⚠️ 以下所有内容总结都来自于 大语言模型的能力,如有错误,仅供参考,谨慎使用
🔴 请注意:千万不要用于严肃的学术场景,只能用于论文阅读前的初筛!
💗 如果您觉得我们的项目对您有帮助 ChatPaperFree ,还请您给我们一些鼓励!⭐️ HuggingFace免费体验
2025-04-15 更新
Generative AI for Film Creation: A Survey of Recent Advances
Authors:Ruihan Zhang, Borou Yu, Jiajian Min, Yetong Xin, Zheng Wei, Juncheng Nemo Shi, Mingzhen Huang, Xianghao Kong, Nix Liu Xin, Shanshan Jiang, Praagya Bahuguna, Mark Chan, Khushi Hora, Lijian Yang, Yongqi Liang, Runhe Bian, Yunlei Liu, Isabela Campillo Valencia, Patricia Morales Tredinick, Ilia Kozlov, Sijia Jiang, Peiwen Huang, Na Chen, Xuanxuan Liu, Anyi Rao
Generative AI (GenAI) is transforming filmmaking, equipping artists with tools like text-to-image and image-to-video diffusion, neural radiance fields, avatar generation, and 3D synthesis. This paper examines the adoption of these technologies in filmmaking, analyzing workflows from recent AI-driven films to understand how GenAI contributes to character creation, aesthetic styling, and narration. We explore key strategies for maintaining