⚠️ 以下所有内容总结都来自于 大语言模型的能力,如有错误,仅供参考,谨慎使用
🔴 请注意:千万不要用于严肃的学术场景,只能用于论文阅读前的初筛!
💗 如果您觉得我们的项目对您有帮助 ChatPaperFree ,还请您给我们一些鼓励!⭐️ HuggingFace免费体验
2025-04-12 更新
VideoSPatS: Video SPatiotemporal Splines for Disentangled Occlusion, Appearance and Motion Modeling and Editing
Authors:Juan Luis Gonzalez Bello, Xu Yao, Alex Whelan, Kyle Olszewski, Hyeongwoo Kim, Pablo Garrido
We present an implicit video representation for occlusions, appearance, and motion disentanglement from monocular videos, which we call Video SPatiotemporal Splines (VideoSPatS). Unlike previous methods that map time and coordinates to deformation and canonical colors, our VideoSPatS maps input coordinates into Spatial and Color Spline deformation fields $D_s$ and $D_c$, which disentangle motion and appearance in videos. With spline-based parametrization, our method naturally generates temporally consistent flow and guarantees long-term temporal consistency, which is crucial for convincing video editing. Using multiple prediction branches, our VideoSPatS model also performs layer separation between the latent video and the selected occluder. By disentangling