⚠️ 以下所有内容总结都来自于 大语言模型的能力,如有错误,仅供参考,谨慎使用
🔴 请注意:千万不要用于严肃的学术场景,只能用于论文阅读前的初筛!
💗 如果您觉得我们的项目对您有帮助 ChatPaperFree ,还请您给我们一些鼓励!⭐️ HuggingFace免费体验
2025-04-15 更新
FMLGS: Fast Multilevel Language Embedded Gaussians for Part-level Interactive Agents
Authors:Xin Tan, Yuzhou Ji, He Zhu, Yuan Xie
The semantically interactive radiance field has long been a promising backbone for 3D real-world applications, such as embodied AI to achieve scene understanding and manipulation. However, multi-granularity interaction remains a challenging task due to the ambiguity of language and degraded quality when it comes to queries upon object components. In this work, we present FMLGS, an approach that supports part-level open-vocabulary query within 3D Gaussian Splatting (3DGS). We propose an efficient pipeline for building and querying consistent object- and part-level semantics based on Segment Anything Model 2 (SAM2). We designed a semantic deviation strategy to solve the problem of language ambiguity among object parts, which interpolates the semantic features of fine-grained targets for enriched information. Once trained, we can query both objects and their describable parts using natural language. Comparisons with other state-of-the-art methods prove that our method can not only better locate specified part-level targets, but also achieve first-place performance concerning both speed and accuracy, where FMLGS is 98 x faster than LERF, 4 x faster than LangSplat and 2.5 x faster than LEGaussians. Meanwhile, we further integrate FMLGS as a virtual agent that can interactively navigate through 3D scenes, locate targets, and respond to user demands through a chat interface, which demonstrates the potential of our work to be further expanded and applied in the future.
语义交互辐射场长期以来一直是三维现实世界应用(如实现场景理解和操作的嵌入式AI)的支柱。然而,由于语言的不明确性和对物体组件查询时的质量下降,多粒度交互仍然是一项具有挑战性的任务。在这项工作中,我们提出了FMLGS方法,它支持在三维高斯拼贴(3DGS)内进行部分级别的开放词汇查询。我们基于Segment Anything Model 2(SAM2)构建了一个高效的管道,用于构建和查询一致的对象和部分级别的语义。我们设计了一种语义偏差策略来解决物体部分之间语言歧义的问题,通过插值精细目标的语义特征来丰富信息。一旦训练完成,我们可以使用自然语言查询对象及其可描述的部分。与其他最先进的方法的比较证明,我们的方法不仅可以更好地定位指定的部分级别目标,而且在速度和准确性方面都取得了第一名成绩,其中FMLGS比LERF快98倍,比LangSplat快4倍,比LEGaussians快2.5倍。同时,我们将FMLGS进一步集成为一个虚拟代理,可以交互式地浏览三维场景、定位目标并通过聊天界面响应用户需求,这证明了我们工作的潜力在未来得到进一步扩展和应用。
论文及项目相关链接
Summary
本文介绍了语义交互辐射场在三维现实世界应用中的潜力,特别是在物体识别和操作方面的应用。针对多粒度交互中的语言模糊和查询对象组件质量下降的问题,提出了一种基于三维高斯拼贴(3DGS)的FMLGS方法,支持部分级别的开放词汇查询。该方法通过建立和查询一致的对象和部分级别语义,解决了语言模糊的问题,并插入了精细目标的语义特征以丰富信息。与其他最先进的方法相比,FMLGS不仅能更好地定位指定的部分级别目标,而且在速度和准确性方面都取得了第一名。此外,还进一步将FMLGS集成为一个虚拟代理,可以交互地浏览三维场景、定位目标并通过聊天界面响应用户需求,展示了该方法的未来扩展和应用潜力。
