⚠️ 以下所有内容总结都来自于 大语言模型的能力,如有错误,仅供参考,谨慎使用
🔴 请注意:千万不要用于严肃的学术场景,只能用于论文阅读前的初筛!
💗 如果您觉得我们的项目对您有帮助 ChatPaperFree ,还请您给我们一些鼓励!⭐️ HuggingFace免费体验
2025-04-12 更新
PRAD: Periapical Radiograph Analysis Dataset and Benchmark Model Development
Authors:Zhenhuan Zhou, Yuchen Zhang, Ruihong Xu, Xuansen Zhao, Tao Li
Deep learning (DL), a pivotal technology in artificial intelligence, has recently gained substantial traction in the domain of dental auxiliary diagnosis. However, its application has predominantly been confined to imaging modalities such as panoramic radiographs and Cone Beam Computed Tomography, with limited focus on auxiliary analysis specifically targeting Periapical Radiographs (PR). PR are the most extensively utilized imaging modality in endodontics and periodontics due to their capability to capture detailed local lesions at a low cost. Nevertheless, challenges such as resolution limitations and artifacts complicate the annotation and recognition of PR, leading to a scarcity of publicly available, large-scale, high-quality PR analysis datasets. This scarcity has somewhat impeded the advancement of DL applications in PR analysis. In this paper, we present PRAD-10K, a dataset for PR analysis. PRAD-10K comprises 10,000 clinical periapical radiograph images, with pixel-level annotations provided by professional dentists for nine distinct anatomical structures, lesions, and artificial restorations or medical devices, We also include classification labels for images with