⚠️ 以下所有内容总结都来自于 大语言模型的能力,如有错误,仅供参考,谨慎使用
🔴 请注意:千万不要用于严肃的学术场景,只能用于论文阅读前的初筛!
💗 如果您觉得我们的项目对您有帮助 ChatPaperFree ,还请您给我们一些鼓励!⭐️ HuggingFace免费体验
2025-04-12 更新
SuperQ-GRASP: Superquadrics-based Grasp Pose Estimation on Larger Objects for Mobile-Manipulation
Authors:Xun Tu, Karthik Desingh
Grasp planning and estimation have been a longstanding research problem in robotics, with two main approaches to find graspable poses on the objects: 1) geometric approach, which relies on 3D models of objects and the gripper to estimate valid grasp poses, and 2) data-driven, learning-based approach, with models trained to identify grasp poses from raw sensor observations. The latter assumes comprehensive geometric coverage during the training phase. However, the data-driven approach is typically biased toward tabletop scenarios and struggle to generalize to out-of-distribution scenarios with larger objects (e.g. chair). Additionally, raw sensor data (e.g. RGB-D data) from a single view of these larger objects is often incomplete and necessitates additional observations. In this paper, we take a geometric approach, leveraging advancements in object modeling (e.g. NeRF) to build an implicit model by taking RGB images from views around the target object. This model enables the extraction