Key Takeaways
- FMLGS方法支持在三维高斯拼贴(3DGS)中进行部分级别的开放词汇查询。
- 建立和查询对象及部分级别的语义,解决语言模糊问题。
- 通过插值精细目标的语义特征来丰富信息。
- FMLGS在定位部分级别目标方面表现出色,且速度和准确性均居首位。
- FMLGS与其他先进方法相比,具有更高的效率,例如比LERF快98倍,比LangSplat快4倍,比LEGaussians快2.5倍。
- FMLGS被集成为一个虚拟代理,可以交互地浏览三维场景、定位目标。
点此查看论文截图
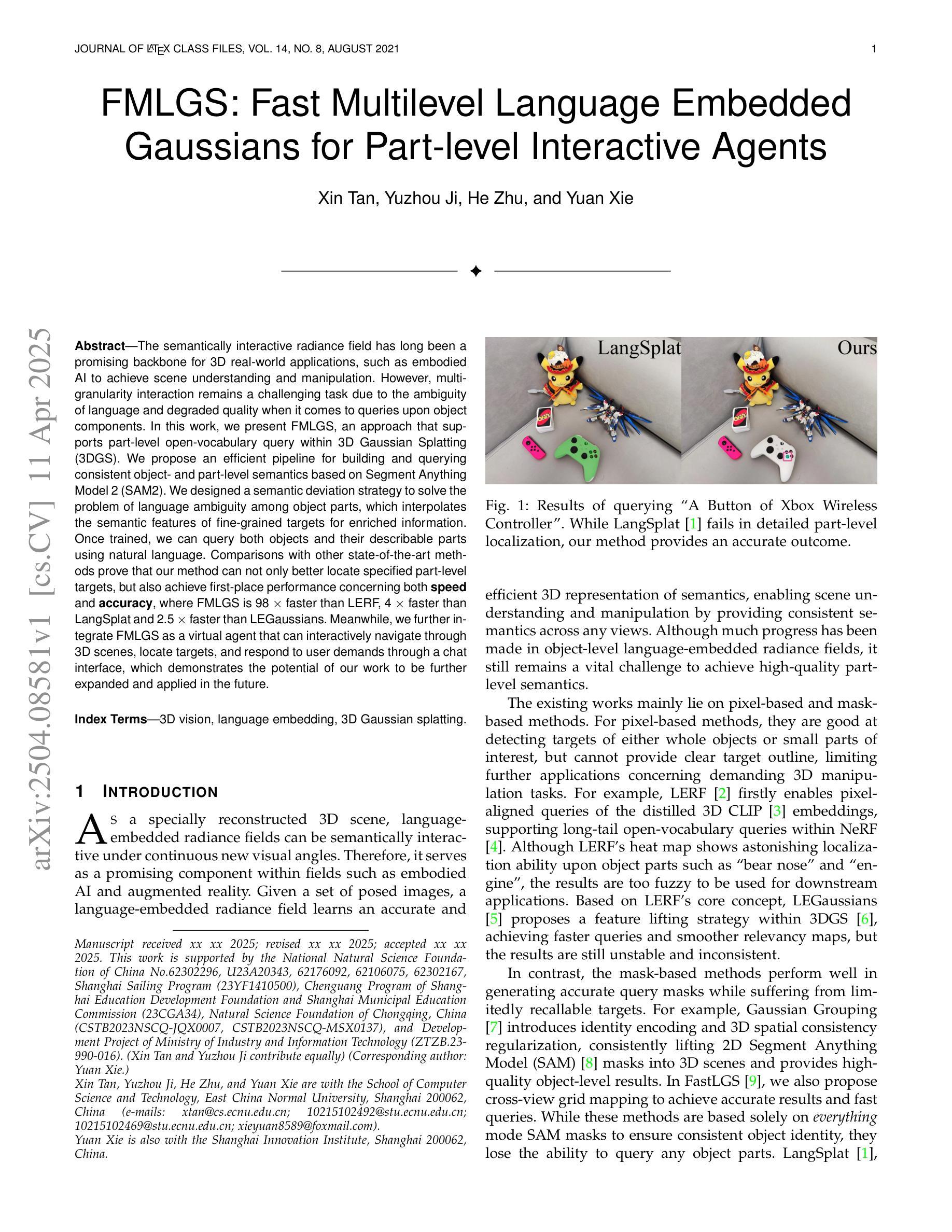
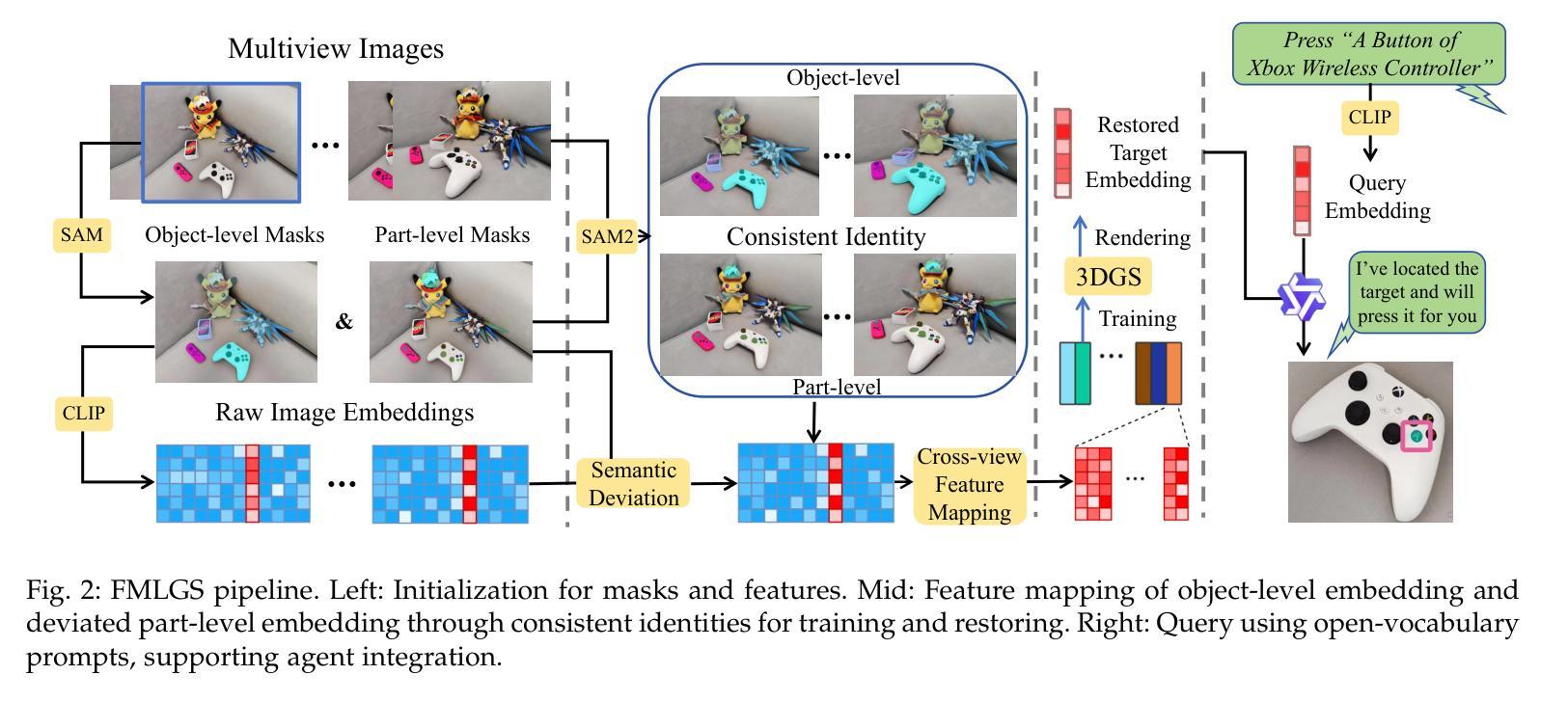
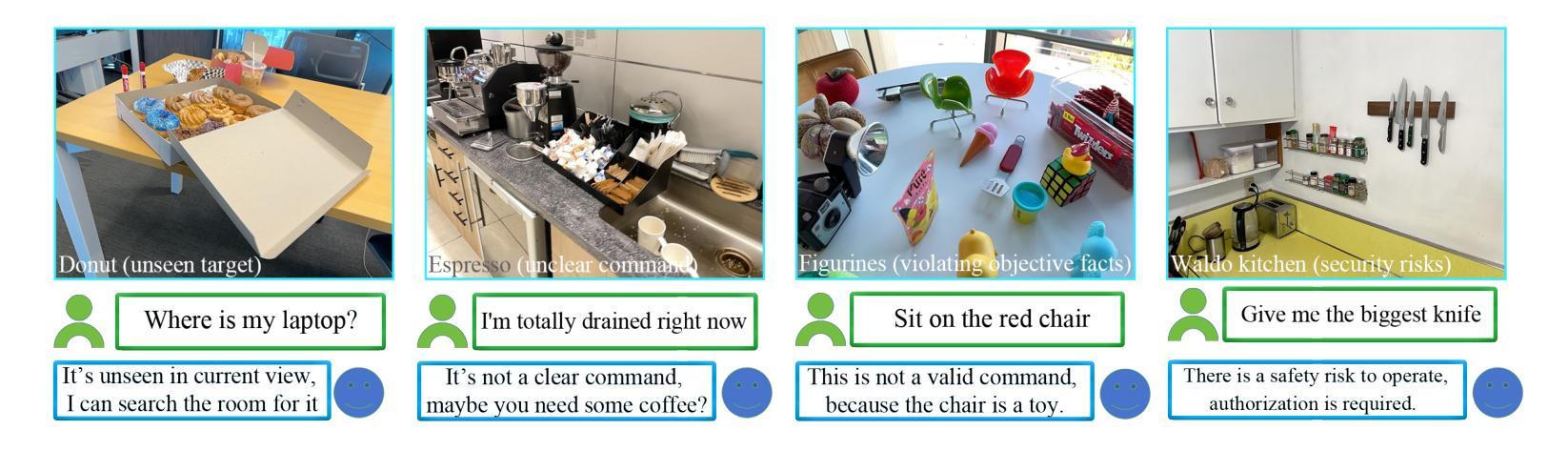
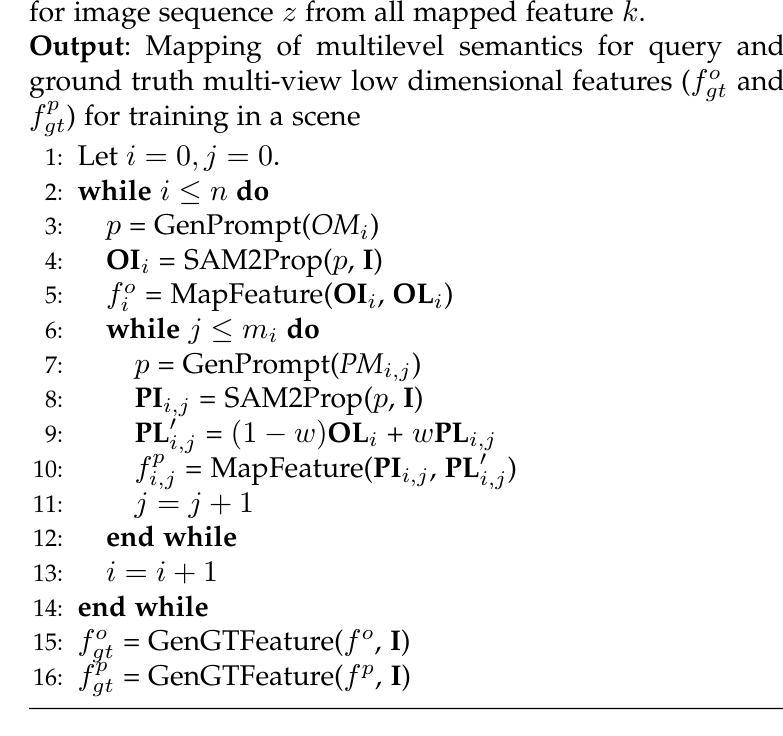
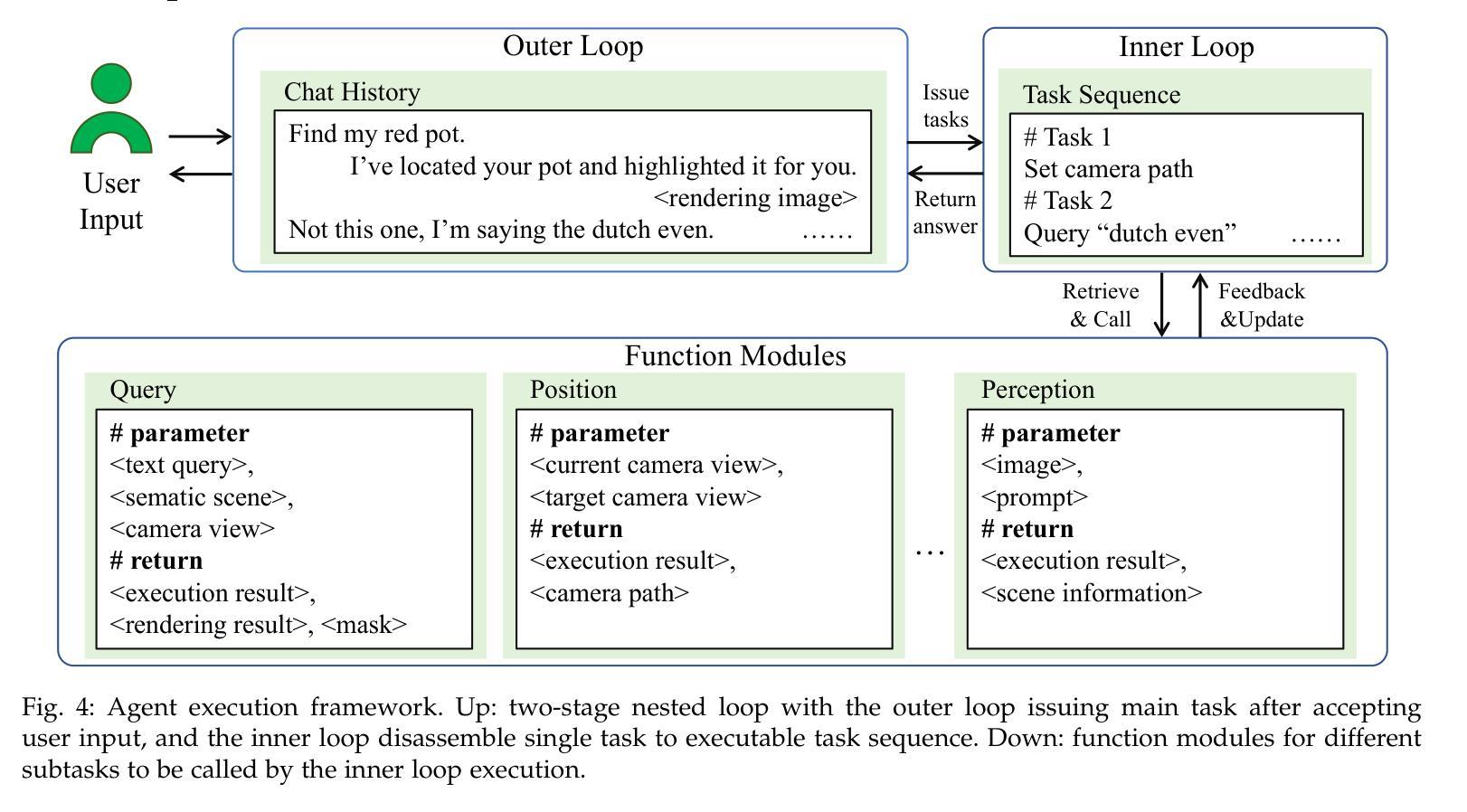
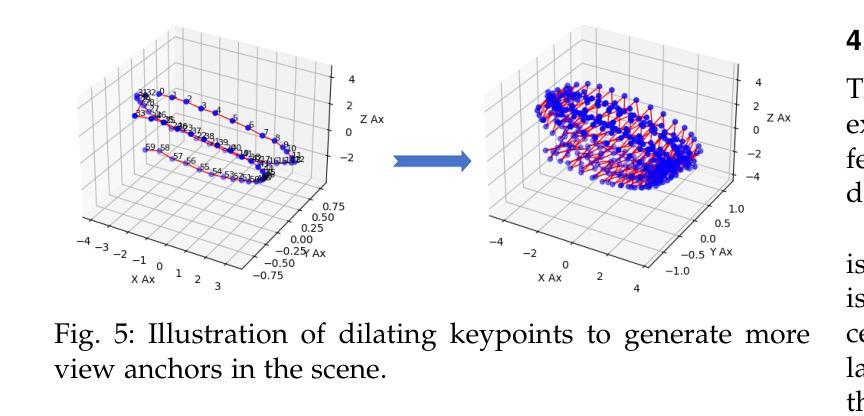
In-2-4D: Inbetweening from Two Single-View Images to 4D Generation
Authors:Sauradip Nag, Daniel Cohen-Or, Hao Zhang, Ali Mahdavi-Amiri
We propose a new problem, In-2-4D, for generative 4D (i.e., 3D + motion) inbetweening from a minimalistic input setting: two single-view images capturing an object in two distinct motion states. Given two images representing the start and end states of an object in motion, our goal is to generate and reconstruct the motion in 4D. We utilize a video interpolation model to predict the motion, but large frame-to-frame motions can lead to ambiguous interpretations. To overcome this, we employ a hierarchical approach to identify keyframes that are visually close to the input states and show significant motion, then generate smooth fragments between them. For each fragment, we construct the 3D representation of the keyframe using Gaussian Splatting. The temporal frames within the fragment guide the motion, enabling their transformation into dynamic Gaussians through a deformation field. To improve temporal consistency and refine 3D motion, we expand the self-attention of multi-view diffusion across timesteps and apply rigid transformation regularization. Finally, we merge the independently generated 3D motion segments by interpolating boundary deformation fields and optimizing them to align with the guiding video, ensuring smooth and flicker-free transitions. Through extensive qualitative and quantitiave experiments as well as a user study, we show the effectiveness of our method and its components. The project page is available at https://in-2-4d.github.io/
