⚠️ 以下所有内容总结都来自于 大语言模型的能力,如有错误,仅供参考,谨慎使用
🔴 请注意:千万不要用于严肃的学术场景,只能用于论文阅读前的初筛!
💗 如果您觉得我们的项目对您有帮助 ChatPaperFree ,还请您给我们一些鼓励!⭐️ HuggingFace免费体验
2025-04-15 更新
COP-GEN-Beta: Unified Generative Modelling of COPernicus Imagery Thumbnails
Authors:Miguel Espinosa, Valerio Marsocci, Yuru Jia, Elliot J. Crowley, Mikolaj Czerkawski
In remote sensing, multi-modal data from various sensors capturing the same scene offers rich opportunities, but learning a unified representation across these modalities remains a significant challenge. Traditional methods have often been limited to single or dual-modality approaches. In this paper, we introduce COP-GEN-Beta, a generative diffusion model trained on optical, radar, and elevation data from the Major TOM dataset. What sets COP-GEN-Beta apart is its ability to map any subset of modalities to any other, enabling zero-shot modality translation after training. This is achieved through a sequence-based diffusion transformer, where each modality is controlled by its own timestep embedding. We extensively evaluate COP-GEN-Beta on thumbnail images from the Major TOM dataset, demonstrating its effectiveness in generating high-quality samples. Qualitative and quantitative evaluations validate the model’s performance, highlighting its potential as a powerful pre-trained model for future remote sensing tasks.
在遥感领域,从不同传感器捕捉同一场景的多模态数据提供了丰富的机会,但学习这些模态的统一表示仍然是一个重大挑战。传统方法通常局限于单一或双模态方法。在本文中,我们介绍了COP-GEN-Beta,这是一种在Major TOM数据集的光学、雷达和地形数据上训练的生成扩散模型。COP-GEN-Beta的独特之处在于,它能够将任何模态子集映射到其他模态,从而在训练后实现零样本模态翻译。这是通过基于序列的扩散变压器实现的,其中每个模态由其自己的时间步长嵌入控制。我们在Major TOM数据集的缩略图图像上对COP-GEN-Beta进行了广泛评估,证明了其生成高质量样本的有效性。定性和定量评估验证了该模型的性能,突显了其在未来遥感任务中作为强大预训练模型的潜力。
论文及项目相关链接
PDF Accepted at CVPR 2025 Workshop MORSE
Summary
本文介绍了在遥感领域中,多模态数据所带来的丰富机会和挑战。提出了一种名为COP-GEN-Beta的生成扩散模型,该模型能够在光学、雷达和高度数据上进行训练,并能实现任意模态之间的转换。通过基于序列的扩散变压器实现,每个模态由其自己的时间步嵌入控制。在Major TOM数据集上的缩略图图像评估中,证明了COP-GEN-Beta生成高质量样本的有效性。该模型表现出强大的性能,有望在未来遥感任务中作为预训练模型应用。
