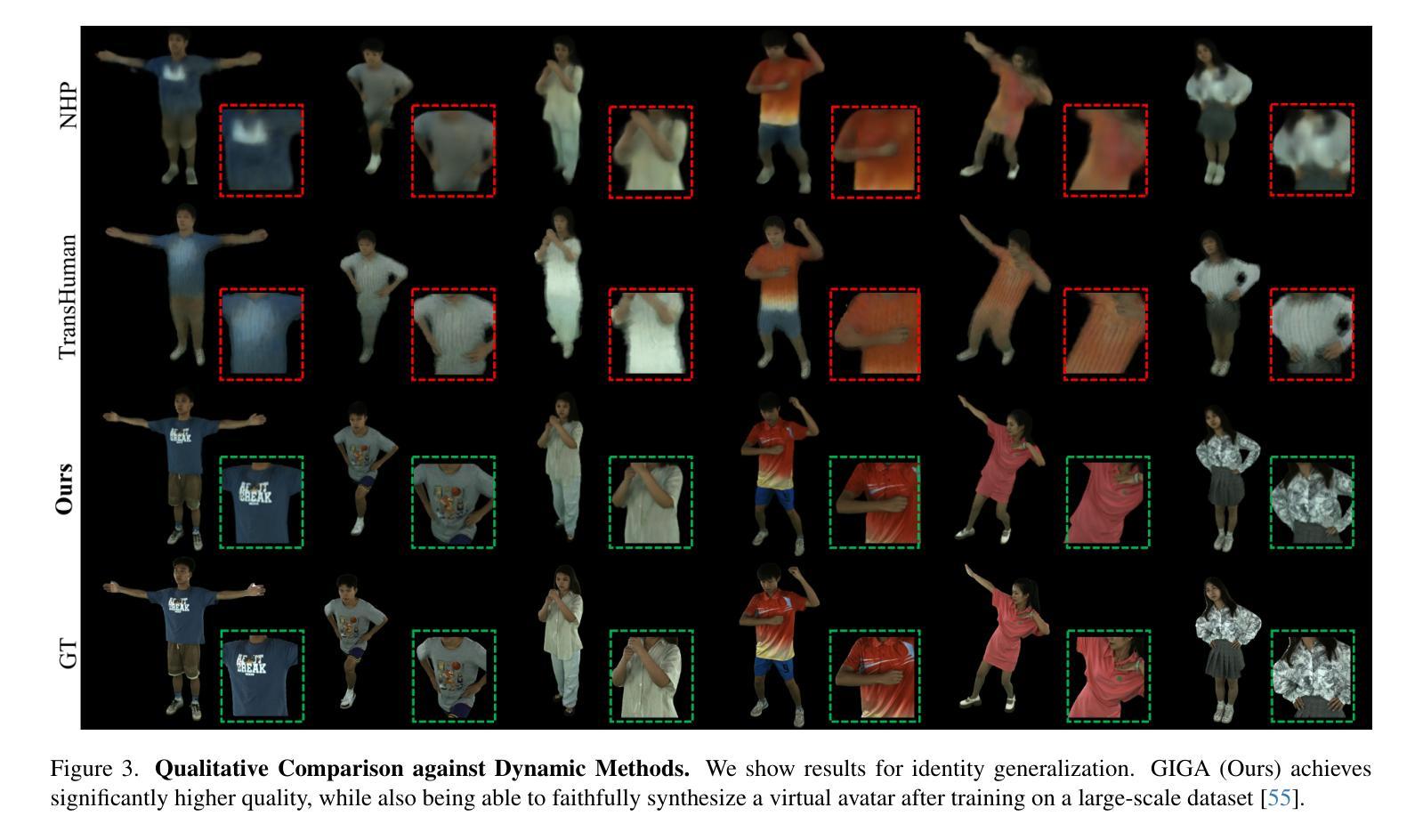