occlusions, appearance, and motion, our method enables better spatiotemporal modeling and editing of diverse videos, including in-the-wild talking head videos with challenging occlusions, shadows, and specularities while maintaining an appropriate canonical space for editing. We also present general video modeling results on the DAVIS and CoDeF datasets, as well as our own talking head video dataset collected from open-source web videos. Extensive ablations show the combination of $D_s$ and $D_c$ under neural splines can overcome motion and appearance ambiguities, paving the way for more advanced video editing models.
我们提出了一种隐式视频表示方法,用于从单目视频中解决遮挡、外观和运动分离的问题,我们称之为Video SPatiotemporal Splines(VideoSPatS)。不同于以往将时间和坐标映射到变形和典型颜色的方法,我们的VideoSPatS将输入坐标映射到空间和时间样条变形场$D_s$和$D_c$,从而对视频中的运动和外观进行分离。基于样条的参数化方法,我们的方法自然地生成了时间一致的流,保证了长期的时间一致性,这对于令人信服的视频编辑至关重要。使用多个预测分支,我们的VideoSPatS模型还可以在潜在视频和选定遮挡物之间进行层分离。通过分离遮挡、外观和运动,我们的方法能够更好地对多样化的视频进行时空建模和编辑,包括野外带有挑战的遮挡物、阴影和光泽的说话人头视频,同时保持适当的编辑规范空间。我们还展示了在DAVIS和CoDeF数据集上的通用视频建模结果,以及我们自己从开源网络视频收集的说唱头视频数据集。大量的消融研究证明,神经样条下的$D_s$和$D_c$的组合可以克服运动和外观的模糊性,为更先进的视频编辑模型铺平了道路。
论文及项目相关链接
PDF CVPR25, project website: https://juanluisg-flwls.github.io/videospats-website/