Key Takeaways
- 多模态数据在遥感中提供了丰富的信息机会,但学习跨这些模态的统一表示仍然是一个挑战。
- 传统方法通常局限于单一或双模态方法。
- COP-GEN-Beta是一种生成扩散模型,能够在多种模态数据进行训练,包括光学、雷达和高度数据。
- COP-GEN-Beta能够实现零样本模态翻译,即任何模态都可以映射到其他模态。
- 这通过基于序列的扩散变压器实现,每个模态由其自己的时间步嵌入控制。
- 在Major TOM数据集上的缩略图图像评估中,COP-GEN-Beta表现出生成高质量样本的能力。
点此查看论文截图
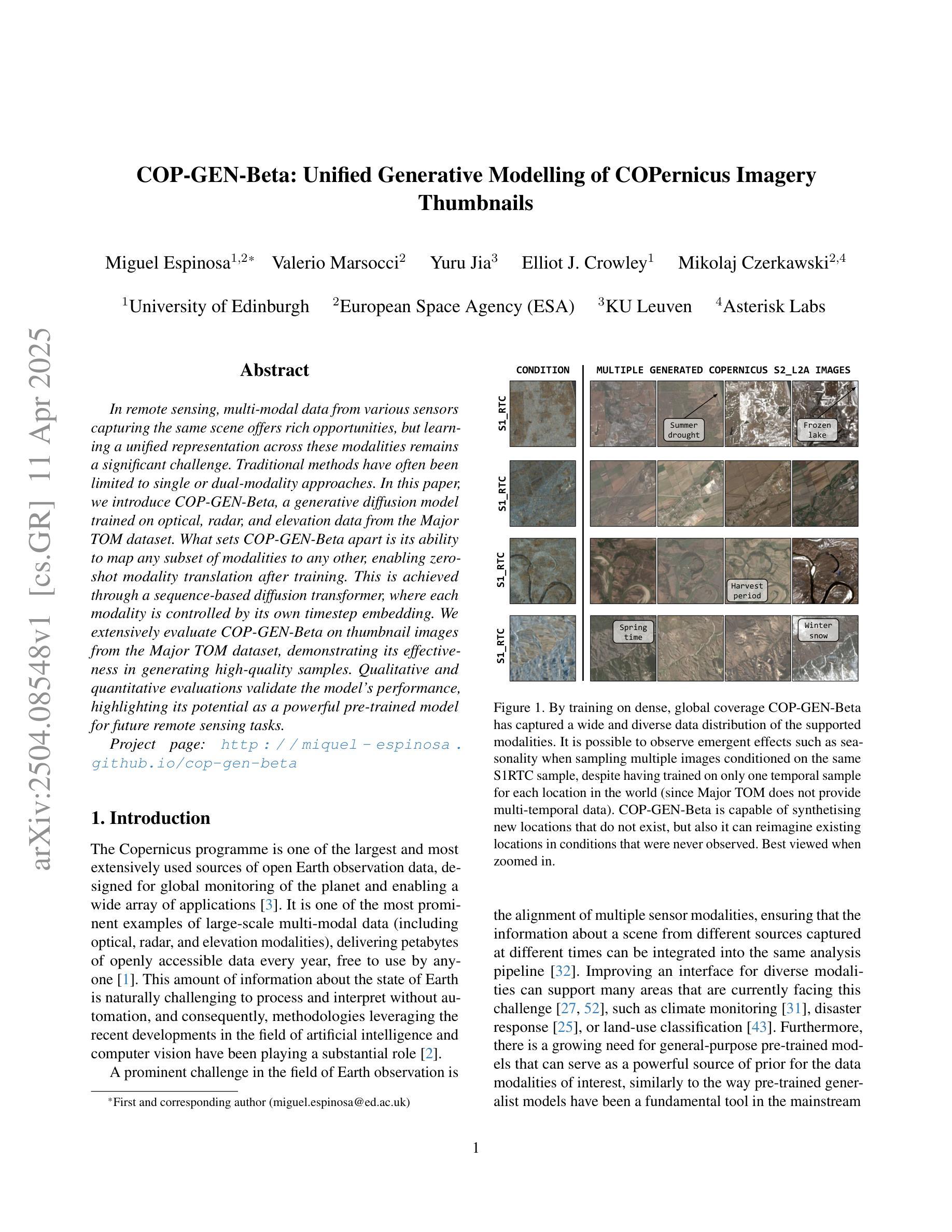
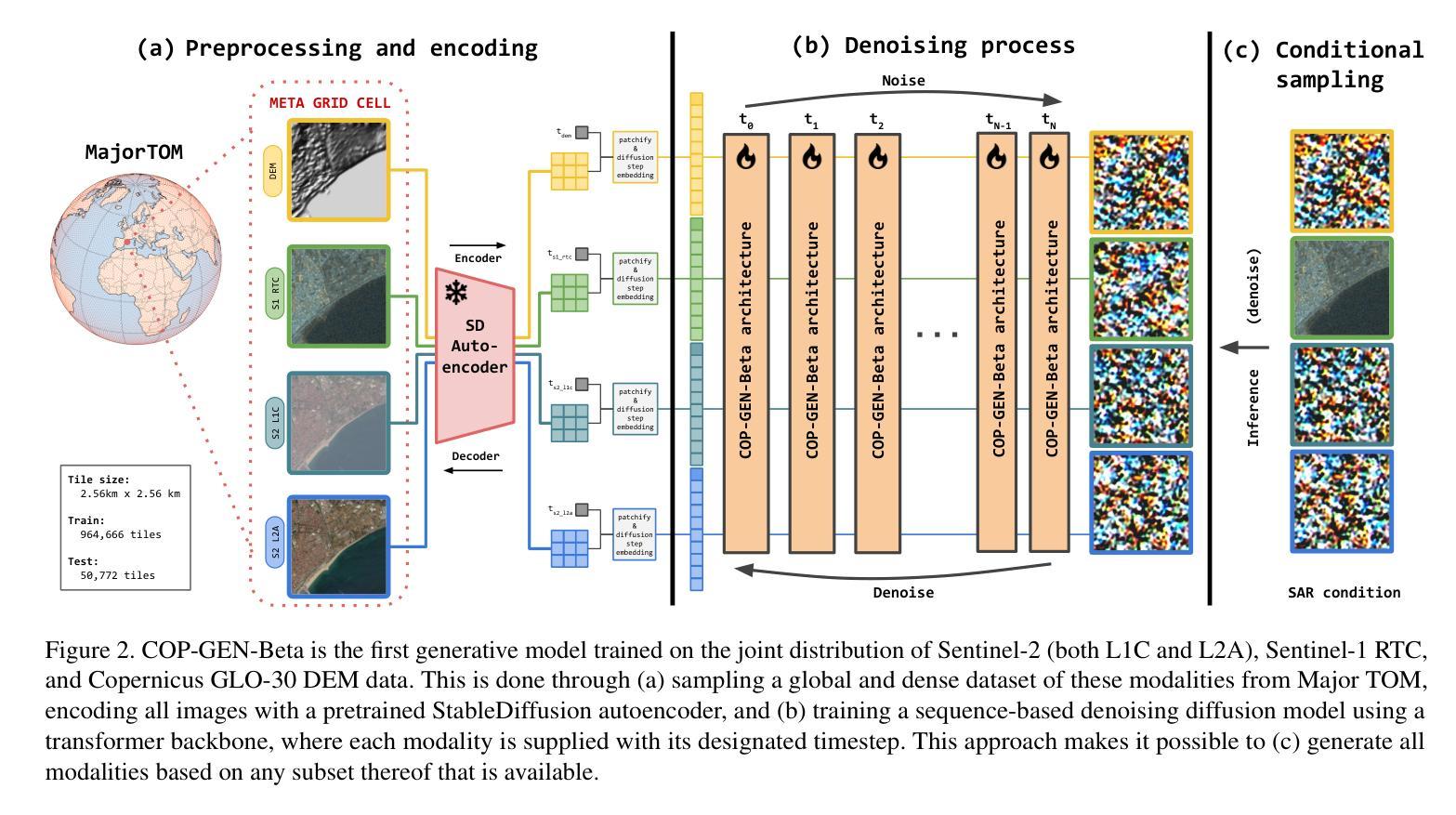
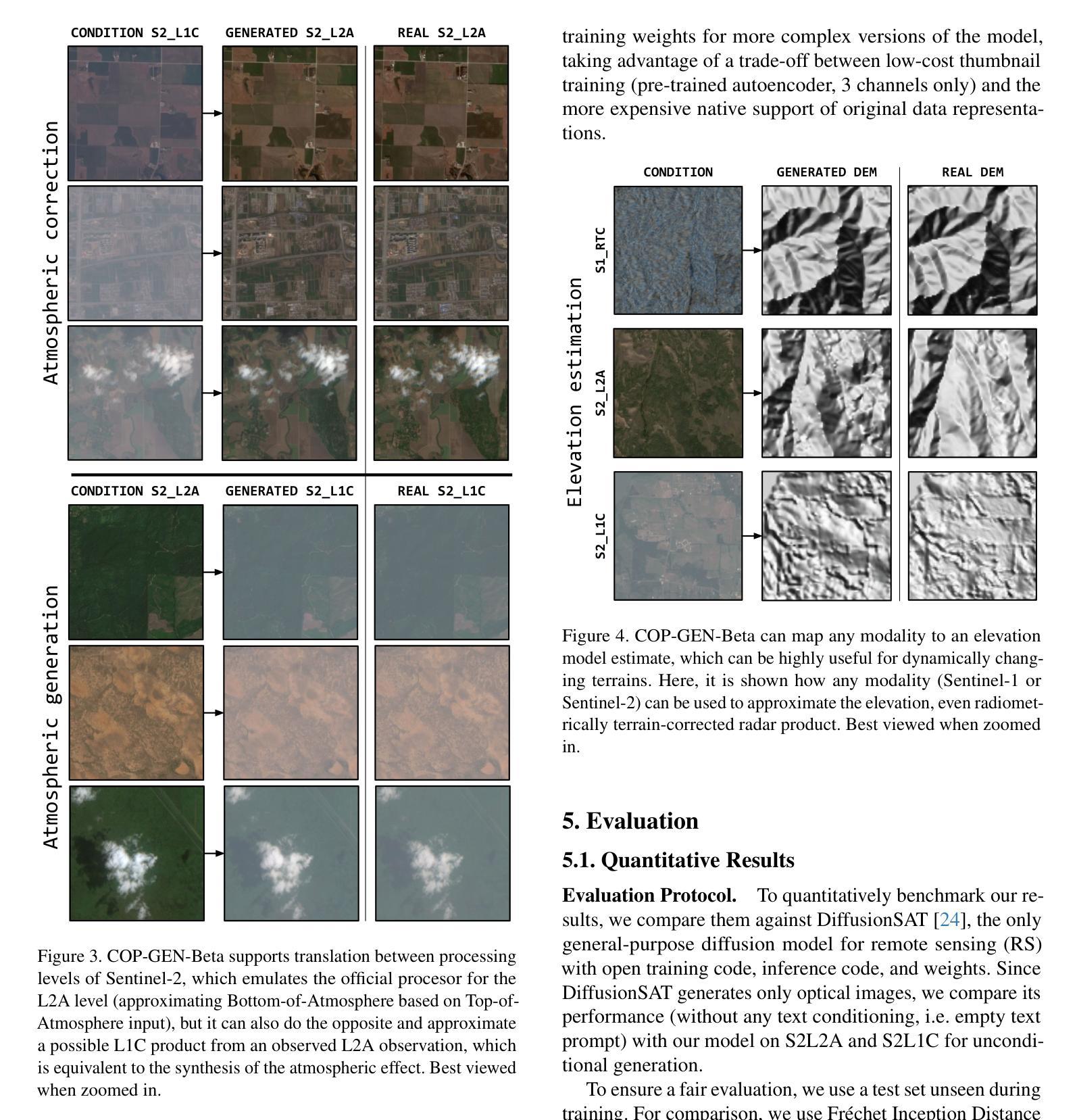
Fine-Grained Retrieval-Augmented Generation for Visual Question Answering
Authors:Zhengxuan Zhang, Yin Wu, Yuyu Luo, Nan Tang
Visual Question Answering (VQA) focuses on providing answers to natural language questions by utilizing information from images. Although cutting-edge multimodal large language models (MLLMs) such as GPT-4o achieve strong performance on VQA tasks, they frequently fall short in accessing domain-specific or the latest knowledge. To mitigate this issue, retrieval-augmented generation (RAG) leveraging external knowledge bases (KBs), referred to as KB-VQA, emerges as a promising approach. Nevertheless, conventional unimodal retrieval techniques, which translate images into textual descriptions, often result in the loss of critical visual details. This study presents fine-grained knowledge units, which merge textual snippets with entity images stored in vector databases. Furthermore, we introduce a knowledge unit retrieval-augmented generation framework (KU-RAG) that integrates fine-grained retrieval with MLLMs. The proposed KU-RAG framework ensures precise retrieval of relevant knowledge and enhances reasoning capabilities through a knowledge correction chain. Experimental findings demonstrate that our approach significantly boosts the performance of leading KB-VQA methods, achieving an average improvement of approximately 3% and up to 11% in the best case.