typical conditions or lesions. Furthermore, we introduce a DL network named PRNet to establish benchmarks for PR segmentation tasks. Experimental results demonstrate that PRNet surpasses previous state-of-the-art medical image segmentation models on the PRAD-10K dataset. The codes and dataset will be made publicly available.
深度学习(DL)是人工智能中的一项关键技术,最近在牙科辅助诊断领域获得了大量的关注和应用。然而,其主要的应用主要集中在全景X线摄影和锥形束计算机断层扫描等成像模式上,对于针对根尖周射片(PR)的辅助分析关注较少。由于根尖周射片能够以较低的成本捕捉到详细的局部病变,因此它是牙髓学和牙周病学中应用最广泛的成像方式。然而,分辨率限制和伪影等挑战使得根尖周射片的标注和识别变得复杂,导致公开可用的大规模高质量PR分析数据集稀缺。这种稀缺状况在一定程度上阻碍了深度学习在PR分析中的应用发展。在本文中,我们介绍了PRAD-10K数据集,该数据集用于PR分析。PRAD-10K包含10,000张临床根尖周射片图像,由专业牙医提供像素级标注,涵盖九种不同的解剖结构、病变以及人工修复体或医疗设备。我们还为典型状况或病变的图像提供了分类标签。此外,我们还引入了一个名为PRNet的深度学习网络,为PR分割任务建立基准测试。实验结果表明,PRNet在PRAD-10K数据集上的表现超过了之前的顶尖医疗图像分割模型。代码和数据集将公开可用。