Summary:
本文提出了一种基于单目视频的遮挡、外观和运动分离技术的视频时空曲线模型(VideoSPatS)。该模型将输入坐标映射到空间颜色曲线变形场,以解耦视频中的运动和外观。通过曲线参数化,模型可自然生成时间一致的流,保证长期时间一致性,对视频编辑至关重要。该模型采用多个预测分支,实现潜在视频和选定遮挡物的层次分离。通过解耦遮挡、外观和运动,该方法可实现各种视频的时空建模和编辑,包括具有挑战性的遮挡、阴影和光泽的实时谈话视频,同时保持适当的编辑空间。在DAVIS和CoDeF数据集上以及自己的谈话视频数据集上的实验验证了模型的有效性。
Key Takeaways:
- VideoSPatS模型使用空间颜色曲线变形场来解耦视频中的运动和外观。
- 通过曲线参数化,模型能生成时间一致的流并保障长期时间一致性。
- 多个预测分支用于层次分离潜在视频和遮挡物。
- 模型实现了各种视频的时空建模和编辑,包括处理具有挑战性的遮挡、阴影和光泽的实时谈话视频。
- 模型在DAVIS和CoDeF数据集以及自有谈话视频数据集上的实验验证了其有效性。
- VideoSPatS模型提供了一种有效的视频编辑方法,可为视频编辑提供更高级别的模型。
点此查看论文截图
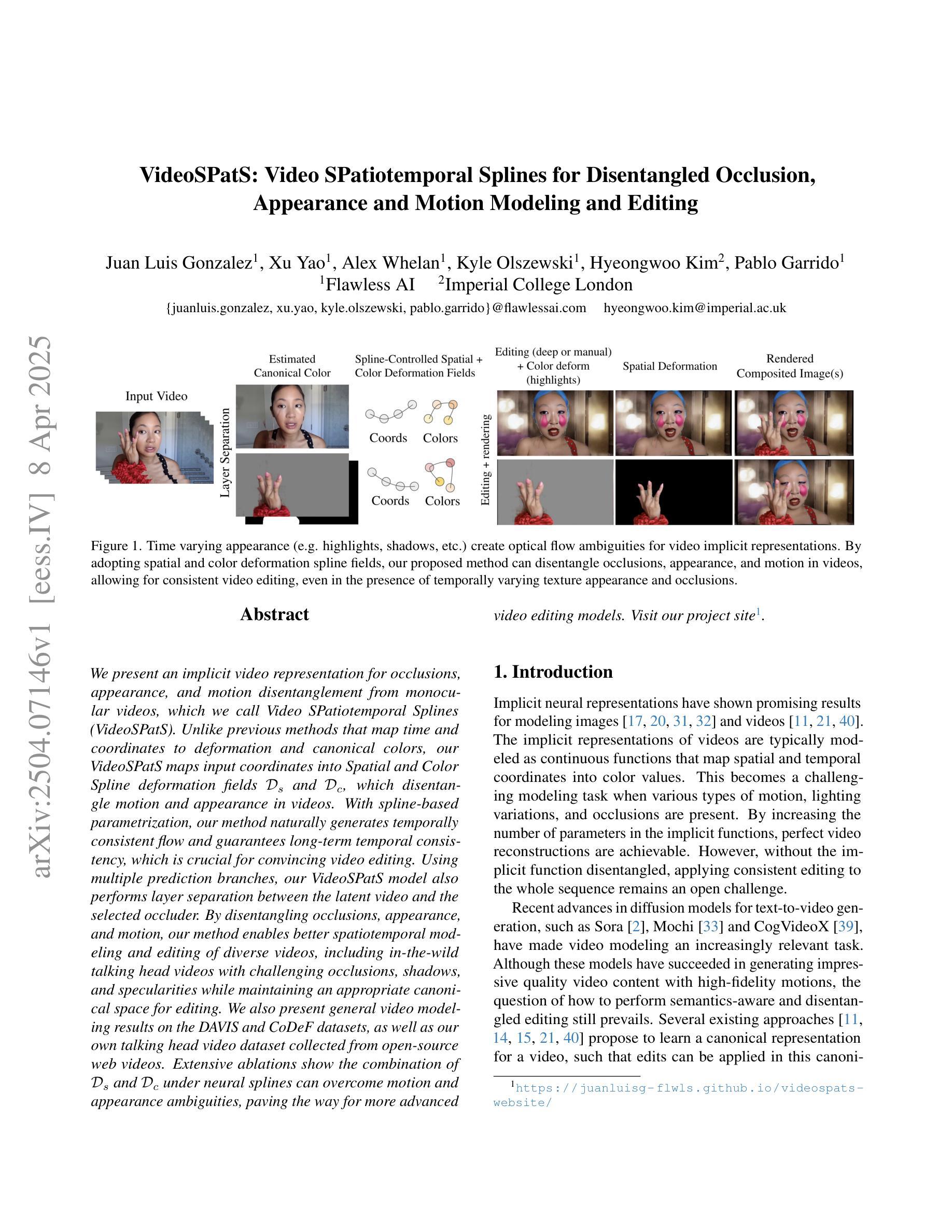
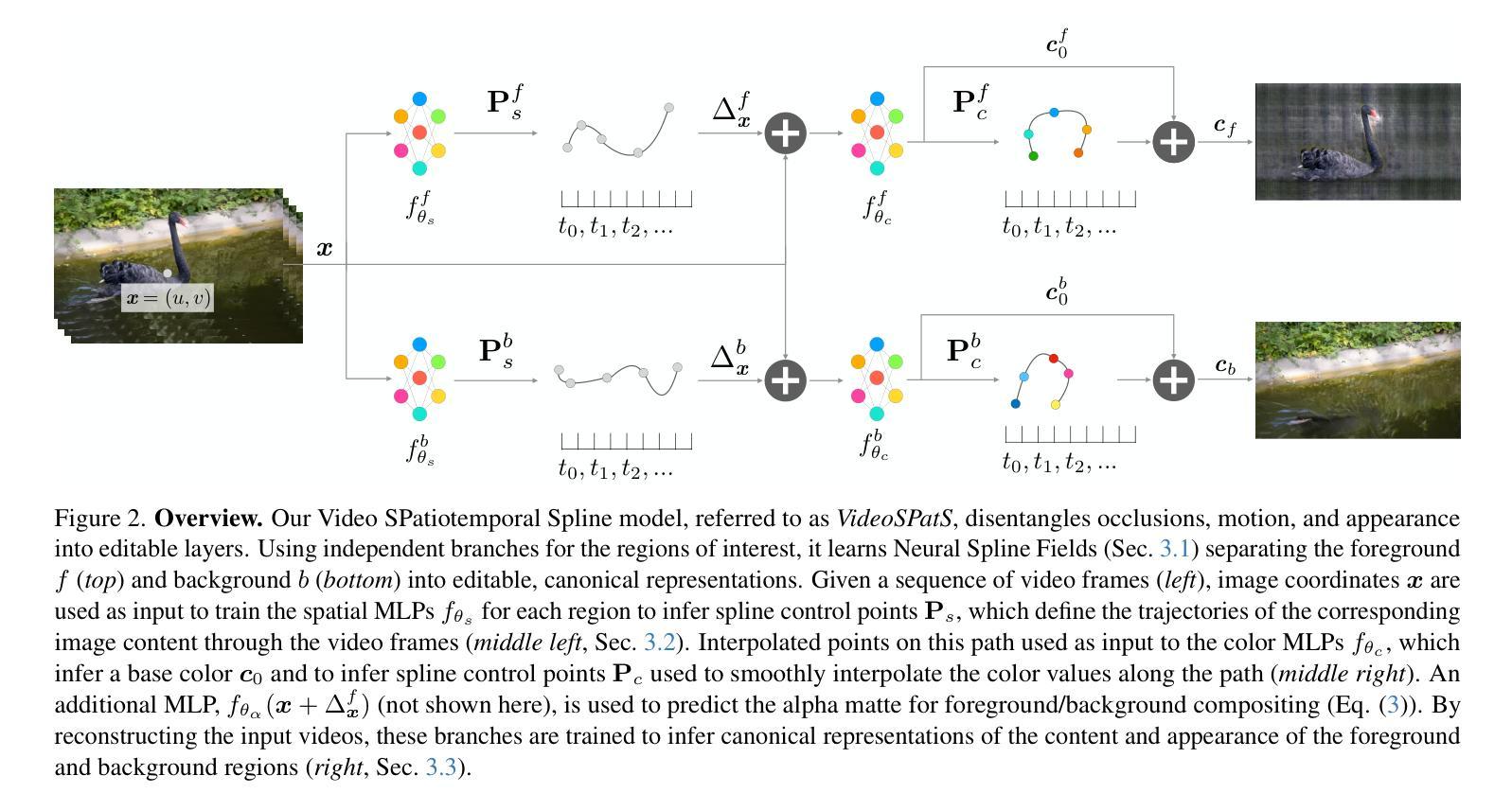
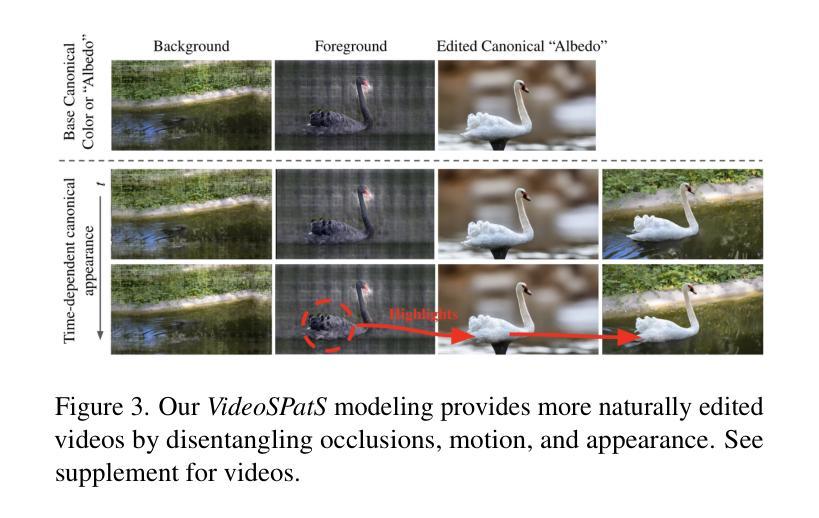
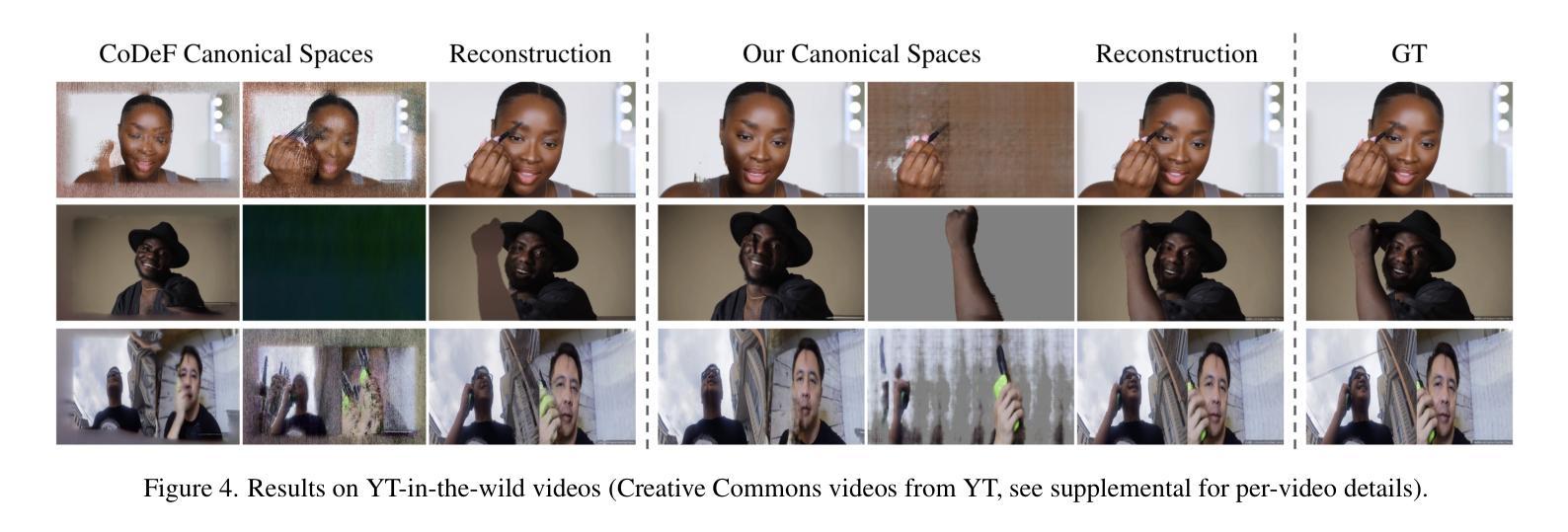
Playmate: Flexible Control of Portrait Animation via 3D-Implicit Space Guided Diffusion
Authors:Xingpei Ma, Jiaran Cai, Yuansheng Guan, Shenneng Huang, Qiang Zhang, Shunsi Zhang
Recent diffusion-based talking face generation models have demonstrated impressive potential in synthesizing videos that accurately match a speech audio clip with a given reference identity. However, existing approaches still encounter significant challenges due to uncontrollable factors, such as inaccurate lip-sync, inappropriate head posture and the lack of fine-grained control over facial expressions. In order to introduce more face-guided conditions beyond speech audio clips, a novel two-stage training framework Playmate is proposed to generate more lifelike facial expressions and talking faces. In the first stage, we introduce a decoupled implicit 3D representation along with a meticulously designed motion-decoupled module to facilitate more accurate attribute disentanglement and generate expressive talking videos directly from audio cues. Then, in the second stage, we introduce an emotion-control module to encode emotion control information into the latent space, enabling fine-grained control over emotions and thereby achieving the ability to generate talking videos with desired emotion. Extensive experiments demonstrate that Playmate outperforms existing state-of-the-art methods in terms of video quality and lip-synchronization, and improves flexibility in controlling emotion and head pose. The code will be available at https://playmate111.github.io.