视觉问答(VQA)专注于通过利用图像中的信息来提供对自然语言问题的答案。尽管最前沿的多模态大型语言模型(MLLMs),如GPT-4o,在VQA任务上表现出强大的性能,但它们通常在访问特定领域或最新知识时显得不足。为了缓解这个问题,利用外部知识库(KBs)的检索增强生成(RAG),被称为KB-VQA,作为一种有前途的方法而出现。然而,传统的单模态检索技术将图像翻译成文本描述,这往往会导致关键视觉细节的丢失。本研究提出了精细粒度知识单元,其将文本片段与存储在向量数据库中的实体图像进行合并。此外,我们介绍了知识单元检索增强生成框架(KU-RAG),它将精细粒度检索与MLLMs集成在一起。所提出的KU-RAG框架确保了相关知识的精确检索,并通过知识校正链增强了推理能力。实验结果表明,我们的方法显著提高了领先的KB-VQA方法的性能,平均提高了约3%,在最佳情况下提高了高达11%。
论文及项目相关链接
Summary
视觉问答(VQA)旨在通过利用图像中的信息来回答自然语言问题。尽管先进的多模态大型语言模型(MLLMs)如GPT-4o在VQA任务上表现出强大的性能,但它们通常无法获取特定领域的最新知识。为了缓解这一问题,利用外部知识库的检索增强生成(RAG)方法,即KB-VQA,成为一种有前景的方法。然而,传统的单模态检索技术将图像转换为文本描述,往往会丢失关键的视觉细节。