我们针对生成式四维(即三维加动态)中间插帧提出一个新问题,即In-2-4D,从极简输入设置开始:两张捕捉物体处于两种不同运动状态的单视图图像。给定两张代表物体运动开始和结束状态的照片,我们的目标是在四维中生成并重建运动。我们利用视频插值模型来预测运动,但较大的帧间运动可能导致模糊的解释。为了克服这一问题,我们采用分层方法来识别视觉上接近输入状态的关键帧,并显示有显著的运动,然后在它们之间生成平滑片段。对于每个片段,我们使用高斯涂抹技术构建关键帧的三维表示。片段内的临时帧引导运动,并通过变形场将其转变为动态高斯。为了提高时间一致性和优化三维运动,我们扩大了跨时间步骤的多视角扩散的自注意力,并应用了刚性变换正则化。最后,我们通过插值边界变形场并对其进行优化来合并独立生成的三维运动片段,以确保平滑且无闪烁的过渡与指导视频对齐。通过广泛的定性和定量实验以及用户研究,我们展示了我们的方法和其组件的有效性。项目页面可在[https://in-2-4d.github.io/]查看。
论文及项目相关链接
PDF Technical Report
Summary
针对生成式4D(即3D+运动)插值的新问题In-2-4D,提出从极简输入设置中进行研究:两张捕捉物体两种不同运动状态的单视图图像。给定表示物体运动起始和结束状态的两张图像,我们的目标是生成并重建4D中的运动。利用视频插值模型预测运动,但针对大幅帧间运动导致的模糊解读问题,采用层次方法识别视觉上接近输入状态且运动明显的关键帧,然后生成它们之间的平滑片段。为每个片段构建3D表示,利用时间帧指导运动,通过变形场将其转化为动态高斯。为提高时间一致性和优化3D运动,扩大跨时间步的多视图扩散自注意力,应用刚性变换正则化。最后,通过插值边界变形场并优化与指导视频对齐,合并独立生成的3D运动片段,确保平滑无闪烁过渡。本研究通过定性、定量实验和用户研究验证了方法和组件的有效性。项目页面可访问:[https://in-2-4d.github.io/] 。
Key Takeaways
- 提出了一个新的研究问题In-2-4D,专注于从两个单视图图像生成物体的4D运动。
- 利用视频插值模型预测运动,并采用层次方法处理大幅帧间运动带来的模糊解读问题。
- 通过识别关键帧并构建其3D表示,利用变形场转化为动态高斯,以指导运动。
- 为提高时间一致性和优化3D运动,扩大了自注意力并应用了刚性变换正则化。
- 通过插值和优化边界变形场,实现了独立生成的3D运动片段的合并,确保了平滑过渡。
- 通过定性、定量实验和用户研究验证了方法和组件的有效性。
点此查看论文截图
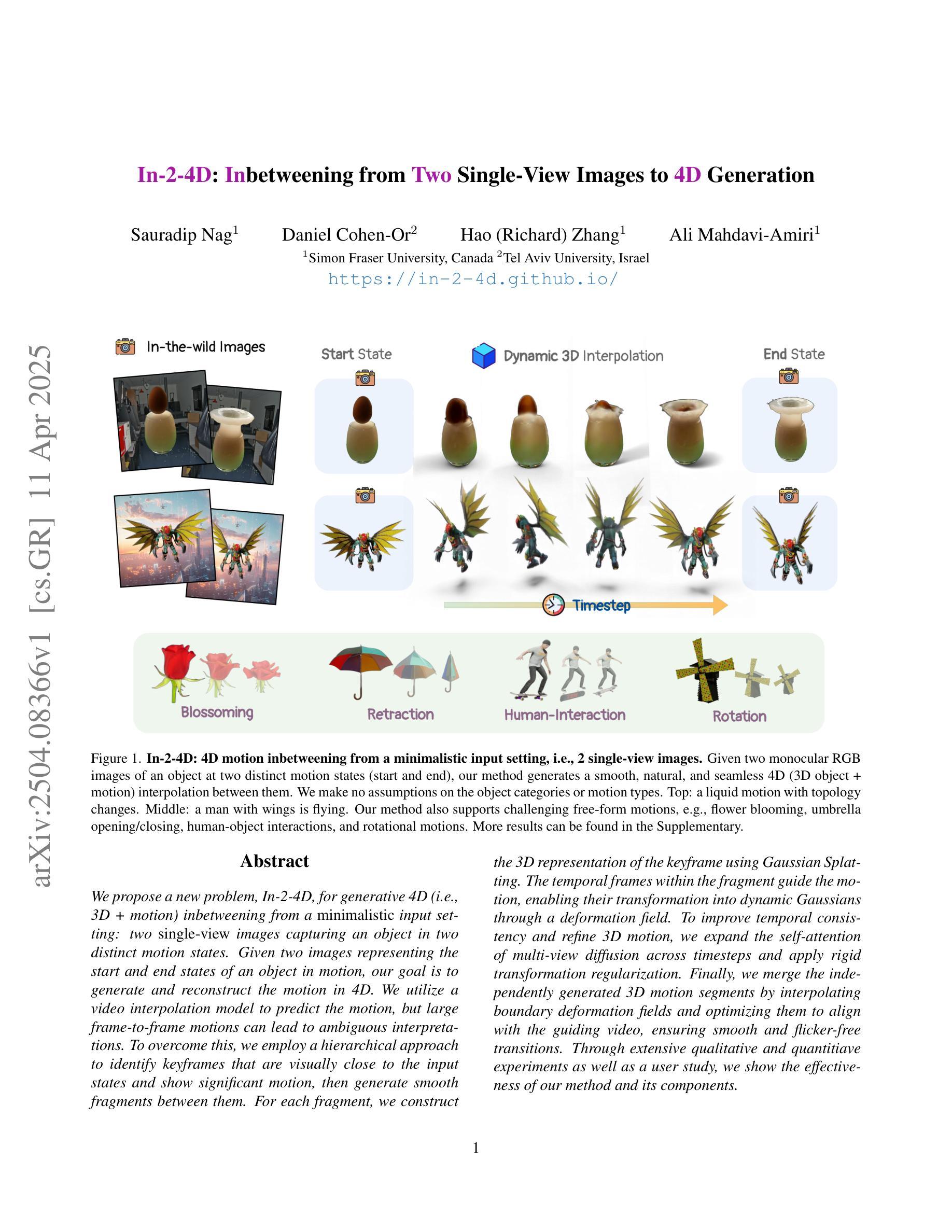
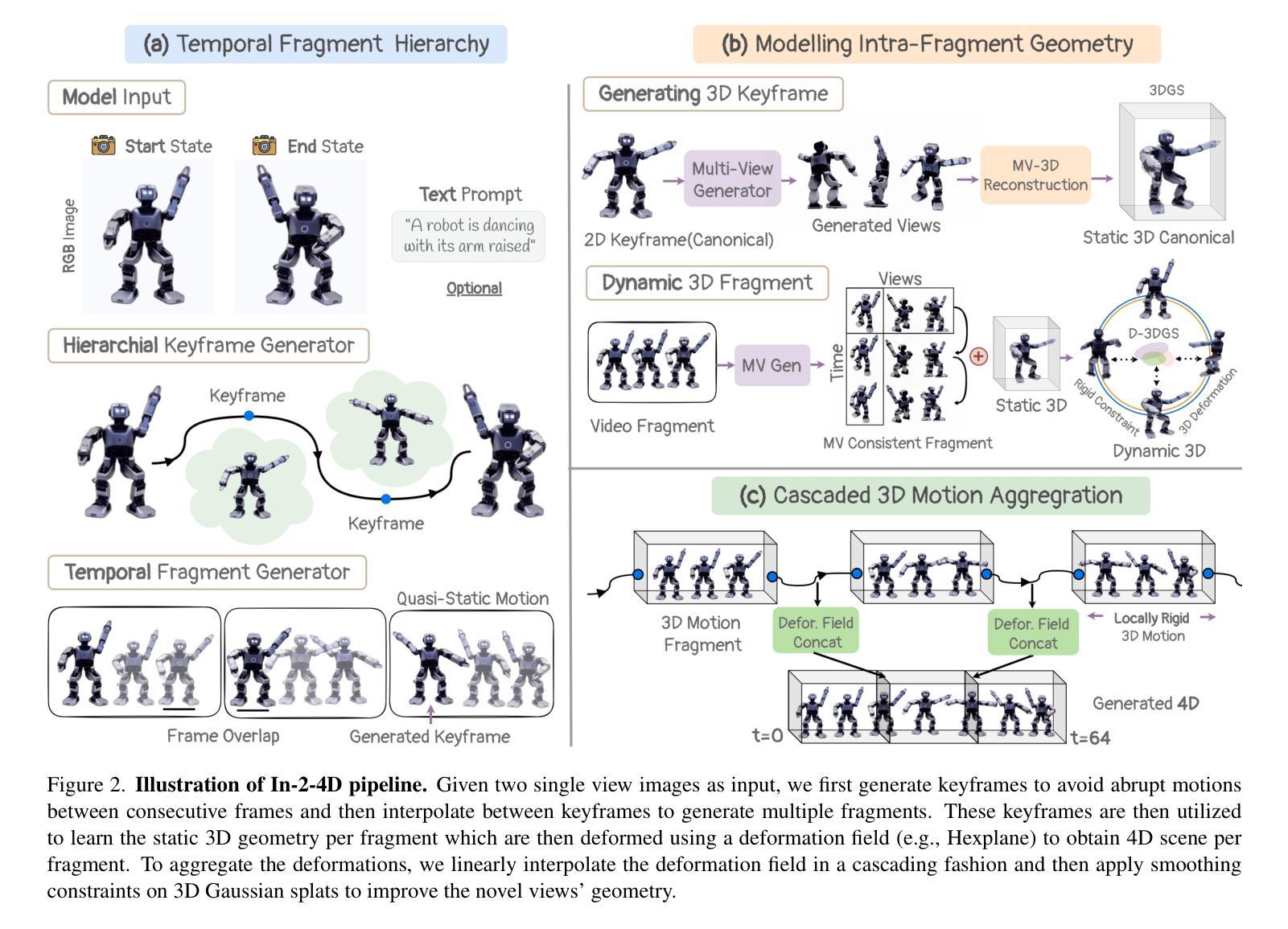
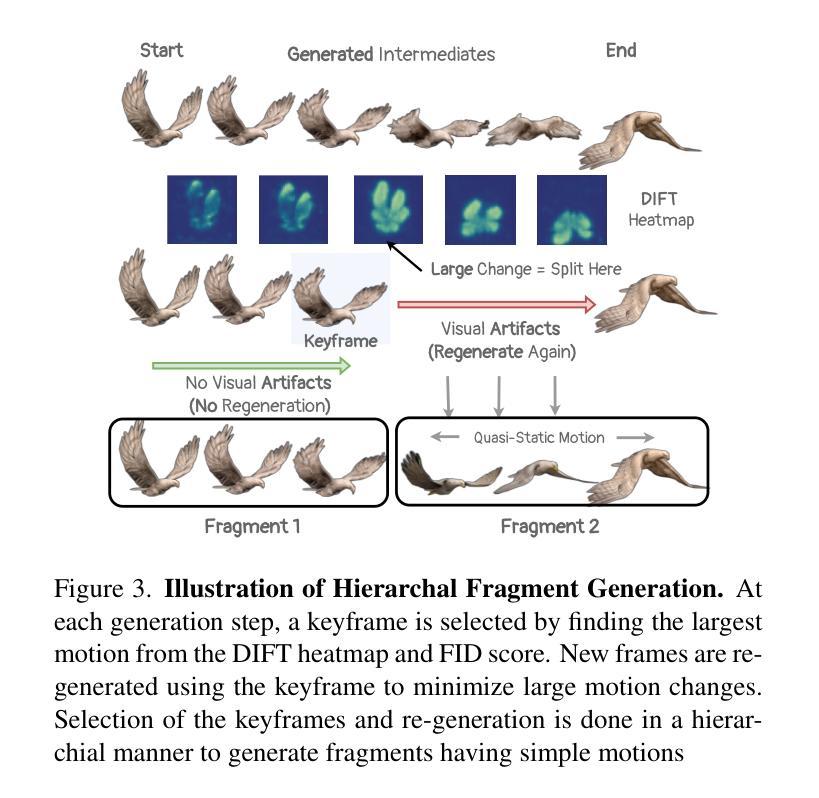
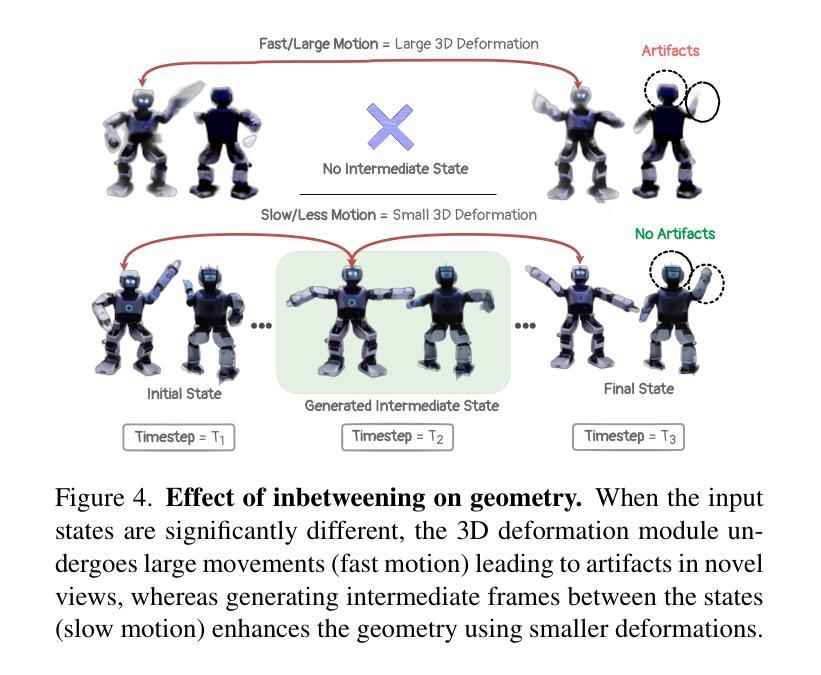
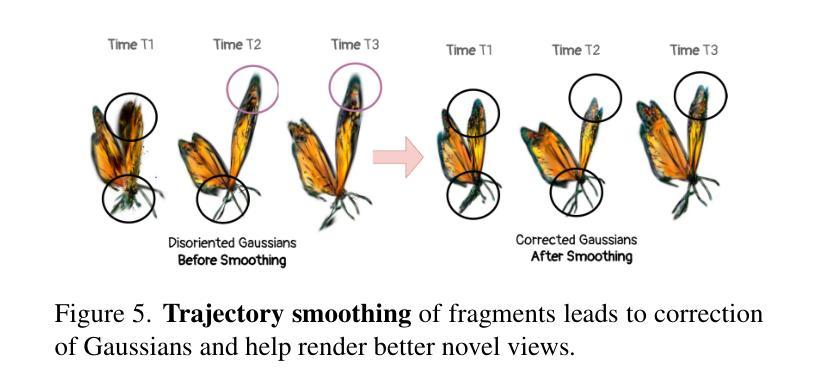
3D Student Splatting and Scooping
Authors:Jialin Zhu, Jiangbei Yue, Feixiang He, He Wang
Recently, 3D Gaussian Splatting (3DGS) provides a new framework for novel view synthesis, and has spiked a new wave of research in neural rendering and related applications. As 3DGS is becoming a foundational component of many models, any improvement on 3DGS itself can bring huge benefits. To this end, we aim to improve the fundamental paradigm and formulation of 3DGS. We argue that as an unnormalized mixture model, it needs to be neither Gaussians nor splatting. We subsequently propose a new mixture model consisting of flexible Student’s t distributions, with both positive (splatting) and negative (scooping) densities. We name our model Student Splatting and Scooping, or SSS. When providing better expressivity, SSS also poses new challenges in learning. Therefore, we also propose a new principled sampling approach for optimization. Through exhaustive evaluation and comparison, across multiple datasets, settings, and metrics, we demonstrate that SSS outperforms existing methods in terms of quality and parameter efficiency, e.g. achieving matching or better quality with similar numbers of components, and obtaining comparable results while reducing the component number by as much as 82%.