论文及项目相关链接
PDF 11 pages & Under Review
摘要
深度学习技术在牙科辅助诊断领域的应用日益广泛,但主要局限于全景放射影像和锥形束计算机断层扫描等成像方式。针对根尖周放射影像(PR)的辅助分析相对较少。PR是牙髓病学和牙周病学中最广泛使用的成像方式,因其能低价捕捉局部详细病变。然而,分辨率限制和人工制品等挑战使得PR的标注和识别变得复杂,缺乏公开的大规模高质量PR分析数据集。本文提出了PRAD-10K数据集,包含10,000张临床根尖周放射影像图像,由专业牙医对九种不同的解剖结构、病变、人工修复体或医疗设备进行像素级标注。此外,还包括典型状况或病变的图像分类标签。本文还介绍了一种名为PRNet的深度学习网络,为PR分割任务建立基准。实验结果表明,PRNet在PRAD-10K数据集上的表现优于先前的最先进的医学图像分割模型。代码和数据集将公开发布。
关键见解
- 深度学习在牙科辅助诊断中的应用主要局限于成像方式如全景放射影像和锥形束计算机断层扫描。
- 根尖周放射影像(PR)在牙髓病学和牙周病学中广泛使用,但标注和识别存在挑战。
- 缺乏公开的大规模高质量PR分析数据集。
- 提出了PRAD-10K数据集,包含10,000张临床PR图像,并由专业牙医进行像素级标注。
- 介绍了名为PRNet的深度学习网络,为PR分割任务提供基准。
- PRNet在PRAD-10K数据集上的表现优于其他医学图像分割模型。
- 代码和数据集将公开发布,有助于推动未来的研究。
点此查看论文截图
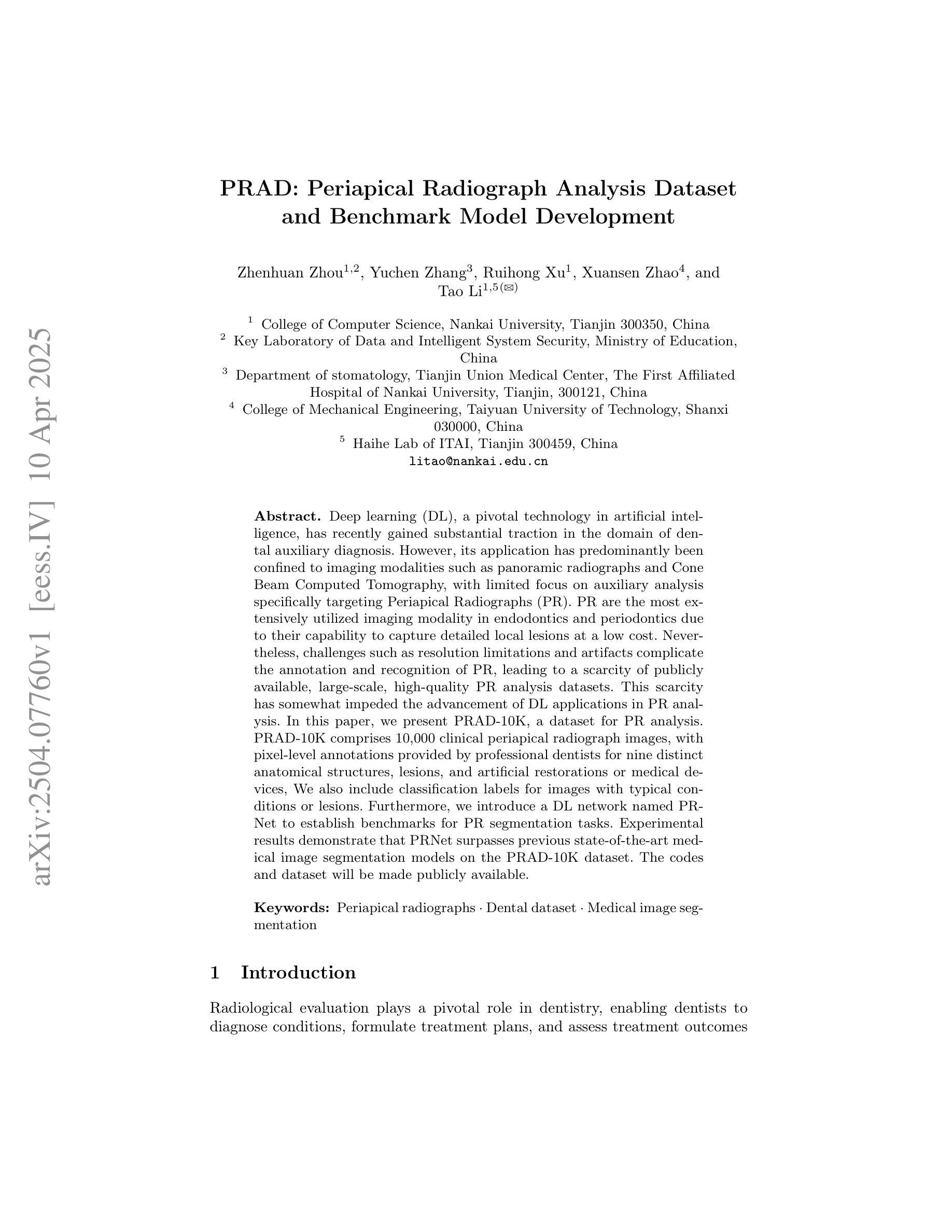