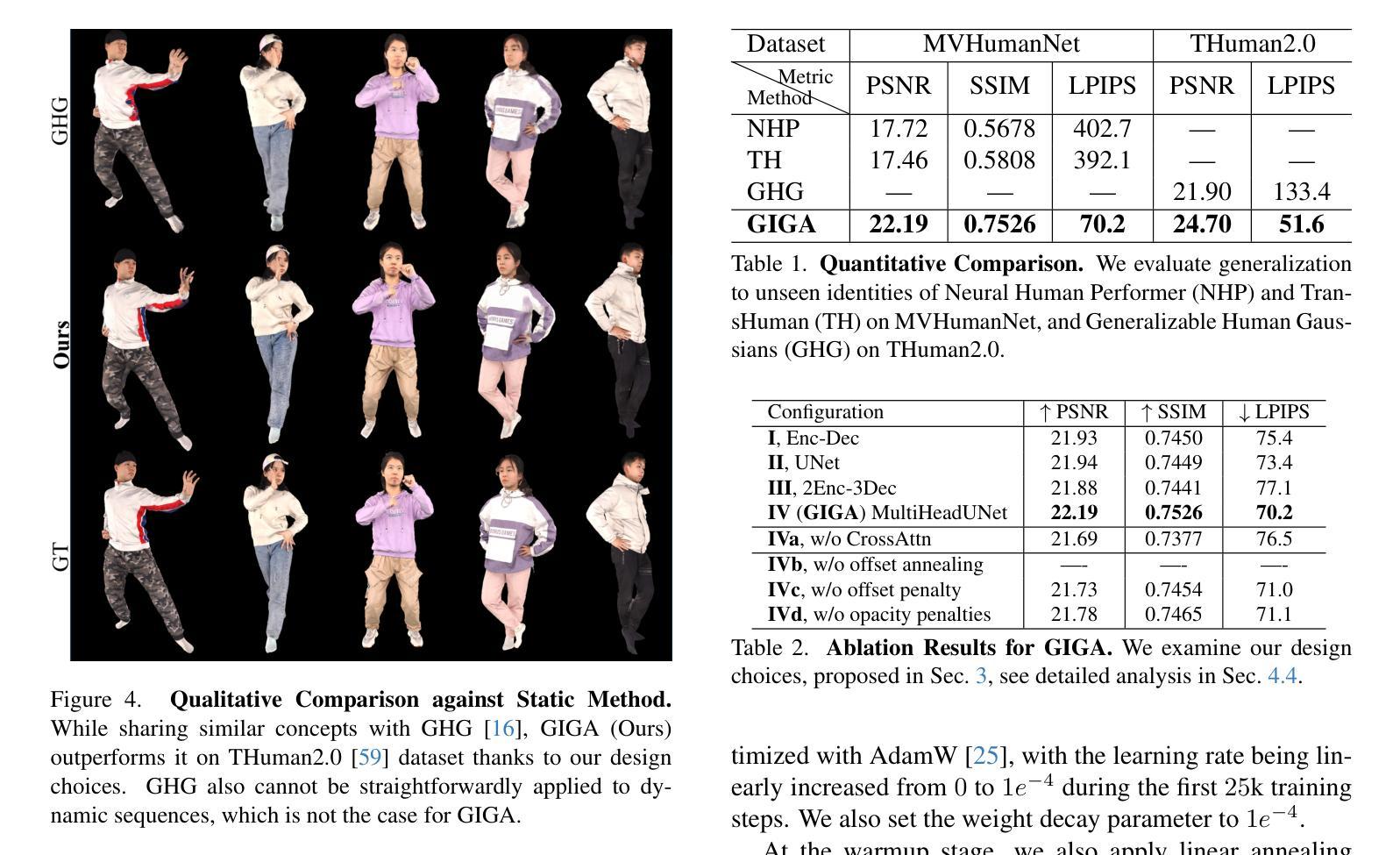