最近,3D高斯平铺(3DGS)为新型视角合成提供了新的框架,并掀起了神经网络渲染和相关应用研究领域的新一波热潮。由于3DGS正成为许多模型的基础组件,因此对3DGS本身的任何改进都能带来巨大的好处。为此,我们致力于改进3DGS的基本范式和公式。我们认为,作为一种未归一化的混合模型,它既不需要是高斯分布也不需要是平铺。随后,我们提出了一种新的混合模型,由灵活的Student’s t分布组成,包括正向(平铺)和负向(挖勺)密度。我们将我们的模型命名为Student Splatting and Scooping,或SSS。在提供更好表达性的同时,SSS也给学习带来了新的挑战。因此,我们还提出了一种新的原则采样方法进行优化。通过多个数据集、设置和指标的全面评估与比较,我们证明了SSS在质量和参数效率方面优于现有方法,例如在实现相似数量的组件时达到相匹配或更好的质量,并在减少组件数量高达82%的情况下获得相当的结果。
论文及项目相关链接
Summary
近期,3D高斯混合模型(3DGS)为新型视角合成提供了新的框架,并在神经渲染和相关应用中引发了新的研究热潮。为提高其基础范式和公式化表示,研究团队提出改进方案,认为现有的3DGS作为非归一化混合模型,既不需要遵循高斯分布也不需要采用拼贴方式。于是,他们提出了一种新的混合模型——SSS模型,该模型由灵活的Student’s t分布组成,包括正向(拼贴)和负向(勺挖)密度。SSS模型在提高表达性的同时,也给学习带来了新的挑战。通过多个数据集、设置和指标的全面评估与比较,研究表明SSS在质量和参数效率方面优于现有方法,如以相似数量的组件实现相匹配或更好的质量,并减少组件数量达82%时仍能获得相当的结果。
Key Takeaways
- 3DGS为新型视角合成提供了新框架,引发神经渲染领域研究热潮。
- 研究团队旨在改进其基础范式和公式化表示方法,指出非归一化混合模型的优势和不足。
- 提出的新的混合模型——SSS模型由灵活的Student’s t分布组成,兼具正向拼贴和负向勺挖功能。
- SSS模型提高表达性的同时带来了学习方面的挑战。
点此查看论文截图
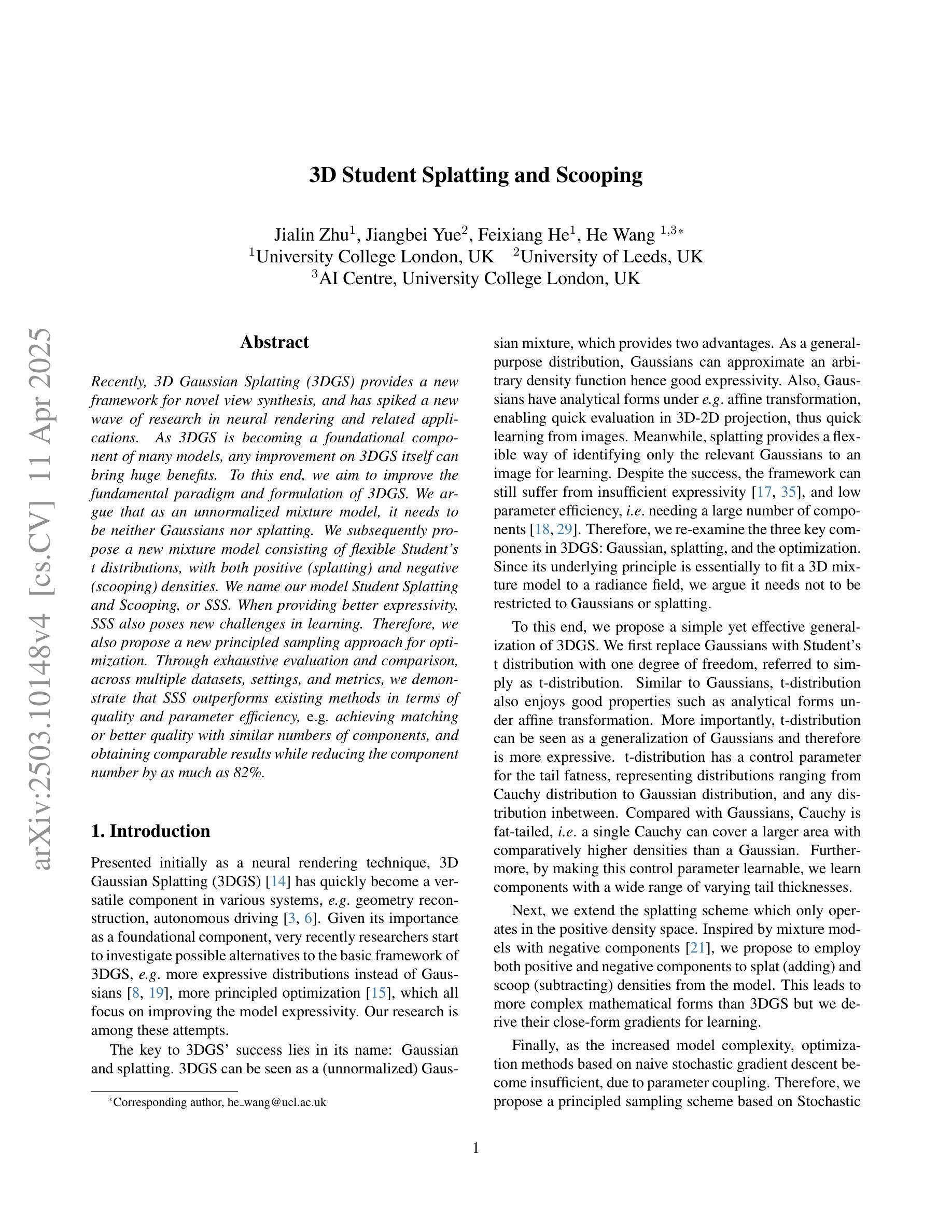
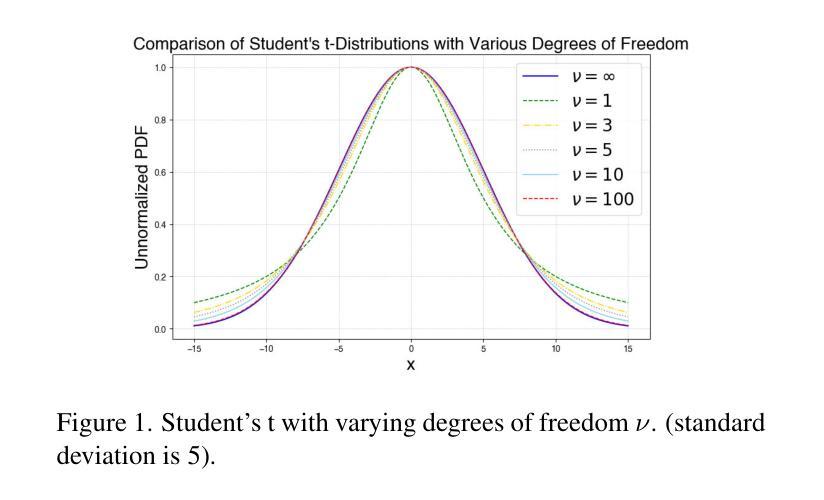
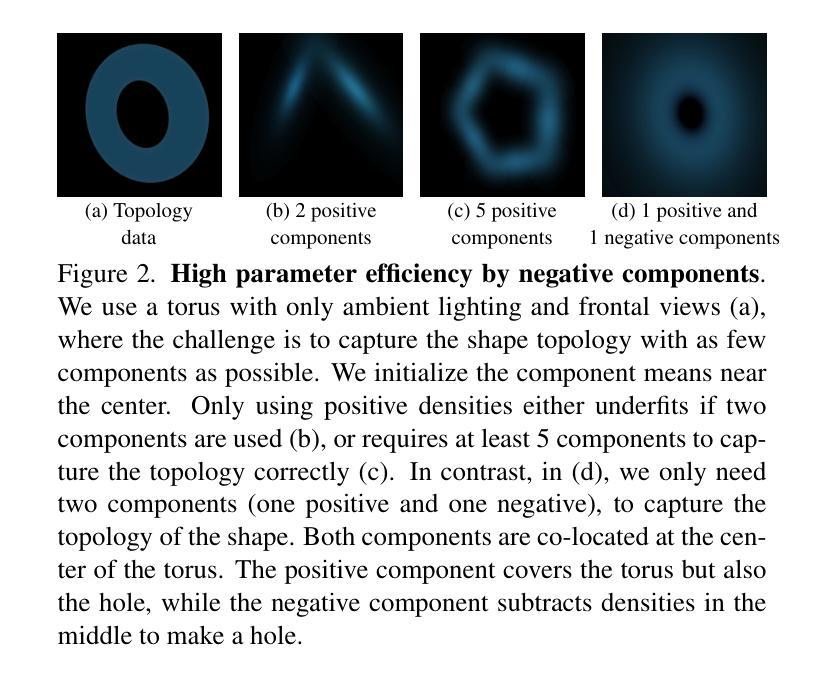
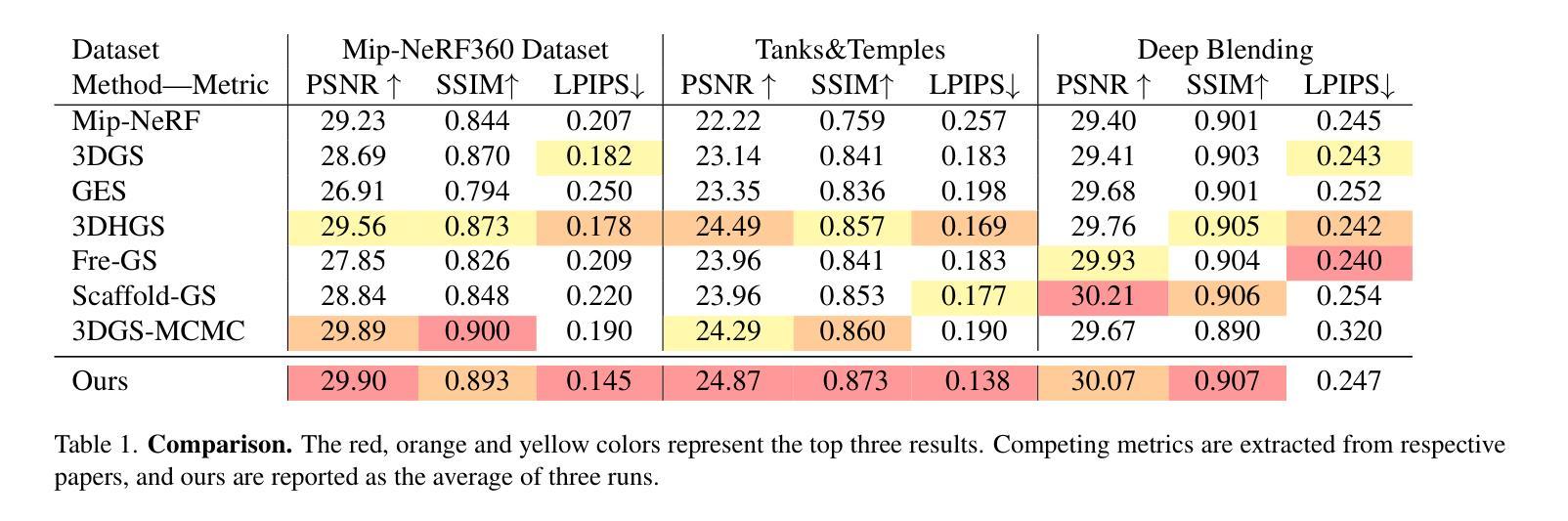
GaussianSpa: An “Optimizing-Sparsifying” Simplification Framework for Compact and High-Quality 3D Gaussian Splatting
Authors:Yangming Zhang, Wenqi Jia, Wei Niu, Miao Yin
3D Gaussian Splatting (3DGS) has emerged as a mainstream for novel view synthesis, leveraging continuous aggregations of Gaussian functions to model scene geometry. However, 3DGS suffers from substantial memory requirements to store the multitude of Gaussians, hindering its practicality. To address this challenge, we introduce GaussianSpa, an optimization-based simplification framework for compact and high-quality 3DGS. Specifically, we formulate the simplification as an optimization problem associated with the 3DGS training. Correspondingly, we propose an efficient “optimizing-sparsifying” solution that alternately solves two independent sub-problems, gradually imposing strong sparsity onto the Gaussians in the training process. Our comprehensive evaluations on various datasets show the superiority of GaussianSpa over existing state-of-the-art approaches. Notably, GaussianSpa achieves an average PSNR improvement of 0.9 dB on the real-world Deep Blending dataset with 10$\times$ fewer Gaussians compared to the vanilla 3DGS. Our project page is available at https://noodle-lab.github.io/gaussianspa/.