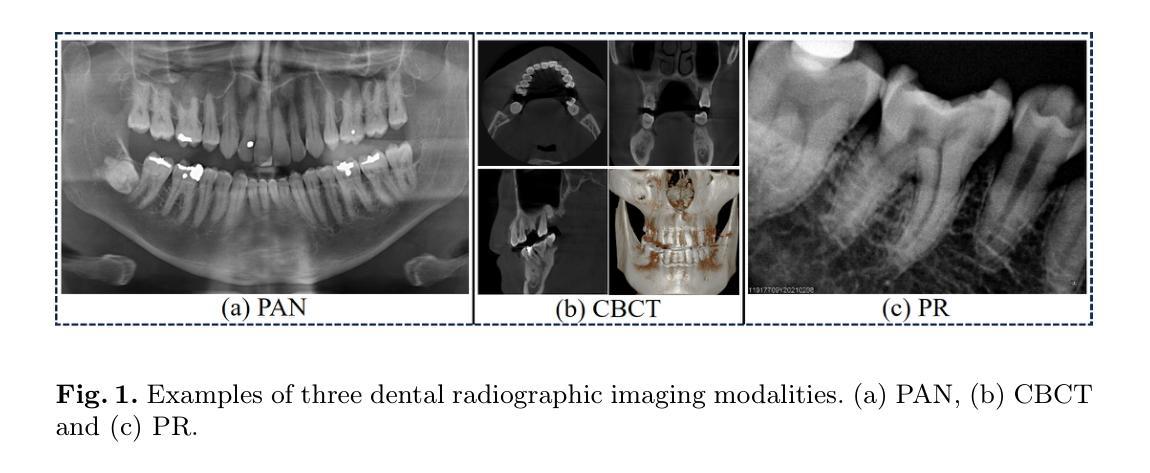
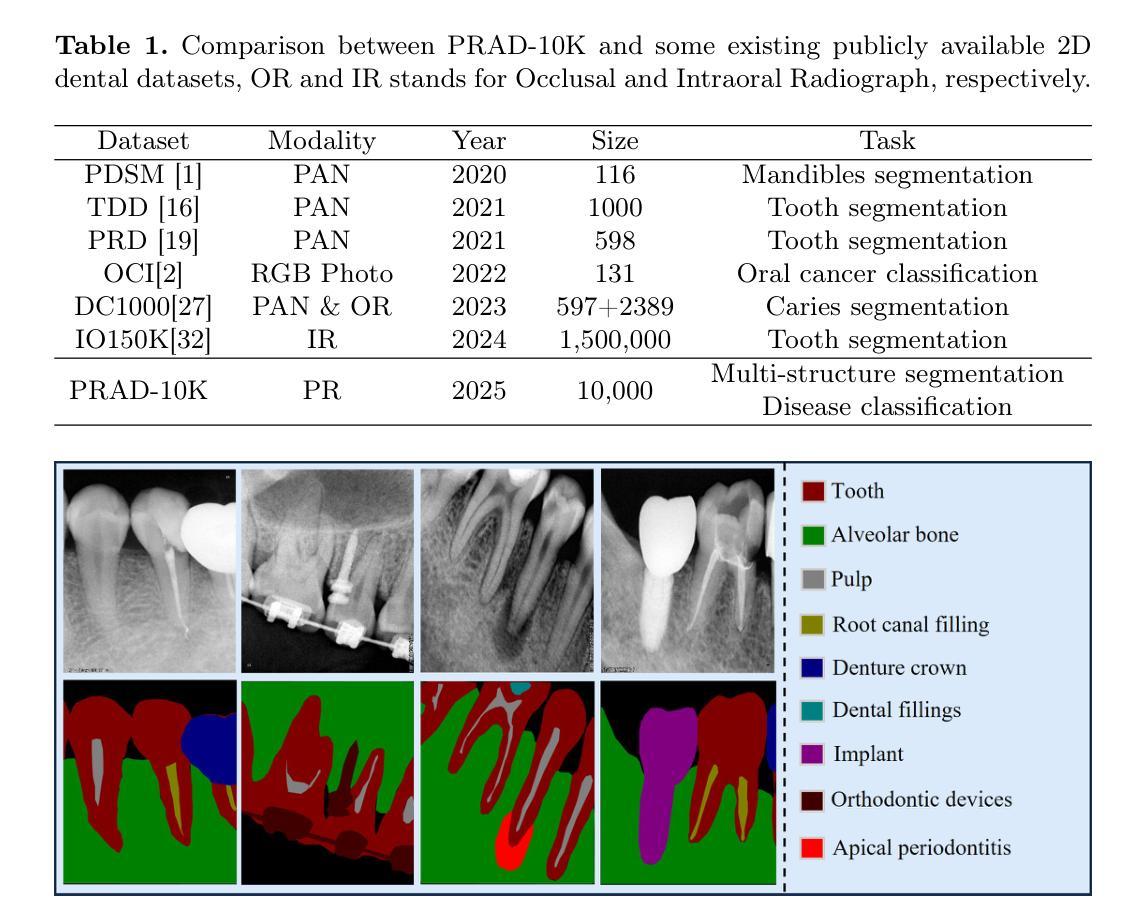
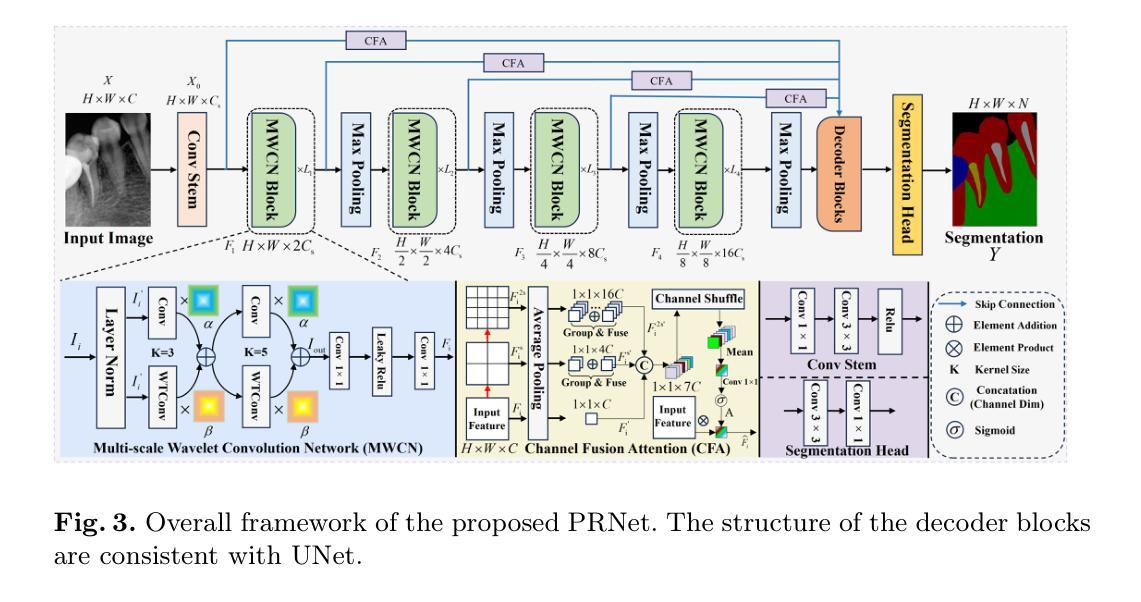
nnLandmark: A Self-Configuring Method for 3D Medical Landmark Detection
Authors:Alexandra Ertl, Shuhan Xiao, Stefan Denner, Robin Peretzke, David Zimmerer, Peter Neher, Fabian Isensee, Klaus Maier-Hein
Landmark detection plays a crucial role in medical imaging tasks that rely on precise spatial localization, including specific applications in diagnosis, treatment planning, image registration, and surgical navigation. However, manual annotation is labor-intensive and requires expert knowledge. While deep learning shows promise in automating this task, progress is hindered by limited public datasets, inconsistent