character consistency, achieving stylistic coherence, and ensuring motion continuity. Additionally, we highlight emerging trends such as the growing use of 3D generation and the integration of real footage with AI-generated elements. Beyond technical advancements, we examine how GenAI is enabling new artistic expressions, from generating hard-to-shoot footage to dreamlike diffusion-based morphing effects, abstract visuals, and unworldly objects. We also gather artists’ feedback on challenges and desired improvements, including consistency, controllability, fine-grained editing, and motion refinement. Our study provides insights into the evolving intersection of AI and filmmaking, offering a roadmap for researchers and artists navigating this rapidly expanding field.
生成式人工智能(GenAI)正在改变电影制作,为艺术家提供文本到图像、图像到视频扩散、神经辐射场、化身生成和3D合成等工具。本文研究了这些技术在电影制作中的应用,通过分析最近的AI驱动电影的工作流程,了解GenAI如何在角色创作、艺术风格和叙事方面做出贡献。我们探讨了保持角色一致性、实现风格连贯和确保动作连续性的关键策略。此外,我们还强调了新兴趋势,如日益增长的3D使用以及与AI生成元素相结合的实景拍摄。除了技术进步之外,我们还探讨了GenAI如何支持新的艺术表达形式,从生成难以拍摄的画面到基于梦幻扩散的变形效果、抽象视觉和非现实世界物体。我们还收集了艺术家对挑战和期望改进的反馈,包括一致性、可控性、精细编辑和运动优化。我们的研究为人工智能和电影制作不断演变的交集提供了深刻见解,为研究人员和艺术家在这个迅速扩展的领域提供了一条路线图。