3D高斯融合(3DGS)已成为新型视图合成的主流方法,它通过连续聚集的高斯函数来模拟场景几何。然而,为了存储大量高斯数据,3DGS需要巨大的内存,阻碍了其实际应用。为了解决这一挑战,我们引入了GaussianSpa,这是一个基于优化的简化框架,用于实现紧凑且高质量的3DGS。具体来说,我们将简化表述为与3DGS训练相关的优化问题。相应地,我们提出了一种高效的“优化-精简”解决方案,该方案交替解决两个独立的子问题,在训练过程中逐渐对高斯施加强烈的稀疏性。我们在各种数据集上的综合评估表明,GaussianSpa优于现有的最新方法。值得注意的是,在现实世界深度混合数据集上,与原始3DGS相比,使用更少的高斯(减少10倍),GaussianSpa实现了平均PSNR值提高0.9 dB。我们的项目页面位于https://noodle-lab.github.io/gaussianspa/。
论文及项目相关链接
PDF CVPR 2025. Project page at https://noodle-lab.github.io/gaussianspa/
Summary
这篇文本介绍了针对主流的三维高斯合成技术(3DGS)所存在的内存需求问题,提出了一种新的优化简化框架GaussianSpa。该技术使用优化算法来减少高斯数量的同时保持高质量的三维合成效果。在多个数据集上的评估显示,GaussianSpa相较于现有技术具有显著优势,特别是在真实世界的Deep Blending数据集上,使用更少的高斯数量实现了平均PSNR提升0.9 dB。
Key Takeaways
- 3DGS技术已成为主流的新视角合成方法,利用连续的高斯函数集合建模场景几何。
- 3DGS存在内存需求大的问题,存储大量高斯。
- 提出GaussianSpa框架,通过优化算法简化3DGS,实现紧凑且高质量的三维合成。
- GaussianSpa将简化问题建模为与3DGS训练相关的优化问题。
- GaussianSpa采用“优化-精简”策略,交替解决两个独立子问题,逐步对训练过程中的高斯施加强稀疏性。
- 在多个数据集上的评估显示GaussianSpa优于现有技术。
点此查看论文截图
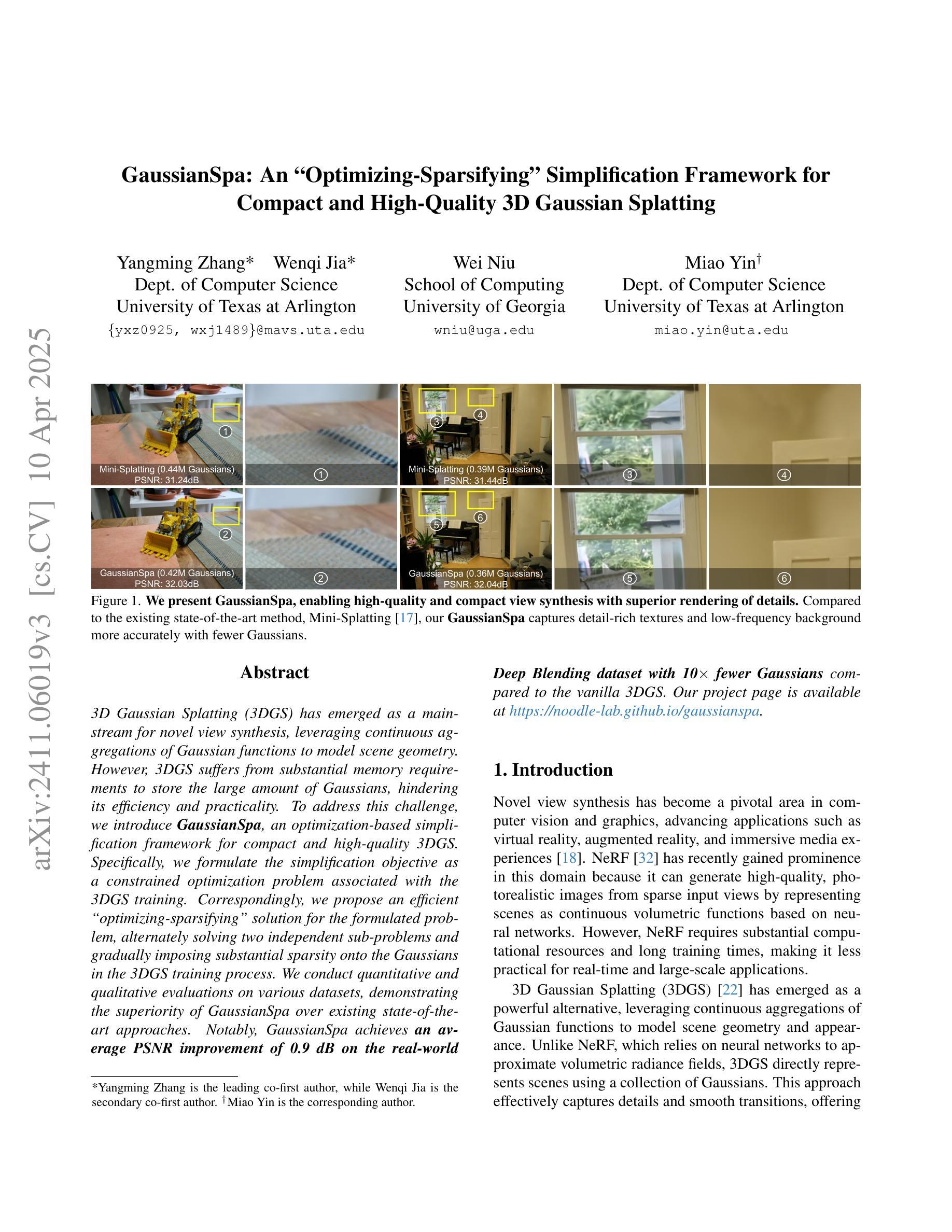
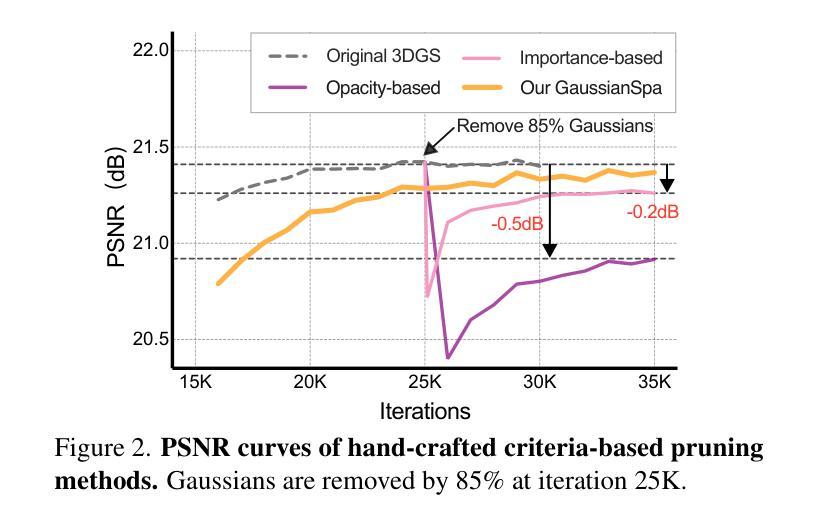
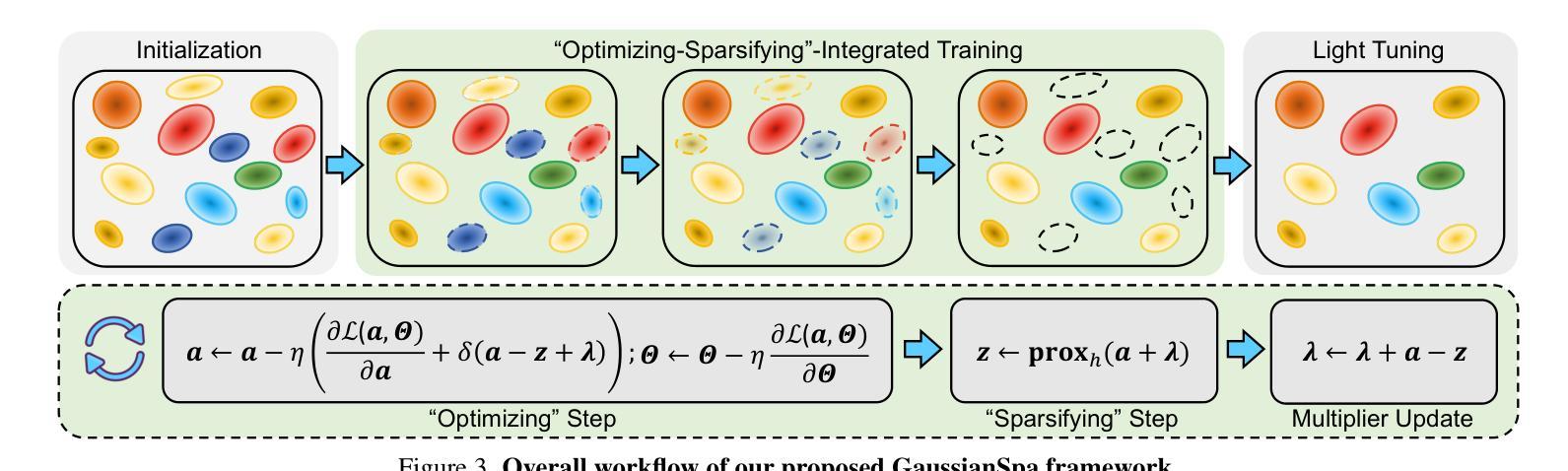
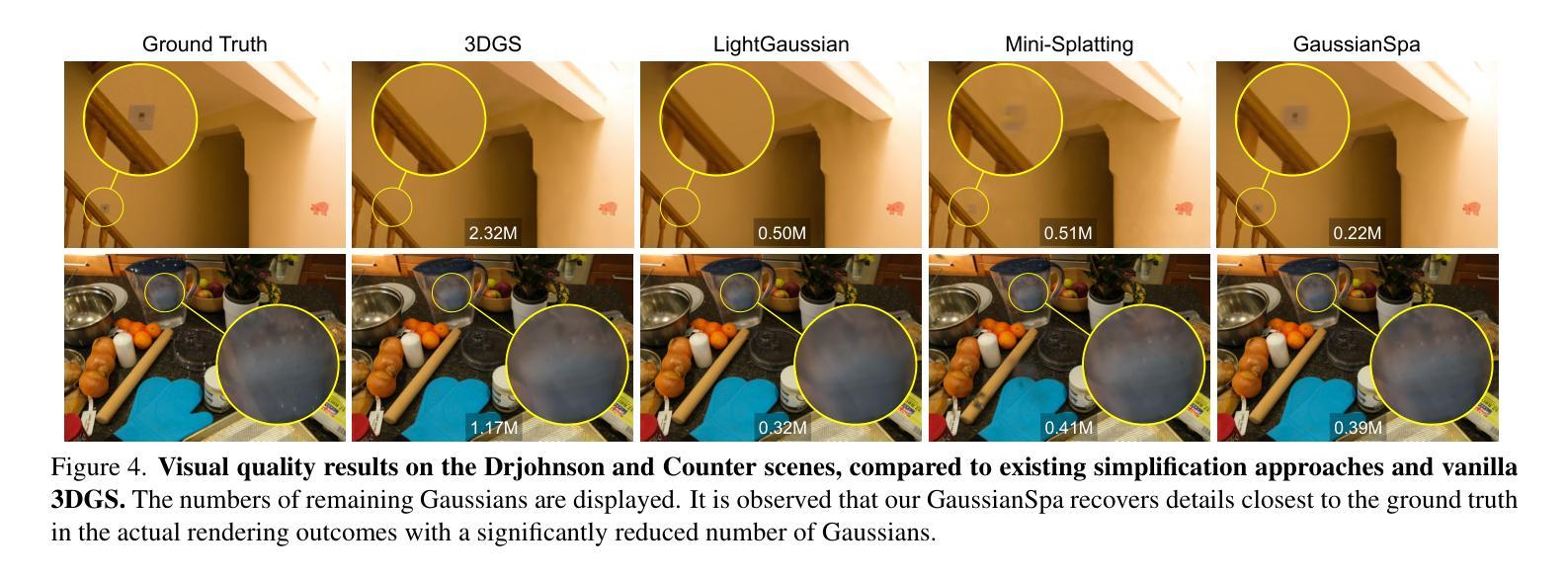
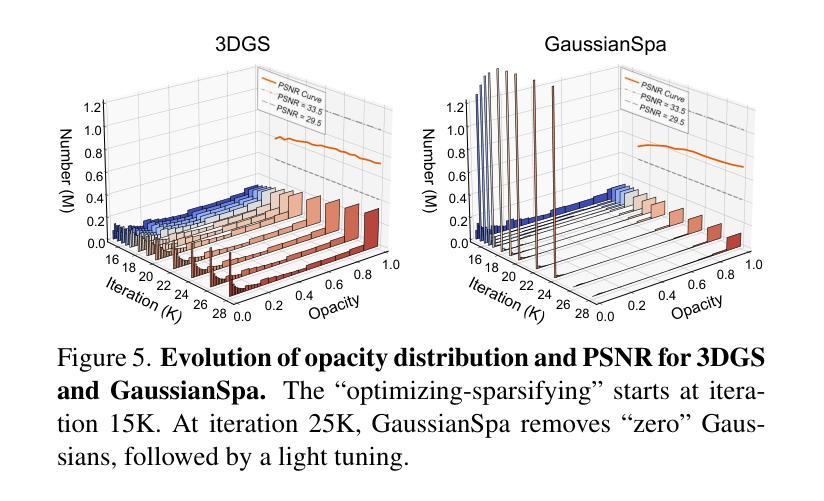
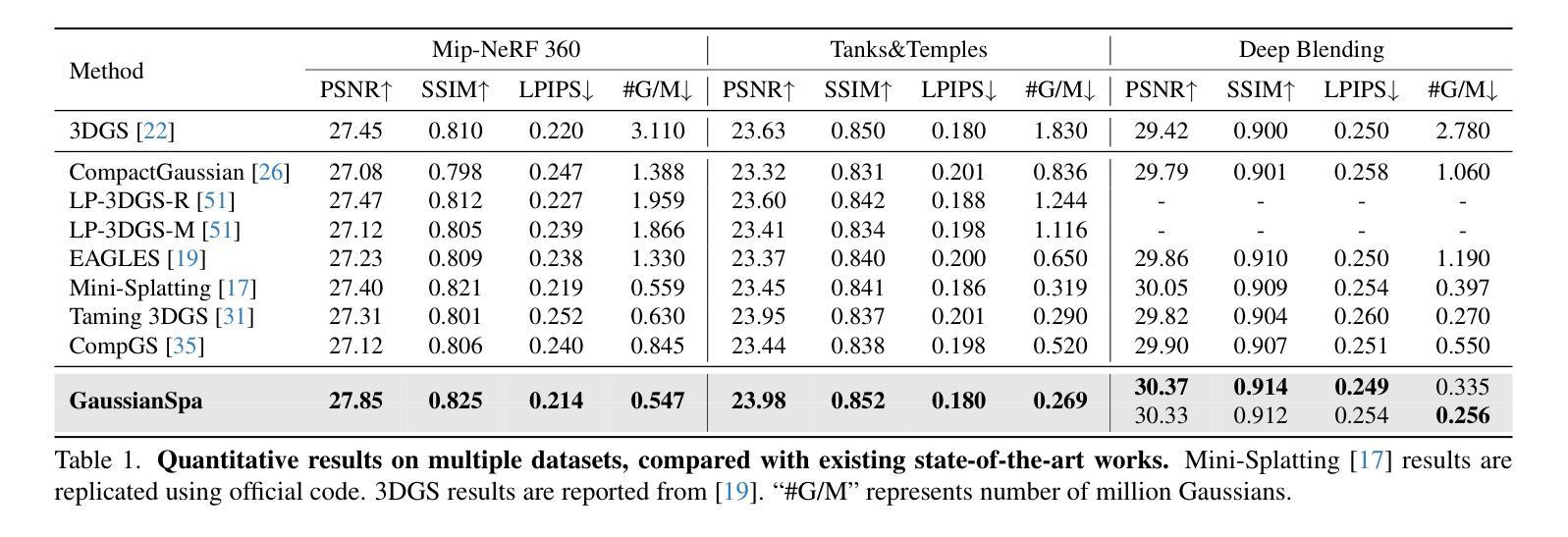
E-3DGS: Gaussian Splatting with Exposure and Motion Events
Authors:Xiaoting Yin, Hao Shi, Yuhan Bao, Zhenshan Bing, Yiyi Liao, Kailun Yang, Kaiwei Wang
Achieving 3D reconstruction from images captured under optimal conditions has been extensively studied in the vision and imaging fields. However, in real-world scenarios, challenges such as motion blur and insufficient illumination often limit the performance of standard frame-based cameras in delivering high-quality images. To address these limitations, we incorporate a transmittance adjustment device at the hardware