of explicit mesh model while also capturing the visual appearance from novel viewpoints that is useful for perception tasks like object detection and pose estimation. We further decompose the NeRF-reconstructed 3D mesh into superquadrics (SQs) – parametric geometric primitives, each mapped to a set of precomputed grasp poses, allowing grasp composition on the target object based on these primitives. Our proposed pipeline overcomes the problems: a) noisy depth and incomplete view of the object, with a modeling step, and b) generalization to objects of any size. For more qualitative results, refer to the supplementary video and webpage https://bit.ly/3ZrOanU
抓取规划和估计是机器人技术中的一个长期研究问题,主要有两种方法来确定物体上的可抓取姿势:1)几何方法,它依赖于物体的3D模型和夹具来估计有效的抓取姿势;2)数据驱动、基于学习的方法,通过模型从原始传感器观测数据中识别抓取姿势。后者假设在训练阶段具有全面的几何覆盖。然而,数据驱动的方法通常偏向于桌面场景,在面向大型物体(例如椅子)的非常规场景中难以推广。此外,来自这些大型物体单一视角的原始传感器数据(例如RGB-D数据)通常是不完整的,需要额外的观察。在本文中,我们采用几何方法,利用物体建模的最新进展(例如NeRF),通过采集目标物体周围视角的RGB图像来构建隐式模型。该模型能够提取显式网格模型,同时从新颖的视角捕捉视觉外观,这对于物体检测和姿态估计等感知任务非常有用。我们进一步将NeRF重建的3D网格分解为超级二次曲面(SQs)——参数化几何基元,每个基元映射到一组预计算的抓取姿势,从而允许基于这些基元对目标物体进行抓取组合。我们提出的管道克服了以下问题:a)物体的噪声深度和不完全视图,通过建模步骤;b)推广到任何大小的物体。有关更多定性结果,请参阅补充视频和网页https://bit.ly/3ZrOanU。
论文及项目相关链接
PDF 8 pages, 7 figures, accepted by ICRA 2025
Summary