论文及项目相关链接
PDF Accepted at CVPR 2025 CVEU workshop: AI for Creative Visual Content Generation Editing and Understanding
Summary
生成式人工智能(GenAI)在影视制作领域的应用正带来深刻变革,为艺术家提供文本转图像、图像转视频扩散、神经辐射场、虚拟角色生成及3D合成等工具。本文通过分析近期采用GenAI技术的电影制作流程,探讨GenAI如何在角色创作、美学风格及叙事等方面发挥作用。同时,本文关注如何保持角色一致性、实现风格连贯及确保动作连续性等关键策略,并强调3D技术的崛起及与真实影像的结合趋势。此外,GenAI如何促成新的艺术表现形式的探索,如生成难以拍摄的镜头、梦幻般的扩散变形效果、抽象视觉及异次元物体等。本文亦汇集艺术家对挑战及改进点的反馈,展望AI与影视制作领域的交融发展,为研究者及艺术家提供导航。
Key Takeaways
- 生成式人工智能在影视制作中带来革命性变化,提供多种工具支持。
- GenAI助力角色创作、美学风格及叙事发展。
- 关键策略包括保持角色一致性、实现风格连贯及确保动作连续性。
- 3D技术的崛起及与真实影像的结合成为新兴趋势。
- GenAI促成新的艺术表现形式的探索,如抽象视觉及异次元物体等。
- 艺术家对GenAI的挑战及改进点提供宝贵反馈。
点此查看论文截图
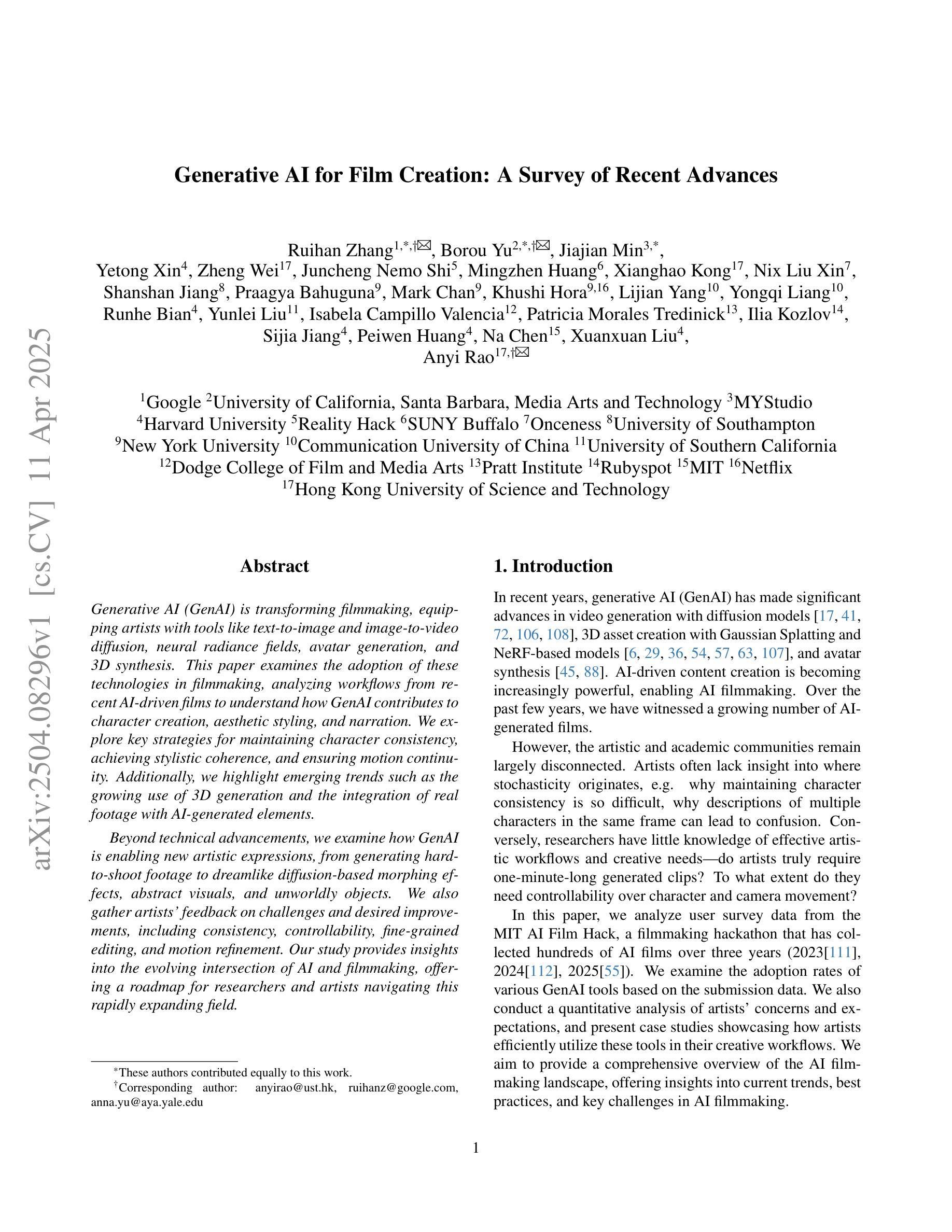
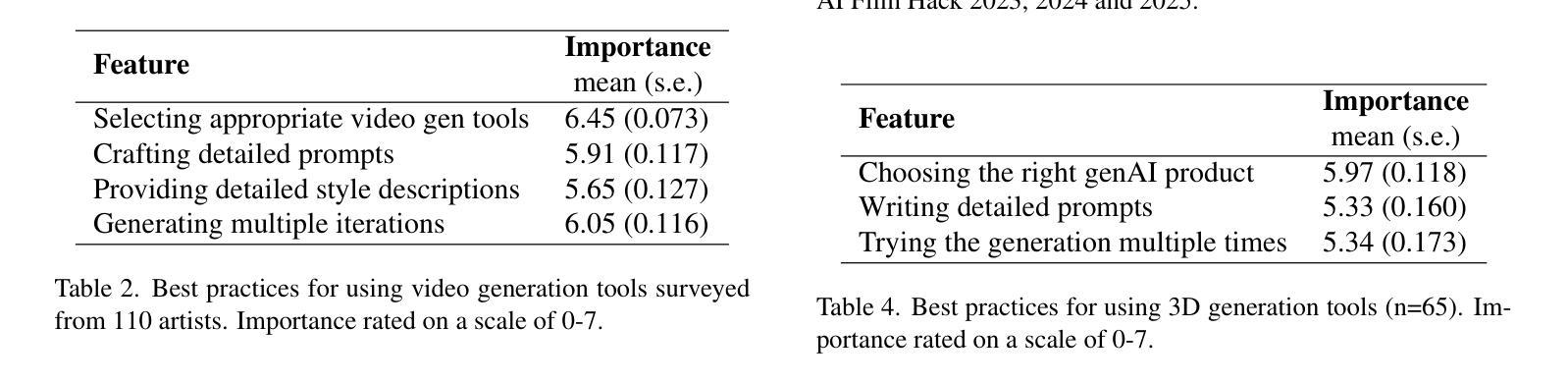
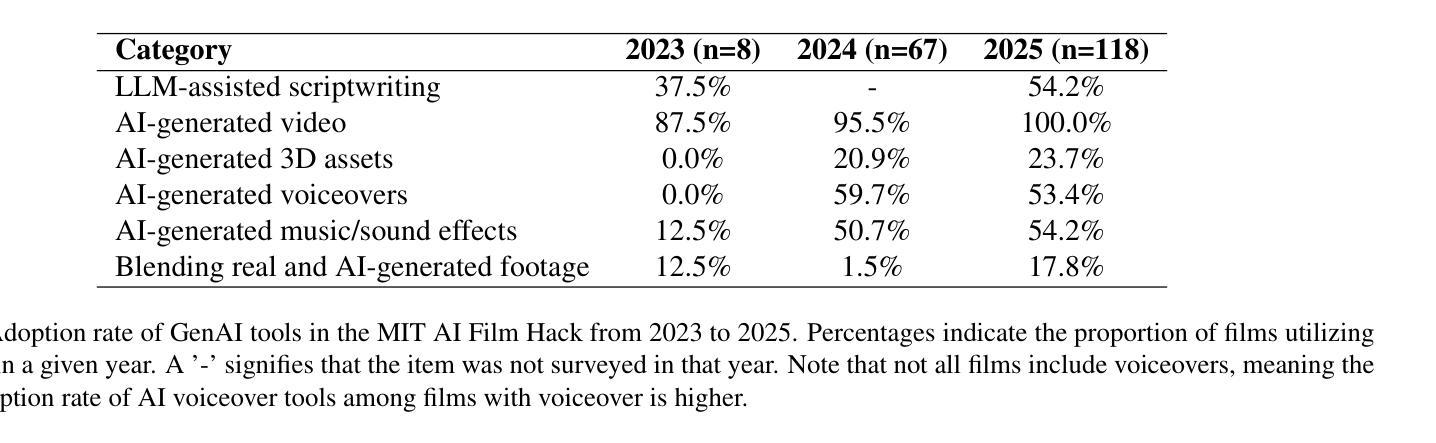
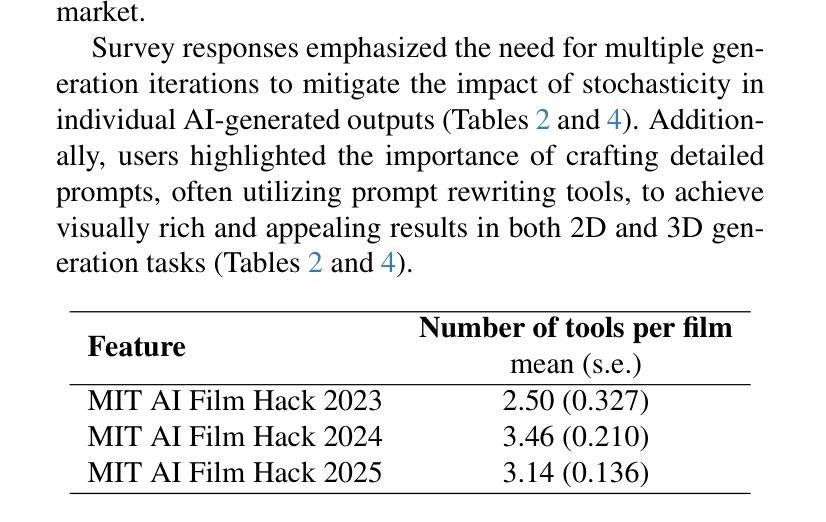
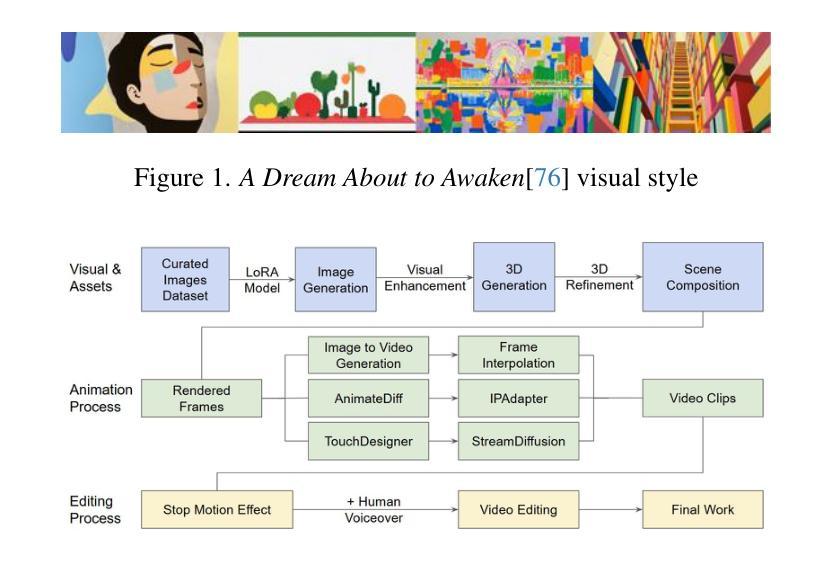
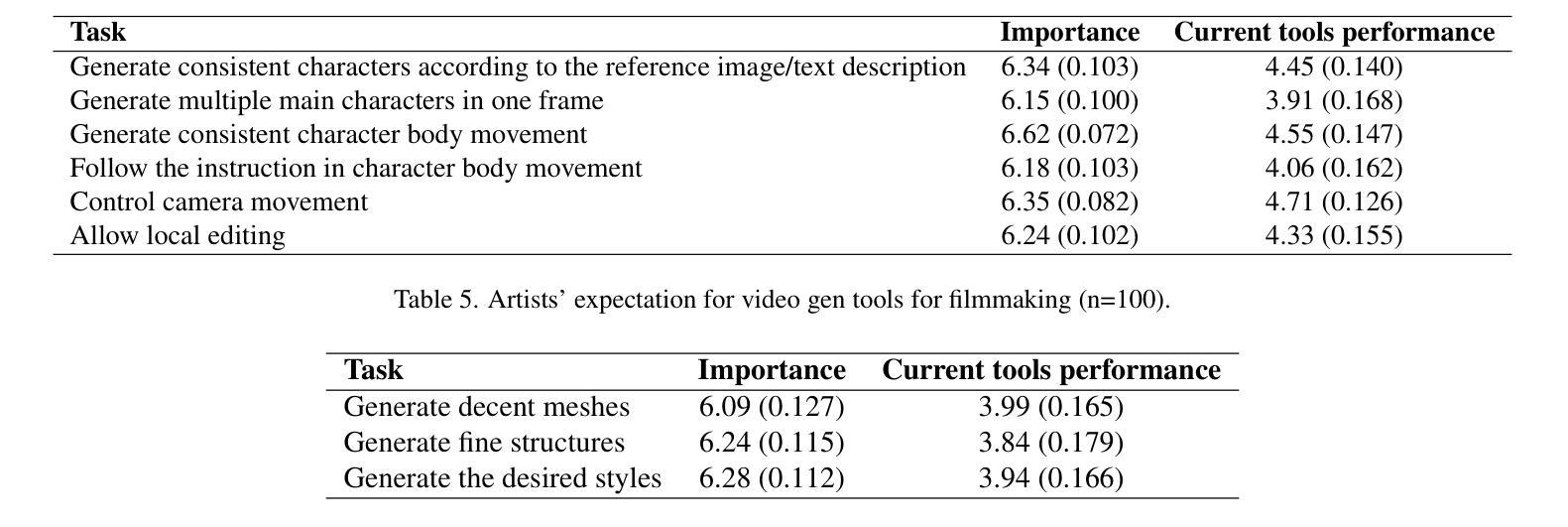
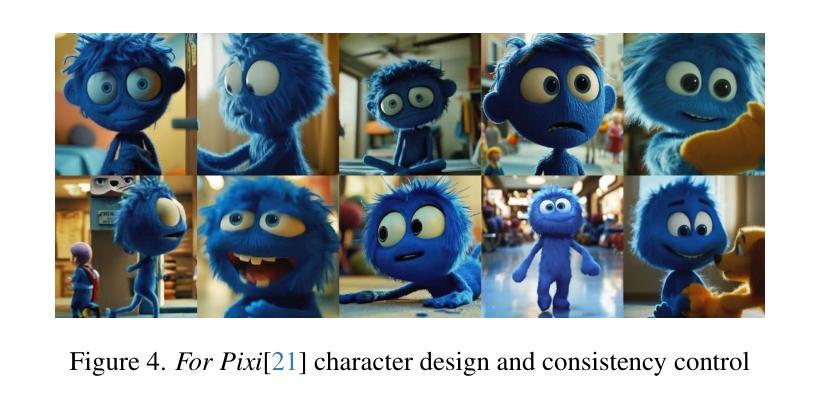