benchmarks, and non-standardized baselines, restricting reproducibility, fair comparisons, and model generalizability. This work introduces nnLandmark, a self-configuring deep learning framework for 3D medical landmark detection, adapting nnU-Net to perform heatmap-based regression. By leveraging nnU-Net’s automated configuration, nnLandmark eliminates the need for manual parameter tuning, offering out-of-the-box usability. It achieves state-of-the-art accuracy across two public datasets, with a mean radial error (MRE) of 1.5 mm on the Mandibular Molar Landmark (MML) dental CT dataset and 1.2 mm for anatomical fiducials on a brain MRI dataset (AFIDs), where nnLandmark aligns with the inter-rater variability of 1.5 mm. With its strong generalization, reproducibility, and ease of deployment, nnLandmark establishes a reliable baseline for 3D landmark detection, supporting research in anatomical localization and clinical workflows that depend on precise landmark identification. The code will be available soon.
地标检测在依赖精确空间定位的医学成像任务中起着至关重要的作用,包括诊断、治疗计划、图像注册和手术导航等特定应用。然而,手动注释需要耗费大量劳动力且需要专业知识。深度学习虽然在自动化此任务方面显示出潜力,但公共数据集的有限性、基准测试的不一致性和非标准化基准等因素阻碍了其进展,限制了可重复性、公平比较和模型的通用性。这项工作介绍了nnLandmark,这是一个用于3D医学地标检测的深度学习框架,它通过采用nnU-Net进行基于热图的回归来实现自我配置。nnLandmark利用nnU-Net的自动配置功能,消除了对手动参数调整的需求,提供开箱即用的可用性。在公共数据集中达到了业界前沿的准确度,在下颌磨牙地标(MML)牙科CT数据集上的平均径向误差(MRE)为1.5毫米,在解剖标记物脑MRI数据集(AFIDs)上为1.2毫米,nnLandmark与平均组内差异评估的误差为毫米级(通常为小于或等于两毫米)。凭借其强大的通用性、可重复性和易于部署的特点,nnLandmark为可靠的基线提供了可靠的基线支持,用于依赖精确地标识别的解剖定位和临床工作流程研究。代码很快将发布。