level, enabling event cameras to capture both motion and exposure events for diverse 3D reconstruction scenarios. Motion events (triggered by camera or object movement) are collected in fast-motion scenarios when the device is inactive, while exposure events (generated through controlled camera exposure) are captured during slower motion to reconstruct grayscale images for high-quality training and optimization of event-based 3D Gaussian Splatting (3DGS). Our framework supports three modes: High-Quality Reconstruction using exposure events, Fast Reconstruction relying on motion events, and Balanced Hybrid optimizing with initial exposure events followed by high-speed motion events. On the EventNeRF dataset, we demonstrate that exposure events significantly improve fine detail reconstruction compared to motion events and outperform frame-based cameras under challenging conditions such as low illumination and overexposure. Furthermore, we introduce EME-3D, a real-world 3D dataset with exposure events, motion events, camera calibration parameters, and sparse point clouds. Our method achieves faster and higher-quality reconstruction than event-based NeRF and is more cost-effective than methods combining event and RGB data. E-3DGS sets a new benchmark for event-based 3D reconstruction with robust performance in challenging conditions and lower hardware demands. The source code and dataset will be available at https://github.com/MasterHow/E-3DGS.
在视觉和成像领域,从最优条件下捕获的图像进行3D重建已经得到了广泛的研究。然而,在真实场景中,运动模糊和照明不足等挑战往往限制了基于标准帧的相机在提供高质量图像方面的性能。为了解决这些局限性,我们在硬件层面融入了一个透光率调整设备,使得事件相机能够捕捉运动和曝光事件,适用于多种3D重建场景。当设备处于非活跃状态时,会收集由相机或物体移动触发的运动事件,而在较慢的运动过程中捕捉曝光事件(通过受控的相机曝光生成),以重建灰度图像,用于基于事件的高品质训练和优化的三维高斯延展技术(Event-Based 3D Gaussian Splatting,简称3DGS)。