E-3DGS: Gaussian Splatting with Exposure and Motion Events
Authors:Xiaoting Yin, Hao Shi, Yuhan Bao, Zhenshan Bing, Yiyi Liao, Kailun Yang, Kaiwei Wang
Achieving 3D reconstruction from images captured under optimal conditions has been extensively studied in the vision and imaging fields. However, in real-world scenarios, challenges such as motion blur and insufficient illumination often limit the performance of standard frame-based cameras in delivering high-quality images. To address these limitations, we incorporate a transmittance adjustment device at the hardware level, enabling event cameras to capture both motion and exposure events for diverse 3D reconstruction scenarios. Motion events (triggered by camera or object movement) are collected in fast-motion scenarios when the device is inactive, while exposure events (generated through controlled camera exposure) are captured during slower motion to reconstruct grayscale images for high-quality training and optimization of event-based 3D Gaussian Splatting (3DGS). Our framework supports three modes: High-Quality Reconstruction using exposure events, Fast Reconstruction relying on motion events, and Balanced Hybrid optimizing with initial exposure events followed by high-speed motion events. On the EventNeRF dataset, we demonstrate that exposure events significantly improve fine detail reconstruction compared to motion events and outperform frame-based cameras under challenging conditions such as low illumination and overexposure. Furthermore, we introduce EME-3D, a real-world 3D dataset with exposure events, motion events, camera calibration parameters, and sparse point clouds. Our method achieves faster and higher-quality reconstruction than event-based NeRF and is more cost-effective than methods combining event and RGB data. E-3DGS sets a new benchmark for event-based 3D reconstruction with robust performance in challenging conditions and lower hardware demands. The source code and dataset will be available at https://github.com/MasterHow/E-3DGS.