基于NeRF技术的几何方法,利用RGB图像构建目标对象的隐式模型,提取显式的三维网格模型,并分解为超二次曲面(SQs)。每个SQ映射到一组预计算的抓取姿态,实现了基于这些几何原语的目标对象抓取组合。该方法克服了对象深度噪声和不完全观察的建模问题,并推广至任意大小的对象抓取任务。
Key Takeaways
- 该方法采用几何方法解决机器人的抓取规划和估计问题。
- 利用NeRF技术构建目标对象的隐式模型,进而提取显式的三维网格模型。
- 通过将网格模型分解为超二次曲面(SQs),提高了抓取的灵活性和适应性。
- 每个SQ映射到一组预计算的抓取姿态,实现基于几何原语的抓取组合。
- 方法克服了对象深度噪声和不完全观察的建模问题。
- 该方法能够推广至任意大小的对象抓取任务。
点此查看论文截图
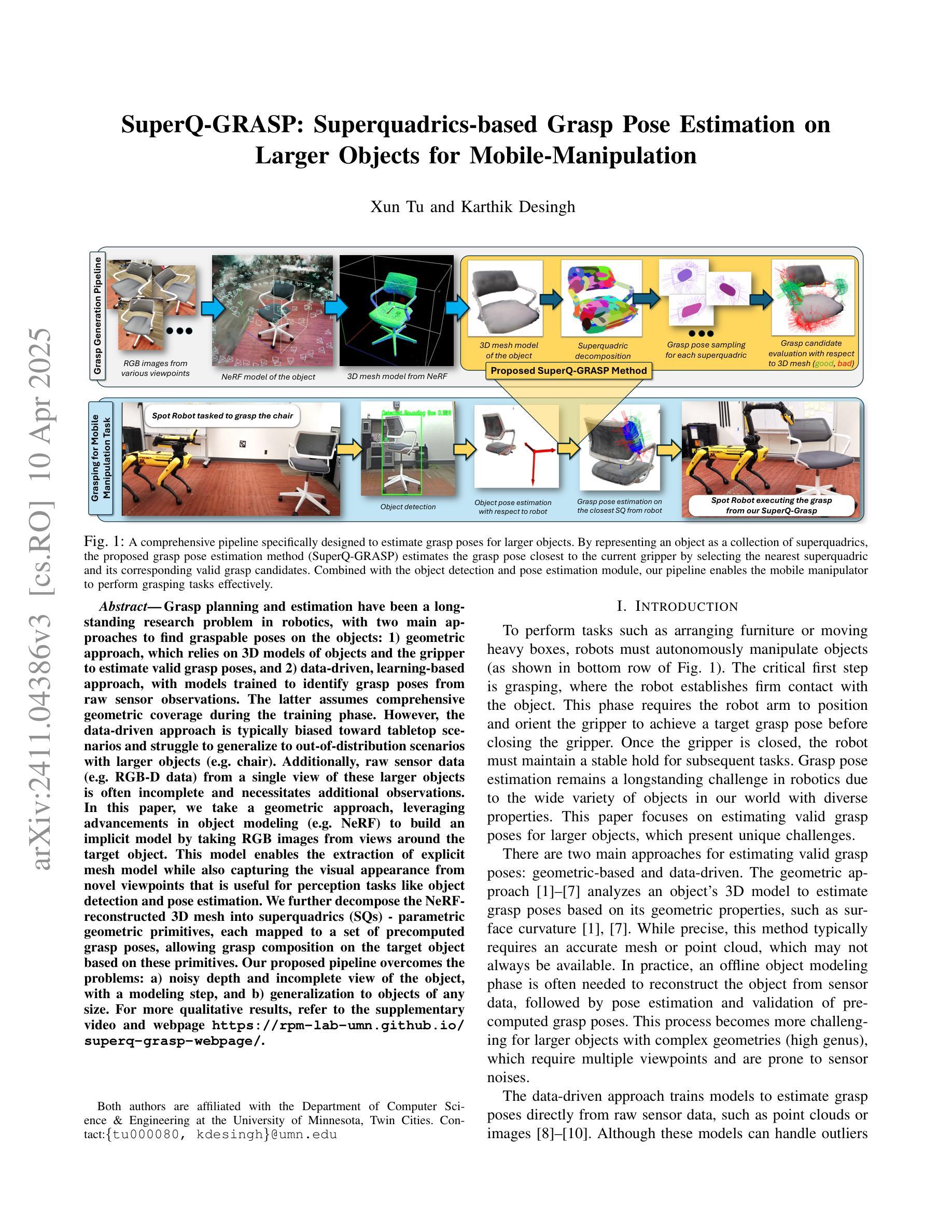
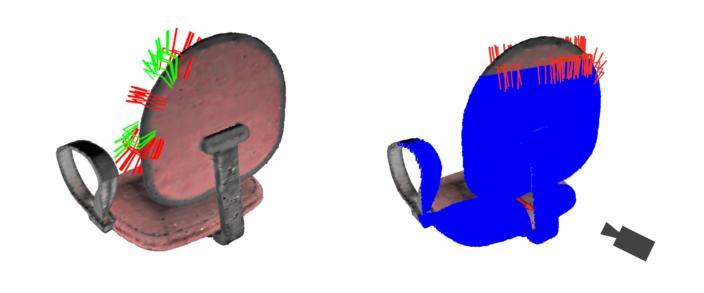
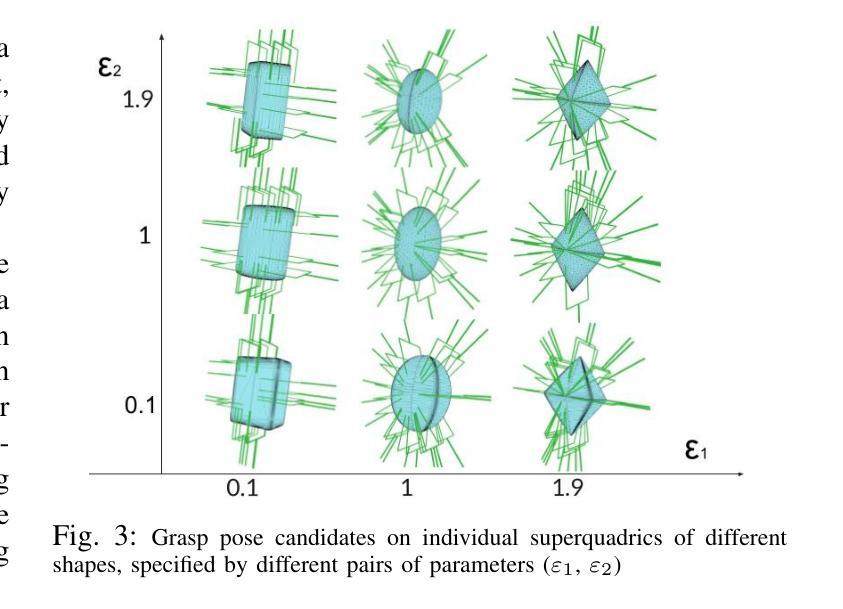
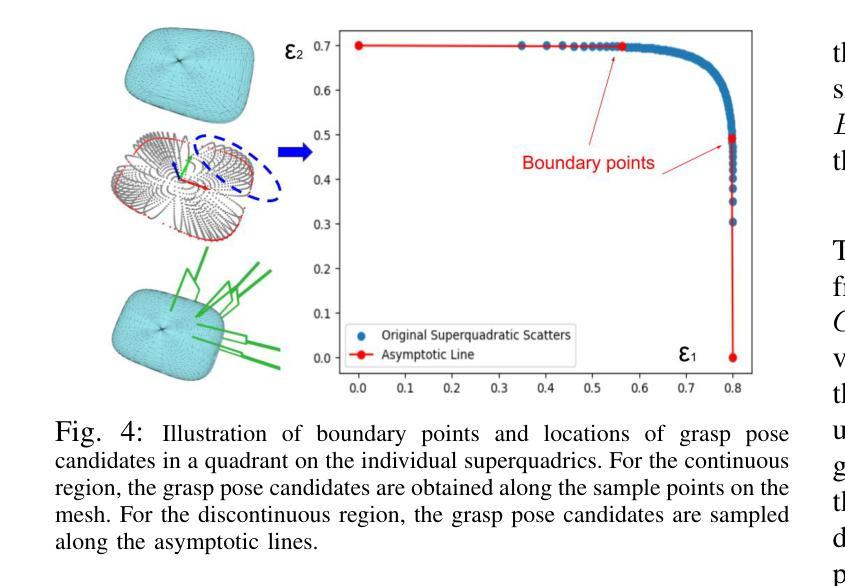
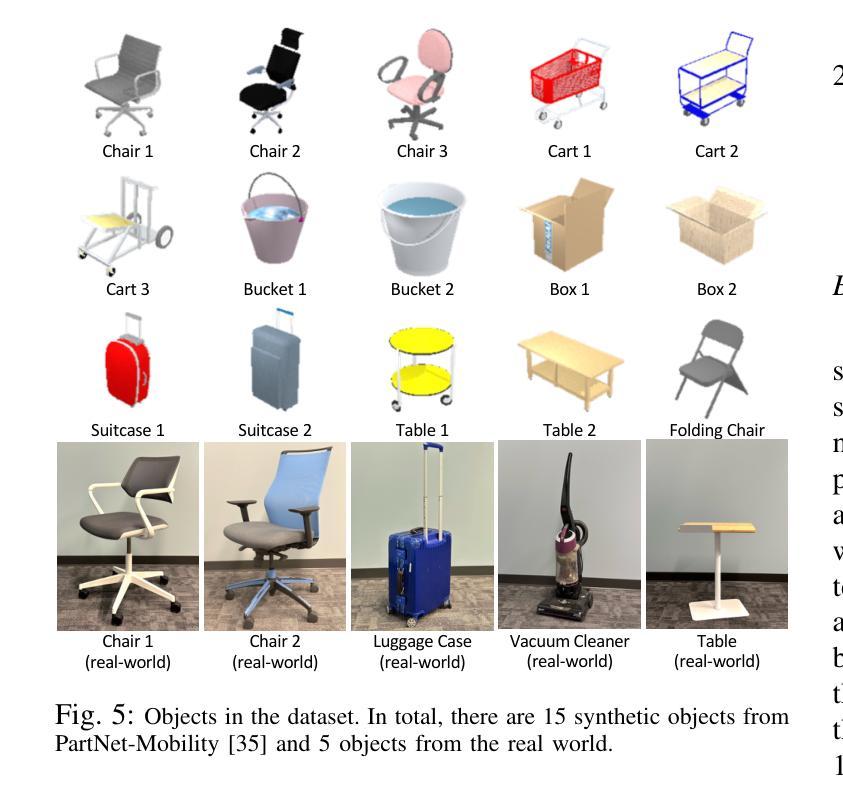
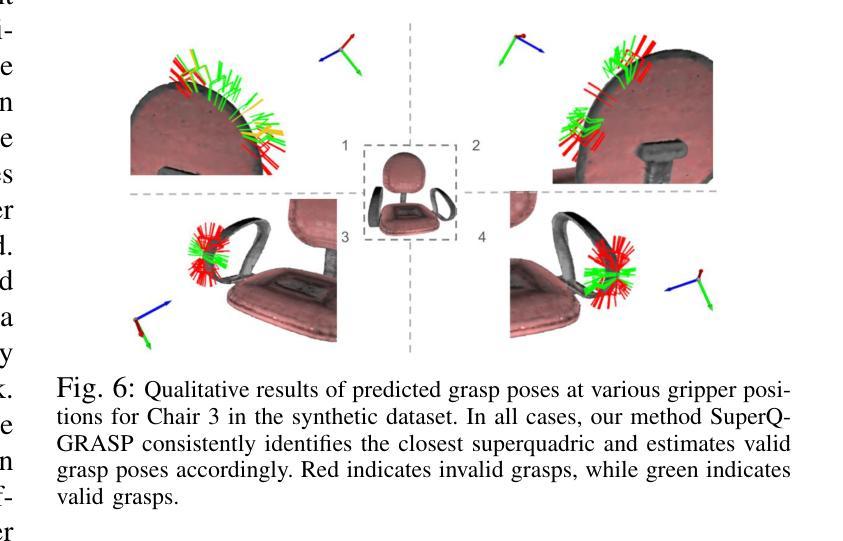
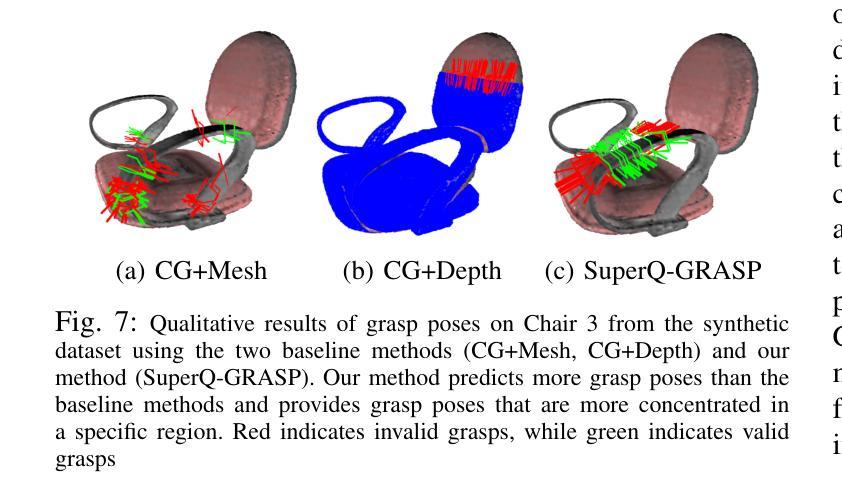
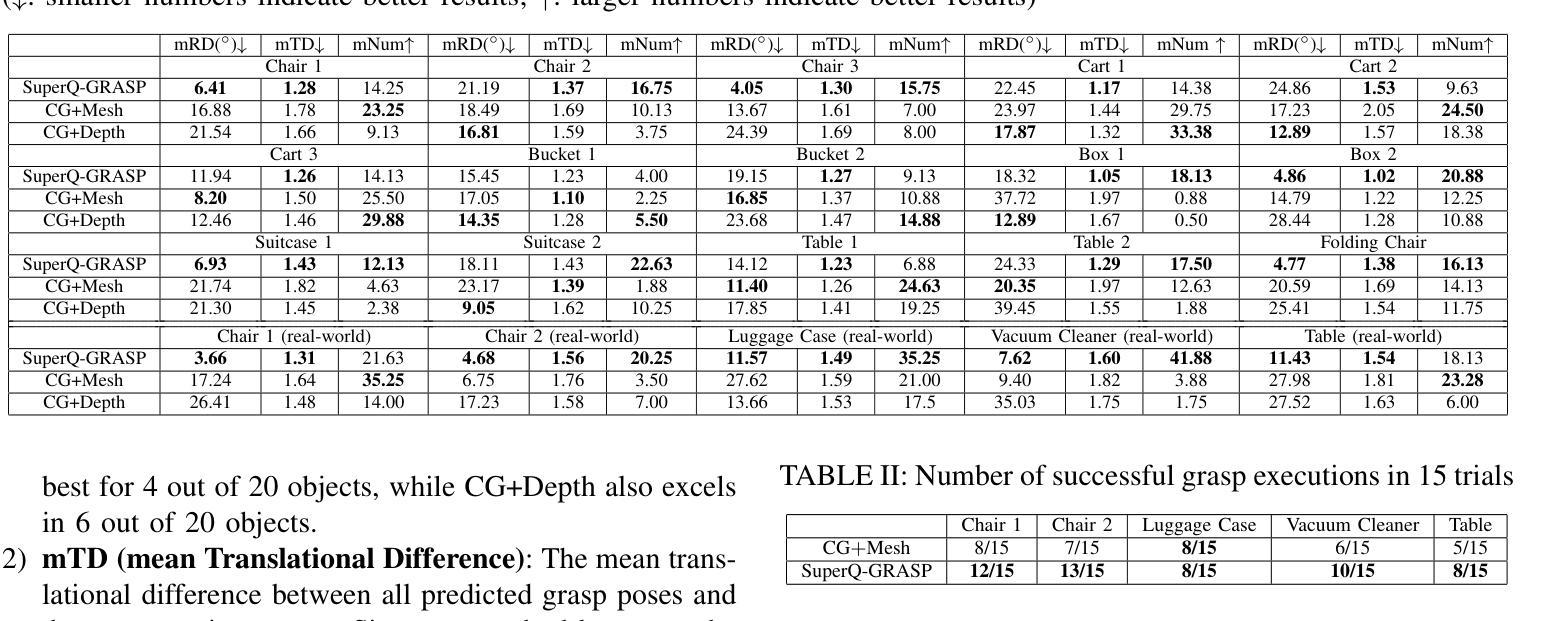