我们的框架支持三种模式:使用曝光事件的优质重建、依赖运动事件的快速重建以及平衡混合优化模式,先通过初始曝光事件再进行高速运动事件。在EventNeRF数据集上,我们证明了与运动事件相比,曝光事件能显著改善精细细节的重建效果,并在低光照和过度曝光等挑战性条件下优于基于帧的相机。此外,我们还引入了包含曝光事件、运动事件、相机校准参数和稀疏点云的真实世界三维数据集EME-3D。我们的方法实现了比基于事件的NeRF更快、更高质量的重建效果,并且相比于结合事件和RGB数据的方法更为经济实惠。E-3DGS为基于事件的3D重建设定了一个新的基准,其在具有挑战性的条件下表现出稳健的性能并降低了硬件需求。源代码和数据集将在https://github.com/MasterHow/E-3DGS上提供。
论文及项目相关链接
PDF Accepted to Applied Optics (AO). The source code and dataset will be available at https://github.com/MasterHow/E-3DGS
Summary
本文介绍了在理想条件下从图像实现3D重建已被广泛研究,但在真实场景中,面临如运动模糊和光照不足等挑战,限制了标准帧基相机的高性能表现。为此,研究者们在硬件层面融入了一种透射调整装置,使事件相机能够捕捉运动和曝光事件,适用于各种3D重建场景。利用事件采集运动信息并结合曝光事件实现高质量图像,用于训练和优化基于事件的高斯平铺(3DGS)。本文提出了一种新方法E-3DGS,其包括三种模式以适应不同需求。相较于仅使用运动事件或基于帧的相机,暴露事件能够显著改善细节重建。同时引入了包含事件参数的EME-3D数据集以供使用。最终实验结果表明新方法速度快且精度高。该文章的重点内容摘要。尽管面临复杂条件和较低硬件需求的挑战,但其提供了一个可靠的框架实现高效果性能事件基础的三维重建。。总结来看这是一种创新性较高的研究成果。Key Takeaways:
- 实现了一种基于事件相机的高性能三维重建方法E-3DGS,解决了真实场景中运动模糊和光照不足的问题。
- 通过在硬件层面融入透射调整装置,捕捉运动和曝光事件用于不同需求的重建场景。
点此查看论文截图
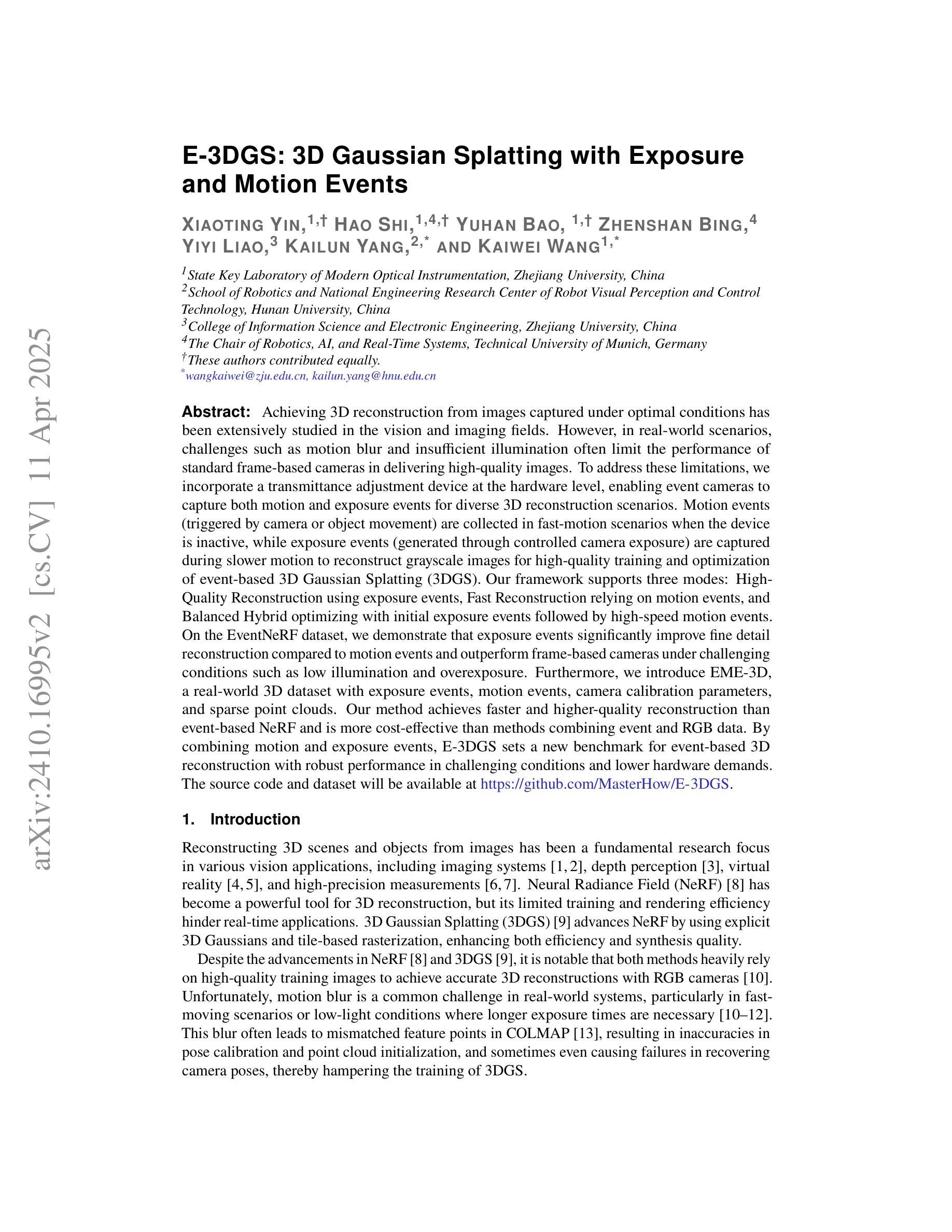
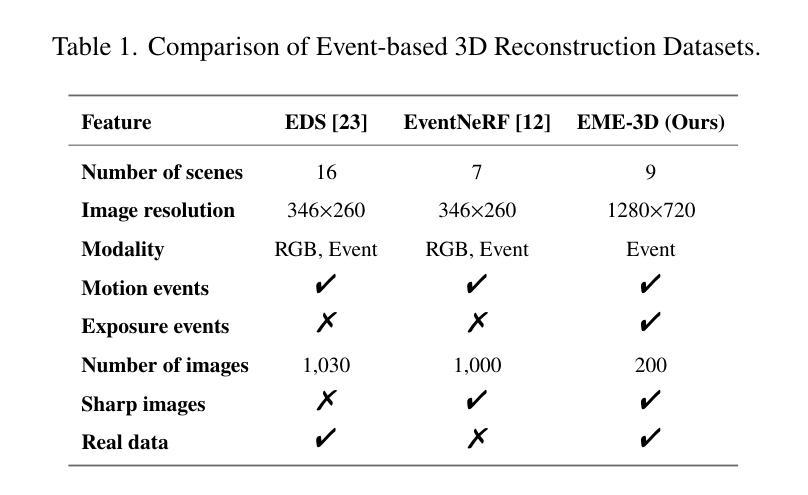
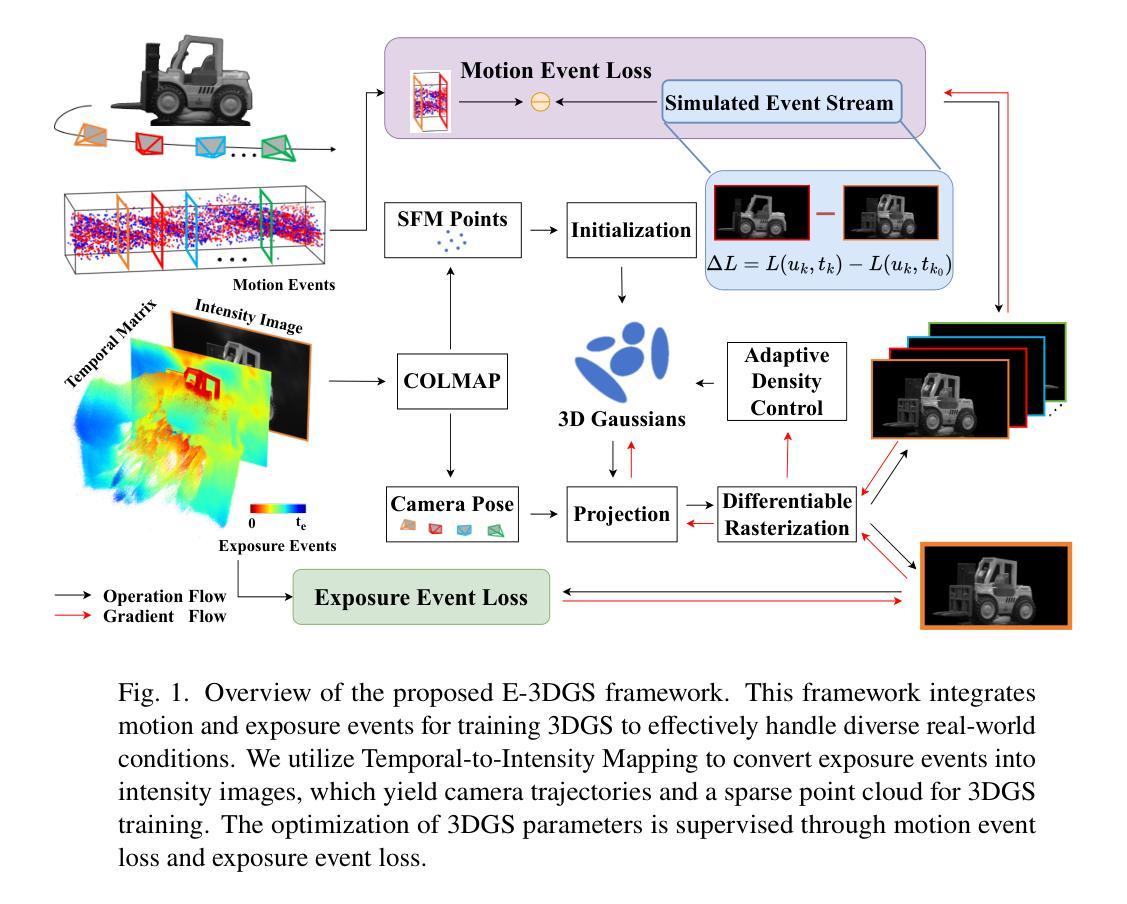
Generative Object Insertion in Gaussian Splatting with a Multi-View Diffusion Model
Authors:Hongliang Zhong, Can Wang, Jingbo Zhang, Jing Liao
Generating and inserting new objects into 3D content is a compelling approach for achieving versatile scene recreation. Existing methods, which rely on SDS optimization or single-view inpainting, often struggle to produce high-quality results. To address this, we propose a novel method for object insertion in 3D content represented by Gaussian Splatting. Our approach introduces a multi-view diffusion model, dubbed MVInpainter, which is built upon a pre-trained stable video diffusion model to facilitate view-consistent