在视觉和成像领域,从在最佳条件下拍摄的照片进行三维重建已经得到了广泛的研究。然而,在现实场景中,运动模糊和照明不足等挑战往往限制了基于标准帧的相机在提供高质量图像方面的性能。为了解决这些局限性,我们在硬件层面加入了一个透射率调整设备,使得事件相机能够捕捉运动和曝光事件,适用于多种三维重建场景。当设备处于非活动状态时,在快速运动场景中收集运动事件(由相机或物体运动触发),而曝光事件(通过控制相机曝光产生)则在较慢的运动过程中被捕捉,以重建灰度图像,用于基于事件的三维高斯展布(3DGS)的高质量训练和优化。我们的框架支持三种模式:使用曝光事件进行高质量重建、依赖运动事件的快速重建,以及优化平衡混合,先使用初始曝光事件然后是高速运动事件。在EventNeRF数据集上,我们证明与运动事件相比,曝光事件能显著改善精细细节的重建,并且在低光照和过度曝光等具有挑战性的条件下优于基于帧的相机。此外,我们还引入了EME-3D,这是一个包含曝光事件、运动事件、相机校准参数和稀疏点云的真实世界三维数据集。我们的方法实现了比基于事件NeRF更快、更高质量的重建,并且比结合事件和RGB数据的方法更具成本效益。E-3DGS为基于事件的3D重建设定了一个新的基准,其在具有挑战性的条件下的性能稳健且硬件要求较低。源代码和数据集将在https://github.com/MasterHow/E-3DGS上提供。
论文及项目相关链接
PDF Accepted to Applied Optics (AO). The source code and dataset will be available at https://github.com/MasterHow/E-3DGS
Summary
本研究解决了真实环境下标准帧相机面临运动模糊和照明不足等挑战的问题。通过硬件层面的透射调整装置,事件相机能够捕捉运动和曝光事件,用于多种3D重建场景。提出一种新型事件基础3D高斯Splatting(E-3DGS)方法,包含高质量重建、快速重建和平衡混合优化三种模式。