论文及项目相关链接
摘要
基于深度学习的三维医学标志点检测框架nnLandmark问世。它借助nnU-Net的自适应配置,实现热点图回归,省去了手动参数调整,具有开箱即用的特性。在两组公开数据集上取得了最先进的准确度,其中在下颌磨牙标志点牙科CT数据集上的平均径向误差(MRE)为1.5毫米,脑MRI数据集上的解剖基准点平均径向误差为1.2毫米。nnLandmark与可靠的解剖学匹配标准相比具有良好通用性、可重复性和易于部署的特点,为依赖于精确标志点识别的解剖定位和临床工作流程研究建立了可靠的基准线。代码即将发布。
关键见解
- nnLandmark是一个用于三维医学标志点检测的深度学习框架,基于nnU-Net进行热点图回归。
- nnLandmark消除了手动参数调整的需要,具有开箱即用的特性。
- nnLandmark在公开数据集上取得了最先进的准确度表现。在特定的牙科CT和MRI数据集上,其平均径向误差低于或接近手动标注的误差范围。
- nnLandmark具有良好的通用性,能够在不同的医学图像数据集中识别标志点。这显示了其适应不同研究和临床需求的潜力。
- nnLandmark有助于实现精确的解剖定位,从而改进诊断和治疗计划等任务。精确的解剖定位是许多医疗程序成功的关键。
点此查看论文截图
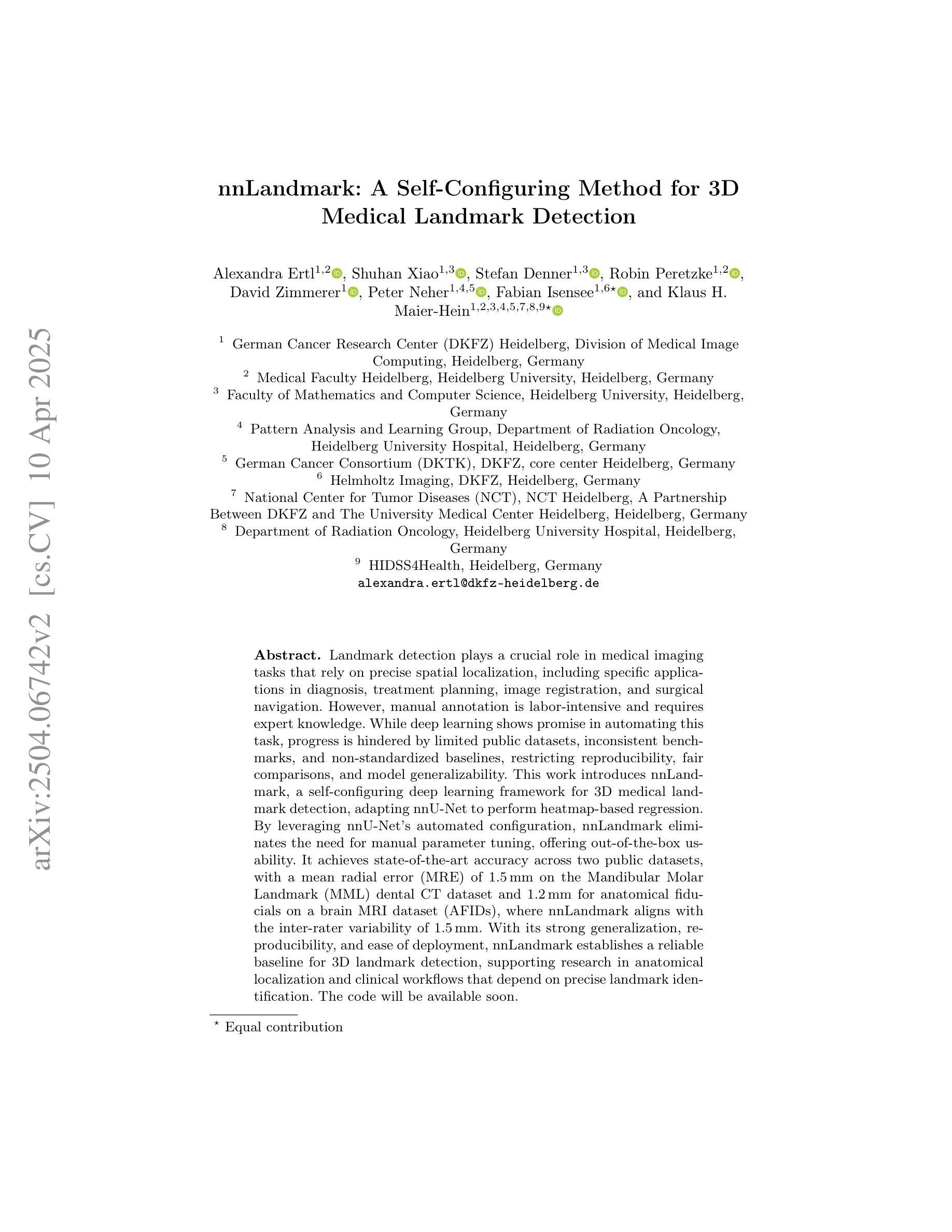
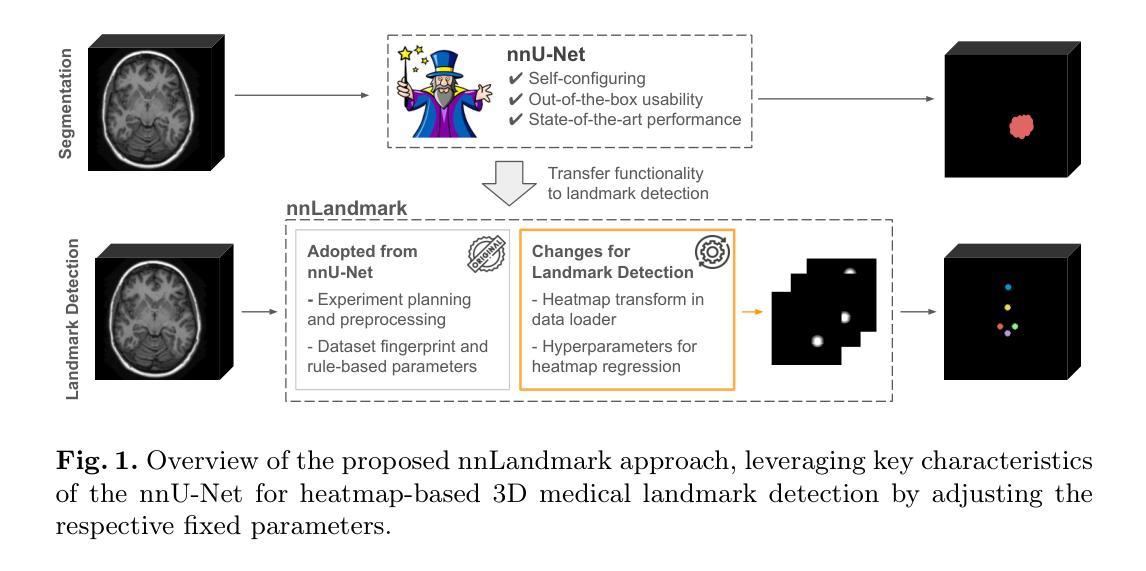
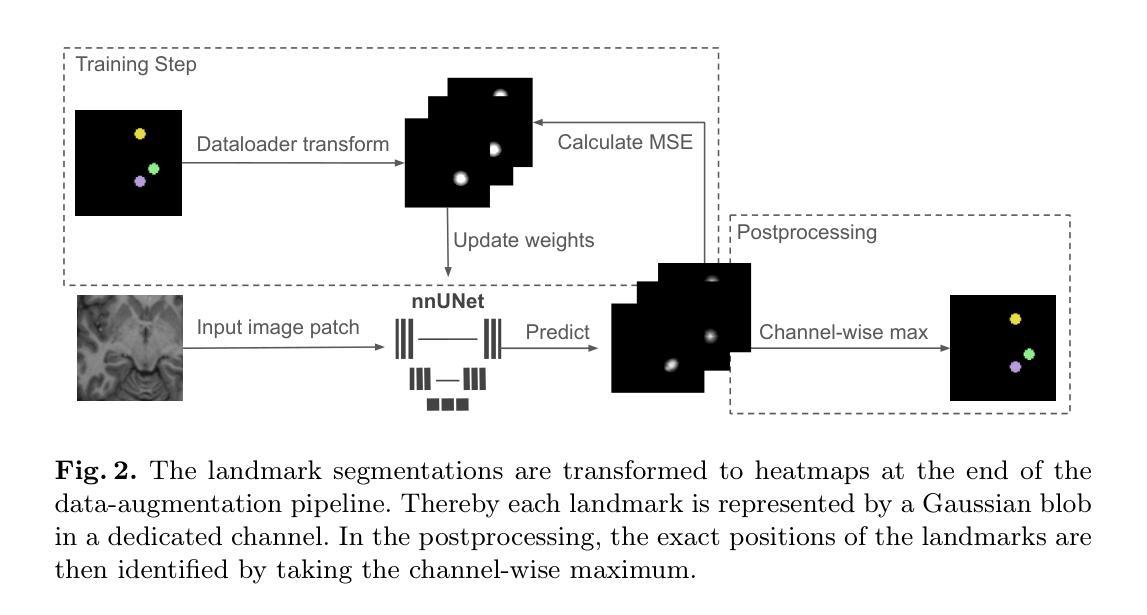
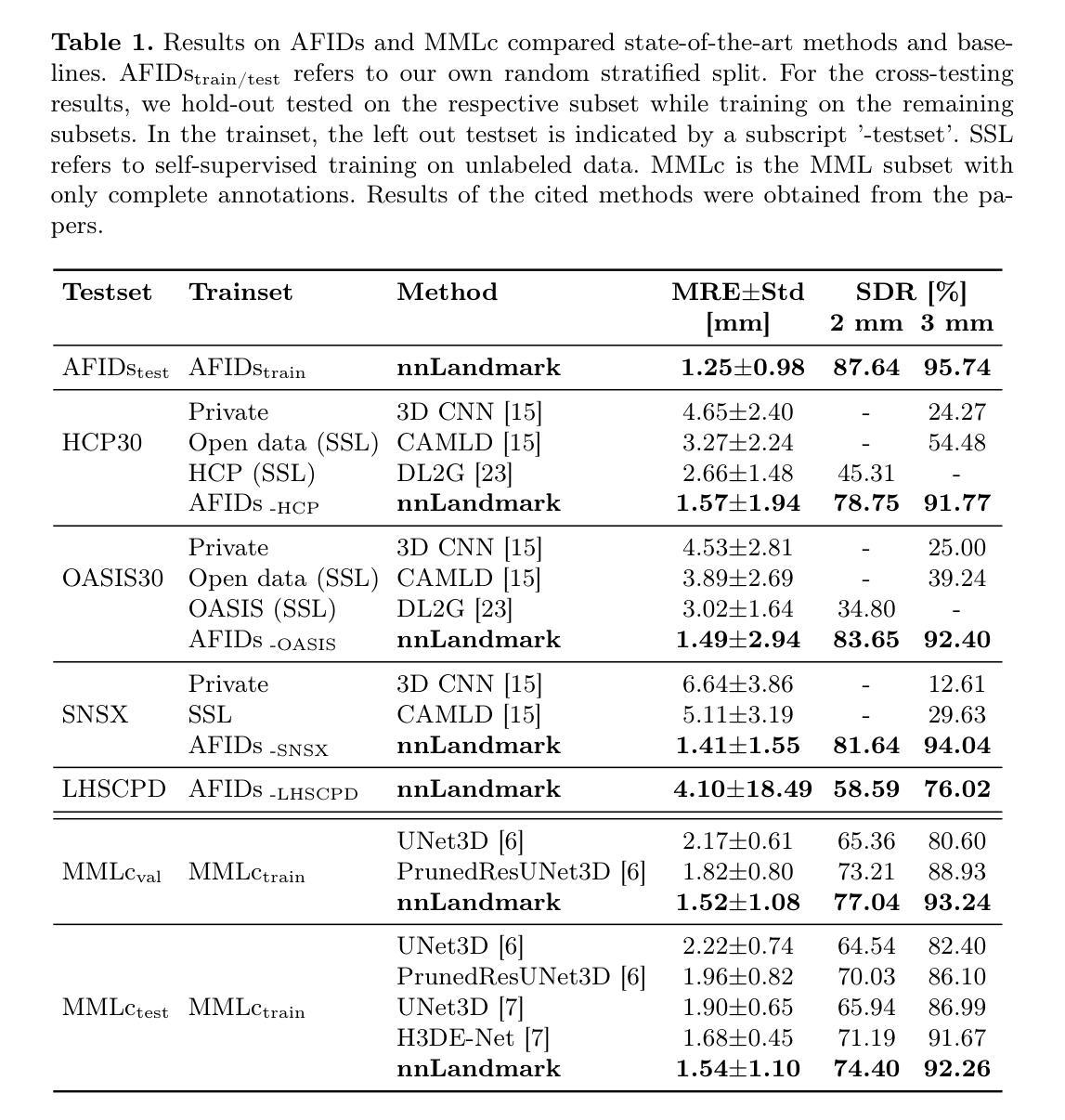