object inpainting. Within MVInpainter, we incorporate a ControlNet-based conditional injection module to enable controlled and more predictable multi-view generation. After generating the multi-view inpainted results, we further propose a mask-aware 3D reconstruction technique to refine Gaussian Splatting reconstruction from these sparse inpainted views. By leveraging these fabricate techniques, our approach yields diverse results, ensures view-consistent and harmonious insertions, and produces better object quality. Extensive experiments demonstrate that our approach outperforms existing methods.
在3D内容中生成并插入新物体是实现多样场景重建的吸引人的方法。现有的方法通常依赖于SDS优化或单视图补全,很难产生高质量的结果。为了解决这个问题,我们提出了一种新的在3D内容中插入物体的方法,该方法由高斯拼贴表示。我们的方法引入了一种多视图扩散模型,称为MVInpainter,它基于预训练的稳定视频扩散模型,以促进视图一致的对象补全。在MVInpainter中,我们采用了基于ControlNet的条件注入模块,以实现可控和更可预测的多视图生成。在生成多视图补全结果后,我们进一步提出了一种掩膜感知的3D重建技术,以从这些稀疏的补全视图中优化高斯拼贴重建。通过利用这些制作技术,我们的方法能产生多样化的结果,确保视图一致且和谐的插入,并产生更好的物体质量。大量实验表明,我们的方法优于现有方法。
论文及项目相关链接
PDF Accepted by Visual Informatics. Project Page: https://github.com/JiuTongBro/MultiView_Inpaint
Summary
本文提出了一种基于高斯平铺表示的三维内容中对象插入的新方法。该方法通过引入多视角扩散模型MVInpainter,结合预训练的稳定视频扩散模型,实现了视角一致的对象填充。通过控制网络基础的条件注入模块,实现了可控且更可预测的多视角生成。同时,提出了一种掩膜感知的3D重建技术,用于从稀疏填充视角优化高斯平铺重建。该方法能产生多样化的结果,确保视角一致且和谐地插入对象,并产生更好的对象质量。
Key Takeaways
- 提出了一种基于高斯平铺的三维内容中对象插入新方法,解决了现有方法质量不高的问题。
- 引入了多视角扩散模型MVInpainter,结合预训练稳定视频扩散模型,实现视角一致的对象填充。
- 使用了控制网络基础的条件注入模块,实现可控且预测性强的多视角生成。
- 提出了掩膜感知的3D重建技术,用于优化从稀疏填充视角的高斯平铺重建。
- 方法能产生多样化结果,确保视角一致且和谐地插入对象。
- 该方法提高了对象插入的质量,优于现有方法。
点此查看论文截图
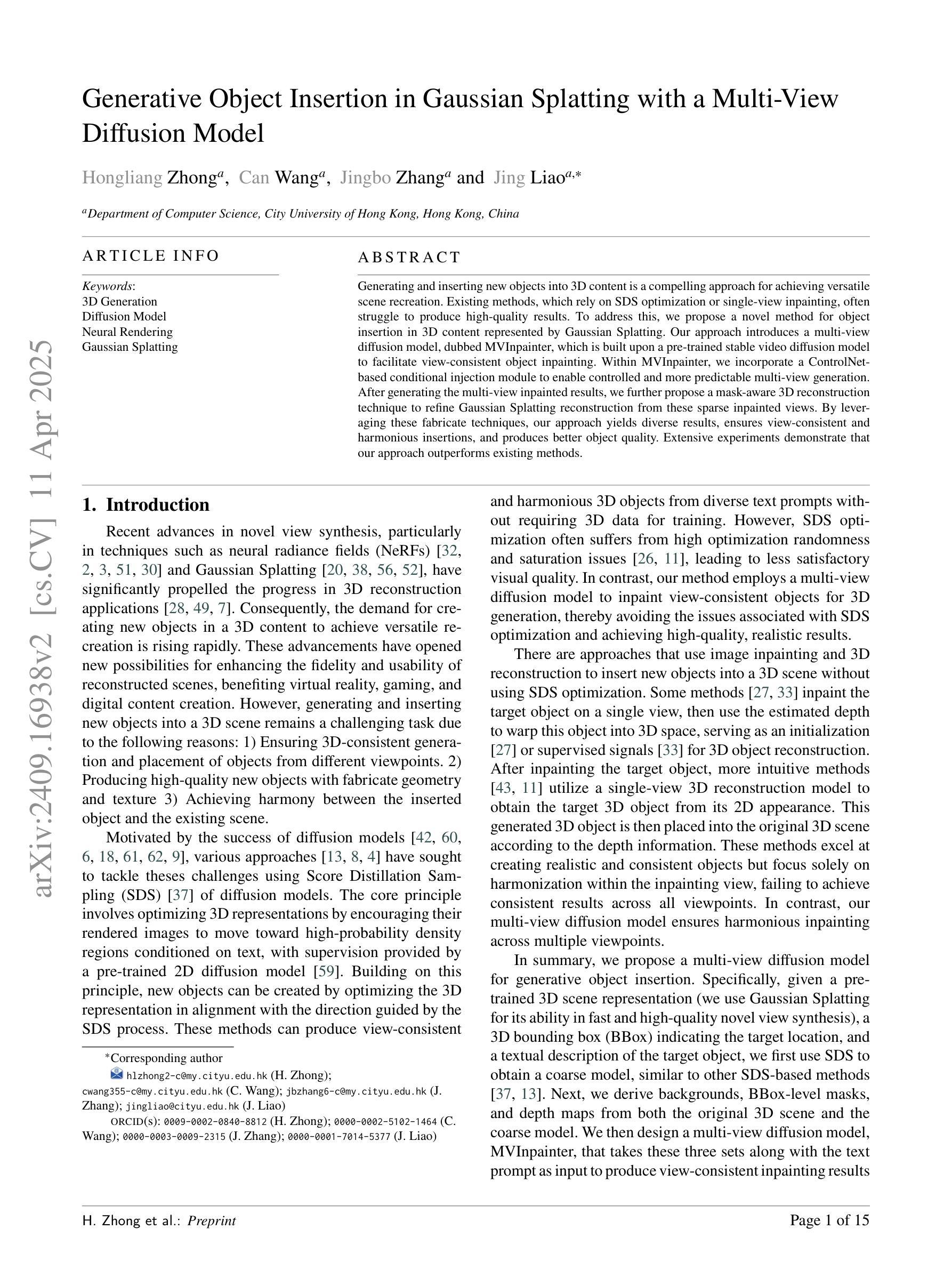
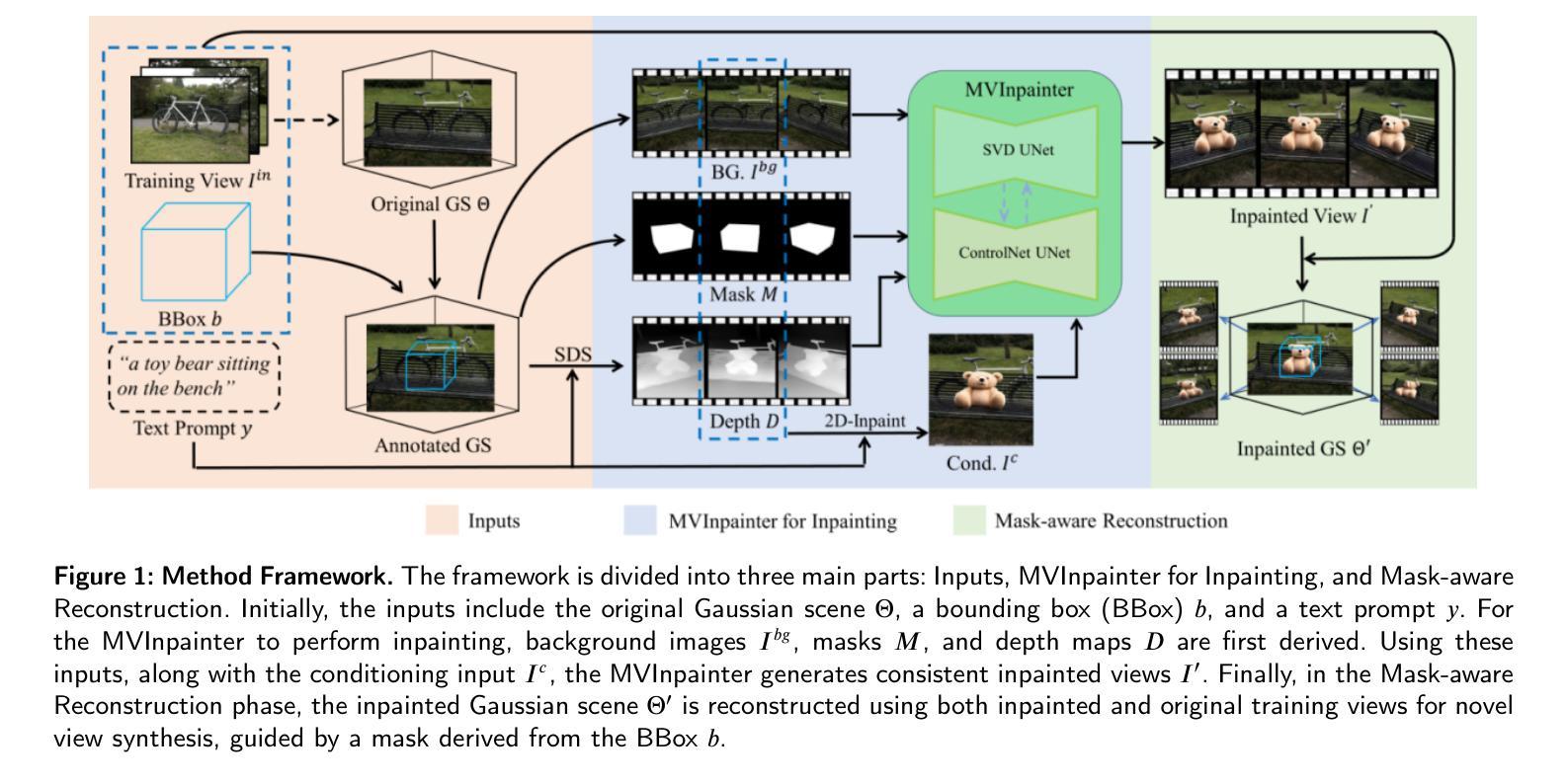
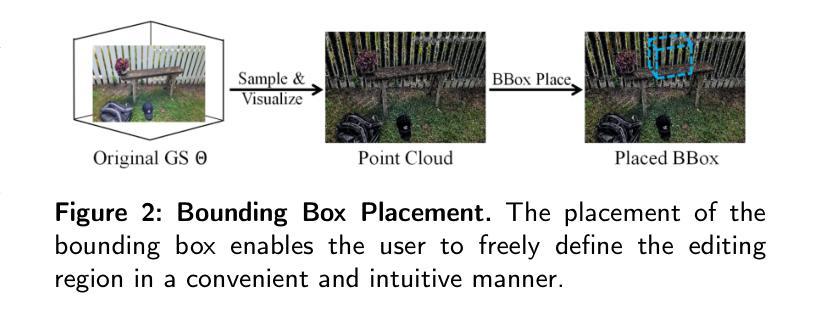
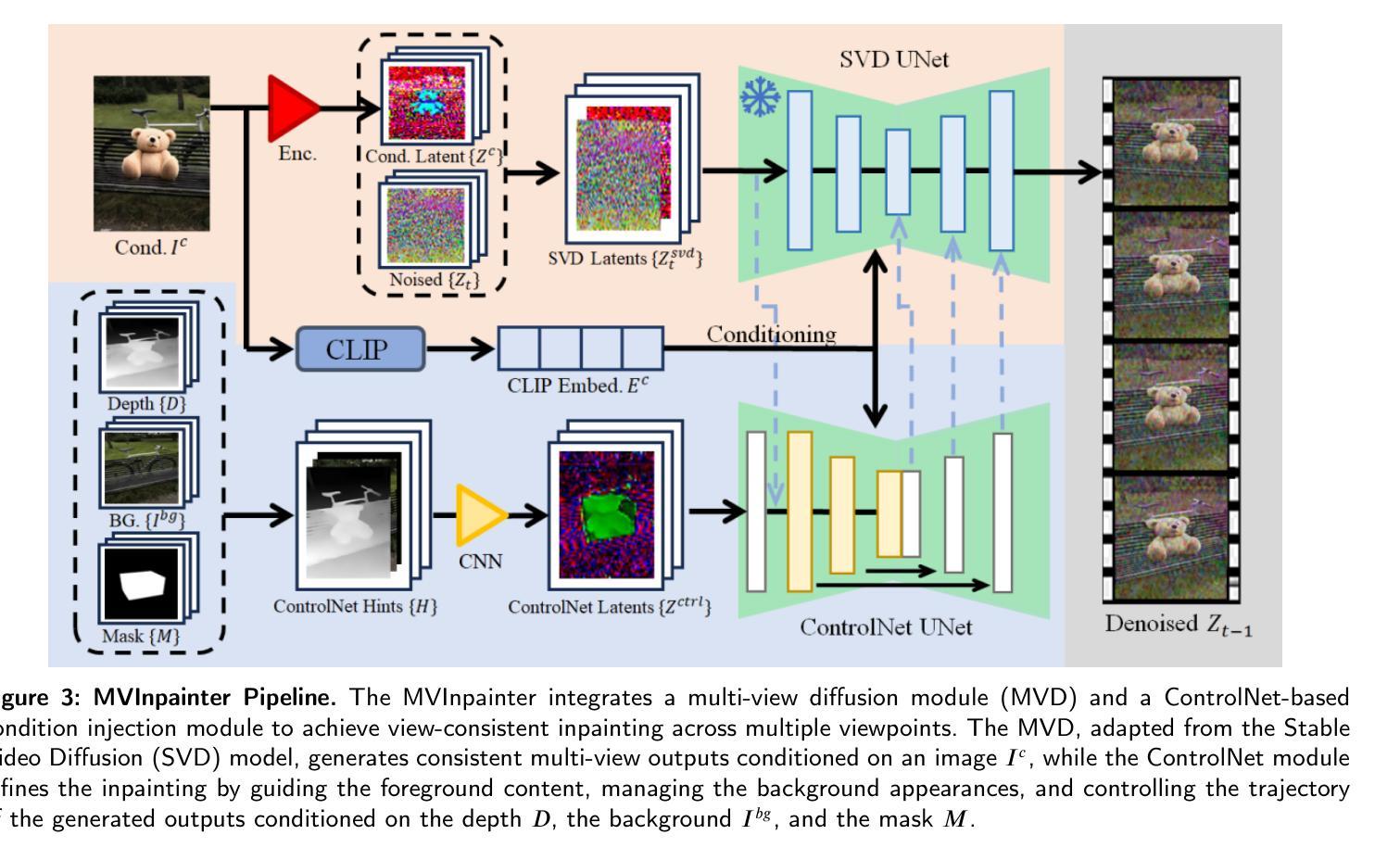
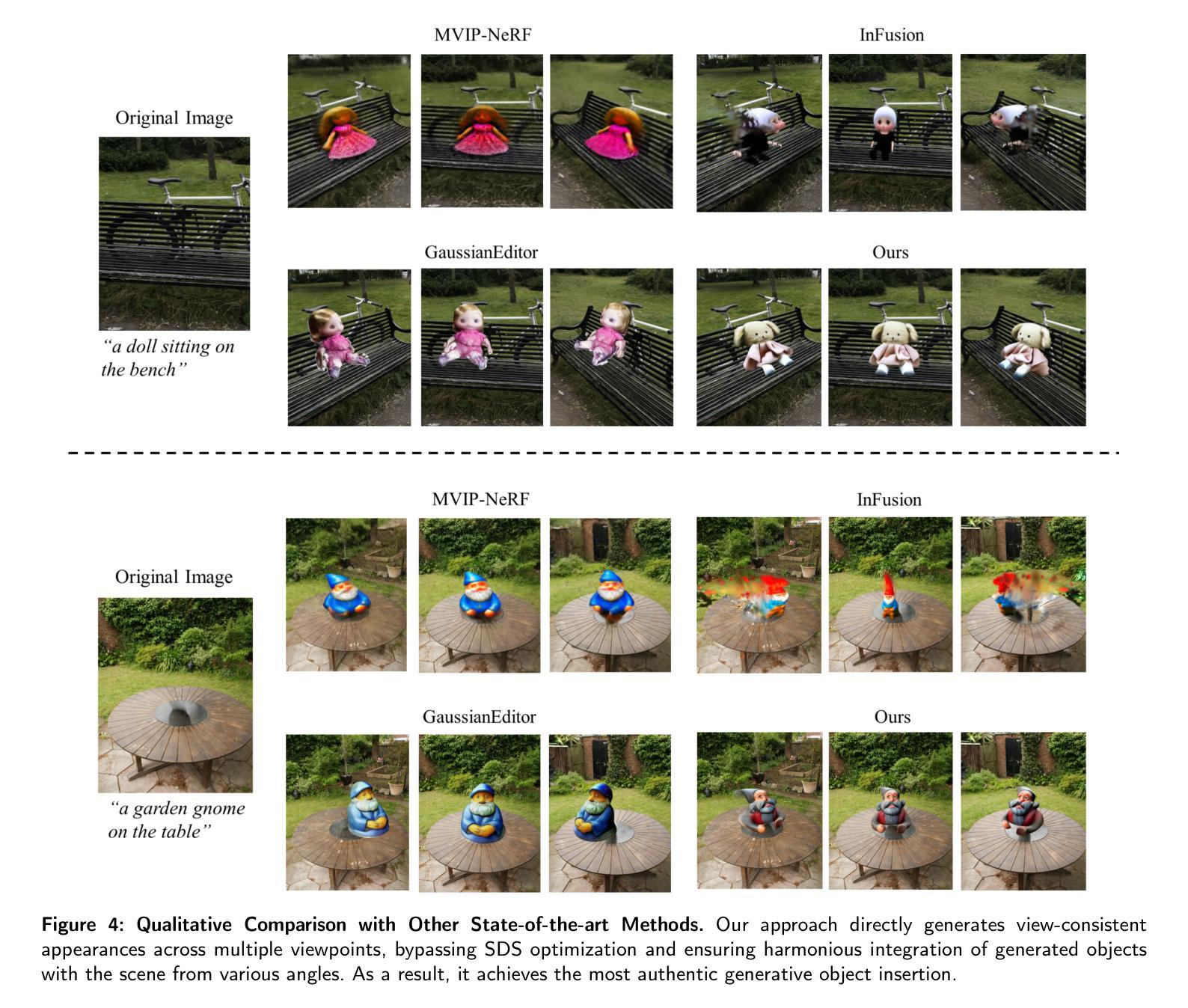
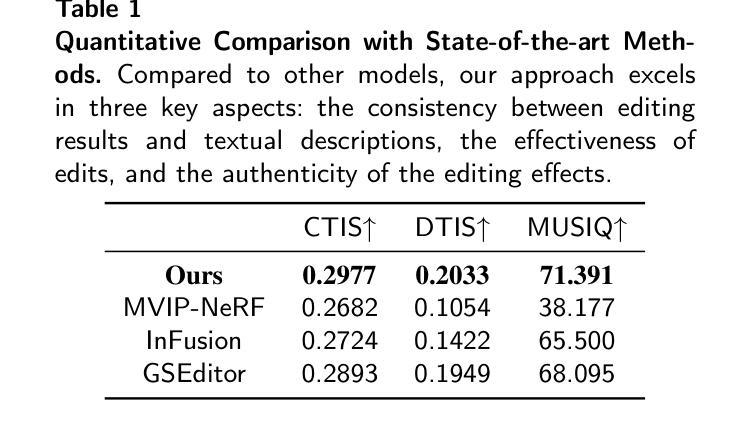