在EventNeRF数据集上验证,曝光事件在精细细节重建上显著优于运动事件,且在低光照和过度曝光等挑战条件下表现优于帧相机。同时引入EME-3D数据集并设置新基准,实现快速且高质量重建。
Key Takeaways
- 面对真实环境中运动模糊和照明不足的挑战,传统帧相机性能受限。
- 通过硬件透射调整装置,事件相机能够捕捉运动和曝光事件,为多样化3D重建提供数据。
- 引入新型事件基础3D高斯Splatting(E-3DGS)方法,包含多种模式以适应不同需求。
- 在EventNeRF数据集上验证,曝光事件在精细细节重建上优于运动事件。
- E-3DGS在挑战条件下(如低光照和过度曝光)表现优于其他方法。
- 引入EME-3D数据集,为事件相机3D重建提供真实世界数据。
点此查看论文截图
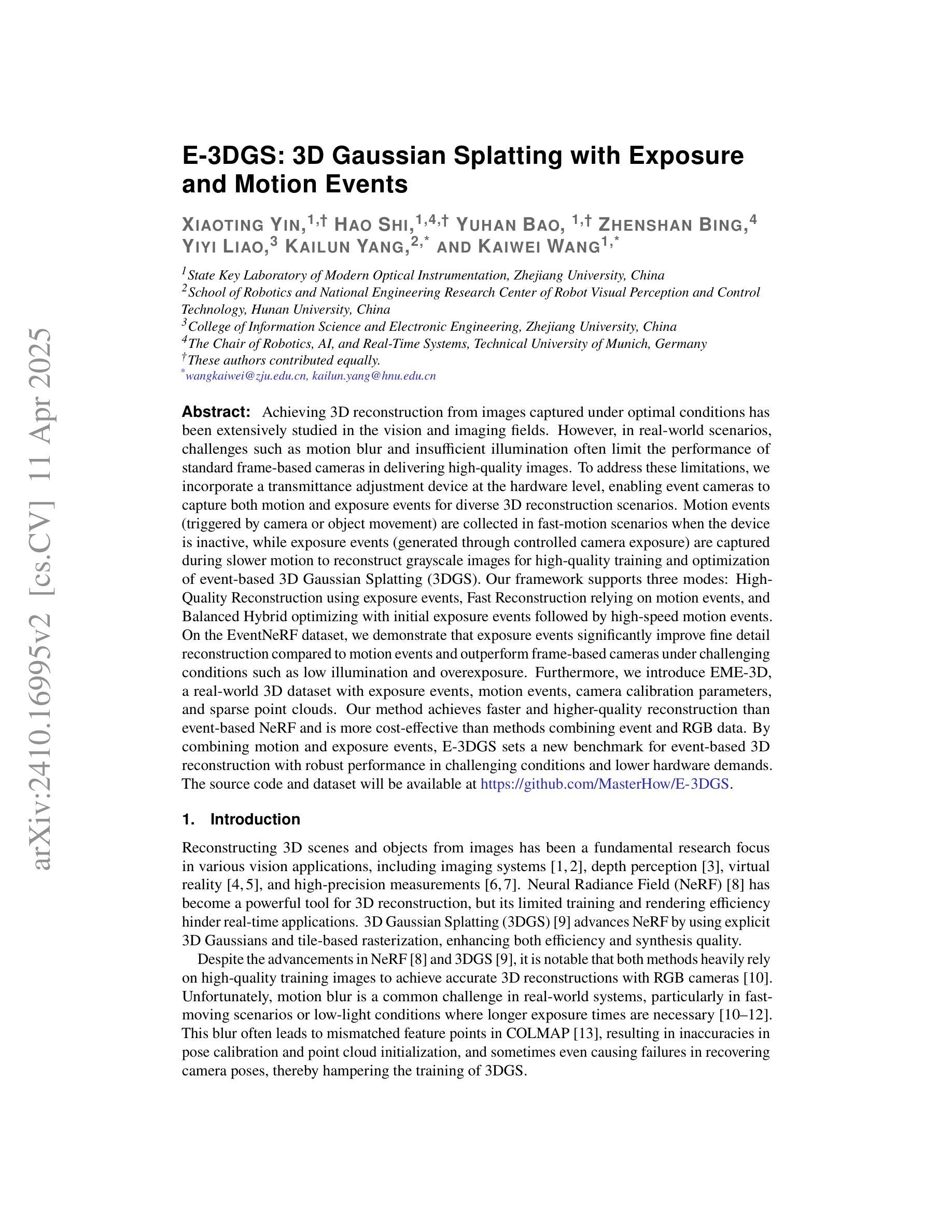
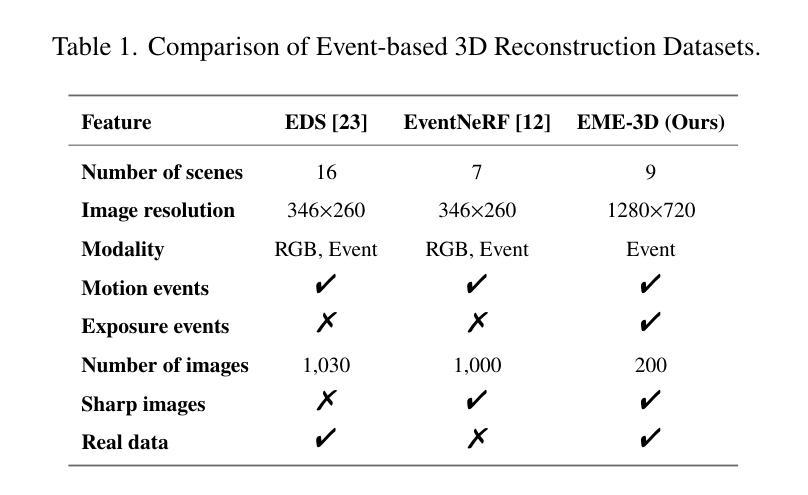
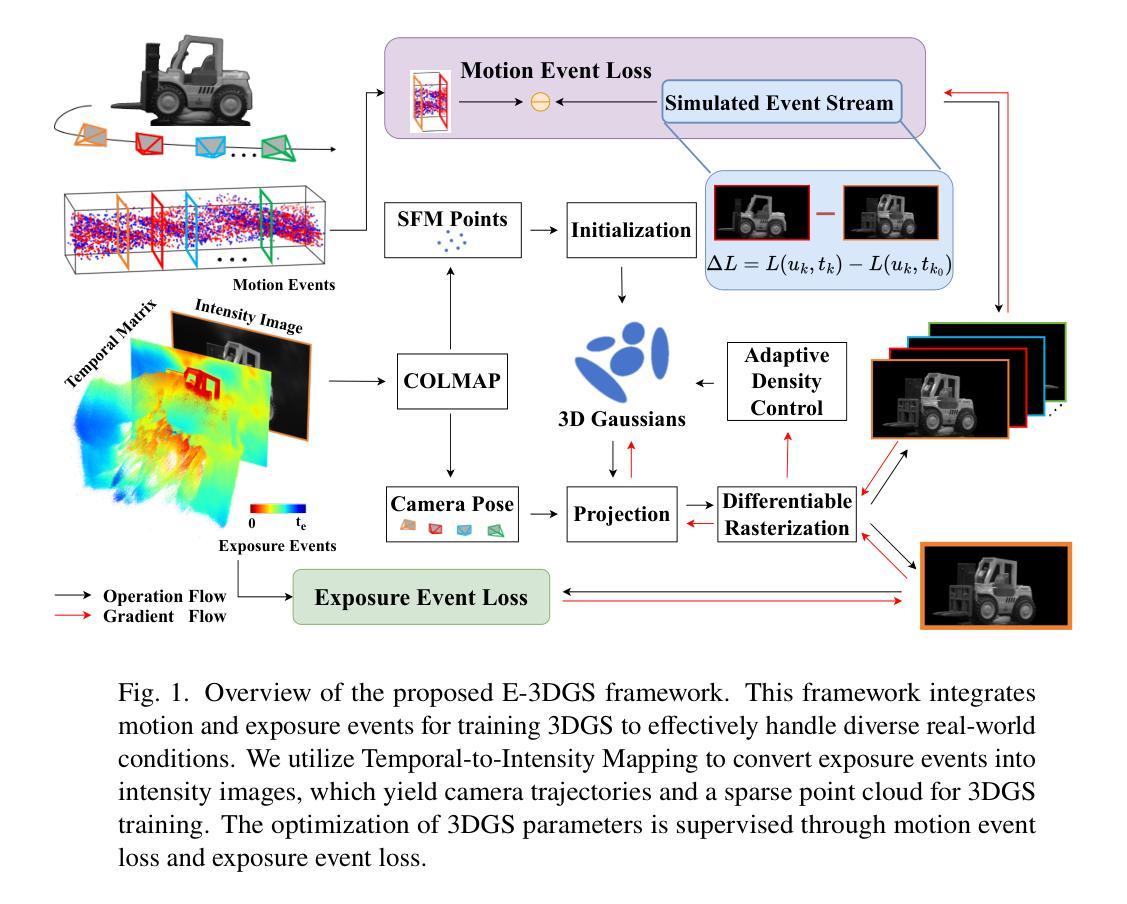