本研究提出精细知识单元,将文本片段与存储在向量数据库中的实体图像合并。此外,我们引入了知识单元检索增强生成框架(KU-RAG),将精细检索与MLLMs相结合。KU-RAG框架确保精确检索相关知识,并通过知识校正链增强推理能力。实验结果表明,我们的方法显著提高了领先的KB-VQA方法的性能,平均提高了约3%,最高提高了11%。
Key Takeaways
- VQA主要依赖图像信息回答自然语言问题。
- 多模态大型语言模型在VQA任务上表现良好,但在获取特定领域或最新知识方面存在不足。
- 检索增强生成方法(RAG)结合外部知识库(KBs)以缓解知识获取问题,形成KB-VQA。
- 传统单模态检索技术会丢失视觉细节。
- 引入精细知识单元和知识单元检索增强生成框架(KU-RAG)。
- KU-RAG能精确检索相关知识,并通过知识校正链增强推理能力。
点此查看论文截图
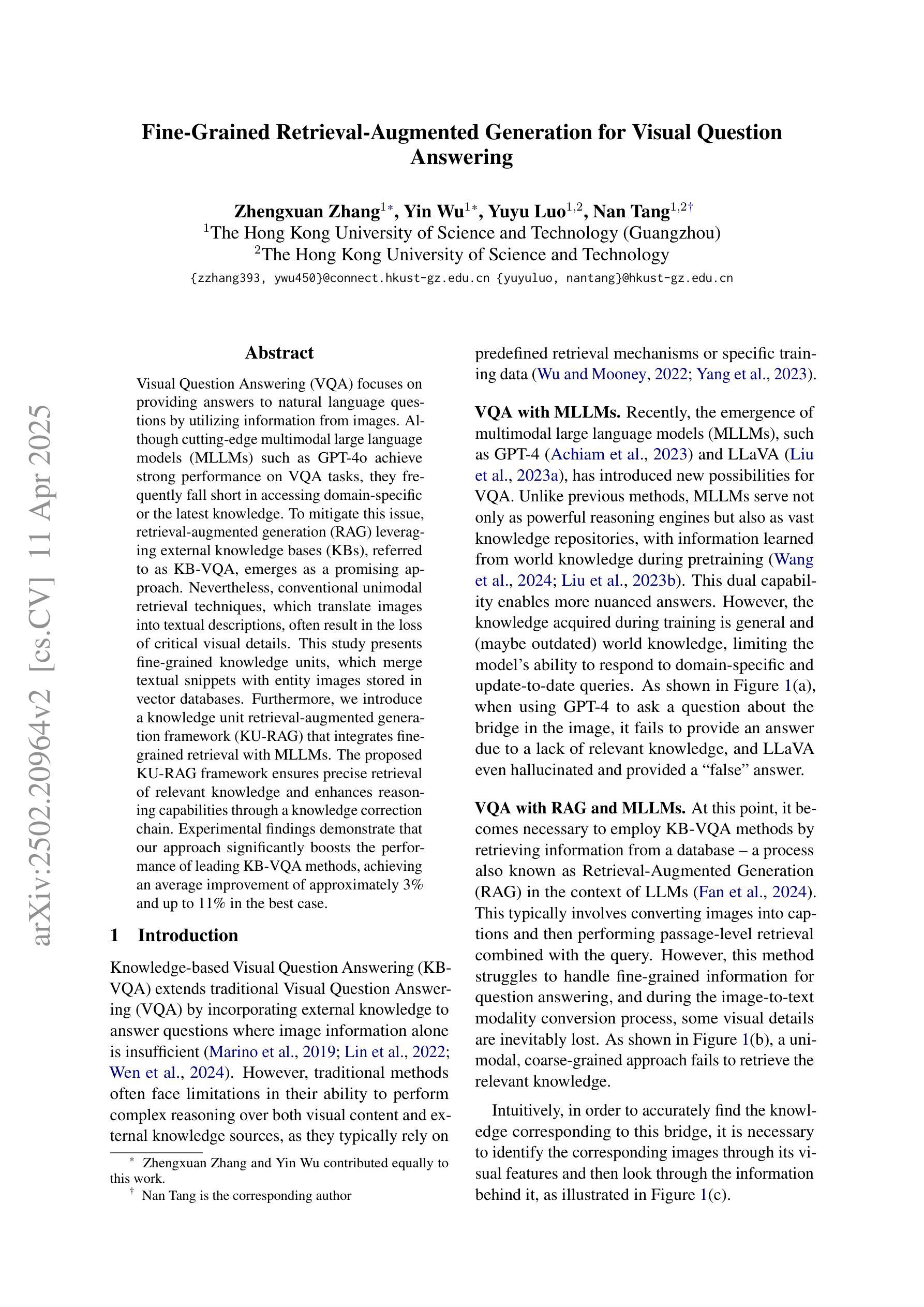
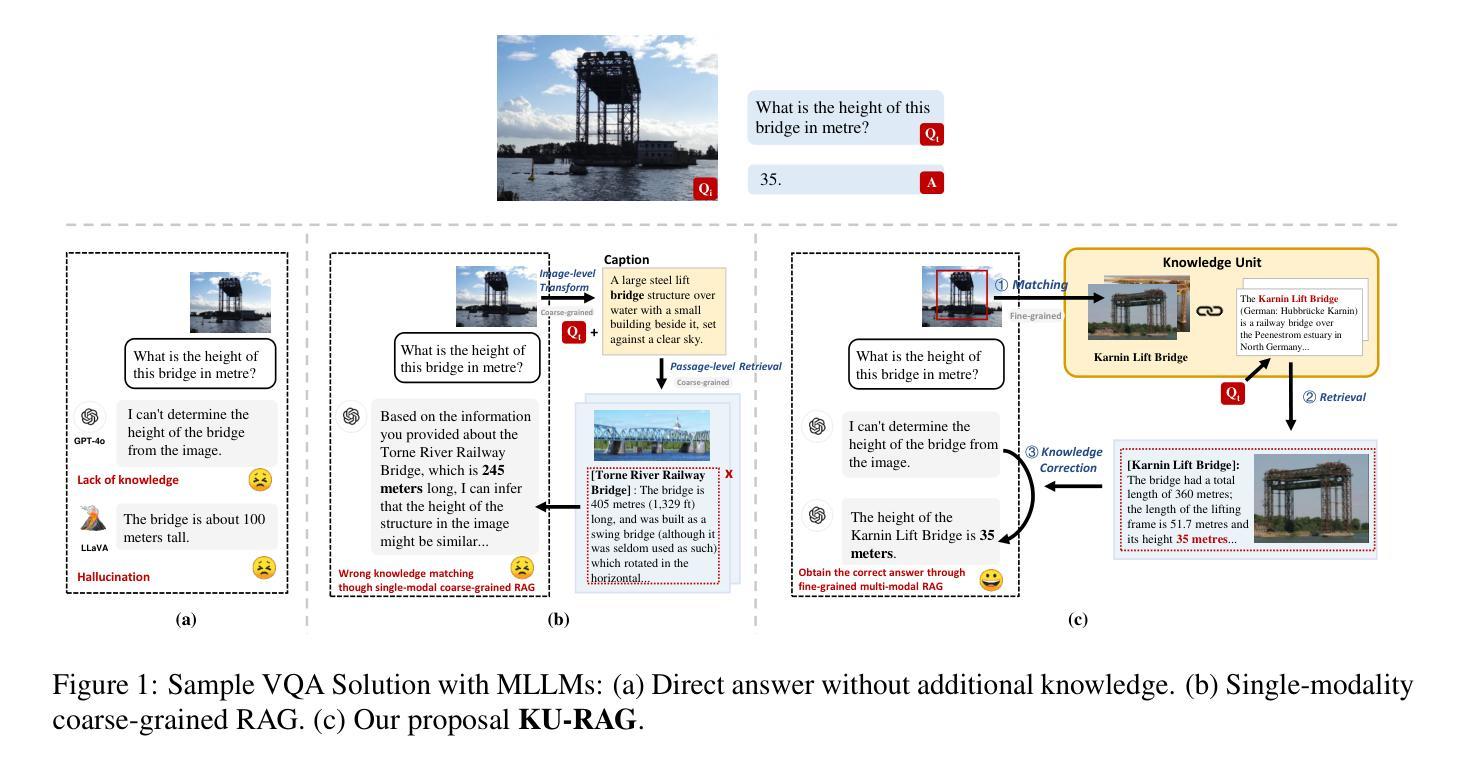
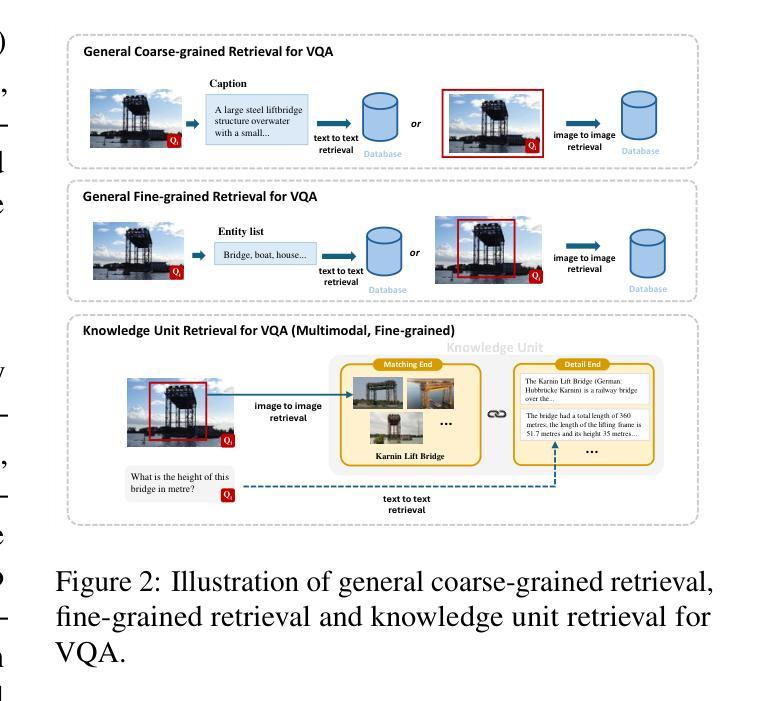
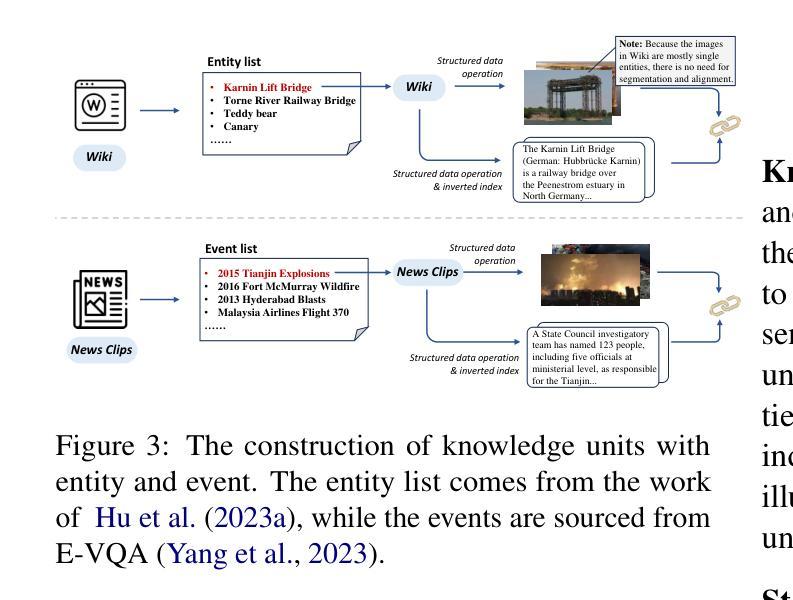
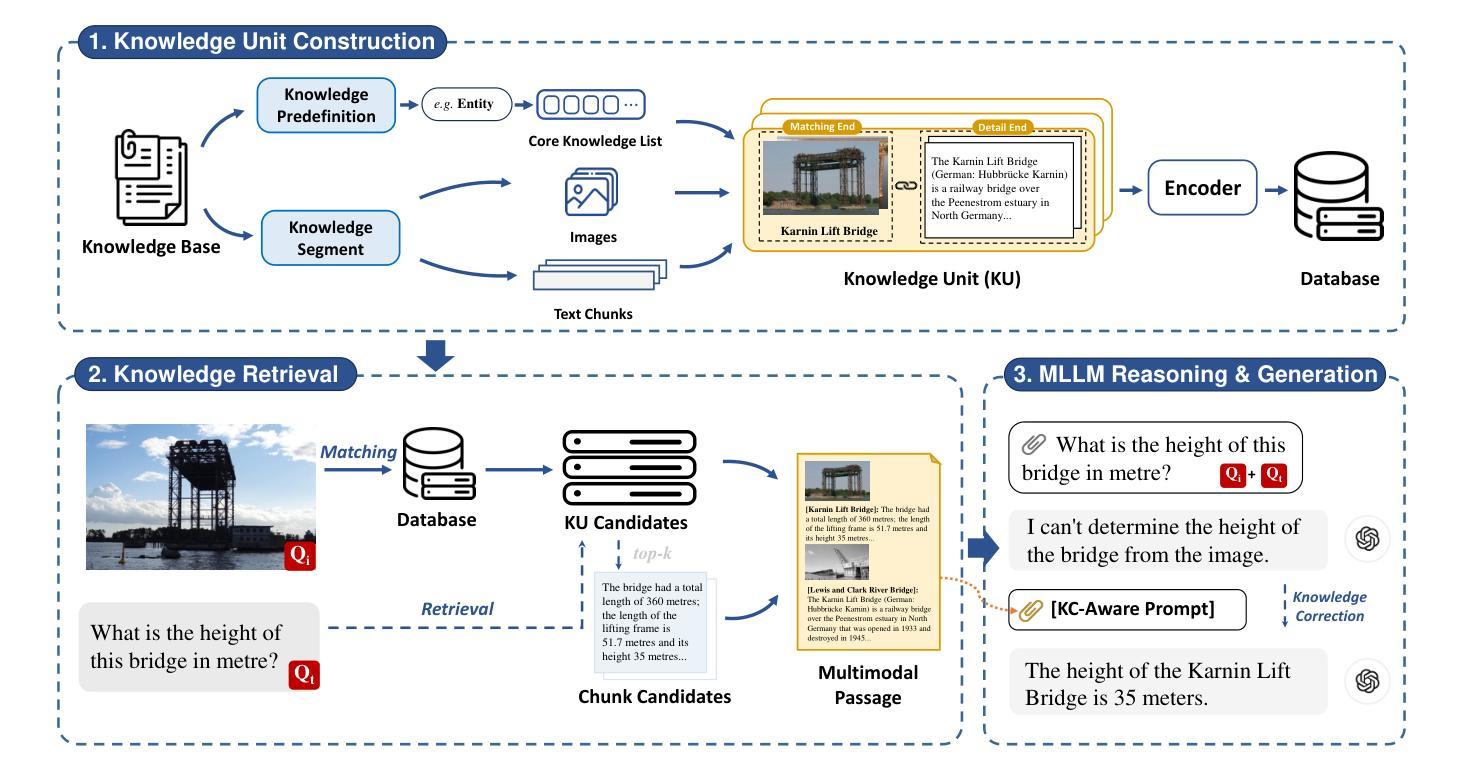