近期基于扩散的说话人脸生成模型在合成视频方面展示出了令人印象深刻的潜力,能够准确地将给定的参考身份与语音音频片段相匹配。然而,现有方法仍然面临着由于不可控因素带来的重大挑战,例如唇同步不准确、头部姿势不自然以及对面部表情的精细控制不足。为了引入除了语音音频片段之外更多的人脸引导条件,提出了一种新颖的两阶段训练框架Playmate,以生成更逼真的面部表情和说话人脸。在第一阶段,我们引入了解耦隐式3D表示以及精心设计的运动解耦模块,以促更准确的属性分解,并直接从音频线索生成富有表现力的说话视频。然后,在第二阶段,我们引入情绪控制模块,将情绪控制信息编码到潜在空间,实现对情绪的精细控制,从而生成具有所需情绪的说话视频的能力。大量实验表明,Playmate在视频质量和唇同步方面超越了现有的最先进方法,提高了控制情绪和头部姿势的灵活性。代码将在https://playmate111.github.io上提供。
论文及项目相关链接
Summary
近期扩散式谈话面部生成模型在合成视频方面展现出令人印象深刻的潜力,能够根据给定的参考身份和语音音频片段准确匹配。然而,现有方法仍然面临诸多挑战,如不可控制的因素导致的唇同步不准确、头部姿势不当以及对面部表情的精细控制不足。为了引入更多面部指导条件,提出了一种新型的两阶段训练框架Playmate,以生成更逼真的面部表情和谈话面部。第一阶段引入了解耦隐式3D表示和精心设计的运动解耦模块,以促进更精确的属性分解,并直接从音频线索生成表达性谈话视频。第二阶段引入情感控制模块,将情感控制信息编码到潜在空间,实现对情感的精细控制,从而生成具有所需情感的谈话视频。实验表明,Playmate在视频质量和唇同步方面优于现有最先进的方法,并且在控制情绪和头部姿势方面更具灵活性。
Key Takeaways
- 扩散式谈话面部生成模型能够根据参考身份和语音音频片段合成视频。
- 现有方法面临唇同步不准确、头部姿势不当和缺乏面部表情精细控制的挑战。
- Playmate是一个新型的两阶段训练框架,用于生成更逼真的面部表情和谈话面部。
- 第一阶段通过解耦隐式3D表示和运动解耦模块促进属性分解,直接从音频生成表达性视频。
- 第二阶段引入情感控制模块,实现对情感的精细控制,并生成具有所需情感的谈话视频。
- Playmate在视频质量和唇同步方面优于现有方法。
点此查看论文截图
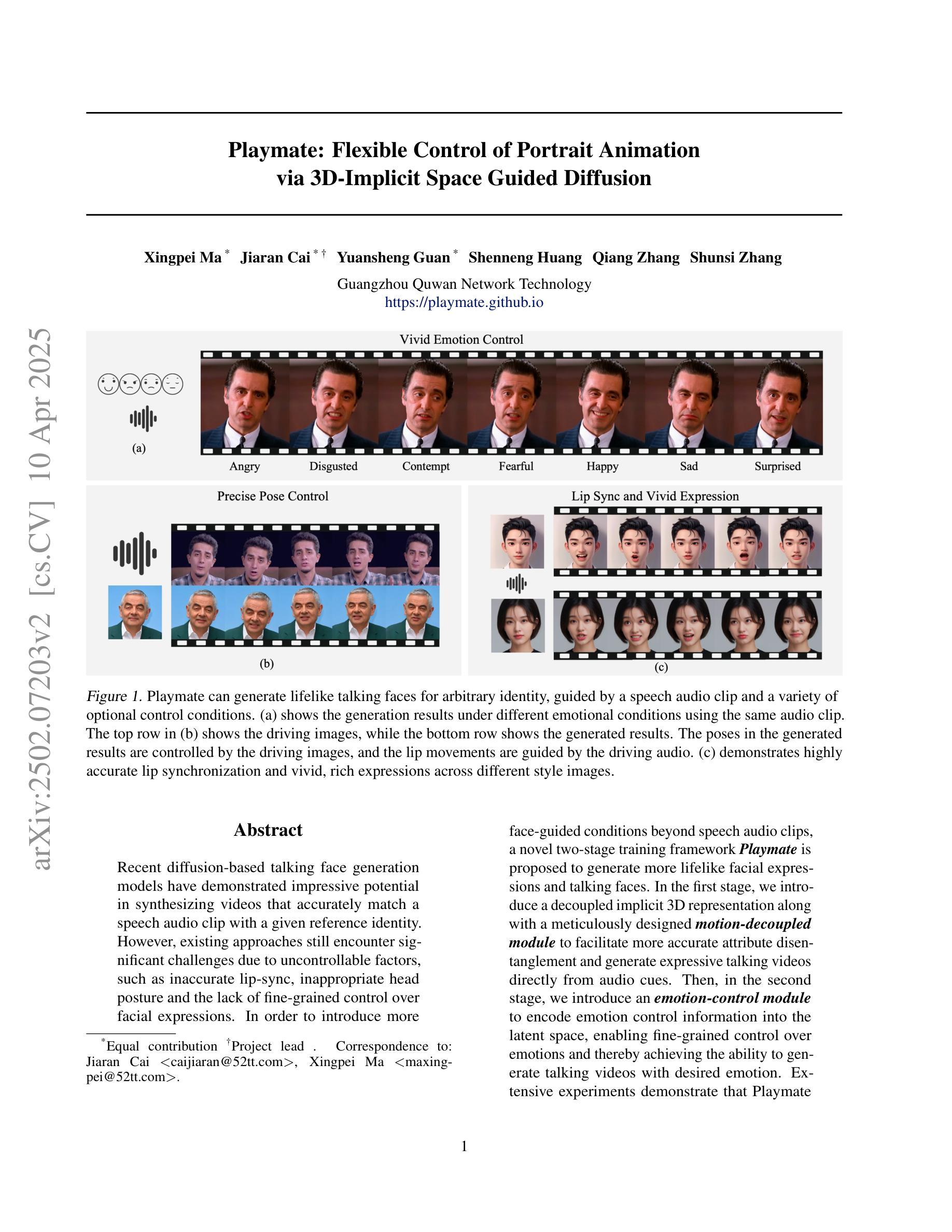
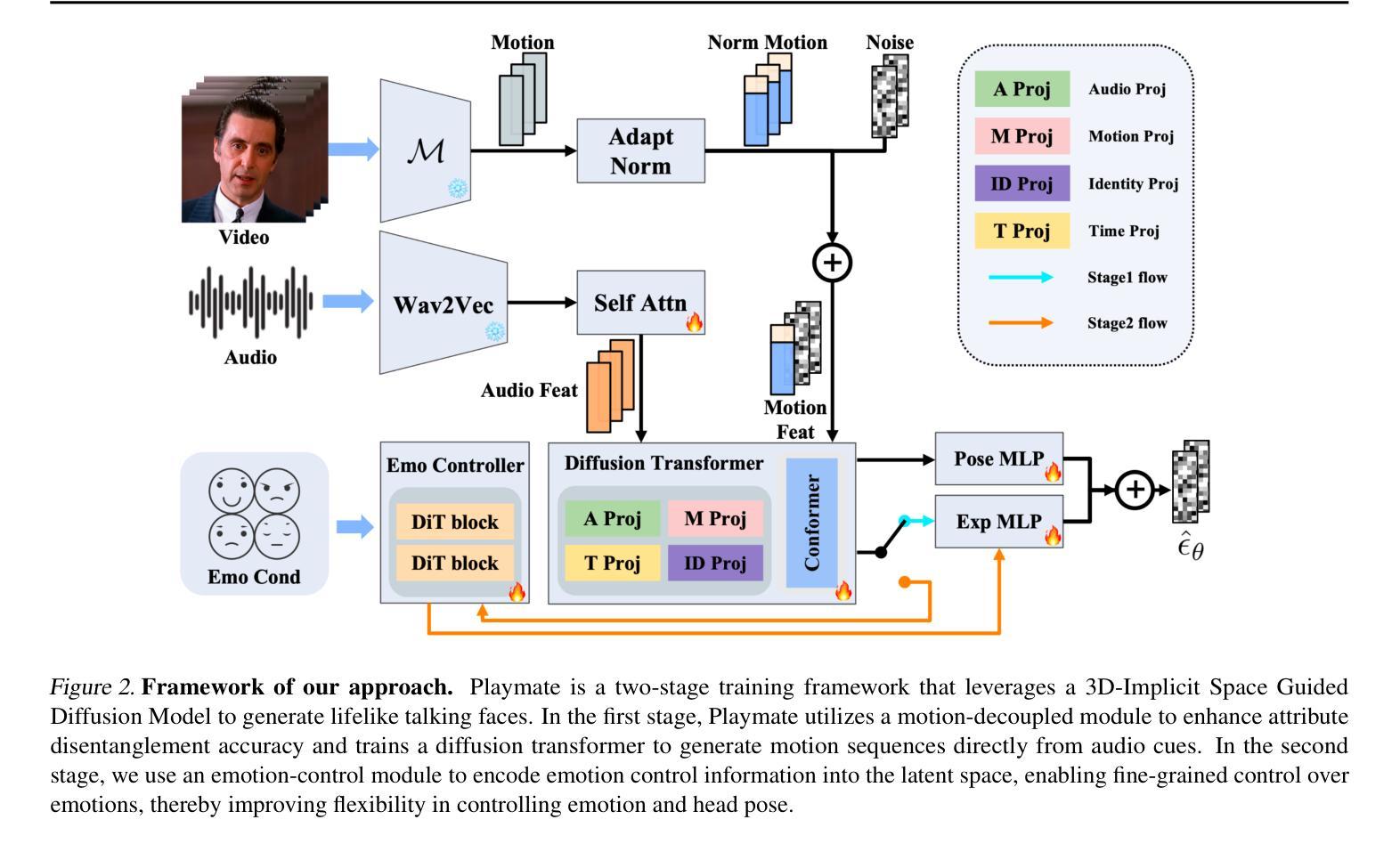
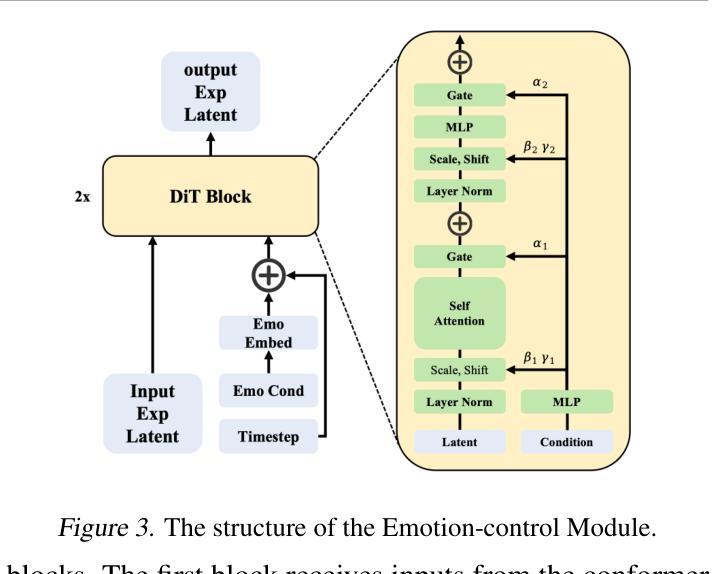
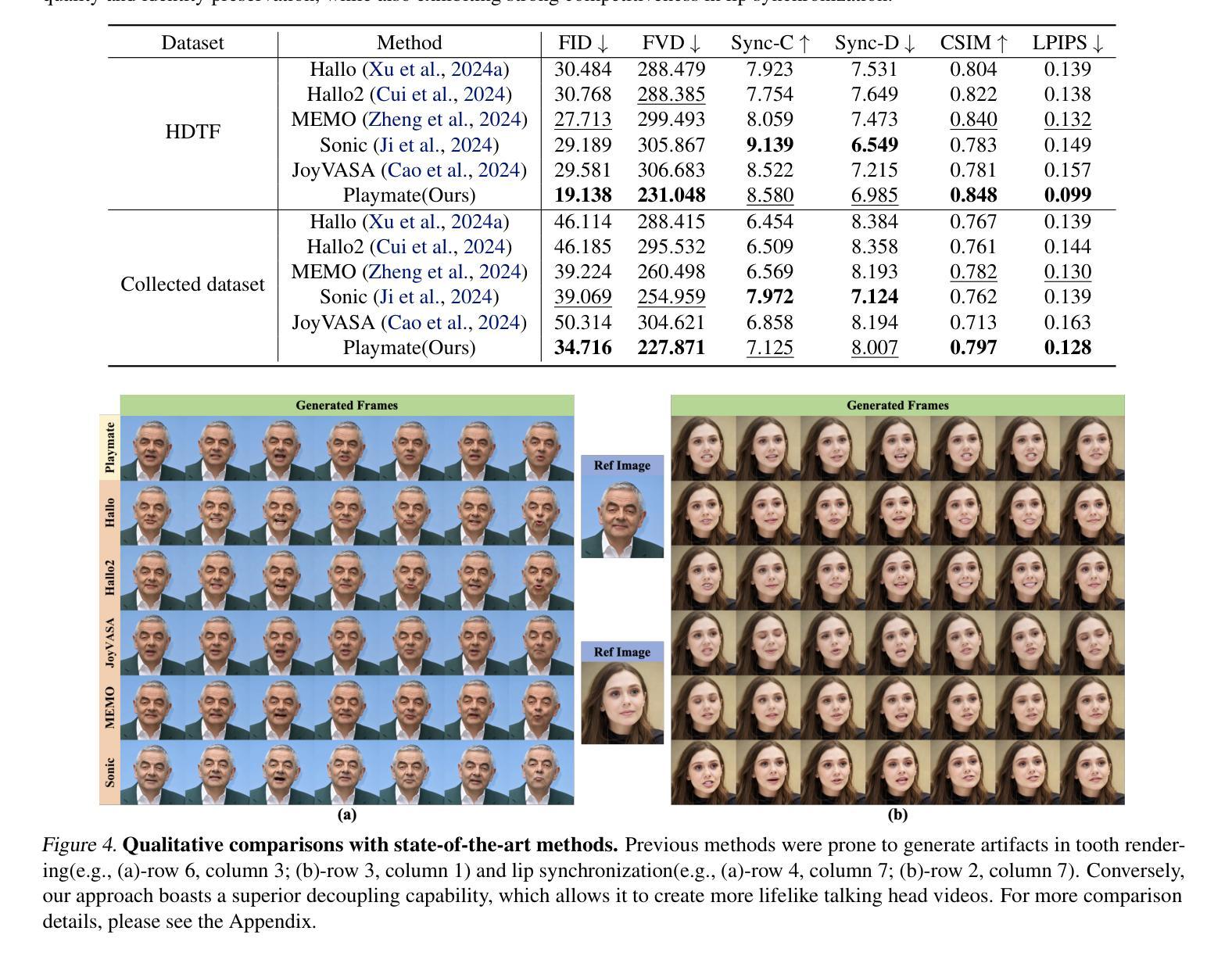